![]() |
![]() |
![]() |
Monday Sep 1st 09:00-18:00
BIRTE: Business Intelligence for the Real-Time Enterprise
Location: Diamond 1
Chair: Umesh Dayal, Nesime Tatbul

Business Intelligence for the Real-Time Enterprise (BIRTE 2014)
Castellanos Malu G (Hewlett Packard),Dayal Umeshwar (Hewlett Packard)
Data4U: Bringing the Value of Big Data to Users
Location: Diamond 2
Chair: Rada Chirkova, NC State University, USA;Jun Yang, Duke University, USA;

First Workshop on Bringing the Value of Big Data to Users (Data4U 2014)First Workshop on Bringing the Value of Big Data to Users (Data4U 2014)
Rada Chirkova (North Carolina State University), Jun Yang (Duke University)
IMDM: In-Memory Data Management and Analytics
Location: Diamond 3
Chair: Justin Levandoski, Microsoft Research;Andy Pavlo, Carnegie Mellon University;

2nd International Workshop on In-Memory Data Management and Analytics (IMDM 2014)
Justin Levandoski (Microsoft Research),Andy Pavlo (Carnegie Mellon University),Thomas Neumann (Technische Universität München), Arun Jagatheesan (University of California)
ADMS: Accelerating Data Management Systems
Location: Diamond 4

Fifth International Workshop on Accelerating Data Management Systems Using Modern Processor and Storage Architectures (ADMS 2014)
Rajesh Bordawekar, IBM
PhD Workshop: PhD Workshop
Location: Diamond 5
Chair: Erich Neuhold, the University of Vienna;Yunyao Li, IBM Research - Almaden;

PhD Workshop
Tamer Özsu (University of Waterloo),Katerina Tzompanaki (University Paris Sud),Martin Boissier (Hasso Plattner Insitute),Vivek Shah (University of Copenhagen),Marcus Leich (TU Berlin),Sobhan Badiozamany (Uppsala University),Ying Yang (SUNY (University at Buffalo),Xiang Ji (New Jersey Institute of Technology)
Monday Sep 1st 12:15-13:45
Sep 1st Lunch
Location: Crystal
Sep 1st Lunch
Monday Sep 1st 19:00-21:00
Welcome Reception
Location: Crystal
Chair: Welcome Reception Chair
Welcome Reception
Tuesday Sep 2nd 08:30-10:00
Industrial Keynote: Hasso Plattner; Academic Keynote: Volker Markl
Location: Crystal
Chair: Aoying Zhou


The Impact of Columnar In-Memory Databases on Enterprise Systems
Hasso Plattner, SAP
Five years ago I proposed a common database approach for transaction processing and analytical systems using a columnar in-memory database, disputing the common belief that column stores are not suitable for transactional workloads. Today, the concept has been widely adopted in academia and industry and it is proven that it is feasible to run analytical queries on large data sets directly on a redundancy-free schema, eliminating the need to maintain pre-built aggregate tables during data entry transactions. The resulting reduction in transaction complexity leads to a dramatic simplification of data models and applications, redefining the way we build enterprise systems. First analyses of productive applications adopting this concept confirm that system architectures enabled by in-memory column stores are conceptually superior for business transaction processing compared to row-based approaches. Additionally, our analyses show a shift of enterprise workloads to even more read-oriented processing due to the elimination of updates of transaction-maintained aggregates.
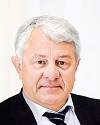
Bio: Prof. Dr. h.c. mult. Hasso Plattner is one of the co-founders of SAP AG and has been Chairman of the Supervisory Board since May 2003. In this role and as Chief Software Advisor, he concentrates on defining the medium- and longterm technology strategy and direction of SAP. He also heads the Technology Committee of the SAP Supervisory Board. Hasso Plattner received his Master¡¯s Degree in Communications Engineering from the University of Karlsruhe. In 1990, the University of Saarbr¨¹cken awarded him an honorary doctorate and in 1994, he was granted an honorary full professorship. In 1997, as chairman of SAP America, Inc., co-chairman of SAP and the chief architect of SAP R/3, Hasso Plattner received the Information Technology Leadership Award for Global Integration as part of the Computerworld Smithsonian Awards Program. In 1998, he was inducted into the German Hall of Fame. In 2002, Hasso Plattner was appointed Honorary Doctor, and in 2004 Honorary Professor by the University of Potsdam. Hasso Plattner also founded the Hasso Plattner Institute (HPI) for IT Systems Engineering at the University of Potsdam in 1998 with the largest single private contribution to a university ever made in Germany. Through his continuing financial support, he is helping the HPI in its efforts to become a center for the education of world-class software specialists.


Breaking the Chains: On Declarative Data Analysis and Data Independence in the Big Data Era
Volker Markl, Technische Universität Berlin
Data management research, systems, and technologies have drastically improved the availability of data analysis capabilities, particularly for non-experts, due in part to low-en-try barriers and reduced ownership costs (e.g., for data management infrastructures and applications). Major reasons for the widespread success of database systems and today’s multi-billion dollar data management market include data independence, separating physical representation and storage from the actual information, and declarative languages, separating the program specification from its intended execution environment. In contrast, today’s big data solutions do not offer data independence and declarative specification. As a result, big data technologies are mostly employed in newly-established companies with IT-savvy employees or in large well-established companies with big IT departments. We argue that current big data solutions will continue to fall short of widespread adoption, due to usability problems, despite the fact that in-situ data analytics technologies achieve a good degree of schema independence. In particular, we consider the lack of a declarative specification to be a major road-block, contributing to the scarcity in available data scientists available and limiting the application of big data to the IT-savvy industries. In particular, data scientists currently have to spend a lot of time on tuning their data analysis programs for specific data characteristics and a specific execution environment. We believe that the research com-munity needs to bring the powerful concepts of declarative specification to current data analysis systems, in order to achieve the broad big data technology adoption and effectively deliver the promise that novel big data technologies offer.
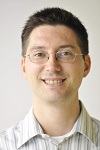
Bio: Volker Markl is a Full Professor and Chair of the Database Systems and Information Management (DIMA) group at the Technische Universitat Berlin (TU Berlin) as well as an adjunct status-only professor at the University of Toronto. Earlier in his career, Dr. Markl lead a research group at FORWISS, the Bavarian Research Center for Knowledge-based Systems in Munich, Germany, and was a Research Staff member & Project Leader at the IBM Almaden Research Center in San Jose, California, USA. Dr. Markl has published numerous research papers on indexing, query optimization, lightweight information integration, and scalable data processing. He holds 7 patents, has transferred technology into several commercial products, and advises several companies and startups. He has been speaker and principal investigator of the Stratosphere research project that resulted in the "Apache Flink" big data analytics system. Dr. Markl currently serves as the secretary of the VLDB Endowment and was recently elected as one of Germany's leading "digital minds" (Digitale Köpfe) by the German Informatics Society (GI).
Tuesday Sep 2nd 10:30-12:15
Papers : Graph Data II
Location: Diamond 1
Chair: Jeffrey Yu


Simple, Fast, and Scalable Reachability Oracle
Ruoming Jin* (Kent State University), Guan Wang (Kent State University)
A reachability oracle (or hop labeling) assigns each vertex v two sets of vertices: Lout(v) and Lin(v), such that u reaches v iff $L_{out}(u) \cap L_{in}(v) \neq \emptyset$. Despite their simplicity and elegance,reachability oracles have failed to achieve efficiency in more than ten years since their introduction: the main problem is high constructioncost, which stems from a set-cover framework and theneed to materialize transitive closure. In this paper, we present two simple and efficient labeling algorithms, Hierarchical-Labeling and Distribution-Labeling, which can work onmassive real-world graphs:their construction time is an order of magnitude faster than the setcover based labeling approach, and transitive closure materialization is not needed. On large graphs, their index sizes and their query performance can now beat the state-of-the-art transitive closurecompression and online search approaches.


Reachability Querying: An Independent Permutation Labeling Approach
Hao WEI* (The Chinese University f HK), Jeffrey Xu Yu (The Chinese University of Hong Kong), Can Lu (The Chinese University of Hong Kong), Ruoming Jin (Kent State University)
Reachability query is a fundamental graph operation which answers whether a vertex can reach another vertex over a large directed graph G with n vertices and m edges, and has been extensively studied. In the literature, all the approaches compute a label for every vertex in a graph G by index construction offline. The query time for answering reachability queries online is affected by the quality of the labels computed in index construction. The three main costs are the index construction time, the index size, and the query time. Some of the up-to-date approaches can answer reachability queries efficiently, but spend non-linear time to construct an index. Some of the up-to-date approaches construct an index in linear time and space, but may need to depth-first search G at run-time in O(n + m). In this paper, as the first, we propose a new randomized labeling approach to answer reachability queries, and the randomness is by independent permutation. We conduct extensive experimental studies to compare with the up-to-date approaches using 19 large real datasets used in the existing work and synthetic datasets. We confirm the efficiency of our approach.


Toward a Distance Oracle for Billion-Node Graphs
Zichao Qi (Tsinghua University), Yanghua Xiao* (Fudan University), Bin Shao (Microsoft Research Asia), Haixun Wang (Google Research)
The emergence of real life graphs with billions of nodes poses significant challenges for managing and querying these graphs. One of the fundamental queries submitted to graphs is the shortest distance query. Online BFS (breadth-first search) and offline pre-computing pairwise shortest distances are prohibitive in time or space complexity for billion-node graphs. In this paper, we study the feasibility of building distance oracles for billion-node graphs. A distance oracle provides approximate answers to shortest distance queries by using a pre-computed data structure for the graph. Sketch-based distance oracles are good candidates because they assign each vertex a sketch of bounded size, which means they have linear space complexity. However, state-of-the-art sketch-based distance oracles lack efficiency or accuracy when dealing with big graphs. In this paper, we address the scalability and accuracy issues by focusing on optimizing the three key factors that affect the performance of distance oracles: landmark selection, distributed BFS, and answer generation. We conduct extensive experiments on both real networks and synthetic networks to show that we can build distance oracles of affordable cost and efficiently answer shortest distance queries even for billion-node graphs.


Hop Doubling Label Indexing for Point-to-Point Distance Querying on Scale-Free Networks
Minhao Jiang* (HKUST), Ada Wai-Chee Fu (Chinese University of Hong Kong), Raymond Chi-Wing Wong (Hong Kong University of Science and Technology), Yanyan Xu (CUHK)
We study the problem of point-to-point distance querying for massive scale-free graphs, which is important for numerous applications. Given a directed or undirected graph, we propose to build an index for answering such queries based on a novel hop-doubling labeling technique. We derive bounds on the index size, the computation costs and I/O costs based on the properties of unweighted scale-free graphs. We show that our method is much more efficient and effective compared to the state-of-the-art techniques, in terms of both querying time and indexing costs. Our empirical study shows that our method can handle graphs that are orders of magnitude larger than existing methods.


Path Problems in Temporal Graphs
Huanhuan Wu (CUHK), James Cheng* (CUHK), Silu Huang (CUHK), Yiping Ke (Institute of High Performance Computing), Yi Lu (CUHK), Yanyan Xu (CUHK)
Shortest path is a fundamental graph problem with numerous applications. However, the concept of classic shortest path is insufficient or even flawed in a temporal graph, as the temporal information determines the order of activities along any path. In this paper, we show the shortcomings of classic shortest path in a temporal graph, and study various concepts of ``shortest'' path for temporal graphs. Computing these temporal paths is challenging as subpaths of a ``shortest'' path may not be ``shortest'' in a temporal graph. We investigate properties of the temporal paths and propose efficient algorithms to compute them. We tested our algorithms on real world temporal graphs to verify their efficiency, and also show that temporal paths are essential for studying temporal graphs by comparing shortest paths in normal static graphs.


Finding the Cost-Optimal Path with Time Constraint over Time-Dependent Graphs
Yajun Yang* (Tianjin University), Hong Gao (Harbin Institute of Technology), Jeffrey Xu Yu (The Chinese University of Hong Kong), Jianzhong Li (Harbin Institute of Technology)
Shortest path query is an important problem and has been well studied in static graphs. However, in practice, the costs of edges in graphs always change over time. We call such graphs as time-dependent graphs. In this paper, we study how to find a cost-optimal path with time constraint in time-dependent graphs. Most existing works regarding the Time-Dependent Shortest Path (TDSP) problem focus on finding a shortest path with the minimum travel time. All these works are based on the following fact: the earliest arrival time at a vertex v can be derived from the earliest arrival time at v's neighbors. Unfortunately, this fact does not hold for our problem. In this paper, we propose a novel algorithm to compute a cost-optimal path with time constraint in time-dependent graphs. We show that the time and space complexities of our algorithm are O(kn log n + mk) and O((n + m)k) respectively. We confirm the effectiveness and efficiency of our algorithm through conducting experiments on real datasets with synthetic cost.
Papers : OLAP
Location: Diamond 2
Chair: Chris Re


Counting and Sampling Triangles from a Graph Stream
Pavan Aduri (Iowa State University), Kanat Tangwongsan* (IBM T. J. Watson Research Center), Srikanta Tirthapura (Iowa State University), Kun-Lung Wu (IBM T.J. Watson Research Center)
This paper presents a new space-efficient algorithm for counting and sampling triangles--and more generally, constant-sized cliques--in a massive graph whose edges arrive as a stream. Compared to prior work, our algorithm yields significant improvements in the space and time complexity for these fundamental problems. Our algorithm is simple to implement and has very good practical performance on large graphs.


A Sampling Algebra for Aggregate Estimation
Supriya Nirkhiwale* (University of Florida), Alin Dobra (University of Florida), Christopher Jermaine (Rice University)
As of 2005, sampling has been incorporated in all major database systems. While efficient sampling techniques are easily realizable,determining the accuracy of an estimate obtained from the sample isstill an unresolved problem. In this paper, we present a theoreticalframework that allows an elegant treatment of the problem. We base ourwork on generalized uniform sampling (GUS), a class of samplingmethods that subsumes a wide variety of sampling techniques. Weintroduce a key notion of equivalence that allows GUS samplingoperators to commute with selection and join, and derivation ofconfidence intervals. We illustrate the theory through extensiveexamples and give indications on how to use it to provide meaningful estimates indatabase systems.


DimmWitted: A Study of Main-Memory Statistical Analytics
Ce Zhang* (University of Wisconsin-Madison), Chris Re (Stanford)
We perform the first study of the tradeoff space of access methods and replication to support statistical analytics using first-order methods executed in the main memory of a Non-Uniform Memory Access (NUMA) machine. Statistical analytics systems differ from conventional SQL-analytics in the amount and types of memory incoherence that they can tolerate. Our goal is to understand tradeoffs in accessing the data in row- or column-order and at what granularity one should share the model and data for a statistical task. We study this new tradeoff space and discover that there are tradeoffs between hardware and statistical efficiency. We argue that our tradeoff study may provide valuable information for designers of analytics engines: for each system we consider, our prototype engine can run at least one popular task at least 100x faster. We conduct our study across five architectures using popular models, including SVMs, logistic regression, Gibbs sampling, and neural networks.


Instant Loading for Main Memory Databases
Tobias Mühlbauer* (Technische Universität München), Wolf Roediger (TUM), Robert Seilbeck (Technische Universität München), Angelika Reiser (Technische Universität München), Alfons Kemper (Technische Universität München), Thomas Neumann (Technische Universität München)
eScience and big data analytics applications are facing the challenge of efficiently evaluating complex queries over vast amounts of structured text data archived in network storage solutions. To analyze such data in traditional disk-based database systems, it needs to be bulk loaded, an operation whose performance largely depends on the wire speed of the data source and the speed of the data sink, i.e., the disk. As the speed of network adapters and disks has stagnated in the past, loading has become a major bottleneck. The delays it is causing are now ubiquitous as text formats are a preferred storage format for reasons of portability.But the game has changed: Ever increasing main memory capacities have fostered the development of in-memory database systems and very fast network infrastructures are on the verge of becoming economical. While hardware limitations for fast loading have disappeared, current approaches for main memory databases fail to saturate the now available wire speeds of tens of Gbit/s. With Instant Loading, we contribute a novel CSV loading approach that allows scalable bulk loading at wire speed. This is achieved by optimizing all phases of loading for modern super-scalar multi-core CPUs. Large main memory capacities and Instant Loading thereby facilitate a very efficient data staging processing model consisting of instantaneous load-work-unload cycles across data archives on a single node. Once data is loaded, updates and queries are efficiently processed with the flexibility, security, and high performance of relational main memory databases.


The Case for Personal Data-Driven Decision Making
Jennie Duggan*, MIT
Data-driven decision making (D3M) has shown great promise in professional pursuits such as business and government. Here, policymakers collect and analyze data to make their operations more efficient and equitable. Progress in bringing the benefits of D3M to everyday life has been slow. For example, a student asks, "If I pursue an undergraduate degree at this university, what are my expected lifetime earnings?". Presently there is no principled way to search for this, because an accurate answer depends on the student and school. Such queries are personalized, winnowing down large datasets for specific circumstances, rather than applying well-defined predicates. They predict decision outcomes by extrapolating from relevant examples. This vision paper introduces a new approach to D3M that is designed to empower the individual to make informed choices. Here, we highlight research opportunities for the data management community arising from this proposal.
Industrial : Joins
Location: Diamond 3
Chair: Benny Kimelfeld


Joins on Encoded and Partitioned Data
Jae-Gil Lee* (KAIST)*,Gopi Attaluri (IBM Software Group),Ronald Barber (IBM Almaden Research Center),Naresh Chainani (IBM Software Group),Oliver Draese (IBM Software Group),Frederick Ho (IBM Informix),Stratos Idreos (Harvard University),Min-Soo Kim (DGIST),Sam Lightstone (IBM Software Group),Guy Lohman (IBM Almaden Research Center),Konstantinos Morfonios (Oracle),Keshava Murthy (IBM Informix),Ippokratis Pandis (IBM Almaden),Lin Qiao (LinkedIn),Vijayshankar Raman (IBM Almaden Research Center),Vincent Kulandai Samy (IBM Almaden Research Center),Richard Sidle (IBM Almaden Research Center),Knut Stolze (IBM Software Group),Liping Zhang (IBM Software Group)
Compression has historically been used to reduce the cost of storage, I/Os from that storage, and buffer pool utilization, at the expense of the CPU required to decompress data every time it is queried. However, significant additional CPU efficiencies can be achieved by deferring decompression as late in query processing as possible and performing query processing operations directly on the still-compressed data. In this paper, we investigate the benefits and challenges of performing joins on compressed (or encoded) data. We demonstrate the benefit of independently optimizing the compression scheme of each join column, even though join predicates relating values from multiple columns may require translation of the encoding of one join column into the encoding of the other. We also show the benefit of compressing "payload" data other than the join columns "on the fly," to minimize the size of hash tables used in the join. By partitioning the domain of each column and defining separate dictionaries for each partition, we can achieve even better overall compression as well as increased flexibility in dealing with new values introduced by updates. Instead of decompressing both join columns participating in a join to resolve their different compression schemes, our system performs a light-weight mapping of only qualifying rows from one of the join columns to the encoding space of the other at run time. Consequently, join predicates can be applied directly on the compressed data. We call this procedure encoding translation. Two alternatives of encoding translation are developed and compared in the paper. We provide a comprehensive evaluation of these alternatives using product implementations of each on the TPC-H data set, and demonstrate that performing joins on encoded and partitioned data achieves both superior performance and excellent compression.


Of Snowstorms and Bushy Trees
Rafi Ahmed* (Oracle)*,Rajkumar Sen (Oracle USA),Meikel Poess (Oracle),Sunil Chakkappen (Oracle USA)
Many workloads for analytical processing in commercial RDBMSs are dominated by snowstorm queries, which are characterized by references to multiple large fact tables and their associated smaller dimension tables. This paper describes a technique for bushy join tree optimization for snowstorm queries in Oracle database system. This technique generates bushy join trees containing subtrees that produce substantially reduced sets of rows and, therefore, their joins with other subtrees are generally much more efficient than joins in the left-deep trees. The generation of bushy join trees within an existing commercial physical optimizer requires extensive changes to the optimizer. Further, the optimizer will have to consider a large join permutation search space to generate efficient bushy join trees. The novelty of the approach is that bushy join trees can be generated outside the physical optimizer using logical query transformation that explores a considerably pruned search space. The paper describes an algorithm for generating optimal bushy join trees for snowstorm queries using an existing query transformation framework. It also presents performance results for this optimization, which show significant execution time improvements.


Execution Primitives for Scalable Joins and Aggregations in Map Reduce
Srinivas Vemuri (Link)*,Rui Liu (LinkedIn)
Analytics on Big Data is critical to derive business insights and drive innovation in today's Internet companies. Such analytics involve complex computations on large datasets, and are typically performed on MapReduce based frameworks such as Hive and Pig. However, in our experience, these systems are still quite limited in performing at scale. In particular, calculations that involve complex joins and aggregations, e.g. statistical calculations, scale poorly on these systems. In this paper we propose novel primitives for scaling such calculations. We propose a new data model for organizing datasets into calculation data units that are organized based on user-defined cost functions. We propose new operators that take advantage of these organized data units to significantly speed up joins and aggregations. Finally, we propose strategies for dividing the aggregation load uniformly across worker processes that are very effective in avoiding skews and reducing (or in some cases even removing) the associated overheads. We have implemented all our proposed primitives in a framework called Rubix, which has been in production at LinkedIn for nearly a year. Rubix powers several applications and processes TBs of data each day. We have seen remarkable improvements in speed and cost of complex calculations due to these primitives.


Advanced Join Strategies for Large-Scale Distributed Computation
Nico Bruno* (Microsoft)*,YONGCHUL KWON (Microsoft),Ming-Chuan Wu (Microsoft)
Companies providing cloud-scale data services have increasing needs to store and analyze massive data sets (e.g., search logs, click streams, and web graph data). For cost and performance reasons, processing is typically done on large clusters of thousands of commodity machines by using high level scripting languages. In the recent past, there has been significant progress in adapting well-known techniques from traditional relational DBMSs to this new scenario. However, important challenges remain open. In this paper we study the very common join operation, discuss some unique challenges in the large-scale distributed scenario, and explain how to efficiently and robustly process joins in a distributed way. Specifically, we introduce novel execution strategies that leverage opportunities not available in centralized scenarios, and others that robustly handle data skew. We report experimental validations of our approaches on Scope production clusters, which power the Applications and Services Group at Microsoft.
Papers : MapReduce
Location: Diamond 4
Chair: Kyuseok Shim


Multi-Query Optimization in MapReduce Framework
Guoping Wang* (NUS), Chee-Yong Chan (National University of Singapore)
MapReduce has recently emerged as a new paradigm for large-scale data analysis due to its high scalability, fine-grained fault tolerance and easy programming model. Since different jobs often share similar work (e.g., several jobs scan the same input file or produce the same map output),there are many opportunities to optimize the performance for a batch of jobs. In this paper, we propose two new techniques for multi-job optimization in the MapReduce framework. The first is a generalized grouping technique (which generalizes the recently proposed MRShare technique) that merges multiple jobs into a single job thereby enabling the merged jobs to share both the scan of the input file as well as the communication of the common map output. The second is a materialization technique that enables multiple jobs to share both the scan of the input file as well as the communication of the common map output via partial materialization of the map output of some jobs (in the map and/orreduce phase). Our second contribution is the proposal of a new optimization algorithm that given an input batch of jobs, produces an optimal plan by a judicious partitioning of the jobs into groups and an optimal assignment of the processing technique to each group. Our experimental results on Hadoop demonstrate that our new approach significantly outperforms the state-of-the-art technique, MRShare, by up to 107%.


Optimization for iterative queries on MapReduce
Makoto Onizuka* (NTT), Hiroyuki Kato (National Institute of Informatics), Soichiro Hidaka (National Institute of Informatics), Keisuke Nakano (University of Electro-Communications), Zhenjiang Hu (National Institute of Informatics)
We propose OptIQ, a query optimization approach for iterative queries in distributed environment. OptIQ removes redundant computations among different iterations by extending the traditional techniques of view materialization and incremental view evaluation. First, OptIQ decomposes iterative queries into invariant and variant views, and materializes the former view. Redundant computations are removed by reusing the materialized view among iterations. Second, OptIQ incrementally evaluates the variant view, so that redundant computations are removed by skipping the evaluation on converged tuples in the variant view. We verify the effectiveness of OptIQ through the queries of PageRank and k-means clustering on real datasets. The results show that OptIQ achieves high efficiency, up to five times faster than is possible without removing the redundant computations among iterations.


Parallel Computation of Skyline and Reverse Skyline Queries Using MapReduce
Yoonjae Park (Seoul National University), Jun-Ki Min (Korea Univ. of Tech. & Edu.), Kyuseok Shim* (Seoul National University)
The skyline operator and its variants such as dynamic skyline and reverse skyline operators have attracted considerable attention recently due to their broad applications. However, computations of such operators are challenging today since there is an increasing trend of applications to deal with big data. For such data-intensive applications, the MapReduce framework has been widely used recently.In this paper, we propose efficient parallel algorithms for processing the skyline and its variants using MapReduce. We first build histograms to effectively prune out non-skyline (non-reverse skyline) points in advance. We next partition data based on the regions divided by the histograms and compute candidate (reverse) skyline points for each region independently using MapReduce. Finally, we check whether each candidate point is actually a (reverse) skyline point or not in every region independently. Our performance study confirms the effectiveness and scalability of the proposed algorithms.


ClusterJoin: A Similarity Joins Framework using Map-Reduce
Akash Das Sarma (Stanford University), Yeye He* (Microsoft Research), Surajit Chaudhuri (Microsoft Research)
Similarity join is the problem of finding pairs of records with similarity score greater than some threshold. In this paper we study the problem of scaling up similarity join for different metric distance functions using Map Reduce. We propose a ClusterJoin framework that partitions the data space based on the underlying data distribution, and distributes each record to partitions in which they may produce join results based on the distance threshold. We design a set of strong candidate filters specific to different distance functions using a novel bisector-based framework, so that each record only needs to be distributed to a small number of partitions while still guaranteeing correctness. To address data skewness, which is common for high dimensional data, we further develop a dynamic load balancing scheme using sampling, which provides strong probabilistic guarantees on the size of partitions, and greatly improves scalability. Experimental evaluation using real data sets shows that our approach is considerably more scalable compared to state-of-the-art algorithms, especially for high dimensional data with low distance thresholds.


Hybrid Parallelization Strategies for Large-Scale Machine Learning in SystemML
Matthias Boehm* (IBM Research - Almaden), Shirish Tatikonda (IBM Research), Berthold Reinwald (IBM Research - Almaden), Prithviraj Sen (IBM Research - Almaden), Yuanyuan Tian (IBM Almaden Research Center), Doug Burdick (IBM Research - Almaden), Shivakumar Vaithyanathan (IBM Research - Almaden)
SystemML aims at declarative, large-scale machine learning (ML) on top of MapReduce, where high-level ML scripts with R-like syntax are compiled to programs of MR jobs. The declarative specification of ML algorithms enables---in contrast to existing large-scale machine learning libraries---automatic optimization. SystemML's primary focus is on data parallelism but many ML algorithms inherently exhibit opportunities for task parallelism as well. A major challenge is how to efficiently combine both types of parallelism for arbitrary ML scripts and workloads. In this paper, we present a systematic approach for combining task and data parallelism for large-scale machine learning on top of MapReduce. We employ a generic Parallel FOR construct (ParFOR) as known from high performance computing (HPC). Our core contributions are (1) complementary parallelization strategies for exploiting multi-core and cluster parallelism, as well as (2) a novel cost-based optimization framework for automatically creating optimal parallel execution plans. Experiments on a variety of use cases showed that this achieves both efficiency and scalability due to automatic adaptation to ad-hoc workloads and unknown data characteristics.


Rank Join Queries in NoSQL Databases
Nikos Ntarmos (School of Computing Science (University of Glasgow (Glasgow (UK), Ioannis Patlakas (Max-Planck Institute for Informatics), PETER TRIANTAFILLOU* (University of Glasgow)
Rank (i.e., top-k) join queries, play a key role in modern analytics tasks. However, despite their importance and unlike centralized settings, they have been completely overlooked in cloudstore NoSQL settings. We attempt to fill this gap: We contribute a suit of solutions and study their performance comprehensively. Baseline solutions are offered using SQL-like languages (like Hive and Pig), based on MapReduce jobs. We first provide solutions that are based on specialized indices, which may themselves be accessed using either MapReduce or coordinator-based strategies. The first, index-based solution adapts a popular centralized rank-join algorithm. The second index-based solution, is based on inverted indices, which are accessed with MapReduce jobs. We further contribute a novel statistical structure comprising histograms and Bloom filters. We provide (i) MapReduce algorithms showing how to build these indices and statistical structures, (ii) algorithms to allow for online updates to these indices, and (iii) query processing algorithms utilizing them. We implemented all algorithms in Hadoop (HDFS) and HBase and test them on TPC-H datasets of various scales, utilizing different queries on tables of various sizes and different score-attribute distributions. We ported our implementations to Amazon EC2 and "in-house" lab clusters of various scales. We provide performance results for three metrics: query execution time, network bandwidth consumption, and dollar-cost for query execution.
Papers : Social and Recommender Systems
Location: Diamond 5
Chair: Lei Chen


GeoScope: Online detection of GeoCorrelated Information Trends In Social Networks
Ceren Budak*), Microsoft Research), Theodore Georgiou), Divyakant Agrawal), Amr El Abbadi),)
The First Law of Geography states "Everything is related to everything else, but near things are more related than distant things". This spatial significance has implications in various applications, trend detection being one of them. In this paper we propose a new algorithmic tool, GeoScope, to detect geo-trends. GeoScope is a data streams solution that detects correlations between topics and locations in a sliding window, in addition to analyzing topics and locations independently. GeoScope offers theoretical guarantees for detecting all trending correlated pairs while requiring only sublinear space and running time. We perform various human validation tasks to demonstrate the value of GeoScope. The results show that human judges prefer GeoScope to the best performing baseline solution 4:1 in terms of the geographical significance of the presented information. As the Twitter analysis demonstrates, GeoScope successfully filters out topics without geo-intent and detects various local interests such as emergency events, political demonstrations or cultural events. Experiments on Twitter show that GeoScope has perfect recall and near-perfect precision.


Horton+: A Distributed System for Processing Declarative Reachability Queries over Partitioned Graphs
Mohamed Sarwat* (University of Minnesota), Sameh Elnikety (Microsoft Research), Yuxiong He (Microsoft Research), Mohamed Mokbel (University of Minnesota)
Horton+ is a graph query processing system that executes declarative reachability queries on a partitioned attributed multi-graph. It employs a query language, query optimizer, and a distributed execution engine. The query language expresses declarative reachability queries, and supports closures and predicates on node and edge attributes to match graph paths. We introduce three algebraic operators, select, traverse, and join, and a query is compiled into an execution plan containing these operators. As reachability queries access the graph elements in a random access pattern, the graph is therefore maintained in the main memory of a cluster of servers to reduce query execution time. We develop a distributed execution engine that processes a query plan in parallel on the graph servers. Since the query language is declarative, we build a query optimizer that uses graph statistics to estimate predicate selectivity. We experimentally evaluate the system performance on a cluster of 16 graph servers using synthetic graphs as well as a real graph from an application that uses reachability queries. The evaluation shows (1) the efficiency of the optimizer in reducing query execution time, (2) system scalability with the size of the graph and with the number of servers, and (3) the convenience of using declarative queries.


Towards Social Data Platform: Automatic Topic-focused Monitor for Twitter Stream
Rui Li* (University of Illinois), Shengjie Wang (University of Illinois at Urbana-Champaign), Kevin Chang (UIUC)
Many novel applications have been built based on analyzing tweets about specific topics. While these applications provide different kinds of analysis, they share a common task of monitoring "target" tweets from the Twitter stream for a topic. The current solution for this task tracks a set of manually selected keywords with Twitter APIs. Obviously, this manual approach has many limitations. In this paper, we propose a data platform to automatically monitor target tweets from the Twitter stream for any given topic. To monitor target tweets in an optimal and continuous way, we design Automatic Topic-focused Monitor (ATM), which iteratively 1) samples tweets from the stream and 2) selects keywords to track based on the samples. To realize ATM, we develop a tweet sampling algorithm to sample sufficient unbiased tweets with available Twitter APIs, and a keyword selection algorithm to efficiently select keywords that have a near-optimal coverage of target tweets under cost constraints. We conduct extensive experiments to show the effectiveness of ATM. E.g., ATM covers 90% of target tweets for a topic and improves the manual approach by 49%.


An efficient reconciliation algorithm for social networks
Silvio Lattanzi* (Google), Nitish Korula),)
People today typically use multiple online social networks (Facebook, Twitter, Google+, LinkedIn, etc.). Each online network represents a subset of their "real" ego-networks. An interesting and challenging problem is to reconcile these on- line networks, that is, to identify all the accounts belonging to the same individual. Besides providing a richer under- standing of social dynamics, the problem has a number of practical applications. At first sight, this problem appears algorithmically challenging. Fortunately, a small fraction of individuals explicitly link their accounts across multiple networks; our work leverages these connections to identify a very large fraction of the network. Our main contributions are to mathematically formalize the problem for the first time, and to design a simple, lo- cal, and efficient parallel algorithm to solve it. We are able to prove strong theoretical guarantees on the algorithm’s performance on well-established network models (Random Graphs, Preferential Attachment). We also experimentally confirm the effectiveness of the algorithm on synthetic and real social network data sets.


Reverse k-Ranks Query
Zhao Zhang* (ECNU), Cheqing Jin (East China Normal University), qiangqiang Kang (ECNU)
Finding matching customers for a given product based on individual user’s preference is critical for many applications, especially in e-commerce. Recently, the reverse top-k query is proposed to return a number of customers who regard a given product as one of the k most favorite products based on a linear model. Although a few "hot" products can be returned to some customers via reverse top-k query, a large proportion of products (over 90%, as our example illustrates, see Figure 2) cannot find any matching customers. Inspired by this observation, we propose a new kind of query (R-kRanks) which finds for a given product, the top-k customers whose rank for the product is highest among all customers, to ensure 100% coverage for any given product, no matter it is hot or niche. Not limited to e-commerce, the concept of customer-product can be extended to a wider range of applications, such as dating and job-hunting. Unfortunately, existing approaches for reverse top-k query cannot be used to handle R-kRanks conveniently due to infeasibility of getting enough elements for the query result. Hence, we propose three novel approaches to efficiently process R-kRanks query, including one tree-based method and two batch-pruning-based methods. Analysis of theoretical and experimental results on real and synthetic data sets illustrates the efficacy of the proposed methods.


Supporting Distributed Feed-Following Apps over Edge Devices
Badrish Chandramouli (Microsoft Research), Suman Nath (MSR), Wenchao Zhou* (Georgetown University)
In feed-following applications such as Twitter and Facebook, users (consumers) follow a large number of other users (producers) to get personalized feeds, generated by blending producers' feeds. With the proliferation of Cloud-connected smart edge devices such as smartphones, producers and consumers of many feed-following applications reside on edge devices and the Cloud. An important design goal of such applications is to minimize communication (and energy) overhead of edge devices. In this paper, we abstract distributed feed-following applications as a view maintenance problem, with the goal of optimally placing the views on edge devices and in the Cloud to minimize communication overhead between edge devices and the Cloud. The view placement problem for general network topology is NP Hard; however, we show that for the special case of Cloud-edge topology, locally optimal solutions yield a globally optimal view placement solution. Based on this powerful result, we propose view placement algorithms that are highly efficient, yet provably minimize global network cost. Compared to existing works on feed-following applications, our algorithms are more general---they support views with selection, projection, correlation (join) and arbitrary black-box operators, and can even refer to other views. We have implemented our algorithms within a distributed feed-following architecture over real smartphones and the Cloud. Experiments over real datasets indicate that our algorithms are highly scalable and orders-of-magnitude more efficient than existing strategies for optimal placement. Further, our results show that optimal placements generated by our algorithms are often several factors better than simpler schemes.
Tutorial : Causality and explanation in databases
Location: Bauhinia 1
Chair: Tutorial 1 Chair


Causality and explanation in databases
Alexandra Meliou, Sudeepa Roy, Dan Suciu
With the surge in the availability of information, there is a great demand for tools that assist users in understanding their data. While today's exploration tools rely mostly on data visualization, users often want to go deeper and understand the underlying causes of a particular observation. This tutorial surveys research on causality and explanation for data-oriented applications. We will review and summarize the research thus far into causality and explanation in the database and AI communities, giving researchers a snapshot of the current state of the art on this topic, and propose a unified framework as well as directions for future research. We will cover both the theory of causality/explanation and some applications; we also discuss the connections with other topics in database research like provenance, deletion propagation, why-not queries, and OLAP techniques.
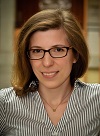
Bio: Alexandra Meliou is an Assistant Professor in the School of Computer Science at the University of Massachusetts. She received her PhD and Masters degrees from the University of California Berkeley in 2009 and 2005 respectively. She completed her postdoctoral work in 2012 at the University of Washington. She has made contributions to the areas of provenance, causality in databases, data cleaning, and sensor networks. She received a 2008 Siebel scholarship, a 2012 Sigmod best demo award, and a 2013 Google faculty award.
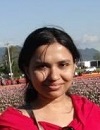
Bio: Sudeepa Roy is a Postdoctoral Researcher in Computer Science at the University of Washington. Her current research focuses on theory and applications of causality/explanations in databases. She has also worked on provenance in databases and workflows, probabilistic databases, information extraction, and crowd sourcing. During her doctoral studies at the University of Pennsylvania, she was a recipient of the Google PhD Fellowship in structured data.
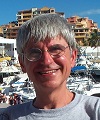
Bio: Dan Suciu is a Professor in Computer Science at the University of Washington. He made contributions to semistructured data, data privacy, probabilistic databases, and causality/explanations in databases. He is a Fellow of the ACM, holds twelve US patents, received the best paper award in SIGMOD 2000 and ICDT 2013, the ACM PODS Alberto Mendelzon Test of Time Award in 2010 and in 2012, the 10 Year Most Influential Paper Award in ICDE 2013, and is a recipient of the NSF Career Award and of an Alfred P. Sloan Fellowship. He has given past tutorials in VLDB and SIGMOD (on semistructured data and XML, and on probabilistic database).
Demo 1
Location: Pearl
Chair: Demo 1 Chair


X-LiSA: Cross-lingual Semantic Annotation
Lei Zhang*, KIT
The ever-increasing quantities of structured knowledge on the Web and the impending need of multilinguality and cross-linguality for information access pose new challenges but at the same time open up new opportunities for knowledge extraction research. In this regard, cross-lingual semantic annotation has emerged as a topic of major interest and it is essential to build tools that can link words and phrases in unstructured text in one language to resources in structured knowledge bases in any other language. In this paper, we demonstrate X-LiSA, an infrastructure for cross-lingual semantic annotation, which supports both service-oriented and user-oriented interfaces for annotating text documents and web pages in different languages using resources from Wikipedia and Linked Open Data (LOD).


Combining Interaction, Speculative Query Execution and Sampling in the DICE System
Prasanth Jayachandran (The Ohio State University),Karthik Tunga (The Ohio State University),Niranjan Kamat* (The Ohio State University),Arnab Nandi (Ohio State University)
The interactive exploration of data cubes has become a popular application, especially over large datasets. In this paper, we present DICE, a combination of a novel frontend query interface and distributed aggregation backend that enables interactive cube exploration. DICE provides a convenient, practical alternative to the typical offline cube materialization strategy by allowing the user to explore facets of the data cube, trading off accuracy for interactive response-times, by sampling the data. We consider the time spent by the user perusing the results of their current query as an opportunity to execute and cache the most likely followup queries. The frontend presents a novel intuitive interface that allows for sampling-aware aggregations, and encourages interaction via our proposed faceted model. The design of our backend is tailored towards the low-latency user interaction at the frontend, and vice-versa. We discuss the synergistic design behind both the frontend user experience and the backend architecture of DICE; and, present a demonstration that allows the user to fluidly interact with billion-tuple datasets within sub-second interactive response times.


STMaker--A System to Make Sense of Trajectory Data
Han Su* (University of Queensland),Kai Zheng (University of Queensland),KAI ZENG (UCLA),Jiamin Huang (Nanjing University),xiaofang Zhou (University of Queensland)
Widely adoption of GPS-enabled devices generates large amounts of trajectories every day. The raw trajectory data describes the movement history of moving objects by a sequence of longitude, latitude, time-stamp triples, which are nonintuitive for human to perceive the prominent features of the trajectory, such as where and how the moving object travels. In this demo, we present the STMaker system to help users make sense of individual trajectories. Given a trajectory, STMaker can automatically extract the significant semantic behavior of the trajectory, and summarize the behavior by a short human-readable text. In this paper, we first introduce the phrases of generating trajectory summarizations, and then show several real trajectory summarization cases.


Interactive Join Query Inference with JIM
Angela Bonifati (University of Lille INRIA),Radu Ciucanu* (University of Lille INRIA),Slawek Staworko (University of Lille INRIA)
Specifying join predicates may become a cumbersome task in many situations e.g., when the relations to be joined come from disparate data sources, when the values of the attributes carry little or no knowledge of metadata, or simply when the user is unfamiliar with querying formalisms. Such task is recurrent in many traditional data management applications, such as data integration, constraint inference, and database denormalization, but it is also becoming pivotal in novel crowdsourcing applications. We present Jim (Join Inference Machine), a system for interactive join specification tasks, where the user infers an n-ary join predicate by selecting tuples that are part of the join result via Boolean membership queries. The user can label tuples as positive or negative, while the system allows to identify and gray out the uninformative tuples i.e., those that do not add any information to the final learning goal. The tool also guides the user to reach her join inference goal with a minimal number of interactions.


MESA: A Map Service to Support Fuzzy Type Ahead Search over Geo-Textual Data
Yuxin Zheng* (NUS),Zhifeng Bao (University of Tasmania),Lidan Shou (Zhejiang University),Anthony Tung (National University of Singapore)
Geo-textual data are ubiquitous these days. Recent study on spatial keyword search focused on the processing of queries which retrieve objects that match certain keywords within a spatial region. To ensure effective data retrieval, various extensions were done including the tolerance of errors in keyword matching and the search-as-you-type feature using prefix matching. We present MESA, a map application to support different variants of spatial keyword query. In this demonstration, we adopt the autocompletion paradigm that generates the initial query as a prefix matching query. If there are few matching results, other variants are performed as a form of relaxation that reuses the processing done in earlier phases. The types of relaxation allowed include spatial region expansion and exact/approximate prefix/substring matching. MESA adopts the client-server architecture. It provides fuzzy type-ahead search over geo-textual data. The core of MESA is to adopt a unifying search strategy, which incrementally applies the relaxation in an appropriate order to maximize the efficiency of query processing. In addition, MESA equips a user-friendly interface to interact with users and visualize results. MESA also provides customized search to meet the needs of different users.


R3: A Real-time Route Recommendation System
Henan Wang* (Tsinghua University),Guoliang Li (Tsinghua University),Hu Huiqi (Tsinghua University),Chen Shuo (Tsinghua University),Shen Bingwen (Tsinghua University),Wu Hao (SAP Labs),Wen-syan Li (SAP)
Existing route recommendation systems have two main weaknesses. First, they usually recommend the same route for all users and cannot help control traffic jam. Second, they do not take full advantage of real-time traffic to recommend the best routes. To address these two problems, we develop a real-time route recommendation system, called R3, aiming to provide users with the real-time-traffic-aware routes. R3 recommends diverse routes for different users to alleviate the traffic pressure. R3 utilizes historical taxi driving data and real-time traffic data and integrates them together to provide users with real-time route recommendation.


PDQ: Proof-driven Query Answering over Web-based Data
Michael Benedikt* (Oxford University),Julien Leblay (Oxford University),Efthymia Tsamoura (Oxford University)
The data needed to answer queries is often available through Web-based APIs. Indeed, for a given query there may be many Web-based sources which can be used to answer it, with the sources overlapping in their vocabularies, and differing in their access restrictions (required arguments) and cost. We introduce PDQ (Proof-Driven Query Answering), a system for determining a query plan in the presence of web-based sources. It is: constraint-aware -- exploiting relationships between sources to rewrite an expensive query into a cheaper one, access-aware -- abiding by any access restrictions known in the sources, and cost-aware -- making use of any cost information that is available about services. PDQ proceeds by generating query plans from proofs that a query is answerable. We demonstrate the use of PDQ and its effectiveness in generating low-cost plans.


Data In, Fact Out: Automated Monitoring of Facts by FactWatcher
Naeemul Hassan* (University of Texas at Arlington),Afroza Sultana (UNIVERSITY OF TEXAS AT ARLINGT),You Wu (Duke University),Gensheng Zhang (University of Texas at Arlington),Chengkai Li (The University of Texas at Arlington),Jun Yang (Duke University),Cong Yu (Google Research)
Towards computational journalism, we present FactWatcher, a system that helps journalists identify data-backed, attention-seizing facts which serve as leads to news stories. FactWatcher discovers three types of facts, including situational facts, one-of-the-few facts, and prominent streaks, through a unified suite of data model, algorithm framework, and fact ranking measure. Given an append-only database, upon the arrival of a new tuple, FactWatcher monitors if the tuple triggers any new facts. Its algorithms efficiently search for facts without exhaustively testing all possible ones. Furthermore, FactWatcher provides multiple features in striving for an end-to-end system, including fact ranking, fact-to-statement translation and keyword-based fact search.


OceanST: A Distributed Analytic System for Large-scale Spatiotemporal Mobile Broadband Data
Mingxuan Yuan (Noah's Ark Lab),Fei Wang (Huawei Noah's Ark Research Lab),Dongni Ren (Hong Kong University),Ke Deng* (Noah's Ark Research Lab),Jia Zeng (Noah's Ark Lab),Yanhua Li (HUAWEI Noah's Ark Lab),Bing Ni (Huawei Noah's Ark Research Lab),Xiuqiang He (Huawei Noah’s Ark Lab)
With the increasing prevalence of versatile mobile devices and the fast deployment of broadband mobile networks, a huge volume of Mobile Broadband (MBB) data has been generated over time. The MBB data naturally contain rich information of a large number of mobile users, covering a considerable fraction of whole population nowadays, including the mobile applications they are using at different locations and time; the MBB data may present the unprecedentedly large knowledge base of human behavior which has highly recognized commercial and social value. However, the storage, management and analysis of the huge and fast growing volume of MBB data post new and significant challenges to the industrial practitioners and research community. In this demonstration, we present a new, MBB data tailored, distributed analytic system named OceanST which has addressed a series of problems and weaknesses of the existing systems, originally designed for more general purpose and capable to handle MBB data to some extent. OceanST is featured by (i) efficiently loading of ever-growing MBB data, (ii) a bunch of spatiotemporal aggregate queries and basic analysis APIs frequently found in various MBB data application scenarios, and (iii) sampling-based approximate solution with provable accuracy bound to cope with huge volume of MBB data. The demonstration will show the advantage of OceanST in a cluster of 5 machines using 3TB data.
Tuesday Sep 2nd 12:15-13:45
Sep 2nd Lunch
Location: Crystal
Sep 2nd Lunch
Tuesday Sep 2nd 13:45-15:15
Papers : Provenance and Scientific Data
Location: Diamond 1
Chair: Alexandra Meliou


A Provenance Framework for Data-Dependent Process Analysis
Daniel Deutch* (Tel Aviv University), Yuval Moskovitch (Ben-Gurion University of the Negev), Val Tannen (University of Pennsylvania)
A data-dependent process (DDP) models an application whose control flow is guided by a finite state machine, as well as by the state of an underlying database. DDPs are commonly found e.g., in e-commerce. In this paper we develop a framework supporting the use of provenance in static (temporal) analysis of possible DDP executions. Using provenance support, analysts can interactively test and explore the effect of hypothetical modifications to a DDP's state machine and/or to the underlying database. They can also extend the analysis to incorporate the propagation of annotations from meta-domains of interest, e.g., cost or access privileges. Toward this goal we note that the framework of semiring-based provenance was proven highly effective in fulfilling similar needs in the context of database queries. In this paper we consider novel constructions that generalize the semiring approach to the context of DDP analysis. These constructions address two interacting new challenges: (1) to combine provenance annotations for both information that resides in the database and information about external inputs (e.g., user choices), and (2) to finitely capture infinite process executions. We analyze our solution from theoretical and experimental perspectives, proving its effectiveness.


Multi-Tuple Deletion Propagation: Approximations and Complexity
Benny Kimelfeld* (IBM Research - Almaden), Jan Vondrak (IBM Research - Almaden), David Woodruff (IBM Research - Almaden)
This paper studies the computational complexity of the classic problem of deletion propagation in a relational database, where tuples are deleted from the base relations in order to realize a desired deletion of tuples from the view. Such an operation may result in a (sometimes unavoidable) side effect: deletion of additional tuples from the view, besides the intentionally deleted ones. The goal is to minimize the side effect. The complexity of this problem has been well studied in the case where only a single tuple is deleted from the view. However, only little is known within the more realistic scenario of multi-tuple deletion, which is the topic of this paper. The class of conjunctive queries (CQs) is among the most well studied in the literature, and we focus here on views defined by CQs that are self-join free (sjf-CQs). Our main result is a trichotomy in complexity, classifying all sjf-CQs into three categories: those for which the problem is in polynomial time, those for which the problem is NP-hard but polynomial-time approximable (by a constant-factor), and those for which even an approximation (by any factor) is NP-hard to obtain. A corollary of this trichotomy is a dichotomy in the complexity of deciding whether a side-effect-free solution exists, in the multi-tuple case. We further extend the full classification to accommodate the presence of a constant upper bound on the number of view tuples to delete, and the presence of functional dependencies. Finally, we establish (positive and negative) complexity results on approximability for the dual problem of maximizing the number of view tuples surviving (rather than minimizing the side effect incurred in) the deletion propagation.


Similarity Search for Scientific Workflows
Johannes Starlinger* (Humboldt-Universität zu Berlin), Bryan Brancotte (Université Paris-Sud), Sarah Cohen-Boulakia (Université Paris-Sud), Ulf Leser (Ulf Leser)
With the increasing popularity of scientific workflows, public repositories are gaining importance as a means to share, find, and reuse such workflows. As the sizes of these repositories grow, methods to compare the scientific workflows stored in them become a necessity, for instance, to allow duplicate detection or similarity search. Scientific workflows are complex objects, and their comparison entails a number of distinct steps from comparing atomic elements to comparison of the workflows as a whole. Various studies have implemented methods for scientific workflow comparison and came up with often contradicting conclusions upon which algorithms work best. Comparing these results is cumbersome, as the original studies mixed different approaches for different steps and used different evaluation data and metrics. We contribute to the field (i) by disecting each previous approach into an explicitly defined and comparable set of subtasks, (ii) by comparing in isolation different approaches taken at each step of scientific workflow comparison, reporting on an number of unexpected findings, (iii) by investigating how these can best be combined into aggregated measures, and (iv) by making available a gold standard of over 2000 similarity ratings contributed by 15 workflow experts on a corpus of almost 1500 workflows and re-implementations of all methods we evaluated.


RCSI: Scalable similarity search in thousand(s) of genomes
Sebastian Wandelt* (Humboldt-Universität zu Berlin), Johannes Starlinger (Humboldt-Universität zu Berlin), Marc Bux (Marc Bux), Ulf Leser (Ulf Leser)
Until recently, genomics has concentrated on comparing sequences between species. However, due to the sharply falling cost of sequencing technology, studies of populations of individuals of the same species are now feasible and promise advances in areas such as personalized medicine and treatment of genetic diseases. A core operation in such studies is read mapping, i.e., finding all parts of a set of genomes which are within edit distance k to a given query sequence (k-approximate search). To achieve sufficient speed, current algorithms solve this problem only for one to-be-searched genome and compute only approximate solutions, i.e., miss some k-approximate occurrences. We present RCSI, Referentially Compressed Search Index, which scales to thousand genomes and computes the exact answer. It exploits the fact that genomes of different individuals of the same species are highly similar by first compressing the to-be-searched genomes with respect to a reference genome. Given a query, RCSI then searches the reference and all genome-specific individual differences. We propose efficient data structures for representing compressed genomes and present algorithms for scalable compression and similarity search. We evaluate our algorithms on a set of 1092 human genomes, which amount to app. 3 TB of raw data. RCSI compresses this set by a ratio of 450:1 (26:1 including the search index) and answers similarity queries on a mid-class server in 15ms on average even for comparably large error thresholds, therein significantly outperforming other methods. Furthermore, we present a fast and adaptive heuristic for choosing the best reference sequence for referential compression, a problem that was never studied before at this scale.


$\Upsilon$-DB: Managing scientific hypotheses as uncertain data
Bernardo Gonçalves* (LNCC), Fabio Porto (LNCC)
In view of the paradigm shift that makes science ever more data-driven, we consider deterministic scientific hypotheses as uncertain data. This vision comprises a probabilistic database (p-DB) design methodology for the systematic construction and management of U-relational hypothesis databases, viz., $\Upsilon$-DBs. It introduces hypothesis management as a promising new class of applications for p-DBs. We illustrate the potential of $\Upsilon$-DB as a tool for deep predictive analytics.
Papers : Query Processing I
Location: Diamond 2
Chair: Wolfgang Lehner


Building Efficient Query Engines in a High-Level Language
Yannis Klonatos* (EPFL), Christoph Koch (EPFL), Tiark Rompf (EPFL), Hassan Chafi (Oracle Labs)
In this paper we advocate that it is time for a radical rethinking of database systems design. Developers should be able to leverage high-level programming languages without having to pay a price in efficiency. To realize our vision of abstraction without regret, we present LegoBase, a query engine written in the high-level programming language Scala. The key technique to regain efficiency is to apply generative programming: the Scala code that constitutes the query engine, despite its high-level appearance, is actually a program generator that emits specialized, low-level C code. We show how the combination of high-level and generative program- ming allows to easily implement a wide spectrum of optimizations that are difficult to achieve with existing low-level query compilers, and how it can continuously optimize the query engine. We evaluate our approach with the TPC-H benchmark and show that: (a) with all optimizations enabled, our architecture significantly outperforms a commercial in-memory database system as well as an existing query compiler, (b) these performance improvements require programming just a few hundred lines of high-level code instead of complicated low-level code that is required by exist- ing query compilers and, finally, that (c) the compilation overhead is low compared to the overall execution time, thus making our approach usable in practice for efficiently compiling query engines.


Code generation for efficient query processing in managed runtimes
Fabian Nagel* (University of Edinburgh), Gavin Bierman (Microsoft Research), Stratis Viglas (University of Edinburgh)
In this paper we examine opportunities arising from the convergence of two trends in data management: in-memory database systems (IMDBs), which have received renewed attention following the availability of affordable, very large main memory systems; and language-integrated query, which transparently integrates database queries with programming languages (thus addressing the famous 'impedance mismatch' problem). Language-integrated query not only gives application developers a more convenient way to query external data sources like IMDBs, but also to use the same querying language to query an application's in-memory collections. The latter offers further transparency to developers as the query language and all data is represented in the data model of the host programming language. However, compared to IMDBs, this additional freedom comes at a higher cost for query evaluation. Our vision is to improve in-memory query processing of application objects by introducing database technologies to managed runtimes. We focus on querying and we leverage query compilation to improve query processing on application objects. We explore different query compilation strategies and study how they improve the performance of query processing over application data. We take C# as the host programming language as it supports language-integrated query through the LINQ framework. Our techniques deliver significant performance improvements over the default LINQ implementation. Our work makes important first steps towards a future where data processing applications will commonly run on machines that can store their entire datasets in-memory, and will be written in a single programming language employing language-integrated query and IMDB-inspired runtimes to provide transparent and highly efficient querying.


Storing and Querying Tree-Structured Records in Dremel
Foto Afrati* (National Technical University of Athens), Dan Delorey (Google), Mosha Pasumansky (Google), Jeffrey Ullman (Stanford University)
We investigate nested relations, where each record has a schema. The schema is given by a hierarchical tree with nodes that are attributes with leaf attributes having values. We explore filter and aggregate queries that are given in a dialect of SQL. Complications arise because of {\em repeated} attributes, i.e., attributes that are allowed to have more than one values. We focus on a special class of queries that are processed in Dremel on column-stored data in a way that results in query processing time that is linear on the size of the relevant data, i.e., data stored in the columns that participate in the query. We formally define the data model, the query language and the algorithms for query processing in column-stored data. The concepts of repetition context and semi-flattening are introduced here that play a central role in understanding this class of queries and their algorithms.


Adaptive Query Processing on RAW Data
Manos Karpathiotakis* (EPFL), Miguel Branco (EPFL), Ioannis Alagiannis (EPFL), Anastasia Ailamaki (EPFL)
Database systems deliver impressive performance for large classes of workloads as the result of decades of research into optimizing database engines. High performance, however, is achieved at the cost of versatility. In particular, database systems only operate efficiently over loaded data, i.e., data converted from its original raw format into the system's internal data format. At the same time, data volume continues to increase exponentially and data varies increasingly, with an escalating number of new formats. The consequence is a growing impedance mismatch between the original structures holding the data in the raw files and the structures used by query engines for efficient processing. In an ideal scenario, the query engine would seamlessly adapt itself to the data and ensure efficient query processing regardless of the input data formats, optimizing itself to each instance of a file and of a query by leveraging information available at query time. Today's systems, however, force data to adapt to the query engine during data loading. This paper proposes adapting the query engine to the formats of raw data. It presents RAW, a prototype query engine which enables querying heterogeneous data sources transparently. RAW employs Just-In-Time access paths, which efficiently couple heterogeneous raw files to the query engine and reduce the overheads of traditional general-purpose scan operators. There are, however, inherent overheads with accessing raw data directly that cannot be eliminated, such as converting the raw values. Therefore, RAW also uses column shreds, ensuring that we pay these costs only for the subsets of raw data strictly needed by a query. We use RAW in a real-world scenario and achieve a two-order of magnitude speedup against the existing hand-written solution.


Scalable Progressive Analytics on Big Data in the Cloud
Badrish Chandramouli* (Microsoft Research), Jonathan Goldstein (Microsoft Research), Abdul Quamar (University of Maryland (College Park)
Analytics over the increasing quantity of data stored in the Cloud has become very expensive, particularly due to the pay-as-you-go Cloud computation model. Data scientists typically manually extract samples of increasing data size (progressive samples) using domain-specific sampling strategies for exploratory querying. This provides them with user-control, repeatable semantics, and result provenance. However, such solutions result in tedious workflows that preclude the reuse of work across samples. On the other hand, existing approximate query processing systems report early results, but do not offer the above benefits for complex ad-hoc queries. We propose a new progressive analytics system based on a progress model called Prism that (1) allows users to communicate progressive samples to the system; (2) allows efficient and deterministic query processing over samples; and (3) provides repeatable semantics and provenance to data scientists. We show that one can realize this model for atemporal relational queries using an unmodified temporal streaming engine, by re-interpreting temporal event fields to denote progress. Based on Prism, we build Now!, a progressive data-parallel computation framework for Windows Azure, where progress is understood as a first-class citizen in the framework. Now! works with "progress-aware reducers" - in particular, it works with streaming engines to support progressive SQL over big data. Extensive experiments on Windows Azure with real and synthetic workloads validate the scalability and benefits of Now! and its optimizations, over current solutions for progressive analytics.
Local Industrial : Big Data Platforms
Location: Diamond 3
Chair: Gang Chen

Fatman: Cost-saving and reliable archival storage based on volunteer resources
An Qin (Baidu, Inc), Dianming Hu (Baidu, Inc), Jun Liu (Baidu, Inc), Wenjun Yang (Baidu, Inc), Dai Tan (Baidu, Inc)

yzStack: Provisioning Customizable Solution for Big Data
Sai Wu (Zhejiang University), Chun Chen (Zhejiang University), Gang Chen (Zhejiang University), Ke Chen (Zhejiang University), Lidan Shou (Zhejiang University), Hui Cao (yzBigData Co., Ltd.), He Bai (City Cloud Technology (Hangzhou) Co., Ltd.)

Realization of the Low Cost and High Performance MySQL Cloud Database
Wei Cao (Alibaba Cloud Computing Inc.), Feng Yu (Alibaba Cloud Computing Inc.), Jiasen Xie (Alibaba Cloud Computing Inc.)

Mariana: Tencent Deep Learning Platform and its Applications
Yongqiang Zou (Tencent Inc.), Xing Jin (Tencent Inc.), Yi Li (Tencent Inc.), Zhimao Guo (Tencent Inc.), Eryu Wang (Tencent Inc.), Bin Xiao (Tencent Inc.)
Papers : Cloud and Data Services
Location: Diamond 4
Chair: Amr El Abbadi


Folk-IS: Opportunistic Data Services in Least Developed Countries
Nicolas Anciaux (INRIA/UVSQ), Luc Bouganim* (INRIA), Thierry Delot (UVHC/INRIA), Sergio Ilarri (U. Zaragoza), Leila Kloul (UVSQ), Nathalie Mitton (INRIA), Philippe Pucheral (INRIA/UVSQ)
According to a wide range of studies, IT should become a key facilitator in establishing primary education, reducing mortality and supporting commercial initiatives in Least Developed Countries (LDCs). The main barrier to the development of IT services in these regions is not only the lack of communication facilities, but also the lack of consistent information systems, security procedures, economic and legal support, as well as political commitment. In this paper, we propose the vision of an infrastructureless data platform well suited for the development of innovative IT services in LDCs. We propose a participatory approach, where each individual implements a small subset of a complete information system thanks to highly secure, portable and low-cost personal devices as well as opportunistic networking, without the need of any form of infrastructure. We review the technical challenges that are specific to this approach.


epiC: an Extensible and Scalable System for Processing Big Data
Dawei Jiang (National U of Singapore), Gang Chen (Zhejiang University), Beng Chin Ooi* (National University of Singapore), Kian-Lee Tan (NUS), Sai Wu (Zhejiang University)
The Big Data problem is characterized by the so called 3V features: Volume - a huge amount of data, Velocity - a high data ingestion rate, and Variety - a mix of structured data, semi-structured data, and unstructured data. The state-of-the-art solutions to the Big Data problem are largely based on the MapReduce framework (aka its open source implementation Hadoop). Although Hadoop handles the data volume challenge successfully, it does not deal with the data variety well since MapReduce enforces a key-value data model along with a row-oriented data processing strategy and bundles the data processing model with the underlying concurrent programming model. This paper presents epiC, an extensible system to tackle the Big Data's data variety challenge. epiC introduces a general Actor-like concurrent programming model, independent of the data processing models, for specifying parallel computations. Users process multi-structured datasets with appropriate epiC extensions, the implementation of a data processing model best suited for the data type and auxiliary code for mapping that data processing model into epiC's concurrent programming model. Like Hadoop, programs written in this way can be automatically parallelized and the runtime system takes care of fault tolerance and inter-machine communications. We present the design and implementation of epiC's concurrent programming model. We also present two customized data processing model, an optimized MapReduce extension and a relational model, on top of epiC. Experiments demonstrate the effectiveness and efficiency of our proposed epiC.


On Arbitrage-free Pricing for General Data Queries
Bing-Rong Lin (Penn State University), Daniel Kifer* (Penn State University)
Data is a commodity. Recent research has considered the mathematical problem of setting prices for different queries over data. Ideal pricing functions need to be flexible-defined for arbitrary queries (select-project-join, aggregate, random sample, and noisy privacy-preserving queries). They should be fine-grained-a consumer should not be required to buy the entire database to get answers to simple "low-information" queries (such as selecting only a few tuples or aggregating over only one attribute). Similarly, a consumer may not want to pay a large amount of money, only to discover that the database is empty. Finally, pricing functions should satisfy consistency conditions such as being "arbitrage-free"-consumers should not be able to circumvent the pricing function by deducing the answer to an expensive query from a few cheap queries. Previously proposed pricing functions satisfy some of these criteria (i.e. they are defined for restricted subclasses of queries and/or use relaxed conditions for avoiding arbitrage). In this paper, we study arbitrage-free pricing functions defined for arbitrary queries. We propose new necessary conditions for avoiding arbitrage and provide new arbitrage-free pricing functions. We also prove several negative results related to the tension between flexible pricing and avoiding arbitrage, and show how this tension often results in unreasonable prices.


CPU Sharing Techniques for Performance Isolation in Multitenant Relational Database-as-a-Service
Sudipto Das* (Microsoft Research), Vivek Narasayya (Microsoft Research), Feng Li (NUS), Manoj Syamala (Microsoft Research)
Multi-tenancy and resource sharing are essential to make a Database-as-a-Service (DaaS) cost-effective. However, one major consequence of resource sharing is that the performance of one tenant's workload can be significantly affected by the resource demands of co-located tenants. The lack of performance isolation in a shared environment can make DaaS less attractive to performance-sensitive tenants. Our approach to performance isolation in a DaaS is to isolate the key resources needed by the tenants' workload. In this paper, we focus on the problem of effectively sharing and isolating CPU among co-located tenants in a multi-tenant DaaS. We show that traditional CPU sharing abstractions and algorithms are inadequate to support several key new requirements that arise in DaaS: (a) absolute and fine-grained CPU reservations without static allocation; (b) support elasticity by dynamically adapting to bursty resource demands; and (c) enable the DaaS provider to suitably tradeoff revenue with fairness. We implemented these new scheduling algorithms in a commercial DaaS prototype and extensive experiments demonstrate the effectiveness of our techniques.


Towards Building Wind Tunnels for Data Center Design
Avrilia Floratou* (IBM Almaden Research Center), Frank Bertsch (University of Wisconsin-Madison), Jignesh Patel (University of Wisconsin), Georgios Laskaris (Duke University)
Data center design is a tedious and expensive process. Recently, this process has become even more challenging as users of cloud services expect to have guaranteed levels of availability, durability and performance. A new challenge for the service providers is to find the most cost-effective data center design and configuration that will accommodate the users' expectations, on ever-changing workloads, and constantly evolving hardware and software components. In this paper, we argue that data center design should become a systematic process. First, it should be done using an integrated approach that takes into account both the hardware and the software interdependencies, and their impact on users' expectations. Second, it should be performed in a "wind tunnel", which uses large-scale simulation to systematically explore the impact of a data center configuration on both the users' and the service providers' requirements. We believe that this is the first step towards systematic data center design - an exciting area for future research.
Papers : Web and Knowledge I
Location: Diamond 5
Chair: Cong Yu


Biperpedia: An Ontology for Search Applications
Rahul Gupta (Google), Alon Halevy (Google), Xuezhi Wang (Carnegie Mellon University), Steven Whang* (Google Research), Fei Wu (Google Inc.)
Search engines make significant efforts to recognize queries that can be answered by structured data and invest heavily in creating and maintaining high-precision databases. While these databases have a relatively wide coverage of entities, the number of attributes they model (e.g., {\sc gdp, capital, anthem}) is relatively small. Extending the number of attributes known to the search engine can enable it to more precisely answer queries from the long and heavy tail, extract a broader range of facts from the Web, and recover the semantics of tables on the Web. We describe Biperpedia, an ontology with 1.6M (class, attribute) pairs and 67K distinct attribute names. Biperpedia extracts attributes from the query stream, and then uses the best extractions to seed attribute extraction from text. For every attribute Biperpedia saves a set of synonyms and text patterns in which it appears, thereby enabling it to recognize the attribute in more contexts. In addition to a detailed analysis of the quality of Biperpedia, we show that it can increase the number of Web tables whose semantics we can recover by more than a factor of~4 compared with Freebase.


Semantic Culturomics (Vision Paper)
Fabian Suchanek* (Télécom ParisTech), Nicoleta Preda (University of Versailles)
Newspapers are testimonials of history. The same is increasingly true of social media such as online forums, online communities, and blogs. By looking at the sequence of articles over time, one can discover the birth and the development of trends that marked society and history -- a field known as Culturomics. But Culturomics has so far been limited to statistics on keywords. In this vision paper, we argue that the advent of large knowledge bases (such as YAGO, NELL, DBpedia, and Freebase) will revolutionize the field. If their knowledge is combined with the news articles, it can breathe life into what is otherwise just a sequence of words for a machine. This will allow discovering trends in history and culture, explaining them through explicit logical rules, and making predictions about the events of the future. We predict that this could open up a new field of research, "Semantic Culturomics", in which no longer human text helps machines build up knowledge bases, but knowledge bases help humans understand their society.


From Data Fusion to Knowledge Fusion
Luna Dong* (google), Evgeniy Gabrilovich (Google Inc.), Geremy Heitz (Google Inc.), Wilko Horn (Google Inc.), Kevin Murphy (Google Inc.), Shaohua Sun (Google Inc.), Wei Zhang (Google Inc.)
The task of data fusion is to identify the true values of data items (e.g., the true date of birth for Tom Cruise) among multiple observed values drawn from different sources (e.g., Web sites) of varying (and unknown) reliability. A recent survey [18] has provided a detailed comparison of various fusion methods on Deep Web data. In this paper, we study the applicability of data fusion techniques on a relevant but more challenging terrain: knowledge fusion. Knowledge fusion identifies true subject-predicate-object triples extracted by multiple information extractors from multiple information sources. These extractors perform the tasks of entity linkage and schema alignment, thus introduce an additional source of noise that is quite different to that traditionally considered in the data fusion literature, which only focuses on factual errors in the original sources. We adapt state-of-the-art data fusion techniques and apply them to a knowledge base with 1.6B unique knowledge triples extracted by 12 extractors from over 1 billion Web pages, which is three orders of magnitude larger than the data sets used in previous data fusion papers. We show great promise of the data fusion approaches in solving the knowledge fusion problem, and suggest interesting research directions through a detailed error analysis of our methods.


Scalable Column Concept Determination for Web Tables Using Large Knowledge Bases
Dong Deng (Tsinghua University), Guoliang Li* (Tsinghua University), Yu Jiang (Tsinghua), Jian Li (Tsinghua University), Cong Yu (Google Research)
Tabular data on the Web has become a rich source of structured data that is useful for ordinary users to explore. Due to its potential, tables on the Web have recently attracted a number of studies with the goals of understanding the semantics of those Web tables and providing effective search and exploration mechanisms over them. An important part of table understanding and search is, i.e., identifying the most appropriate concepts associated with the columns of the tables. The problem becomes especially challenging with the availability of increasingly rich knowledge bases that contain hundreds of millions of entities. In this paper, we focus on an important instantiation of the column concept determination problem, namely, the concepts of a column are determined by {\em fuzzy} matching its cell values to the entities within a large knowledge base. We provide an efficient and scalable \mapreduce-based solution that is scalable to both the number of tables and the size of the knowledge base and propose two novel techniques: knowledge concept aggregation and knowledge entity partition. We prove that both the problem of finding the optimal aggregation strategy and that of finding the optimal partition strategy are NP-hard, and propose efficient heuristic techniques by leveraging the {\em hierarchy} of the knowledge base. Experimental results on real-world datasets show that our method achieves high annotation quality and performance, and scales well.


Aggregating Semantic Annotators
Luying Chen (Oxford), Stefano Ortona (Oxford), Giorgio Orsi (Oxford), Michael Benedikt* (Oxford)
A growing number of resources are available for enriching documents with semantic annotations. While originally focused on a few standard classes of annotations, the ecosystem of annotators is now becoming increasingly diverse. Although annotators often have very different vocabularies, with both high-level and specialist concepts, they also have many semantic interconnections. We will show that both the overlap and the diversity in annotator vocabularies motivate the need for semantic annotation integration: middleware that produces a unified annotation on top of diverse semantic annotators. On the one hand, the diversity of vocabulary allows applications to benefit from the much richer vocabulary available in an integrated vocabulary. On the other hand, we present evidence that the most widely-used annotators on the web suffer from serious accuracy deficiencies: the overlap in vocabularies from individual annotators allows an integrated annotator to boost accuracy by exploiting inter-annotator agreement and disagreement. The integration of semantic annotations leads to new challenges, both compared to usual data integration scenarios and to standard aggregation of machine learning tools. We overview an approach to these challenges that performs ontology-aware aggregation. We introduce an approach that requires no training data, making use of ideas from database repair. We experimentally compare this with a supervised approach, which adapts maximal entropy Markov models to the setting of ontology-based annotations. We further experimentally compare both these approaches with respect to ontology-unaware supervised approaches, and to individual annotators.
Tutorial : Systems for Big Graphs
Location: Bauhinia 1
Chair: Tutorial 2 Chair


Systems for Big Graphs
Arijit Khan, Sameh Elnikety
Large-scale, highly-interconnected networks pervade our society and the natural world around us. Graphs represent such complicated structures and schema-less data including the World Wide Web, social networks, knowledge graphs, genome and scientific databases, e-commerce, medical and government records. Graph processing poses interesting system challenges: A graph models entities and their relationships, which are usually irregular and unstructured; and therefore, the computation and data access patterns exhibit poor locality. Although several disk-based graph-indexing techniques have been proposed for specific graph operations, they still cannot provide the level of efficient random access required by graph computation. On the other hand, the scale of graph data easily overwhelms the main memory and computation resources on commodity servers. Today's big-graphs consist of millions of vertices and billions of edges. In these cases, achieving low latency and high throughput requires partitioning the graph and processing the graph data in parallel across a cluster of servers. However, the software and hardware advances that have worked well for developing parallel databases and scientific applications are not necessarily effective for graph problems. Hence, the last few years has seen an unprecedented interest in building systems for big-graphs by various communities including databases, systems, semantic web, and machine learning. In this tutorial, we discuss the design of these emerging systems for processing of big-graphs, key features of distributed graph algorithms, as well as graph partitioning and workload balancing techniques. We discuss the current challenges and highlight some future research directions.
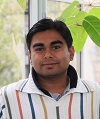
Bio: Arijit Khan is a post-doctorate researcher in the Systems group at ETH Zurich. His research interests span in the area of big-data, big-graphs, and graph systems. He received his PhD from the Department of Computer Science, University of California, Santa Barbara. Arijit is the recipient of the prestigious IBM PhD Fellowship in 2012-13. He co-presented a tutorial on emerging queries over linked data at ICDE 2012.
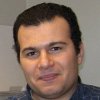
Bio: Sameh Elnikety is a researcher at Microsoft Research in Redmond, Washington. He received his Ph.D. from the Swiss Federal Institute of Technology (EPFL) in Lausanne, Switzerland , and M.S. from Rice University in Houston, Texas. His research interests include distributed server systems, and database systems. Sameh’s work on database replication received the best paper award at Eurosys 2007.
Demo 2
Location: Pearl
Chair: Demo 2 Chair


Faster Visual Analytics through Pixel-Perfect Aggregation
Uwe Jugel* (SAP),Zbigniew Jerzak (SAP),Gregor Hackenbroich (SAP),Volker Markl (TU Berlin)
State-of-the-art visual data analysis tools ignore bandwidth limitations. They fetch millions of records of high-volume time series data from an underlying RDBMS to eventually draw only a few thousand pixels on the screen. In this work, we demonstrate a pixel-aware big data visualization system that dynamically adapts the number of data points transmitted and thus the data rate, while preserving pixel-perfect visualizations. We show how to carefully select the data points to fetch for each pixel of a visualization, using a visualization-driven data aggregation that models the visualization process. Defining all required data reduction operators at the query level, our system trades off a few milliseconds of query execution time for dozens of seconds of data transfer time. The results are significantly reduced response times and a near real-time visualization of millions of data points. Using our pixel-aware system, the audience will be able to enjoy the speed and ease of big data visualizations and learn about the scientific background of our system through an interactive evaluation component, allowing the visitor to measure, visualize, and compare competing visualization-related data reduction techniques.


That's All Folks! Llunatic Goes Open Source
Floris Geerts (University of Antwerp),Giansalvatore Mecca* (Università della Basilicata),Paolo Papotti (QCRI),Donatello Santoro (Università della Basilicata)
It is widely recognized that whenever different data sources need to be integrated into a single target database errors and inconsistencies may arise, so that there is a strong need to apply data-cleaning techniques to repair the data. Despite this need, database research has so far investigated mappings and data repairing essentially in isolation. Unfortunately, schema-mappings and data quality rules interact with each other, so that applying existing algorithms in a pipelined way -- i.e., first exchange then data, then repair the result -- does not lead to solutions even in simple settings. We present the Llunatic mapping and cleaning system, the first comprehensive proposal to handle schema mappings and data repairing in a uniform way. Llunatic is based on the intuition that transforming and cleaning data are different facets of the same problem, unified by their declarative nature. This holistic approach allows us to incorporate unique features into the system, such as configurable user interaction and a tunable trade-off between efficiency and quality of the solutions.


HDBTracker: Aggregate Tracking and Monitoring Over Dynamic Web Databases
Weimo Liu* (The George Washington University),Saad Bin Suhaim (The George Washington University),Saravanan Thirumuruganathan (University of Texas At Arlington),Nan Zhang (George Washington University),Gautam Das (UT Arlington),Ali Jaoua (Qatar University)
Numerous web databases, e.g., amazon.com, eBay.com, are "hidden" behind (i.e., accessible only through) their restrictive search and browsing interfaces. This demonstration showcases HDBTracker, a web-based system that reveals and tracks (the changes of) user-specified aggregate queries over such hidden web databases, especially those that are frequently updated, by issuing a small number of search queries through the public web interfaces of these databases. The ability to track and monitor aggregates has applications over a wide variety of domains - e.g., government agencies can track COUNT of openings at online job hunting websites to understand key economic indicators, while businesses can track the AVG price of a product over a basket of e-commerce websites to understand the competitive landscape and/or material costs. A key technique used in HDBTracker is RS-ESTIMATOR, the first algorithm that can efficiently monitor changes to aggregate query answers over a hidden web database.


BSMA: A Benchmark for Analytical Queries over Social Media Data
Fan Xia* (East China Normal University),Ye Li (East China Normal University),Chengcheng Yu (East China Normal University),Haixin Ma (East China Normal University),Haoji Hu (East China Normal University),Weining Qian (East China Normal University)
The demonstration of a benchmark, named as BSMA, for Benchmarking Social Media Analytics, is introduced in this paper. BSMA is designed to benchmark data management systems supporting analytical queries over social media. It is different to existing benchmarks in that: 1) Both real-life data and a synthetic data generator are provided. The real-life dataset contains a social network of 1.6 million users, and all their tweeting and retweeting activities. The data generator can generate both social networks and synthetic timelines that follow data distributions determined by predefined parameters. 2) A set of workloads are provided. The data generator is in responsible for producing updates. A query generator produces queries based on predefined query templates by generating query arguments online. BSMA workloads cover a large amount of queries with graph operations, temporal queries, hotspot queries, and aggregate queries. Furthermore, the argument generator is capable of sampling data items in the timeline following power-law distribution online. 3) A toolkit is provided to measure and report the performance of systems that implement the benchmark. Furthermore, a prototype system based on dataset and workload of BSMA is also implemented. The demonstration will include two parts, i.e. the internals of data and query generator, as well as the performance testing of reference implementations.


Graph-based Data Integration and Business Intelligence with BIIIG
Andre Petermann* (University of Leipzig),Martin Junghanns (University of Leipzig),Robert Mueller (HTWK Leipzig),Erhard Rahm (university of leipzig)
We demonstrate BIIIG (Business Intelligence with Integrated Instance Graphs), a new system for graph-based data integration and analysis. It aims at improving business analytics compared to traditional OLAP approaches by comprehensively tracking relationships between entities and making them available for analysis. BIIIG supports a largely automatic data integration pipeline for metadata and instance data. Metadata from heterogeneous sources are integrated in a so-called Unified Metadata Graph (UMG) while instance data is combined in a single integrated instance graph (IIG). A unique feature of BIIIG is the concept of business transaction graphs, which are derived from the IIG and which reflect all steps involved in a specific business process. Queries and analysis tasks can refer to the entire instance graph or sets of business transaction graphs. In the demonstration, we perform all data integration steps and present analytic queries including pattern matching and graph-based aggregation of business measures.


SeeDB: Automatically Generating Query Visualizations
Manasi Vartak* (MIT),Samuel Madden (MIT CSAIL),Aditya Parameswaran (Stanford University),Neoklis Polyzotis (University of California - Santa Cruz)
Data analysts operating on large volumes of data often rely on visualizations to interpret the results of queries. However, finding the right visualization for a query is a laborious and time-consuming task. We demonstrate SeeDB, a system that partially automates this task: given a query, SeeDB explores the space of all possible visualizations, and automatically identifies and recommends to the analyst those visualizations it finds to be most "interesting" or "useful". In our demonstration, conference attendees will see SeeDB in action for a variety of queries on multiple real-world datasets.


QUEST: An Exploratory Approach to Robust Query Processing
Anshuman Dutt (Indian Institute of Science),Sumit Neelam (Indian Institute of Science),Jayant Haritsa* (Indian Institute of Science Bangalore)
Selectivity estimates for optimizing declarative SQL queries often differ significantly from those actually encountered during query execution, leading to poor plan choices and inflated response times. We recently proposed a conceptually new approach to address this problem wherein the compile-time estimation process is completely eschewed for error-prone selectivities. Instead, these statistics are systematically discovered at run-time through a precisely calibrated sequence of cost-limited executions from a carefully chosen small set of plans, called the plan bouquet. This construction lends itself to guaranteed worst-case performance bounds, and repeatable execution strategies across multiple invocations of a query. A prototype implementation of the plan bouquet technique, called QUEST, has been incorporated on the PostgreSQL engine. In this demo, we showcase the various features of QUEST which result in novel performance guarantees that open up new possibilities for robust query processing.


Redoop Infrastructure for Recurring Big Data Queries
Chuan Lei* (WPI),Zhongfang Zhuang (WPI),Elke Rundensteiner (WPI),Mohamed Eltabakh (Worcester Polytechnic Institute)
This demonstration presents the Redoop system, the first full-fledged MapReduce framework with native support for recurring big data queries. Recurring queries, repeatedly being executed for long periods of time over evolving high-volume data, have become a bedrock component in most large-scale data analytic applications. Redoop is a comprehensive extension to Hadoop that pushes the support and optimization of recurring queries into Hadoop's core functionality. While backward compatible with regular MapReduce jobs, Redoop achieves an order of magnitude better performance than Hadoop for recurring workloads. Redoop employs innovative window-aware optimization techniques for recurring query execution including adaptive window-aware data partitioning, window-aware task scheduling, and inter-window caching mechanisms. We will demonstrate Redoop's capabilities on a compute cluster against real life workloads including click-stream and sensor data analysis.


PackageBuilder: From Tuples to Packages
Matteo Brucato* (UMass Amherst),Rahul Ramakrishna (UMass Amherst),Azza Abouzied (New York University Abu Dhabi UAE),Alexandra Meliou (Umass Amherst)
In this demo, we present PackageBuilder, a system that extends database systems to support package queries. A package is a collection of tuples that individually satisfy base constraints and collectively satisfy global constraints. The need for package support arises in a variety of scenarios: For example, in the creation of meal plans, users are not only interested in the nutritional content of individual meals (base constraints), but also care to specify daily consumption limits and control the balance of the entire plan (global constraints). We introduce PaQL, a declarative SQL-based package query language, and the interface abstractions which allow users to interactively specify package queries and easily navigate through their results. To efficiently evaluate queries, the system employs pruning and heuristics, as well as state-of-the-art constraint optimization solvers. We demonstrate PackageBuilder by allowing attendees to interact with the system's interface, to define PaQL queries and to observe how query evaluation is performed.


Ontology Assisted Crowd Mining
Yael Amsterdamer* (Tel Aviv University),Susan Davidson (University of Pennsylvania),Tova Milo (Tel Aviv University),Slava Novgorodov (Tel Aviv University),Amit Somech (Tel Aviv University)
We present OASSIS (for Ontology ASSISted crowd mining), a prototype system which allows users to declaratively specify their information needs, and mines the crowd for answers. The answers that the system computes are concise and relevant, and represent frequent, significant data patterns. The system is based on (1) a generic model that captures both ontological knowledge, as well as the individual knowledge of crowd members from which frequent patterns are mined; (2) a query language in which users can specify their information needs and types of data patterns they seek; and (3) an efficient query evaluation algorithm, for mining semantically concise answers while minimizing the number of questions posed to the crowd. We will demonstrate OASSIS using a couple of real-life scenarios, showing how users can formulate and execute queries through the OASSIS UI and how the relevant data is mined from the crowd.


SOPS: A System for Efficient Processing of Spatial-Keyword Publish/Subscribe
Lisi Chen* (NTU),Yan Cui (NTU),Gao Cong (Nanyang Technological University),Xin Cao (NTU)
Massive amount of data that are geo-tagged and associated with text information are being generated at an unprecedented scale. These geo-textual data cover a wide range of topics. Users are interested in receiving up-to-date geo-textual objects (e.g., geo-tagged Tweets) such that their locations meet users' need and their texts are interesting to users. For example, a user may want to be updated with tweets near her home on the topic "dengue fever headache." In this demonstration, we present SOPS, the Spatial-Keyword Publish/Subscribe System, that is capable of efficiently processing spatial keyword continuous queries. SOPS supports two types of queries: (1) Boolean Range Continuous (BRC) query that can be used to subscribe the geo-textual objects satisfying a boolean keyword expression and falling in a specified spatial region; (2) Temporal Spatial-Keyword Top-k Continuous (TaSK) query that continuously maintains up-to-date top-k most relevant results over a stream of geo-textual objects. SOPS enables users to formulate their queries and view the real-time results over a stream of geo-textual objects by browser-based user interfaces. On the server side, we propose solutions to efficiently processing a large number of BRC queries (tens of millions) and TaSK queries over a stream of geo-textual objects.


MLJ: Language-Independent Real-Time Search of Tweets Reported by Media Outlets and Journalists
Masumi Shirakawa* (Osaka University),Takahiro Hara (Osaka University),Shojiro Nishio (Osaka University)
In this demonstration, we introduce MLJ (MultiLingual Journalism, http://mljournalism.com), a first Web-based system that enables users to search any topic of latest tweets posted by media outlets and journalists beyond languages. Handling multilingual tweets in real time involves many technical challenges: language barrier, sparsity of words, and real-time data stream. To overcome the language barrier and the sparsity of words, MLJ harnesses CL-ESA, a Wikipedia-based language-independent method to generate a vector of Wikipedia pages (entities) from an input text. To continuously deal with tweet stream, we propose one-pass DP-means, an online clustering method based on DP-means. Given a new tweet as an input, MLJ generates a vector using CL-ESA and classifies it into one of clusters using one-pass DP-means. By interpreting a search query as a vector, users can instantly search clusters containing latest related tweets from the query without being aware of language differences. MLJ as of March 2014 supports nine languages including English, Japanese, Korean, Spanish, Portuguese, German, French, Italian, and Arabic covering 24 countries.
Tuesday Sep 2nd 15:45-17:15
Papers : Memory Systems
Location: Diamond 1
Chair: Anastasia Ailamaki


Multi-Core, Main-Memory Joins: Sort vs. Hash Revisited
Cagri Balkesen* (ETH Zurich), Gustavo Alonso (Systems Group (ETH Zurich), Jens Teubner (TU Dortmund University), Tamer Ozsu (University of Waterloo)
In this paper we experimentally study the performance of main-memory, parallel, multi-core join algorithms, focusing on sort-merge and (radix-)hash join. The relative performance of these two join approaches have been a topic of discussion for a long time. With the advent of modern multi-core architectures, it has been argued that sort-merge join is now a better choice than radix-hash join. This claim is justified based on the width of SIMD instructions (sort-merge outperforms radix-hash join once SIMD is sufficiently wide), and NUMA awareness (sort-merge is superior to hash join in NUMA architectures). We conduct extensive experiments on the original and optimized versions of these algorithms. The experiments show that, contrary to these claims, radix-hash join is still clearly superior, and sort-merge approaches to performance of radix only when very large amounts of data are involved. The paper also provides the fastest implementations of these algorithms, and covers many aspects of modern hardware architectures relevant not only for joins but for any parallel data processing operator.


Write-limited sorts and joins for persistent memory
Stratis Viglas*, University of Edinburgh
To mitigate the impact of the widening gap between the memory needs of CPUs and what standard memory technology can deliver, system architects have introduced a new class of memory technology termed persistent memory. Persistent memory is byte-addressable, but exhibits asymmetric I/O: writes are typically one order of magnitude more expensive than reads. Byte addressability combined with I/O asymmetry renders the performance profile of persistent memory unique. Thus, it becomes imperative to find new ways to seamlessly incorporate it into database systems. We do so in the context of query processing. We focus on the fundamental operations of sort and join processing. We introduce the notion of write-limited algorithms that effectively minimize the I/O cost. We give a high-level API that enables the system to dynamically optimize the workflow of the algorithms; or, alternatively, allows the developer to tune the write profile of the algorithms. We present four different techniques to incorporate persistent memory into the database processing stack in light of this API. We have implemented and extensively evaluated all our proposals. Our results show that the algorithms deliver on their promise of I/O-minimality and tunable performance. We showcase the merits and deficiencies of each implementation technique, thus taking a solid first step towards incorporating persistent memory into query processing.


Scalable Logging through Emerging Non-Volatile Memory
Tianzheng Wang* (University of Toronto), Ryan Johnson (University of Toronto)
Emerging byte-addressable, non-volatile memory (NVM) is fundamentally changing the design principle of transaction logging. It potentially invalidates the need for flush-before-commit as log records are persistent immediately upon write. Distributed logging - a once prohibitive technique for single node systems in the DRAM era - becomes a promising solution to easing the logging bottleneck because of the non-volatility and high performance of NVM. In this paper, we advocate NVM and distributed logging on multicore and multi-socket hardware. We identify the challenges brought by distributed logging and discuss solutions. To protect committed work in NVM-based systems, we propose passive group commit, a lightweight, practical approach that leverages existing hardware and group commit. We expect that durable processor cache is the ultimate solution to protecting committed work and building reliable, scalable NVM-based systems in general. We evaluate distributed logging with logging-intensive workloads and show that distributed logging can achieve as much as ~3x speedup over centralized logging in a modern DBMS and that passive group commit only induces minuscule overhead.


Storage Management in the NVRAM Era
Steven Pelley* (University of Michigan), Thomas Wenisch (University of Michigan), Brian Gold (Oracle Corporation), Bill Bridge (Oracle Corporation)
Emerging nonvolatile memory technologies (NVRAM) offer an alternative to disk that is persistent, provides read latency similar to DRAM, and is byte-addressable. Such NVRAMs could revolutionize online transaction processing (OLTP), which today must employ sophisticated optimizations with substantial software overheads to overcome the long latency and poor random access performance of disk. Nevertheless, many candidate NVRAM technologies exhibit their own limitations, such as greater-than-DRAM latency, particularly for writes. In this paper, we reconsider OLTP durability management to optimize recovery performance and forward-processing throughput for emerging NVRAMs. First, we demonstrate that using NVRAM as a drop-in replacement for disk allows near-instantaneous recovery, but software complexity necessary for disk (i.e., Write Ahead Logging/ARIES) limits transaction throughput. Next, we consider the possibility of removing software-managed DRAM buffering. Finally, we measure the cost of ordering writes to NVRAM, which is vital for correct recovery. We consider three recovery mechanisms: NVRAM Disk-Replacement, In-Place Updates (transactions persist data in-place), and NVRAM Group Commit (transactions commit/persist atomically in batches). Whereas In-Place Updates offers the simplest design, it introduces persist synchronizations at every page update. NVRAM Group Commit minimizes persist synchronization, offering up to a 50% throughput improvement for large synchronous persist latencies.


WideTable: An Accelerator for Analytical Data Processing
Yinan Li* (Univ. of Wisconsin-Madison), Jignesh Patel (University of Wisconsin)
This paper presents a technique called WideTable that aims to improve the speed of analytical data processing systems. A WideTable is built by denormalizing the database, and then converting complex queries into simple scans on the underlying (wide) table. To avoid the pitfalls associated with denormalization, e.g. space overheads, WideTable uses a combination of techniques including dictionary encoding and columnar storage. When denormalizing the data, WideTable uses outer joins to ensure that queries on tables in the schema graph, which are now nested as embedded tables in the WideTable, are processed correctly. Then, using a packed code scan technique, even complex queries on the original database can be answered by using simple scans on the WideTable(s). We experimentally evaluate our methods in a main memory setting using the queries in TPC-H, and demonstrate the effectiveness of our methods, both in terms of raw query performance and scalability when running on many-core machines.
Papers : Query Optimization
Location: Diamond 2
Chair: Chee Yong Chan


Shared Workload Optimization
Georgios Giannikis* (Systems Group (ETH Zurich), Darko Makreshanski (Systems Group (ETH Zurich), Gustavo Alonso (Systems Group (ETH Zurich), Donald Kossmann (Systems Group (ETH Zurich)
As a result of increases in both the query load and the data managed, as well as changes in hardware architecture (multicore), the last years have seen a shift from query-at-a-time approaches towards shared work (SW) systems where queries are executed in groups. Such groups share operators like scans and joins, leading to systems that process hundreds to thousands of queries in one go. SW systems range from storage engines that use in-memory cooperative scans to more complex query processing engines that share joins over analytical and star schema queries. In all cases, they rely on either single query optimizers, predicate sharing, or on manually generated plans. In this paper we explore the problem of shared workload optimization (SWO) for SW systems. The challenge in doing so is that the optimization has to be done for the entire workload and that results in a class of stochastic knapsack with uncertain weights optimization, which can only be addressed with heuristics to achieve a reasonable runtime. In this paper we focus on hash joins and shared scans and present a first algorithm capable of optimizing the execution of entire workloads by deriving a global executing plan for all the queries in the system. We evaluate the optimizer over the TPC-W and the TPC-H benchmarks. The results prove the feasibility of this approach and demonstrate the performance gains that can be obtained from SW systems.


Aggregation and Ordering in Factorised Databases
Nurzhan Bakibayev (Oxford), Tomas Kocisky (Oxford), Dan Olteanu* (Oxford University), Jakub Zavodny (Oxford)
A common approach to data analysis involves understanding and manipulating succinct representations of data. In earlier work, we put forward a succinct representation system for relational data called factorised databases and reported on the main-memory query engine FDB for select-project-join queries on such databases. In this paper, we extend FDB to support a larger class of practical queries with aggregates and ordering. This requires novel optimisation and evaluation techniques. We show how factorisation coupled with partial aggregation can effectively reduce the number of operations needed for query evaluation. We also show how factorisations of query results can support enumeration of tuples in desired orders as efficiently as listing them from the unfactorised, sorted results. We experimentally observe that FDB can outperform off-the-shelf relational engines by orders of magnitude.


Expressiveness and Complexity of Order Dependencies
Jaroslaw Szlichta* (York University), Parke Godfrey (York University), Jarek Gryz (York University and IBM CAS), Calisto Zuzarte (IBM Toronto)
Dependencies play an important role in databases. We study order dependencies (ODs)—and unidirectional order dependencies (UODs), a proper sub-class of ODs—which describe the relationships among lexicographical orderings of sets of tuples. We consider lexicographical ordering, as by the order-by operator in SQL, because this is the notion of order used in SQL and within query optimization. Our main goal is to investigate the inference problem for ODs, both in theory and in practice. We show the usefulness of ODs in query optimization. We establish the following theoretical results: (i) a hierarchy of order dependency classes; (ii) a proof of co-NP-completeness of the inference problem for the subclass of UODs (and ODs); (iii) a proof of co-NP-completeness of the inference problem of functional dependencies (FDs) from ODs in general, but demonstrate linear time complexity for the inference of FDs from UODs; (iv) a sound and complete elimination procedure for inference over ODs; and (v) a sound and complete polynomial inference algorithm for sets of UODs over restricted domains.


Counter Strike: Generic Top-Down Join Enumeration for Hypergraphs
Pit Fender* (University of Mannheim), Guido Moerkotte (University of Mannheim)
Finding the optimal execution order of join operations is a crucial task of today’s cost-based query optimizers. There are two approaches to identify the best plan: bottom-up and top-down join enumeration. But only the top-down approach allows for branch-and-bound pruning, which can improve compile time by several orders of magnitude while still preserving optimality. For both optimization strategies, efficient enumeration algorithms have been published. However, there are two severe limitations for the top-down approach: The published algorithms can handle only (1) simple (binary) join predicates and (2) inner joins. Since real queries may contain complex join predicates involving more than two relations, and outer joins as well as other non-inner joins, efficient top-down join enumeration cannot be used in practice yet. We develop a novel top-down join enumeration algorithm that overcomes these two limitations. Furthermore, we show that our new algorithm is competitive when compared to the state of the art in bottom-up processing even without playing out its advantage by making use of its branch-and-bound pruning capabilities.


Optimizing Join Enumeration in Transformation-based Query Optimizers
Anil Shanbhag* (IIT Bombay), S Sudarshan (IIT Bombay)
Query optimizers built on the Volcano/Cascades framework, which is based on transformation rules, are used in many commercial databases. Transformation rulesets proposed earlier for join order enumeration in such a framework either allow enumeration of joins with cross-products (which can significantly increase the cost of optimization), or generate a large number of duplicate derivations. In this paper we propose two new rulesets for generating all cross-product free join trees. One of the rulesets is a minor extension of a simple but inefficient ruleset, which we prove is complete (we also show that a naive extension of an efficient ruleset leads to incompleteness). We then propose an efficient new ruleset, which is based on techniques proposed recently for top-down join order enumeration, but unlike earlier work it is cleanly integrated into the Volcano/Cascades framework, and can be used in conjunction with other transformation rules. We show that our ruleset is complete (i.e., it generates the entire search space without cross products) while avoiding inefficiency due to duplicate derivations. We have implemented this ruleset in the PyroJ Optimizer (an implementation of the Volcano optimizer framework) and show that it significantly outperforms the alternatives, in some cases by up to two orders of magnitude, in terms of time taken.
Panel: The Role of Database Systems in the Era of Big Data
Location: Diamond 3
Chair: C. Mohan

The Role of Database Systems in the Era of Big Data
Moderator: C. Mohan (IBM Almaden Research Center) Panelists: Michael Carey (University of California at Irvine), Surajit Chaudhuri (Microsoft), Ashish Gupta (Google), Wolfgang Lehner (Technical University of Dresden) , Christopher Re (Stanford University) and Gera Shegalov (Twitter)
Big Data has become a hot topic in the last few years in both industry and the research community. For the most part, these developments were initially triggered by the requirements of Web 2.0 companies. Both technical and non-technical issues have continued to fuel the rapid pace of developments in the Big Data space. Open source and non-traditional software entities have played key roles in the latter. As it always happens with any emerging technology, there is a fair amount of hype that accompanies the work being done in the name of Big Data. The set of clear-cut distinctions that were made initially between Big Data systems and traditional database management systems are being blurred as the needs of the broader set of (¡°real world¡±) users and developers have come into sharper focus in the last couple of years. In this panel, we will discuss and debate the developments in Big Data and try to distill reality from the hype!
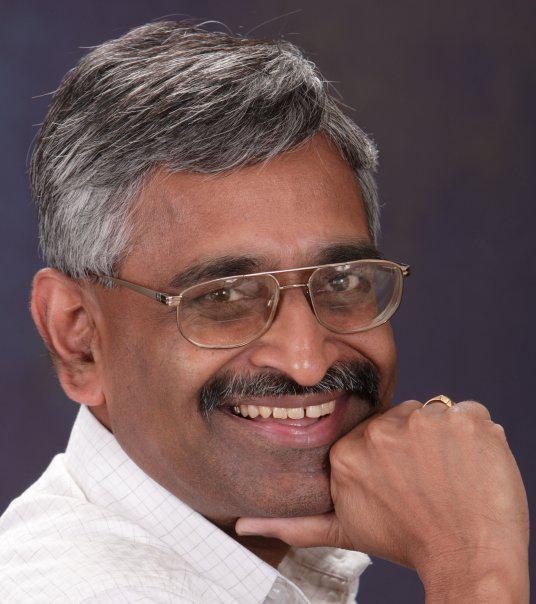
Bio: Dr. C. Mohan has been an IBM researcher for 32 years in the information management area, impacting numerous IBM and non-IBM products, the research and academic communities, and standards, especially with his invention of the ARIES family of locking and recovery algorithms, and the Presumed Abort commit protocol. This IBM, ACM and IEEE Fellow has also served as the IBM India Chief Scientist. In addition to receiving the ACM SIGMOD Innovation Award, the VLDB 10 Year Best Paper Award and numerous IBM awards, he has been elected to the US and Indian National Academies of Engineering, and has been named an IBM Master Inventor. This distinguished alumnus of IIT Madras received his PhD at the University of Texas at Austin. He is an inventor of 40 patents. He has served on the advisory board of IEEE Spectrum and on the IBM Software Group Architecture Board¡¯s Council. Mohan is a frequent speaker in North America, Western Europe and India, and has given talks in 40 countries. More information can be found in his home page at http://bit.ly/CMohan
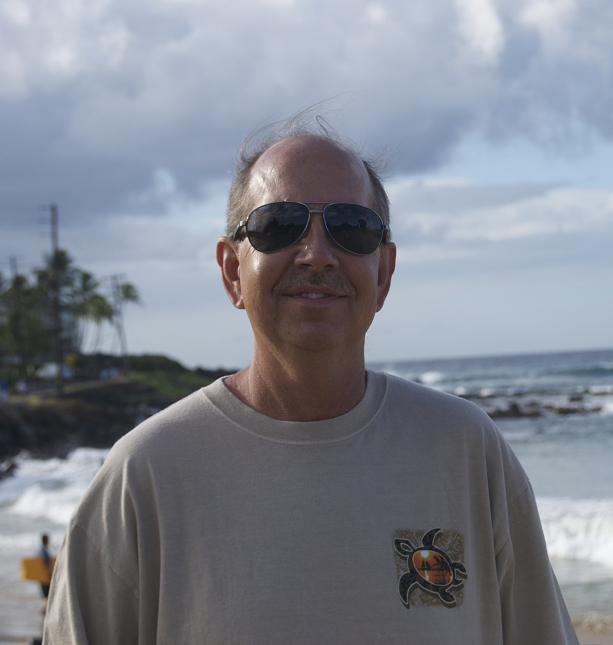
Bio: Michael J. Carey is a Bren Professor of Information and Computer Sciences at UC Irvine. Before joining UCI in 2008, Carey worked at BEA Systems for seven years and led the development of BEA's AquaLogic Data Services Platform product for virtual data integration. He also spent a dozen years teaching at the University of Wisconsin-Madison, five years at the IBM Almaden Research Center working on object-relational databases, and a year and a half at e-commerce platform startup Propel Software during the infamous 2000-2001 Internet bubble. Carey is an ACM Fellow, a member of the National Academy of Engineering, and a recipient of the ACM SIGMOD E.F. Codd Innovations Award. His current interests all center around data-intensive computing and scalable data management (a.k.a. Big Data). He is currently leading the UC Irvine + UC Riverside AsterixDB open source BDMS project.
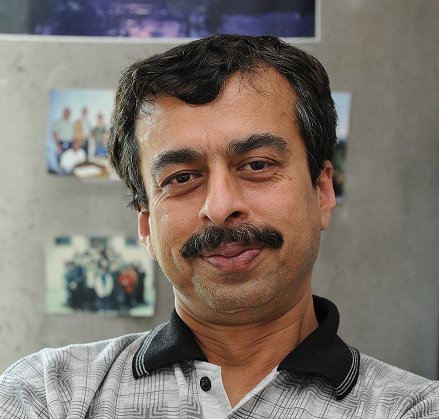
Bio: Surajit Chaudhuri is a Distinguished Scientist at Microsoft Research. His current areas of interest are enterprise data analytics, self-manageability and multi-tenant technology for cloud database services. Working with his colleagues in Microsoft Research and the Microsoft SQL Server team, he helped incorporate the Index Tuning Wizard - and subsequently Database Engine Tuning Advisor - into Microsoft SQL Server. He initiated a project on data cleaning at Microsoft Research whose technology now ships in Microsoft SQL Server. Surajit represents Microsoft Research in the Senior Leadership team of Microsoft Cloud and Enterprise group. He is an ACM Fellow, a recipient of the ACM SIGMOD Edgar F. Codd Innovations Award, ACM SIGMOD Contributions Award, a VLDB 10 year Best Paper Award, and an IEEE Data Engineering Influential Paper Award. He was the Program Committee Chair for ACM SIGMOD 2006, a Co-Chair of ACM SIGKDD 1999 & ACM SOCC 2010, and has served on the editorial boards of ACM TODS and IEEE TKDE. Surajit received his Ph.D. from Stanford University and B.Tech from the Indian Institute of Technology, Kharagpur.
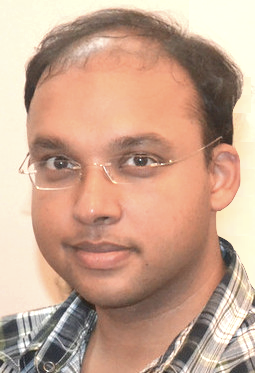
Bio: Ashish Gupta is a Distinguished Software Engineer at Google. Ashish works in the Ads Backend Infrastructure group, where he works on numerous very large scale distributed system problems, including continuous event processing, peta-byte scale storage engines, big data query processing, experiment analysis, etc. Ashish holds a Masters in Computer Science from University of Texas at Austin and did his Bachelors in Computer Science from IIT Delhi.
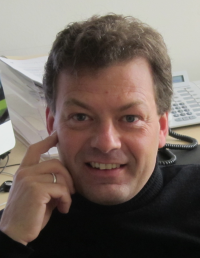
Bio: Wolfgang Lehner is full professor and head of the database technology group at the TU Dresden, Germany. His research is dedicated to the combination of database system architecture with statistical algorithms specifically looking at crosscutting aspects from algorithms down to hardware-related aspects in main-memory centric settings. He is part of TU Dresden's excellence cluster with research topics in energy-aware scheduling, resilient data structures on unreliable hardware, and orchestration wildly heterogeneous systems; he is also a principal investigator of Germany's national 'Competence Center for Scalable Data Services and Solutions' (ScaDS); For many years, Wolfgang maintains a close research relationship with the SAP HANA development team. He is an elected member of the VLDB Endowment, serves on the review board of the German Research Foundation (DFG) and is a newly elected member of the Academy of Europe.
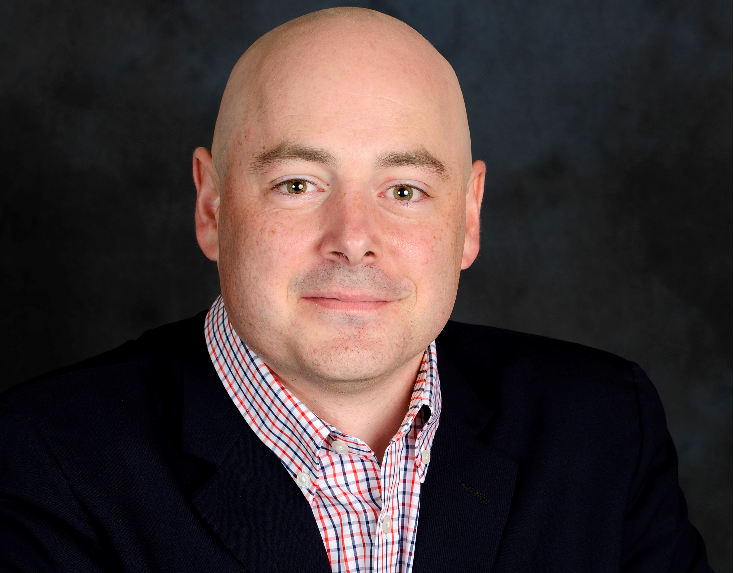
Bio: Christopher (Chris) Re is an assistant professor in the Department of Computer Science at Stanford University. The goal of his work is to enable users and developers to build applications that more deeply understand and exploit data. Chris received his PhD from the University of Washington in Seattle under the supervision of Dan Suciu. For his PhD work in probabilistic data management, Chris received the SIGMOD 2010 Jim Gray Dissertation Award. Chris's papers have received four best-paper or best-of-conference citations, including best paper in PODS 2012, best-of-conference in PODS 2010 twice, and one best-of-conference in ICDE 2009. Chris received an NSF CAREER Award in 2011 and an Alfred P. Sloan fellowship in 2013.
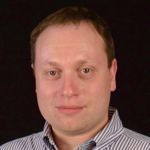
Bio: German "Gera" Shegalov received Masters and PhD in Computer Science from Saarland University in Saarbruecken, Germany. Gera developed the first version of the SQL Sever-based transaction-time database project ImmortalDB as an intern at Microsoft Research. Gera's PhD thesis developed the formal models and provided the model checking proofs for the application recovery Interaction Contracts (IC) framework by Lomet and Weikum. He has also implemented the IC framework for PHP-based web services where the system restores and replays the state of browsers and distributed web service components in an exactly-once manner. Gera's career as a Software Engineer included Oracle Database HA Group and Oracle Java Platform Group where he worked among other things on Persistence, RPC layer, and XA-2PC optimization for JMS helping Oracle win the SPECjAppServer benchmark. For the past ~3 years, Gera has worked in Hadoop space. First with MapR Technologies where he worked on shuffle and sort optimizations in MapReduce, and lately at Twitter where he helps one of the biggest Hadoop installations in the world crunch 30K+ analytics jobs and petabytes of data daily.
Papers : Storage Management
Location: Diamond 4
Chair: Alfons Kemper


Efficient In-memory Data Management: An Analysis
Hao Zhang* (National University of Singapore), Bogdan Marius Tudor (National University of Singapo), Gang Chen (Zhejiang University), Beng Chin Ooi (National University of Singapore)
This paper analyzes the performance of three systems for in-memory data management: Memcached, Redis and the Resilient Distributed datasets(RDD) implemented by Spark. By performing a thorough performance analysis of both analytics operations and fine-grained object operations such as set/get, we show that neither system handles efficiently both types of workloads. For Memcached and Redis the CPU and I/O performance of the TCP stack are the bottlenecks-even when serving in-memory objects within a single server node. RDD does not support efficient get operation for random objects, due to a large startup cost of the get job. Our analysis reveals a set of features that a system must support in order to achieve efficient in-memory data management.


Design and Evaluation of Storage Organizations For Read-Optimized Main-Memory Databases
Craig Chasseur* (University of Wisconsin), Jignesh Patel (University of Wisconsin)
Existing main memory data processing systems employ a variety of storage organizations and make a number of storage-related design choices. The focus of this paper is on systematically evaluating a number of these key storage design choices for main memory analytical (i.e. read-optimized) database settings. Our evaluation produces a number of key insights: First, it is always beneficial to organize data into self-contained memory blocks rather than large files. Second, both column-stores and row-stores display performance advantages for different types of queries, and for high performance both should be implemented as options for the tuple-storage layout. Third, cache-sensitive B+-tree indices can play a major role in accelerating query performance, especially when used in a block-oriented organization. Finally, compression can also play a role in accelerating query performance depending on data distribution and query selectivity.


Trekking Through Siberia: Managing Cold Data in a Memory-Optimized Database
Ahmed Eldawy (University of Minnesota), Justin Levandoski* (Microsoft Research), Paul Larson (Microsoft)
Main memories are becoming sufficiently large that most OLTP databases can be stored entirely in main memory, but this may not be the best solution. OLTP workloads typically exhibit skewed access patterns where some records are hot (frequently accessed) but many records are cold (infrequently or never accessed). It is still more economical to store the coldest records on secondary storage such as flash. In this paper we introduce Siberia, a framework for managing cold data in the Microsoft Hekaton main-memory database engine. We discuss how to migrate cold data to a secondary storage while providing an interface to the user to manipulate both hot and cold data hiding actual data location. We describe how queries of different isolation levels can read and modify data stored in both hot and cold stores without restriction while minimizing number of accesses to cold storage. We also show how records can be migrated between hot and cold stores while the DBMS is online and active. Experiments reveal that for cold data access rates appropriate for main-memory optimized databases, we incur an acceptable 7-14% throughput loss.


Storage Management in AsterixDB
Sattam Alsubaiee* (UC Irvine), Alex Behm (Cloudera), Vinayak Borkar (UC Irvine), Zachary Heilbron (UC Irvine), Young-Seok Kim (UC Irvine), Michael Carey (UC Irvine), Markus Dreseler (UC Irvine), Chen Li (University of California)
Social networks, online communities, mobile devices, and instant messaging applications generate complex, unstructured data at a high rate, resulting in large volumes of data. This poses new challenges for data management systems that aim to ingest, store, index, and analyze such data efficiently. In response, we released the first public version of AsterixDB, an open-source Big Data Management System (BDMS), in June of 2013. This paper describes the storage management layer of AsterixDB, providing a detailed description of its ingestion-oriented approach to local storage and a set of initial measurements of its ingestion-related performance characteristics. In order to support high frequency insertions, AsterixDB has wholly adopted Log-Structured Merge-trees as the storage technology for all of its index structures. We describe how the AsterixDB software framework enables ``LSM-ification'' (conversion from an in-place update, disk-based data structure to a deferred-update, append-only data structure) of any kind of index structure that supports certain primitive operations, enabling the index to ingest data efficiently. We also describe how AsterixDB ensures the ACID properties for operations involving multiple heterogeneous LSM-based indexes. Lastly, we highlight the challenges related to managing the resources of a system when many LSM indexes are used concurrently and present AsterixDB's initial solution.


Anti-Caching: A New Approach to Database Management System Architecture
Justin DeBrabant* (Brown University), Andrew Pavlo (Brown University), Stephen Tu (MIT), Michael Stonebraker (MIT), Stan Zdonik (Brown University)
The traditional wisdom for building disk-based relational database management systems (DBMS) is to organize data in heavily-encoded blocks stored on disk, with a main memory block cache. In order to improve performance given high disk latency, these systems use a multi-threaded architecture with dynamic record-level locking that allows multiple transactions to access the database at the same time. Previous research has shown that this results in substantial over- head for on-line transaction processing (OLTP) applications [15].The next generation DBMSs seek to overcome these limitations with an architecture based on main memory resident data. To over- come the restriction that all data fit in main memory, we propose a new technique, called anti-caching, where cold data is moved to disk in a transactionally-safe manner as the database grows in size. Because data initially resides in memory, an anti-caching ar- chitecture reverses the traditional storage hierarchy of disk-based systems. Main memory is now the primary storage device.We implemented a prototype of our anti-caching proposal in a high-performance, main memory OLTP DBMS and performed a series of experiments across a range of database sizes, workload skews, and read/write mixes. We compared its performance with an open-source, disk-based DBMS optionally fronted by a distributed main memory cache. Our results show that for higher skewed workloads the anti-caching architecture has a performance advan- tage over either of the other architectures tested of up to 9 for a data size 8 larger than memory.
Papers : Database Usability II
Location: Diamond 5
Chair: Letizia Tanca


Support the Data Enthusiast: Challenges for Next-Generation Data-Analysis Systems [Vision Paper]
Kristi Morton* (University of Washington), Magdalena Balazinska (University of Washington), Dan Grossman (University of Washington), Jock Mackinlay (Tableau Software)
We present a vision of next-generation visual analytics services. We argue that these services should provide three related capabilities:support visual and interactive data exploration as they do today, but also suggest relevant data to enrich visualizations, and facilitate the integration and cleaning of that data. Most importantly, they should provide all these capabilities seamlessly in the context of an uninterrupted data analysis cycle. We present the challenges and opportunities in building such next-generation visual analytics services.


A Probabilistic Optimization Framework for the Empty-Answer Problem
Davide Mottin* (University of Trento), Alice Marascu (IBM), Senjuti Basu Roy (Univ of Washington Tacoma), Gautam Das (University of Texas (Arlington), Themis Palpanas (University of Trento), Yannis Velegrakis (University of Trento)
We propose a principled optimization-based interactive query relaxation framework for queries that return no answers. Given an initial query that returns an empty answer set, our framework dynamically computes and suggests alternative queries with less conditions than those the user has initially requested, in order to help the user arrive at a query with a non-empty answer, or at a query for which no matter how many additional conditions are ignored, the answer will still be empty. Our proposed approach for suggesting query relaxations is driven by a novel probabilistic framework based on optimizing a wide variety of application-dependent objective functions. We describe optimal and approximate solutions of different optimization problems using the framework. We analyze these solutions, experimentally verify their efficiency and effectiveness, and illustrate their advantage over the existing approaches.


Toward Computational Fact-Checking
You Wu* (Duke University), Pankaj Agarwal (Duke University), Chengkai Li (The University of Texas at Arlington), Jun Yang (Duke University), Cong Yu (Google Research)
Our news are saturated with claims of ``facts'' made from data. Database research has in the past focused on how to answer queries, but has not devoted much attention to discerning more subtle qualities of the resulting claims, e.g., is a claim ``cherry-picking''? This paper proposes a framework that models claims based on structured data as parameterized queries. A key insight is that we can learn a lot about a claim by perturbing its parameters and seeing how its conclusion changes. This framework lets us formulate practical fact-checking tasks---reverse-engineering (often intentionally) vague claims, and countering questionable claims---as computational problems. Along with the modeling framework, we develop an algorithmic framework that enables efficient instantiations of ``meta'' algorithms by supplying appropriate algorithmic building blocks. We present real-world examples and experiments that demonstrate the power of our model, efficiency of our algorithms, and usefulness of their results.


A System for Management and Analysis of Preference Data
Marie Jacob* (University Of Pennsylvania), Benny Kimelfeld (LogicBlox), Julia Stoyanovich (Drexel University)
Over the past decade, the need to analyze large volumes of rankings and preferences has arisen in applications in different domains. Examples include rank aggregation in genomic data analysis, management of votes in elections, and recommendation systems in e-commerce. The scientific community has established a rich literature of paradigms and algorithms for analyzing preference data. However, little focus has been paid to the challenges of building a system for preference-data management, such as incorporation into larger applications, computational abstractions for usability by data scientists, and scaling up to modern volumes. This vision paper proposes a management system for preference data that aims to address these challenges. We adopt the relational database model, and propose extensions that are specialized to handling preference data. In particular, we introduce a special type of a relation that is designed to represent and store preference data. Moreover, we propose a type of composable operations on preference relations (which we call preference-to-preference functions) that can be embedded in SQL statements in a natural fashion; we illustrate their ability to represent common analytics, as well as their ease of use. Each such an operation can be registered as a database primitive, and hence, can be reused across different applications. We outline the challenges in the establishment of such a system, like the translation of known concepts and algorithms into effective solutions for applications in different domains.


An Approach towards the Study of Symmetric Queries
Marc Gyssens* (Hasselt University), Jan Paredaens (University of Antwerp), Dirk Van Gucht (Indiana University), Jef Wijsen (University of Mons), Yuqing Wu (Indiana University)
Many data-intensive applications have to query a database that involves sequences of sets of objects. It is not uncommon that the order of the sets in such a sequence does not affect the result of the query. Such queries are called symmetric. In this paper, the authors wish to initiate research on symmetric queries. Thereto, a data model is proposed in which a binary relation between objects and set names encodes set membership. On this data model, two query languages are introduced, QuineCALC and SyCALC. They are correlated in a manner that is made precise with the symmetric Boolean functions of Quine, respectively symmetric relational functions, on sequences of sets of given length. The latter do not only involve the Boolean operations union, intersection, and complement, but also projection and Cartesian product. Quine's characterization of symmetric Boolean functions in terms of incidence information is generalized to QuineCALC queries. In the process, an incidence-based normal form for QuineCALC queries is proposed. Inspired by these desirable incidence-related properties of QuineCALC queries, counting-only queries are introduced as SyCALC queries for which the result only depends on incidence information. Counting-only queries are then characterized as quantified Boolean combinations of QuineCALC queries, and a normal form is proposed for them as well. Finally, it is shown that, while it is undecidable whether a SyCALC query is counting-only, it is decidable whether a counting-only query is a QuineCALC query.
Tutorial : Systems for Big Graphs
Location: Bauhinia 1
Chair: Tutorial 2 Chair


Systems for Big Graphs
Arijit Khan, Sameh Elnikety
Large-scale, highly-interconnected networks pervade our society and the natural world around us. Graphs represent such complicated structures and schema-less data including the World Wide Web, social networks, knowledge graphs, genome and scientific databases, e-commerce, medical and government records. Graph processing poses interesting system challenges: A graph models entities and their relationships, which are usually irregular and unstructured; and therefore, the computation and data access patterns exhibit poor locality. Although several disk-based graph-indexing techniques have been proposed for specific graph operations, they still cannot provide the level of efficient random access required by graph computation. On the other hand, the scale of graph data easily overwhelms the main memory and computation resources on commodity servers. Today's big-graphs consist of millions of vertices and billions of edges. In these cases, achieving low latency and high throughput requires partitioning the graph and processing the graph data in parallel across a cluster of servers. However, the software and hardware advances that have worked well for developing parallel databases and scientific applications are not necessarily effective for graph problems. Hence, the last few years has seen an unprecedented interest in building systems for big-graphs by various communities including databases, systems, semantic web, and machine learning. In this tutorial, we discuss the design of these emerging systems for processing of big-graphs, key features of distributed graph algorithms, as well as graph partitioning and workload balancing techniques. We discuss the current challenges and highlight some future research directions.
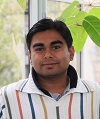
Bio: Arijit Khan is a post-doctorate researcher in the Systems group at ETH Zurich. His research interests span in the area of big-data, big-graphs, and graph systems. He received his PhD from the Department of Computer Science, University of California, Santa Barbara. Arijit is the recipient of the prestigious IBM PhD Fellowship in 2012-13. He co-presented a tutorial on emerging queries over linked data at ICDE 2012.
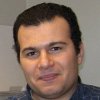
Bio: Sameh Elnikety is a researcher at Microsoft Research in Redmond, Washington. He received his Ph.D. from the Swiss Federal Institute of Technology (EPFL) in Lausanne, Switzerland , and M.S. from Rice University in Houston, Texas. His research interests include distributed server systems, and database systems. Sameh’s work on database replication received the best paper award at Eurosys 2007.
Demo 3
Location: Pearl
Chair: Demo 3 Chair


Ocelot/HyPE: Optimized Data Processing on Heterogeneous Hardware
Max Heimel* (TU Berlin),Sebastian Breß (Unversity of Magdeburg),Michael Saecker (Parstream GmbH),Bastian Koecher (Technische University Berlin),Volker Markl (TU Berlin),Gunter Saake (Unversity of Magdeburg)
The past years saw the emergence of highly heterogeneous server architectures that feature multiple accelerators in addition to the main processor. Efficiently exploiting these systems for data processing is a challenging research problem that comprises many facets, including how to find an optimal operator placement strategy, how to estimate runtime costs across different hardware architectures, and how to manage the code and maintenance blowup caused by having to support multiple architectures. In prior work, we already discussed solutions to some of these problems: First, we showed that specifying operators in a hardware-oblivious way can prevent code blowup while still maintaining competitive performance when supporting multiple architectures. Second, we presented learning cost functions and several heuristics to efficiently place operators across all available devices. In this demonstration, we provide further insights into this line of work by presenting our combined system Ocelot/HyPE. Our system integrates a hardware-oblivious data processing engine with a learning query optimizer for placement decisions, resulting in a highly adaptive DBMS that is specifically tailored towards heterogeneous hardware environments.


MoveMine2.0: Mining Object Relationships from Movement Data
Zhenhui Li (Penn State University),Fei Wu* (Penn State University),Tobias Kin Hou Lei (UIUC),Jiawei Han (University of Illinois)
The development in positioning technology has enabled us to collect a huge amount of movement data from moving objects, such as people, animals, and vehicles. The data embed rich information about the relationships among moving objects and have applications in many fields, e.g., in ecological study and human behavioral study. Previously, we propose a system MoveMine that integrates several start-of-art movement mining methods. However, it does not include recent methods on relationship pattern mining. Thus, we add substantial new methods and propose a new system, MoveMine 2.0, to support mining of dynamic relationship patterns. Newly added methods focus on two types of pairwise relationship patterns: (i) attraction/avoidance relationship, and (ii) following pattern. A user-friendly interface is designed to support interactive exploration of the result and provide flexibility in tuning the parameters. MoveMine 2.0 is tested on multiple types of real datasets to ensure its practical use. Our system provides useful tools for domain experts to gain insights on real dataset. Meanwhile, it will promote further research in relationship mining from moving objects.


WARP: A Partitioning Framework for Aggressive Data Skipping
Liwen Sun* (UC Berkeley),Sanjay Krishnan (UC Berkeley),Reynold Xin (UC Berkeley),Michael Franklin (UC Berkeley)
We propose to demonstrate a fine-grained partitioning framework that reorganizes the data tuples into small blocks at data loading time. The goal is to enable queries to maximally skip scanning data blocks. The partition framework consists of four steps: (1) workload analysis, which extracts features from a query workload, (2) augmentation, which augments each data tuple with a feature vector, (3) reduce, which succinctly represents a set of data tuples using a set of feature vectors, and (4) partitioning, which performs a clustering algorithm to partition the feature vectors and uses the clustering result to guide the actual data partitioning. Our experiments show that our techniques result in a 3-7x query response time improvement over traditional range partitioning due to more effective data skipping.


Interactive Outlier Exploration in Big Data Streams
Lei Cao* (WPI),Qingyang Wang (WPI),Elke Rundensteiner (WPI)
We demonstrate our VSOutlier system for supporting interactive exploration of outliers in big data streams. VSOutlier not only supports a rich variety of outlier types supported by innovative and efficient outlier detection strategies, but also provides a rich set of interactive interfaces to explore outliers in real time. Using the stock transactions dataset from the US stock market and the moving objects dataset from MITRE, we demonstrate that the VSOutlier system enables the analysts to more efficiently identify, understand, and respond to phenomena of interest in near real-time even when applied to high volume streams.


SQL/AA : Executing SQL on an Asymmetric Architecture
Quoc-Cuong To* (INRIA Rocquencourt UVSQ),Benjamin Nguyen (INRIA Rocquencourt University of Versailles),Philippe Pucheral (INRIA/UVSQ)
Current applications, from complex sensor systems (e.g. quantified self) to online e-markets acquire vast quantities of personal information which usually ends-up on central servers. Decentralized architectures, devised to help individuals keep full control of their data, hinder global treatments and queries, impeding the development of services of great interest. This paper promotes the idea of pushing the security to the edges of applications, through the use of secure hardware devices controlling the data at the place of their acquisition. To solve this problem, we propose secure distributed querying protocols based on the use of a tangible physical element of trust, reestablishing the capacity to perform global computations without revealing any sensitive information to central servers. There are two main problems when trying to support SQL in this context: perform joins and perform aggregations. In this paper, we study the subset of SQL queries without joins and show how to secure their execution in the presence of honest-but-curious attackers.


gMission: A General Spatial Crowdsourcing Platform
Zhao Chen* (HKUST),Rui Fu (HKUST),Ziyuan Zhao (HKUST),Zheng Liu (HKUST),Leihao Xia (HKUST),Lei Chen (Hong Kong University of Science and Technology),Peng Cheng (HKUST),Chen Cao (HKUST),Yongxin Tong (HKUST),CHEN ZHANG (HKUST)
As one of the successful forms of using Wisdom of Crowd, crowdsourcing, has been widely used for many human intrinsic tasks, such as image labeling, natural language understanding, market predication and opinion mining. Meanwhile, with advances in pervasive technology, mobile devices, such as mobile phones and tablets, have become extremely popular. These mobile devices can work as sensors to collect multimedia data(audios, images and videos) and location information. This power makes it possible to implement the new crowdsourcing mode: spatial crowdsourcing. In spatial crowdsourcing, a requester can ask for resources related a specific location, the mobile users who would like to take the task will travel to that place and get the data. Due to the rapid growth of mobile device uses, spatial crowdsourcing is likely to become more popular than general crowdsourcing, such as Amazon Turk and Crowdflower. However, to implement such a platform, effective and efficient solutions for worker incentives, task assignment, result aggregation and data quality control must be developed. In this demo, we will introduce gMission, a general spatial crowdsourcing platform, which features with a collection of novel techniques, including geographic sensing, worker detection, and task recommendation. We introduce the sketch of system architecture and illustrate scenarios via several case analysis.


S-Store: A Streaming NewSQL System for Big Velocity Applications
Ugur Cetintemel (Brown University),Daehyun Kim (Intel Labs),Tim Kraska (Brown University),Samuel Madden (MIT CSAIL),David Maier (Portland State University),John Meehan (Brown University),Andy Pavlo (CMU),Michael Stonebraker (MIT CSAIL),Nesime Tatbul* (Intel)
First-generation streaming systems did not pay much attention to state management via ACID transactions. S-Store is a data management system that combines OLTP transactions with stream processing. To create S-Store, we begin with H-Store, a main-memory transaction processing engine, and add primitives to support streaming. This includes triggers and transaction workflows to implement push-based processing, windows to provide a way to bound the computation, and tables with hidden state to implement scoping for proper isolation. This demo explores the benefits of this approach by showing how a naïve implementation of our benchmarks using only H-Store can yield incorrect results. We also show that by exploiting push-based semantics and our implementation of triggers, we can achieve significant improvement in transaction throughput. We demo two modern applications: (i) leaderboard maintenance for a version of "American Idol", and (ii) a city-scale bicycle rental scenario.


CLEar: A Realtime Online Observatory for Bursty and Viral Events
Runquan Xie* (Singapore Management Universit),Feida Zhu (Singapore Management Universit),Hui Ma (Singapore Management University),Wei Xie (Singapore Management University),Chen Lin (Xiamen University)
We describe our demonstration of CLEar (Clairaudient Ear ), a real-time online platform for detecting, monitoring, summarizing, contextualizing and visualizing bursty and viral events, those triggering a sudden surge of public interest and going viral on micro-blogging platforms. This task is challenging for existing methods as they either use complicated topic models to analyze topics in a off-line manner or define temporal structure of fixed granularity on the data stream for online topic learning, leaving them hardly scalable for real-time stream like that of Twitter. In this demonstration of CLEar, we present a three-stage system: First, we show a real-time bursty event detection module based on a data-sketch topic model which makes use of acceleration of certain stream quantities as the indicators of topic burstiness to trigger efficient topic inference. Second, we demonstrate popularity prediction for the detected bursty topics and event summarization based on clustering related topics detected in successive time periods. Third, we illustrate CLEar’s module for contextualizing and visualizing the event evolution both along time-line and across other news media to offer an easier understanding of the events.


AZDBLab: A Laboratory Information System for a Large-scale Empirical DBMS Study
Young-Kyoon Suh* (University of Arizona),Richard Snodgrass (University of Arizona),Rui Zhang (Teradata)
In the database field, while very strong mathematical and engineering work has been done, the scientific approach has been much less prominent. The deep understanding of query optimizers obtained through the scientific approach can lead to better engineered designs. Unlike other domains, there have been few DBMS-dedicated laboratories, focusing on such scientific investigation. In this demonstration, we present a novel DBMS-oriented research infrastructure, called Arizona Database Laboratory (AZDBLab), to assist database researchers in conducting a large-scale empirical study across multiple DBMSes. For them to test their hypotheses on the behavior of query optimizers, AZDBLab can run and monitor a large-scale experiment with thousands (or millions) of queries on different DBMSes. Furthermore, AZDBLab can help users automatically analyze these queries. In the demo, the audience will interact with AZDBLab through the stand-alone application and the mobile app to conduct such a large-scale experiment for a study. The audience will then run a Tucson Timing Protocol analysis on the finished experiment and then see the analysis (data sanity check and timing) results.


Terrain-Toolkit: A Multi-Functional Tool for Terrain Data
Qi Wang (Zhejiang University),Manohar Kaul (Aarhus University),Cheng Long* (HKUST),Raymond Chi-Wing Wong (Hong Kong University of Science and Technology)
Terrain data is becoming increasingly popular both in industry and in academia. Many tools have been developed for visualizing terrain data. However, we find that (1) they usually accept very few data formats of terrain data only; (2) they do not support terrain simplification well which, as will be shown, is used heavily for query processing in spatial databases; and (3) they do not provide the surface distance operator which is fundamental for many applications based on terrain data. Motivated by this, we developed a tool called Terrain-Toolkit for terrain data which accepts a comprehensive set of data formats, supports terrain simplification and provides the surface distance operator.


FORWARD: Data-Centric UIs using Declarative Templates that Efficiently Wrap Third-Party JavaScript Components
Kian Win Ong* (UCSD),Yannis Papakonstantinou (UC San Diego),Erick Zamora (UCSD)
While Ajax programming and the plethora of JavaScript component libraries enable high-quality UIs in web applications, integrating them with page data is laborious and error-prone as a developer has to handcode incremental modifications with trigger-based programming and manual coordination of data dependencies. The FORWARD web framework simplifies the development of Ajax applications through declarative, state-based templates. This declarative, data-centric approach is characterized by the principle of logical/physical independence, which the database community has often deployed successfully. It enables FORWARD to leverage database techniques, such as incremental view maintenance, updatable views, capability-based component wrappers and cost-based optimization to automate efficient live visualizations. We demonstrate an end-to-end system implementation, including a web-based IDE (itself built in FORWARD), academic and commercial applications built in FORWARD and a wide variety of JavaScript components supported by the declarative templates.
Tuesday Sep 2nd 17:15-17:30
Buses to West Lake Show
Location: Crystal
Chair: Buses to West Lake Show
Buses to West Lake Show
Wednesday Sep 3rd 08:30-10:00
Industrial Keynote: Shivakumar Venkataraman;Academic Keynote: Divyakant Agrawal
Location: Crystal
Chair: Beng Chin Ooi


Datacenters as Computers: Google Engineering & Database Research Perspectives
Shivakumar Venkataraman (Google),Divyakant Agrawal (University of California at Santa Barbara)
In this collaborative keynote address, we will share Google's experience in building a scalable data infrastructure that leverages datacenters for managing Google's advertising data over the last decade. In order to support the massive online advertising platform at Google, the data infrastructure must simultaneously support both transactional and analytical workloads. The focus of this talk will be to highlight how the datacenter architecture and the cloud computing paradigm has enabled us to manage the exponential growth in data volumes and user queries, make our services highly available and fault tolerant to massive datacenter outages, and deliver results with very low latencies. We note that other Internet companies have also undergone similar growth in data volumes and user queries. In fact, this phenomenon has resulted in at least two new terms in the technology lexicon: big data and cloud computing. Cloud computing (and datacenters) have been largely responsible for scaling the data volumes from terabytes range just a few years ago to now reaching in the exabyte range over the next couple of years. Delivering solutions at this scale that are fault-tolerant, latency sensitive, and highly available requires a combination of research advances with engineering ingenuity at Google and elsewhere. Next, we will try to answer the following question: is a datacenter just another (very large) computer? Or, does it fundamentally change the design principles for data-centric applications and systems. We will conclude with some of the unique research challenges that need to be addressed in order to sustain continuous growth in data volumes while supporting high throughput and low latencies.
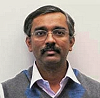
Bio: Shivakumar Venkataraman is Vice President of Engineering for Google's Advertising Infrastructure and Payments Systems. He received his BS in Computer Science from IIT, Madras in 1990 and received his MS and PhD in Computer Science from University of Wisconsin at Madison in 1991 and 1996 respectively. From 1996 to 2000, he worked as an Advisory Software Engineer with IBM working on the development of IBM's federated query optimizers and associated technologies. After leaving IBM in 2000, he worked with Cohera Corporation, PeopleSoft, Required Technologies, and AdeSoft. He also served as a Visiting Faculty member at UC Berkeley in 2002. He has been with Google since 2003 . At Google, Dr. Venkataraman is recognized for the vision in the development of critical technologies for databases: scalable distributed database management system F1, scalable data warehousing solution Mesa, scalable log-processing system Photon, among others.
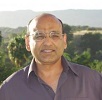
Bio: Divyakant Agrawal is a Professor of Computer Science and the Director of Engineering Computing Infrastructure at the University of California at Santa Barbara. His research expertise is in the areas of database systems, distributed computing, data warehousing, and large-scale information systems. Divy Agrawal is an ACM Distinguished Scientist (2010), an ACM Fellow (2012), and an IEEE Fellow (2012). His current interests are in the areas of scalable data management and data analysis in cloud computing environments, security and privacy of data in the cloud, and scalable analytics over social networks data and social media. In 2013-14, he was on a sabbatical leave from UCSB and served as a Visiting Scientist in the Advertising Infrastructure Group at Google, Inc. in Mountain View, CA. In 2014-15, he will be on leave from UCSB and will serve as a Director of Research in Data Analytics at Qatar Computing Research Institute.
Wednesday Sep 3rd 10:30-12:15
Papers : Paths and Trajectories
Location: Diamond 1
Chair: Wenfei Fan


Finding Shortest Paths on Terrains by Killing Two Birds with One Stone
Manohar Kaul* (Aarhus University), Raymond Chi-Wing Wong (Hong Kong University of Science and Technology), Bin Yang (Aarhus University), Christian Jensen (Aarhus University)
With the increasing availability of terrain data, e.g., from aerial laser scans, the management of such data is attracting increasing attention in both industry and academia. In particular, spatial queries, e.g., k-nearest neighbor and reverse nearest neighbor queries, in Euclidean and spatial network spaces are being extended to terrains. Such queries all rely on an important operation, that of finding shortest surface distances. However, shortest surface distance computation is very time consuming. We propose techniques that enable efficient computation of lower and upper bounds of the shortest surface distance, which enable faster query processing by eliminating expensive distance computations. Empirical studies show that our bounds are much tighter than the best-known bounds in many cases and that they enable speedups of up to 43 times for some well-known spatial queries.


On k-Path Covers and their Applications
Stefan Funke* (Universitaet Stuttgart), Andre Nusser (Universitaet Stuttgart), Sabine Storandt (Universitaet Freiburg)
For a directed graph G with vertex set V we call a subset C of V a k-(All-)Path Cover if C contains a node from any path consisting of k nodes. This paper considers the problem of constructing small k-Path Covers in the context of road networks with millions of nodes and edges. In many application scenarios the set C and its induced overlay graph constitute a very compact synopsis of G which is the basis for the currently fastest data structure for personalized shortest path queries, visually pleasing overlays of subsampled paths, and efficient reporting, retrieval and aggregation of associated data in spatial network databases. Apart from a theoretical investigation of the problem, we provide efficient algorithms that produce very small k-Path Covers for large real-world road networks (with a posteriori guarantees via instance-based lower bounds).


Approximate MaxRS in Spatial Databases
Yufei Tao* (Chinese University of Hong Kong), Xiaocheng Hu (CUHK), dong-Wan Choi (KAIST), Chin-Wan Chung (KAIST)
In the {\em maximizing range sum} (MaxRS) problem, given (i) a set $P$ of 2D points each of which is associated with a positive weight, and (ii) a rectangle $r$ of specific extents, we need to decide where to place $r$ in order to maximize the {\em covered weight} of $r$ -- that is, the total weight of the data points covered by $r$. Algorithms solving the problem exactly entail expensive CPU or I/O cost. In practice, exact answers are often not compulsory in a MaxRS application, where slight imprecision can often be comfortably tolerated, provided that approximate answers can be computed considerably faster. Motivated by this, the present paper studies the {\em $(1-\eps)$-approximate MaxRS problem}, which admits the same inputs as MaxRS, but aims instead to return a rectangle whose covered weight is at least $(1-\eps) m^\*$, where $m^\*$ is the optimal covered weight, and $\eps$ can be an arbitrarily small constant between 0 and 1. We present fast algorithms that settle this problem with strong theoretical guarantees.


PRESS: A Novel Framework of Trajectory Compression in Road Networks
Renchu Song (Fudan University), Weiwei Sun* (Fudan University), Baihua Zheng (Singapore Management University), Yu Zheng (Microsoft Research Asia)
Location data becomes more and more important. In this paper, we focus on the trajectory data, and propose a new framework, namely PRESS (Paralleled Road-Network-Based Trajectory Compression), to effectively compress trajectory data under road network constraints. Different from existing work, PRESS proposes a novel representation for trajectories to separate the spatial representation of a trajectory from the temporal representation, and proposes a Hybrid Spatial Compression (HSC) algorithm and error Bounded Temporal Compression (BTC) algorithm to compress the spatial and temporal information of trajectories respectively. PRESS also supports common spatial-temporal queries without fully decompressing the data. Through an extensive experimental study on real trajectory dataset, PRESS significantly outperforms existing approaches in terms of saving storage cost of trajectory data with bounded errors.


Probabilistic Nearest Neighbor Queries on Uncertain Moving Object Trajectories
Johannes Niedermayer* (LMU Munich), Andreas Züfle (Ludwig-Maximilians-Universitat M¨ unchen), Tobias Emrich (University of Munich), Matthias Renz (Ludwig-Maximilians University Munich), Nikos Mamoulis (University of Hong Kong), Lei Chen (Hong Kong University of Science and Technology), Hans-Peter Kriegel (Ludwig-Maximilians-Universitat M¨ unchen)
Nearest neighbor (NN) queries in trajectory databases have received significant attention in the past, due to their applications in spatio-temporal data analysis. More recent work has considered the realistic case where the trajectories are uncertain; however, only simple uncertainty models have been proposed, which do not allow for accurate probabilistic search. In this paper, we fill this gap by addressing probabilistic nearest neighbor queries in databases with uncertain trajectories modeled by stochastic processes, specifically the Markov chain model. We study three nearest neighbor query semantics that take as input a query state or trajectory $q$ and a time interval, and theoretically evaluate their runtime complexity. Furthermore we propose a sampling approach which uses Bayesian inference to guarantee that sampled trajectories conform to the observation data stored in the database. This sampling approach can be used in Monte-Carlo based approximation solutions. We include an extensive experimental study to support our theoretical results.


Authenticating Top-k Queries in Location-based Services with Confidentiality
Qian Chen* (HKBU), Haibo Hu (Hong Kong Baptist University), Jianliang Xu (Hong Kong Baptist University)
State-of-the-art location-based services (LBSs) involve data owners, requesting clients, and service providers. As LBSs become new business opportunities, there is an increasing necessity to verify the genuineness of service results. Unfortunately, while traditional query authentication techniques can address this issue, they fail to protect the confidentiality of data, which is sensitive location information when LBSs are concerned. Recent work has studied how to preserve such location privacy in query authentication. However, the prior work is limited to range queries, where private values only appear on one side of the range comparison. In this paper, we address the more challenging authentication problem on top-k queries, where private values appear on both sides of a comparison. To start with, we propose two novel cryptographic building blocks, followed by a comprehensive design of authentication schemes for top-k queries based on R-tree and Power Diagram indexes. Optimizations, security analysis, and experimental results consistently show the effectiveness and robustness of the proposed schemes under various system settings and query workloads.
Papers : Text, XML, and String Data
Location: Diamond 2
Chair: Yunyao Li


Supporting Keyword Search in Product Database: A Probabilistic Approach
Huizhong Duan* (University of Illinois), ChengXiang Zhai (University of Illinois), Jinxing Cheng (WalmartLabs), Abhishek Gattani (WalmartLabs)
The ability to let users search for products conveniently in product database is critical to the success of e-commerce. Although structured query languages (e.g. SQL) can be used to effectively access the product database, it is very difficult for end users to learn and use. In this paper, we study how to optimize search over structured product entities (represented by specifications) with keyword queries such as ``cheap gaming laptop". One major difficulty in this problem is the vocabulary gap between the specifications of products in the database and the keywords people use in search queries. To solve the problem, we propose a novel probabilistic entity retrieval model based on query generation, where the entities would be ranked for a given keyword query based on the likelihood that a user who likes an entity would pose the query. Different ways to estimate the model parameters would lead to different variants of ranking functions. We start with simple estimates based on the specifications of entities, and then leverage user reviews and product search logs to improve the estimation. Multiple estimation algorithms are developed based on Maximum Likelihood and Maximum a Posteriori estimators. We evaluate the proposed product entity retrieval models on two newly created product search test collections. The results show that the proposed model significantly outperforms the existing retrieval models, benefiting from the modeling of attribute-level relevance. Despite the focus on product retrieval, the proposed modeling method is general and opens up many new opportunities in analyzing structured entity data with unstructured text data. We show the proposed probabilistic model can be easily adapted for many interesting applications including facet generation and review annotation.


Efficient and Effective KNN Sequence Search with Approximate n-grams
Xiaoli Wang* (NUS), Xiaofeng Ding (HUST), Anthony Tung (National University of Singapore), Zhenjie Zhang (Advanced Digital Science Center)
In this paper, we address the problem of finding $k$-nearest neighbors (KNN) in sequence databases using the edit distance. Unlike most existing works using short and exact $n$-gram matchings together with a filter-and-refine framework for KNN sequence search, our new approach allows us to use longer but approximate $n$-gram matchings as a basis of KNN candidates pruning. Based on this new idea, we devise a pipeline framework over a two-level index for searching KNN in the sequence database. By coupling this framework together with several efficient filtering strategies, i.e. the frequency queue and the well-known Combined Algorithm (CA), our proposal brings various enticing advantages over existing works, including 1) huge reduction on false positive candidates to avoid large overheads on candidate verifications; 2) progressive result update and early termination; and 3) good extensibility to parallel computation. We conduct extensive experiments on three real datasets to verify the superiority of the proposed framework.


When Speed Has a Price: Fast Information Extraction Using Approximate Algorithms
Gonçalo Simões* (INESC-ID and Instituto Superior Técnico), Helena Galhardas (INESC-ID and Instituto Superior Técnico), Luis Gravano (Columbia University)
A wealth of information produced by individuals and organizations is expressed in natural language text. This is a problem since text lacks the explicit structure that is necessary to support rich querying and analysis. Information extraction systems are sophisticated software tools to discover structured information in natural language text. Unfortunately, information extraction is a challenging and time-consuming task. In this paper, we address the limitations of state-of-the-art systems for the optimization of information extraction programs, with the objective of producing efficient extraction executions. Our solution relies on exploiting a wide range of optimization opportunities. For efficiency, we consider a wide spectrum of execution plans, including approximate plans whose results differ in their precision and recall. Our optimizer accounts for these characteristics of the competing execution plans, and uses accurate predictors of their extraction time, recall, and precision. We demonstrate the efficiency and effectiveness of our optimizer through a large-scale experimental evaluation over real-world datasets and multiple extraction tasks and approaches.


String Similarity Joins: An Experimental Evaluation
Yu Jiang (Tsinghua University), Guoliang Li* (Tsinghua University), Jianhua Feng (Tsinghua University), Wen-syan Li (SAP)
String similarity join is an important operation in data integration and cleaning that finds similar string pairs from two collections of strings. More than ten algorithms have been proposed to address this problem in the recent two decades. However, existing algorithms have not been thoroughly compared under the same experimental framework. For example, some algorithms are tested only on specific datasets. This makes it rather difficult for practitioners to decide which algorithms should be used for various scenarios. To address this problem, in this paper we provide a comprehensive survey on a wide spectrum of existing string similarity join algorithms, classify them into different categories based on their main techniques, and compare them through extensive experiments on a variety of real-world datasets with different characteristics. We also report comprehensive findings obtained from the experiments and provide new insights about the strengths and weaknesses of existing algorithms which can guide practitioners to select appropriate algorithms for various scenarios.


Scalable XML Query Processing using Parallel Pushdown Transducers
Peter Ogden* (Imperial College London), David Thomas (Imperial College London London), Peter Pietzuch (Imperial College London)
In online social networking, network monitoring and financial applications, there is a need to query high rate streams of XML data, but methods for executing individual XPath queries on streaming XML data have not kept pace with multicore CPUs. For data-parallel processing, a single XML stream is typically split into well-formed fragments, which are then processed independently. Such an approach, however, introduces a sequential bottleneck and suffers from low cache locality, limiting its scalability across CPU cores.We describe a data-parallel approach for the processing of streaming XPath queries based on pushdown transducers. Our approach permits XML data to be split into arbitrarily sized chunks, with each chunk processed by a parallel automaton instance. Since chunks may be malformed, our automata consider all possible starting states for XML elements and build mappings from starting to finishing states. These mappings can be constructed independently for each chunk by different CPU cores. For streaming queries from the XPathMark benchmark, we show a processing throughput of 2.5 GB/s, with near linear scaling up to 64 CPU cores.


Synthetising Changes in XML Documents as PULs
Federico Cavalieri (University of Genoa), Alessandro Solimando* (University of Genoa), Giovanna Guerrini (University of Genoa)
The ability of efficiently detecting changes in XML documents is crucial in many application contexts. If such changes are represented as XQuery Update Pending Update Lists (PULs), they can then be applied on documents using XQuery Update engines, and document management can take advantage of existing composition, inversion, reconciliation approaches developed in the update processing context. The paper presents an XML edit-script generator with the unique characteristic of using PULs as edit-script language and improving the state of the art from both the performance and the generated edit-script quality perspectives.
Industrial : Transactions
Location: Diamond 3
Chair: Andy Pavlo


Reducing Database Locking Contention Through Multi-version Concurrency
Mohammad Sadoghi* (IBM T.J. Watson Research Cente)*,Mustafa Canim (IBM T.J. Watson Research Center),Bishwaranjan Bhattacharjee (IBM T.J. Watson Research Center),Fabian Nagel (University of Edinburgh),Kenneth Ross (Columbia University)
In multi-version databases, updates and deletions of records by transactions require appending a new record to tables rather than performing in-place updates. This mechanism incurs non-negligible performance overhead in the presence of multiple indexes on a table, where changes need to be propagated to all indexes. Additionally, an uncommitted record update will block other active transactions from using the index to fetch the most recently committed values for the updated record. In general, in order to support snapshot isolation and/or multi-version concurrency, either each active transaction is forced to search a database temporary area (e.g., rollback segments) to fetch old values of desired records, or each transaction is forced to scan the entire table to find the older versions of the record in a multi-version database (in the absence of specialized temporal indexes). In this work, we describe a novel kV-Indirection structure to enable efficient (parallelizable) optimistic and pessimistic multi-version concurrency control by utilizing the old versions of records (at most two versions of each record) to provide direct access to the recent changes of records without the need of temporal indexes. As a result, our technique results in higher degree of concurrency by reducing the clashes between readers and writers of data and avoiding extended lock delays. We have a working prototype of our concurrency model and kV-Indirection structure in a commercial database and conducted an extensive evaluation to demonstrate the benefits of our multi-version concurrency control, and we obtained orders of magnitude speed up over the single-version concurrency control.


TPC-DI: The First Industry Benchmark for Data Integration
Meikel Poess* (Oracle)*,Tilmann Rabl (University of Toronto),Hans-Arno Jacobsen (University of Toronto),Brian Caufield (IBM)
Historically, the process of synchronizing a decision support system with data from operational systems has been referred to as Extract, Transform, Load (ETL) and the tools supporting such process have been referred to as ETL tools. Recently, ETL was replaced by the more comprehensive acronym, data integration (DI). DI describes the process of extracting and combining data from a variety of data source formats, transforming that data into a unified data model representation and loading it into a data store. This is done in the context of a variety of scenarios, such as data acquisition for business intelligence, analytics and data warehousing, but also synchronization of data between operational applications, data migrations and conversions, master data management, enterprise data sharing and delivery of data services in a service-oriented architecture context, amongst others. With these scenarios relying on up-to-date information it is critical to implement a highly performing, scalable and easy to maintain data integration system. This is especially important as the complexity, variety and volume of data is constantly increasing and performance of data integration systems is becoming very critical. Despite the significance of having a highly performing DI system, there has been no industry standard for measuring and comparing their performance. The TPC, acknowledging this void, has released TPC-DI, an innovative benchmark for data integration. This paper motivates the reasons behind its development, describes its main characteristics including workload, run rules, metric, and explains key decisions.


Fuxi: a Fault-Tolerant Resource Management and Job Scheduling System at Internet Scale
Zhuo Zhang (Alibaba Cloud Computing)*,Jie Xu (Beihang University, University of Leeds)
Scalability and fault-tolerance are two fundamental challenges for all distributed computing at Internet scale. Despite many recent advances from both academia and industry, these two problems are still far from settled. In this paper, we present Fuxi, a resource management and job scheduling system that is capable of handling the kind of workload at Alibaba where hundreds of terabytes of data are generated and analyzed everyday to help optimize the company's business operations and user experiences. We employ several novel techniques to enable Fuxi to perform efficient scheduling of hundreds of thousands of concurrent tasks over large clusters with thousands of nodes: 1) an incremental resource management protocol that supports multi-dimensional resource allocation and elastic quota; 2) user-transparent failure recovery where failures of any Fuxi components will not impact the execution of user jobs; and 3) an effective faulty detection mechanism and multi-level blacklisting that prevents them from affecting job execution. Our evaluation results demonstrate that 95% and 91% scheduled CPU/memory utilization can be fulfilled under synthetic workloads, and Fuxi is capable of achieving 2.36TB/minute throughput in GraySort. Additionally, the same Fuxi job only experiences approximately 16% slowdown under a 5% fault-injection rate. Fuxi has been deployed in our production environment since 2009, and it now manages hundreds of thousands of server nodes.


CAP limits in telecom subscriber database design
Javier Arauz* (Ericsson)*
While the notion of a Distributed DBMS has been familiar to the IT industry for several decades, within telecom networks the subscriber data management based on DDBMS technology is a novel addition to a service provider's infrastructure. Service providers are used to telecom networks that are efficient, reliable and easy to maintain and operate, in part thanks to the node model used in designing such networks. A DDBMS spanning a large geographical area however incurs into distributed systems issues not previously seen in telecom networks. Identifying and delivering the right set of trade-offs that satisfies the service providers' needs while staying within the known physical bounds of a distributed system is therefore crucial if DDBMS are to conquer the subscriber management space within telecom networks.
Papers : Privacy and Security
Location: Diamond 4
Chair: Feifei Li


SPARSI: Partitioning Sensitive Data amongst Multiple Adversaries
Theodoros Rekatsinas* (University of Maryland), Amol Deshpande (University of Maryland), Ashwin Machanavajjhala (Duke University)
We present SPARSI, a novel theoretical framework for partitioning sensitive data across multiple non-colluding adversaries. Most work in privacy-aware data sharing has considered disclosing summaries where the aggregate information about the data is preserved, but sensitive user information is protected. Nonetheless, there are applications, including online advertising, cloud computing and crowdsourcing markets, where detailed and fine-grained user-data must be disclosed. We consider a new data sharing paradigm and introduce the problem of privacy-aware data partitioning, where a sensitive dataset must be partitioned among k untrusted parties (adversaries). The goal is to maximize the utility derived by partitioning and distributing the dataset, while minimizing the total amount of sensitive information disclosed. The data should be distributed so that an adversary, without colluding with other adversaries, cannot draw additional inferences about the private information, by linking together multiple pieces of information released to her. The assumption of no collusion is both reasonable and necessary in the above application domains that require release of private user information. SPARSI enables us to formally define privacy-aware data partitioning using the notion of sensitive properties for modeling private information and a hypergraph representation for describing the interdependencies between data entries and private information. We show that solving privacy-aware partitioning is, in general, NP-hard, but for specific information disclosure functions, good approximate solutions can be found using relaxation techniques. Finally, we present a local search algorithm applicable to generic information disclosure functions. We conduct a rigorous performance evaluation with real-world and synthetic datasets that illustrates the effectiveness of SPARSI at partitioning sensitive data while minimizing disclosure.


Understanding Hierarchical Methods for Differentially Private Histograms
Wahbeh Qardaji* (Purdue University), Weining Yang (Purdue University), Ninghui Li (Purdue University)
In recent years, many approaches to differentially privately publish histograms have been proposed. Several approaches rely on constructing tree structures in order to decrease the error when answer large range queries. In this paper, we examine the factors affecting the accuracy of hierarchical approaches by studying the mean squared error (MSE) when answering range queries. We start with one-dimensional histograms, and analyze how the MSE changes with different branching factors, after employing constrained inference, and with different methods to allocate the privacy budget among hierarchy levels. Our analysis and experimental results show that combining the choice of a good branching factor with constrained inference outperform the current state of the art. Finally, we extend our analysis to multi-dimensional histograms. We show that the benefits from employing hierarchical methods beyond a single dimension are significantly diminished, and when there are 3 or more dimensions, it is almost always better to use the Flat method instead of a hierarchy.


Data- and Workload-Aware Query Answering Under Differential Privacy
Chao Li* (University of Massachusetts), Michael Hay (Colgate University), Gerome Miklau (University of Massachusetts), Yue Wang (University of Massachusetts Amherst)
We describe a new algorithm for answering a given set of range queries under epsilon-differential privacy which often achieves substantially lower error than competing methods. Our algorithm satisfies differential privacy by adding noise that is adapted to the input data and to the given query set. We first privately learn a partitioning of the domain into buckets that suit the input data well. Then we privately estimate counts for each bucket, doing so in a manner well-suited for the given query set. Since the performance of the algorithm depends on the input database, we evaluate it on a wide range of real datasets, showing that we can achieve the benefits of data-dependence on both ``easy'' and ``hard'' databases.


Optimal Security-Aware Query Processing
Marco Guarnieri* (Institute of Information Security (ETH Zurich), David Basin (Institute of Information Security (ETH Zurich)
Security-Aware Query Processing is the problem of computing answers to queries in the presence of access control policies. We present general impossibility results for the existence of optimal algorithms for Security-Aware Query Processing and classify query languages for which such algorithms exist. In particular, we show that for the relational calculus there are no optimal algorithms, whereas optimal algorithms exist for some of its fragments, such as the existential fragment. We also establish relationships between two different models of Fine-Grained Access Control, called Truman and Non-Truman models, which have been previously presented in the literature as distinct. For optimal Security-Aware Query Processing, we show that the Non-Truman model is a special case of the Truman model for boolean queries in the relational calculus, moreover the two models coincide for more powerful languages, such as the relational calculus with aggregation operators. In contrast, these two models are distinct for non-boolean queries.


A Framework for Protecting Worker Location Privacy in Spatial Crowdsourcing
Hien To* (University of Southern Califor), Gabriel Ghinita (University of Massachusetts Boston), Cyrus Shahabi (USC)
{\em Spatial Crowdsourcing (SC)} is a transformative platform that engages individuals, groups and communities in the act of collecting, analyzing, and disseminating environmental, social and other spatio-temporal information. The objective of SC is to outsource a set of spatio-temporal tasks to a set of {\em workers}, i.e., individuals with mobile devices that perform the tasks by physically traveling to specified locations of interest. However, current solutions require the workers, who in many cases are simply volunteering for a cause, to disclose their locations to untrustworthy entities. In this paper, we introduce a framework for protecting location privacy of workers participating in SC tasks. We argue that existing location privacy techniques are not sufficient for SC, and we propose a mechanism based on differential privacy and geocasting that achieves effective SC services while offering privacy guarantees to workers. We investigate analytical models and task assignment strategies that balance multiple crucial aspects of SC functionality, such as task completion rate, worker travel distance and system overhead. Extensive experimental results on real-world datasets show that the proposed technique protects workers' location privacy without incurring significant performance metrics penalties.


Calibrating Data to Sensitivity in Private Data Analysis, A Platform for Differentially-Private Analysis of Weighted Datasets
Davide Proserpio* (Boston University), Sharon Goldberg (Boston University), Frank McSherry (Microsoft)
We present an approach to differentially private computation in which one does not scale up the magnitude of noise for challenging queries, but rather scales down the contributions of challenging records. While scaling down all records uniformly is equivalent to scaling up the noise magnitude, we show that scaling records non-uniformly can result in substantially higher accuracy by bypassing the worst-case requirements of differential privacy for the noise magnitudes. This paper details the data analysis platform wPINQ, which generalizes the Privacy Integrated Query (PINQ) to weighted datasets. Using a few simple operators (including a non-uniformly scaling Join operator) wPINQ can reproduce (and improve) several recent results on graph analysis and introduce new generalizations (e.g., counting triangles with given degrees). We also show how to integrate probabilistic inference techniques to synthesize datasets respecting more complicated (and less easily interpreted) measurements.
Papers : Data Curation
Location: Diamond 5
Chair: Ihab Ilyas


Scalable Discovery of Unique Column Combinations
Arvid Heise* (Hasso-Plattner Institute), Jorge Quiané-Ruiz (QCRI), Ziawasch Abedjan (Hasso-Plattner Institute), Anja Jentzsch (Hasso-Plattner Institute), Felix Naumann (Hasso-Plattner Institute)
The discovery of all unique (and non-unique) column combinations in a given dataset is at the core of any data profiling effort. The results are useful for a large number of areas of data management, such as anomaly detection, data integration, data modeling, duplicate detection, indexing, and query optimization. However, discovering all unique and non-unique column combinations is an NP-hard problem, which in principle requires to verify an exponential number of column combinations for uniqueness on all data values. Thus, achieving efficiency and scalability in this context is a tremendous challenge by itself. In this paper, we devise DUCC, a scalable and efficient approach to the problem of finding all unique and non-unique column combinations in big datasets. We first model the problem as a graph coloring problem and analyze the pruning effect of individual combinations. We then present our hybrid column-based pruning technique, which traverses the lattice in a depth-first and random walk combination. This strategy allows DUCC to typically depend on the solution set size and hence to prune large swaths of the lattice. DUCC also incorporates row-based pruning to run uniqueness checks in just few milliseconds. To achieve even higher scalability, DUCC runs on several CPU cores (scale-up) and compute nodes (scale-out) with a very low overhead. We exhaustively evaluate DUCC using three datasets (two real and one synthetic) with several millions rows and hundreds of attributes. We compare DUCC with related work: Gordian and HCA. The results show that DUCC is up to more than 2 orders of magnitude faster than Gordian and HCA (631x faster than Gordian and 398x faster than HCA). Finally, a series of scalability experiments shows the efficiency of DUCC to scale up and out.


Discovering Denial Constraints
Xu Chu (University of Waterloo), Ihab Ilyas ( University of Waterloo), Paolo Papotti* (QCRI)
Integrity constraints (ICs) provide a valuable tool for enforcing correct application semantics. However, designing ICs requires experts and time. Proposals for automatic discovery have been made for some formalisms, such as functional dependencies (FDs) and their extension conditional functional dependencies (CFDs). Unfortunately, these dependencies cannot express many common business rules. For example, an American citizen cannot have lower salary and higher tax rate than another citizen in the same state. In this paper, we tackle the challenges of discovering dependencies in a more expressive integrity constraint language, namely Denial Constrains (DCs). DCs are expressive enough to overcome the limits of previous languages and, at the same time, have enough structure to allow efficient discovery and application in several scenarios. We lay out theoretical and practical foundations for DCs, including a set of sound inference rules and a linear algorithm for implication testing. We then develop an efficient instance-driven DC discovery algorithm (FASTDC) and propose a novel scoring function to rank DCs for user validation. Using real-world and synthetic datasets, we experimentally evaluate the scalability and effectiveness of our proposed solution.


Repairing Vertex Labels under Neighborhood Constraints
Shaoxu Song* (Tsinghua University), Hong Cheng (The Chinese University of Hong Kong), Jeffrey Xu Yu (The Chinese University of Hong Kong), Lei Chen (Hong Kong University of Science and Technology)
A broad class of data, ranging from similarity networks, workflow networks to protein networks, can be modeled as graphs with data values as vertex labels. The vertex labels (data values) are often dirty for various reasons such as typos or erroneous reporting of results in scientific experiments. Neighborhood constraints, specifying label pairs that are allowed to appear on adjacent vertexes in the graph, are employed to detect and repair erroneous vertex labels. In this paper, we study the problem of repairing vertex labels to make graphs satisfy neighborhood constraints. Unfortunately, the relabeling problem is proved to be NP-hard, which motivates us to devise approximation methods for repairing, and identify in- teresting special cases (star and clique constraints) that can be efficiently solved. We propose several approximate repairing algorithms including greedy heuristics, contraction method and a hybrid approach. The performances of algorithms are also analyzed for the special case. Our extensive experimental evaluation, on both synthetic and real data, demonstrates the effectiveness of eliminating frauds in several types of application networks. Remarkably, the hybrid method performs well in practice, i.e., guarantees termiation, while achieving high effectiveness at the same time.


Query-Driven Approach to Entity Resolution
Hotham Altwaijry* (University of california - Irv), Dmitri Kalashnikov (UC Irvine), Sharad Mehrotra (University of California (Irvine)
This paper explores "on-the-fly" data cleaning in the context of a user query. A novel Query-Driven Approach (QDA) is developed that performs a minimal number of cleaning steps that are only necessary to answer a given selection query correctly. The comprehensive empirical evaluation of the proposed approach demonstrates its significant advantage in terms of efficiency over traditional techniques for query-driven applications.


Progressive Approach to Relational Entity Resolution
Yasser Altowim* (UC Irvine), Dmitri Kalashnikov (University of California- Irvine), sharad Mehrotra (University of California- Irvine)
This paper proposes a progressive approach to entity resolution (ER) that allows users to explore a trade-off between the resolution cost and the achieved quality of the resolved data. In particular, our approach aims to produce the highest quality result given a constraint on the resolution budget, specified by the user. Our proposed method monitors and dynamically reassesses the resolution progress to determine which parts of the data should be resolved next and how they should be resolved. The comprehensive empirical evaluation of the proposed approach demonstrates its significant advantage in terms of efficiency over the traditional ER techniques for the given problem settings.


Crowdsourcing Algorithms for Entity Resolution
Norases Vesdapunt* (Stanford University), Kedar Bellare (Facebook), Nilesh Dalvi (Facebook)
In this paper, we study a hybrid human-machine approach for solving the problem of Entity Resolution (ER). The goal of ER is to identify all records in a database that refer to the same underlying entity, and are therefore duplicates of each other. Our input is a graph over all the records in a database, where each edge has a probability denoting our prior belief (based on Machine Learning models) that the pair of records represented by the given edge are duplicates. Our objective is to resolve all the duplicates by asking humans to verify the equality of a subset of edges, leveraging the transitivity of the equality relation to infer the remaining edges (e.g. a = c can be inferred given a = b and b = c). We consider the problem of designing optimal strategies for asking questions to humans that minimize the expected number of questions asked. Using our theoretical framework, we analyze several strategies, and show that a strategy, claimed as "optimal" for this problem in a recent work, can perform arbitrarily bad in theory. We propose alternate strategies with theoretical guarantees. Using both public datasets as well as the production system at Facebook, we show that our techniques are effective in practice.
Tutorial : Knowledge Bases in the Age of Big Data Analytics
Location: Bauhinia 1
Chair: Tutorial 3 Chair


Knowledge Bases in the Age of Big Data Analytics
Fabian M. Suchanek, Gerhard Weikum
The proliferation of knowledge-sharing communities such as Wikipedia and the progress in scalable information extraction from Web and text sources has enabled the automatic construction of very large knowledge bases. Recent endeavors of this kind include academic research projects such as DBpedia, KnowItAll, Probase, ReadTheWeb, and YAGO, as well as industrial ones such as Freebase. These projects provide automatically constructed knowledge bases of facts about named entities, their semantic classes, and their mutual relationships. They usually contain millions of entities and hundreds of millions of facts about them. Such world knowledge in turn enables cognitive applications and knowledge-centric services like disambiguating natural-language text, deep question answering, and semantic search for entities and relations in Web and enterprise data. Prominent examples of how knowledge bases can be harnessed include the Google Knowledge Graph and the IBM Watson question answering system. This tutorial presents state-of-the-art methods, recent advances, research opportunities, and open challenges along this avenue of knowledge harvesting and its applications. Particular emphasis will be on the twofold role of knowledge bases for big-data analytics: using scalable distributed algorithms for harvesting knowledge from Web and text sources, and leveraging entity-centric knowledge for deeper interpretation of and better intelligence with big data.
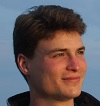
Bio: Fabian M. Suchanek is an associate professor at the Télécom ParisTech University in Paris, France. He obtained his PhD at the Max Planck Institute for Informatics in 2008, which earned him an honorable mention for the ACM SIGMOD Jim Gray Dissertation Award. Later he was a postdoc at Microsoft Research Search Labs in Silicon Valley (in the group of Rakesh Agrawal) and in the WebDam team at INRIA Saclay/France (in the group of Serge Abiteboul), and led an independent Otto Hahn Research Group, funded by the Max Planck Society. Fabian is the main architect of the YAGO ontology, one of the largest public knowledge bases.
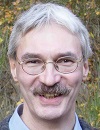
Bio: Gerhard Weikum is a scientific director at the Max Planck Institute for Informatics in Saarbruecken, Germany, where he is leading the department on databases and information systems. He co-authored a comprehensive textbook on transactional systems, received the VLDB 10-Year Award for his work on automatic DB tuning, and is one of the creators of the YAGO knowledge base. Gerhard is an ACM Fellow, a member of several scientific academies in Germany and Europe, and a recipient of a Google Focused Research Award, an ACM SIGMOD Contributions Award, and an ERC Synergy Grant.
Demo 4
Location: Pearl
Chair: Demo 4 Chair


SPIRE: Supporting Parameter-Driven Interactive Rule Mining and Exploration
Xika Lin* (Worcester Polytechnic Institut),Abhishek Mukherji (Worcester Polytechnic Institute),Elke Rundensteiner (Worcester Polytechnic Institute),Matthew Ward (Worcester Polytechnic Institute)
We demonstrate our SPIRE technology for supporting interactive mining of both positive and negative rules at the speed of thought. It is often misleading to learn only about positive rules, yet extremely revealing to find strongly supported negative rules. Key technical contributions of SPIRE including region-wise abstractions of rules, positive-negative rule relationship analysis, rule redundancy management and rule visualization supporting novel exploratory queries will be showcased. The audience can interactively explore complex rule relationships in a visual manner, such as comparing negative rules with their positive counterparts, that would otherwise take prohibitive time. Overall, our SPIRE system provides data analysts with rich insights into rules and rule relationships while significantly reducing manual effort and time investment required.


An Integrated Development Environment for Faster Feature Engineering
Michael Cafarella (University of Michigan),Michael Anderson* (University of Michigan),Yixing Jiang (University of Michigan),Guan Wang (University of Michigan),Bochun Zhang (University of Michigan)
The application of machine learning to large datasets has become a core component of many important and exciting software systems being built today. The extreme value in these trained systems is tempered, however, by the difficulty of constructing them. As shown by the experience of Google, Netflix, IBM, and many others, a critical problem in building trained systems is that of feature engineering. High-quality machine learning features are crucial for the system’s performance but are difficult and time-consuming for engineers to develop. Data-centric developer tools that improve the productivity of feature engineers will thus likely have a large impact on an important area of work. We have built a demonstration integrated development environment for feature engineers. It accelerates one particular step in the feature engineering development cycle: evaluating the effectiveness of novel feature code. In particular, it uses an index and runtime execution planner to process raw data objects (e.g., Web pages) in order of descending likelihood that the data object will be relevant to the user’s feature code. This demonstration IDE allows the user to write arbitrary feature code, evaluate its impact on learner quality, and observe exactly how much faster our technique performs compared to a baseline system.


Pronto: A Software-Defined Networking based System for Performance Management of Analytical Queries on Distributed Data Stores
Pengcheng Xiong* (NEC Labs),Hakan Hacigumus (NEC Labs)
Nowadays data analytics applications are accessing more and more data from distributed data stores, creating large amount of data traffic on the network. Therefore, distributed analytic queries are prone to suffer from bad performance in terms of query execution time when they encounter a network resource contention, which is quite common in a shared network. Typical distributed query optimizers do not have a way to solve this problem because historically they have been treating the network underneath as a black-box: they are unable to monitor it, let alone to control it. However, we are entering a new era of software-defined networking (SDN), which provides visibility into and control of the network's state for the applications including distributed database systems. In this demonstration, we present a system, called Pronto that leverages the SDN capabilities for a distributed query processor to achieve performance improvement and differentiation for analytical queries. The system is the real implementation of our recently developed methods on commercial SDN products. The demonstration shows the shortcomings of a distributed query optimizer, which treats the underlying network as a black box, and the advantages of the SDN-based approach by allowing the users to selectively explore various relevant and interesting settings in a distributed query processing environment.


Getting Your Big Data Priorities Straight: A Demonstration of Priority-based QoS using Social-network-driven Stock Recommendation
Rui Zhang* (IBM Almaden),Reshu Jain (IBM Research - Almaden),Prasenjit Sarkar (IBM Research - Almaden)
As we come to terms with various big data challenges, one vital issue remains largely untouched. That is the optimal multiplexing and prioritization of different big data applications sharing the same underlying infrastructure, for example, a public cloud platform. Given these demanding applications and the necessary practice to avoid over-provisioning, resource contention between applications is inevitable. Priority must be given to important applications (or sub workloads in an application) in these circumstances. This demo highlights the compelling impact prioritization could make, using an example application that recommends promising combinations of stocks to purchase based on relevant Twitter sentiment. The application consists of a batch job and an interactive query, ran simultaneously. Our underlying solution provides a unique capability to identify and differentiate application workloads throughout a complex big data platform. Its current implementation is based on Apache Hadoop and the IBM GPFS distributed storage system. The demo showcases the superior interactive query performance achievable by prioritizing its workloads and thereby avoiding I/O bandwidth contention. The query time is 3.6 x better compared to no prioritization. Such a performance is within 0.3% of that of an idealistic system where the query runs without contention. The demo is conducted on around 3 months of Twitter data, pertinent to the S & P 100 index, with about 4 x 10^12 potential stock combinations considered.


Vertexica: Your Relational Friend for Graph Analytics!
Alekh Jindal* (MIT),Praynaa Rawlani (MIT),Samuel Madden (MIT CSAIL)
In this paper, we present Vertexica, a graph analytics tools on top of a relational database, which is user friendly and yet highly efficient. Instead of constraining programmers to SQL, Vertexica offers a popular vertex-centric query interface, which is more natural for analysts to express many graph queries. The programmers simply provide their vertex-compute functions and Vertexica takes care of efficiently executing them in the standard SQL engine. The advantage of using Vertexica is its ability to leverage the relational features and enable much more sophisticated graph analysis. These include expressing graph algorithms which are difficult in vertex-centric but straightforward in SQL and the ability to compose end-to-end data processing pipelines, including pre- and post- processing of graphs as well as combining multiple algorithms for deeper insights. Vertexica has a graphical user interface and we outline several demonstration scenarios including, interactive graph analysis, complex graph analysis, and continuous and time series analysis.


NScale: Neighborhood-centric Analytics on Large Graphs
Abdul Quamar* (University of Maryland),Amol Deshpande (University of Maryland),Jimmy Lin (University of Maryland)
There is an increasing interest in executing rich and complex analysis tasks over large-scale graphs, many of which require processing and reasoning about a large number of multi-hop neighborhoods or subgraphs in the graph. Examples of such tasks include ego network analysis, motif counting in biological networks, finding social circles, personalized recommendations, link prediction, anomaly de- tection, analyzing influence cascades, and so on. These tasks are not well served by existing vertex-centric graph processing frameworks whose computation and execution models limit the user program to directly access the state of a single vertex, resulting in high communication, scheduling, and memory overheads in executing such tasks. Further, most existing graph processing frameworks also typically ignore the challenges in extracting the relevant portions of the graph that an analysis task is interested in, and loading it onto distributed memory. In this demonstration proposal, we describe NSCALE, a novel end-to-end graph processing framework that enables the distributed execution of complex neighborhood-centric analytics over large- scale graphs in the cloud. NSCALE enables users to write programs at the level of neighborhoods or subgraphs. NSCALE uses Apache YARN for efficient and fault-tolerant distribution of data and computation; it features GEL, a novel graph extraction and loading phase, that extracts the relevant portions of the graph and loads them into distributed memory using as few machines as possible. NSCALE utilizes novel techniques for the distributed execution of user computation that minimize memory consumption by exploit- ing overlap among the neighborhoods of interest. A comprehensive experimental evaluation shows orders-of-magnitude improvements in performance and total cost over vertex-centric approaches.


DPSynthesizer: Differentially Private Data Synthesizer for Privacy Preserving Data Sharing
Haoran Li* (Emory University),Li Xiong (Emory University),Xiaoqian Jiang (UC San Diego),Lifan Zhang (Emory University)
Differential privacy has recently emerged in private statistical data release as one of the strongest privacy guarantees. However, to this date there is no open-source tools for releasing synthetic data in place of the original data under differential privacy. We propose DPSynthesizer, a toolkit for differentially private data synthesization. The core of DPSynthesizer is DPCopula which is designed for high-dimensional data. DPCopula computes a differentially private copula function from which we can sample synthetic data. Copula functions are used to describe the dependence between multivariate random vectors and allow us to build the multivariate joint distribution using one-dimensional marginal distributions. DPSynthesizer also implements a set of state-of-the-art methods for building differentially private histograms from which synthetic data can be generated. We will demonstrate the system using DPCopula as well as other methods with various data sets, showing the feasibility, utility, efficiency of various methods.


SPOT: Locating Social Media Users Based on Social Network Context
Zhi Liu* (University of North Texas),Yan Huang (University of North Texas),Longbo Kong (University of North Texas)
A tremendous amount of information is being shared everyday on social media sites such as Facebook, Twitter or Google+. But only a small portion of users provide their location information, which can be helpful in targeted advertisement and many other services. In this demo we present our large scale user location estimation system, SPOT, which showcase different location estimating models on real world data sets. The demo shows three different location estimation algorithms: a friend-based, a social closeness-based, and an energy and local social coefficient based. The first algorithm is a baseline and the other two new algorithms utilize social closeness information which was traditionally treated as a binary friendship. The two algorithms are based on the premise that friends are different and close friends can help to estimate location better. The demo will also show that all three algorithms benefit from a confidence-based iteration method. The demo is web-based. A user can specify different settings, explore the estimation results on a map, and observe the statistical information, e.g. accuracy and average friends used in the estimation, dynamically. The demo provides two datasets: Twitter (148,860 located users) and Gowalla (99,563 located users). Furthermore, a user can filter users with certain features, e.g. with more than 100 friends, to see how the estimating models work on a particular case. The estimated and real locations of those users as well as their friends will be displayed on the map.


RASP-QS: Efficient and Confidential Query Services in the Cloud
Zohreh Alavi (Wright State University),James Powers (Wright State University),Jiayue Wang (Wright State University),Keke Chen* (Wright State University)
Hosting data query services in public clouds is an attractive solution for its great scalability and significant cost savings. However, data owners also have concerns on data privacy due to the lost control of the infrastructure. This demonstration shows a prototype for efficient and confidential range/kNN query services built on top of the random space perturbation (RASP) method. The RASP approach provides a privacy guarantee practical to the setting of cloud-based computing, while enabling much faster query processing compared to the encryption-based approach. This demonstration will allow users to more intuitively understand the technical merits of the RASP approach via interactive exploration of the visual interface.


Thoth: Towards Managing a Multi-System Cluster
Mayuresh Kunjir* (Duke University),Prajakta Kalmegh (Duke University),Shivnath Babu (Duke University)
Following the `no one size fits all' philosophy, active research in big data platforms is focusing on creating an environment for multiple `one-size' systems to co-exist and co-operate in the same cluster. Consequently, it has now become imperative to provide an \textit{integrated management} solution that provides a database-centric view of the underlying multi-system environment. We outline the proposal of DBMS+, a database management platform over multiple `one-size' systems. Our prototype implementation of DBMS+, called Thoth, adaptively chooses a \textit{best-fit} system based on application requirements. In this demonstration, we propose to showcase Thoth DM, a data management framework for Thoth which consists of a data collection pipeline utility, data consolidation and dispatcher module, and a warehouse for storing this data. We further introduce the notion of apps; an app is a utility that registers with Thoth and interfaces with its warehouse to provide core database management functionalities like dynamic provisioning of resources, designing a multi-system-aware optimizer, tuning of configuration parameters on each system, data storage, and layout schemes. We will demonstrate Thoth in action over Hive, Hadoop, Shark, Spark, and the Hadoop Distributed File System. This demonstration will focus on the following apps: (i) Dashboard for administration and control that will let the audience monitor and visualize a database-centric view of the multi-system cluster, and (ii) Data Layout Recommender apps will allow searching for the optimal data layout in the multi-system setting.
Wednesday Sep 3rd 12:15-13:45
Sep 3rd Lunch
Location: Crystal
Sep 3rd Lunch
Wednesday Sep 3rd 13:45-15:15
Papers : Graph Data I
Location: Diamond 1
Chair: Martin Theobald


Reverse Top-k Search using Random Walk with Restart
Adams Wei Yu* (The University of Hong Kong), Nikos Mamoulis (University of Hong Kong), Hao Su (Stanford University)
With the increasing popularity of social networks, large volumes of graph data are becoming available. Large graphs are also derived by structure extraction from relational, text, or scientific data (e.g., relational tuple networks, citation graphs, ontology networks, protein-protein interaction graphs). Node-to-node proximity is the key building block for many graph-based applications that search or analyze the data. Among various proximity measures, random walk with restart (RWR)is widely adopted because of its ability to consider the global structure of the whole network. Although RWR-based similarity search has been well studied before, there is no prior work on reverse top-$k$ proximity search in graphs based on RWR. We discuss the applicability of this query and show that its direct evaluation using existing methods on RWR-based similarity search has very high computational and storage demands. To address this issue, we propose an indexing technique, paired with an on-line reverse top-$k$ search algorithm. Our experiments show that our technique is efficient and has manageable storage requirements even when applied on very large graphs.


Computing Personalized PageRank Quickly by Exploiting Graph Structures
Takanori Maehara* (National Institute of Informatics), Takuya Akiba (The University of Tokyo), Yoichi Iwata (The University of Tokyo), Ken-ichi Kawarabayashi (National Institute of Informatics)
We propose a new scalable algorithm that can compute Personalized PageRank (PPR) very quickly. The Power method is a state-of-the-art algorithm for computing exact PPR; however, it requires many iterations. Thus reducing the number of iterations is the main challenge. We achieve this by exploiting graph structures of web graphs and social networks. The convergence of our algorithm is very fast. In fact, it requires up to 7.5 times fewer iterations than the Power method and is up to five times faster in actual computation time. To the best of our knowledge, this is the first time to use graph structures explicitly to solve PPR quickly. Our contributions can be summarized as follows. 1. We provide an algorithm for computing a tree decomposition, which is more efficient and scalable than any previous algorithm. 2. Using the above algorithm, we can obtain a ``core-tree decomposition'' of any web graph and social network. This allows us to decompose a web graph and a social network into (1) the ``core,'' which behaves like an expander graph, and (2) a small tree-width graph, which behaves like a ``tree'' in an algorithmic sense. 3. We apply a direct method to the small tree-width part to construct an LU decomposition. 4. Building on the LU decomposition and using it as ``preconditoner,'' we apply GMRES method (a state-of-the-art advanced iterative method) to compute PPR for whole web graphs and social networks.


Distributed SociaLite: A Datalog-Based Language for Large-Scale Graph Analysis
Jiwon Seo* (Stanford), Jongsoo Park (Intel Corporation), Jaeho Shin (Stanford Univ), Monica Lam (Stanford)
Large-scale graph analysis is becoming important with the rise of world-wide social network services.Recently in SociaLite, we proposed extensions to Datalog to efficiently and succinctly implement graph analysis programs on sequential machines.This paper describes novel extensions and optimizations of SociaLite for parallel and distributed executions to support large-scale graph analysis.With distributed SociaLite, programmers simply annotate how data are to be distributed, then the necessary communication is automatically inferred to generate parallel code for cluster of multi-core machines. It optimizes the evaluation of recursive monotone aggregate functions using a delta stepping technique. In addition, approximate computation is supported in SociaLite, allowing programmers to trade off accuracy for less time and space.We evaluated SociaLite with six core graph algorithms used in many social network analyses. Our experiment with 64 Amazon EC2 8-core instances shows that SociaLite programs performed within a factor of two with respect to ideal weak scaling. Compared to optimized Giraph, an open-source alternative of Pregel, SociaLite programs are 4 to 12 times faster across benchmark algorithms, and 22 times more succinct on average.As a declarative query language, SociaLite, with the help of a compiler that generates efficient parallel and approximate code, can be used easily to create many social apps that operate on large-scale distributed graphs.


Probabilistic Query Rewriting for Efficient and Effective Keyword Search on Graph Data
Lei Zhang* (KIT), Thanh Tran (KIT), Achim Rettinger (KIT)
The problem of rewriting keyword search queries on graph data has been studied recently, where the main goal is to clean user queries by rewriting keywords as valid tokens appearing in the data and grouping them into meaningful segments. The main solution to this problem employs heuristics for ranking query rewrites and a dynamic programming algorithm for computing them. Based on a broader set of queries defined by an existing benchmark, we show that the use of these heuristics does not yield good results. We propose a novel probabilistic framework, which enables the optimality of a query rewrite to be estimated in a more principled way. We show that our approach outperforms existing work in terms of effectiveness and efficiency of query rewriting. More importantly, we provide the first results indicating query rewriting can indeed improve overall keyword search runtime performance and result quality.


Summarizing Answer Graphs Induced by Keyword Queries
Yinghui Wu* (UCSB), Shengqi Yang (University of California (Santa Barbara), Mudhakar Srivatsa (IBM T.J.Watson Research Center), Arun Iyengar (IBM T.J.Watson Research Center), Xifeng Yan (University of Santa Barbara)
Keyword search has been popularly used to query graph data. Due to the lack of structure support, a keyword query might generate an excessive number of matches, referred to as "answer graphs", that could include different relationships among keywords. An ignored yet important task is to group and summarize answer graphs that share similar structures and contents for better query interpretation and result understanding. This paper studies the summarization problem for the answer graphs induced by a keyword query Q. (1) A notion of summary graph is proposed to characterize the summarization of answer graphs. Given Q and a set of answer graphs G, a summary graph preserves the relation of the keywords in Q by summarizing the paths connecting the keywords nodes in G. (2) A quality metric of summary graphs, called coverage ratio, is developed to measure information loss of summarization. (3) Based on the metric, a set of summarization problems are formulated, which aim to find minimized summary graphs with certain coverage ratio. (a) We show that the complexity of these summarization problems ranges from PTIME to NP-complete. (b) We provide exact and heuristic summarization algorithms. (4) Using real-life and synthetic graphs, we experimentally verify the effectiveness and the efficiency of our techniques.
Papers : Benchmarking
Location: Diamond 2
Chair: Paul Larson


OLTP-Bench: An Extensible Testbed for Benchmarking Relational Databases
Djellel Eddine Difallah* (University of fribourg), Andrew Pavlo (Carnegie Mellon University), Carlo Curino (Microsoft), Philippe Cudré-Mauroux (University of Fribourg)
Benchmarking is an essential aspect of any database management system (DBMS) effort. Despite several recent advancements, such as pre-configured cloud database images and database-as-a-service (DBaaS) offerings, the deployment of a comprehensive testing plat- form with a diverse set of datasets and workloads is still far from being trivial. In many cases, researchers and developers are limited to a small number of workloads to evaluate the performance charac- teristics of their work. This is due to the lack of a universal bench- marking infrastructure, and to the difficulty of gaining access to real data and workloads. This results in lots of unnecessary engineer- ing efforts and makes the performance evaluation results difficult to compare. To remedy these problems, we present OLTP-Bench, an extensible "batteries included" DBMS benchmarking testbed. The key contributions of OLTP-Bench are its ease of use and extensibil- ity, support for tight control of transaction mixtures, request rates, and access distributions over time, as well as the ability to support all major DBMSs and DBaaS platforms. Moreover, it is bundled with fifteen workloads that all differ in complexity and system de- mands, including four synthetic workloads, eight workloads from popular benchmarks, and three workloads that are derived from real-world applications. We demonstrate through a comprehen- sive set of experiments conducted on popular DBMS and DBaaS offerings the different features provided by OLTP-Bench and the effectiveness of our testbed in characterizing the performance of database services.


QuEval: Beyond high-dimensional indexing à la carte
Martin Schäler* (University of Magdeburg), Alexander Grebhahn (University of Passau), Reimar Schröter (University of Magdeburg), Sandro Schulze (TU Braunschweig), Veit Köppen (University of Magdeburg), Gunter Saake (University of Magdeburg)
In the recent past, the amount of high-dimensional data, such as feature vectors extracted from multimedia data, increased dramatically. A large variety of indexes have been proposed to store and access such data efficiently. However, due to specific requirements of a certain use case, choosing an adequate index structure is a complex and time-consuming task. This may be due to engineering challenges or open research questions.To overcome this limitation, we present QuEval, an open-source framework that can be flexibly extended w.r.t. index structures, distance metrics, and data sets. QuEval provides a unified environment for a sound evaluation of different indexes, for instance, to support tuning of indexes. In an empirical evaluation, we show how to apply our framework, motivate benefits, and demonstrate analysis possibilities.


An Experimental Analysis of Iterated Spatial Joins in Main Memory
Benjamin Sowell* (Amiato), Marcos Vaz Salles (DIKU), Tuan Cao (Google), Alan Demers (Cornell University), Johannes Gehrke (Cornell University)
Many modern applications rely on high-performance processing of spatial data. Examples include location-based services, games, virtual worlds, and scientific simulations such as molecular dynamics and behavioral simulations. These applications deal with large numbers of moving objects that continuously sense their environment, and their data access can often be abstracted as a repeated spatial join. Updates to object positions are interspersed with these join operations, and batched for performance. Even for the most demanding scenarios, the data involved in these joins fits comfortably in the main memory of a cluster of machines, and most applications run completely in main memory for performance reasons.Choosing appropriate spatial join algorithms is challenging due to the large number of techniques in the literature. In this paper, we perform an extensive evaluation of repeated spatial join algorithms for distance (range) queries in main memory. Our study is unique in breadth when compared to previous work: We implement, tune, and compare ten distinct algorithms on several workloads drawn from the simulation and spatial indexing literature. We explore the design space of both index nested loops algorithms and specialized join algorithms, as well as the use of moving object indices that can be incrementally maintained. Surprisingly, we find that when queries and updates can be batched, repeatedly re-computing the join result from scratch outperforms using a moving object index in all but the most extreme cases. This suggests that --- given the code complexity of index structures for moving objects --- specialized join strategies over simple index structures, such as Synchronous Traversal over R-Trees, should be the methods of choice for the above applications.


SQL-on-Hadoop: Full Circle Back to Shared-Nothing Database Architectures
Avrilia Floratou* (IBM Almaden Research Center), Umar Farooq Minhas (IBM Almaden Research Center), Fatma Ozcan (IBM Almaden)
SQL query processing for analytics over Hadoop data has recently gained significant traction. Among many systems providing some SQL support over Hadoop, Hive is the first native Hadoop system that uses an underlying framework such as MapReduce or Tez to process SQL-like statements. Impala, on the other hand, represents the new emerging class of SQL-on-Hadoop systems that exploit a shared-nothing parallel database architecture over Hadoop. Both systems optimize their data ingestion via columnar storage, and promote different file formats: ORC and Parquet. In this paper, we compare the performance of these two systems by conducting a set of cluster experiments using a TPC-H like benchmark and two TPC-DS inspired workloads. We also closely study the I/O efficiency of their columnar formats using a set of micro-benchmarks. Our results show that Impala is 3.3X to 4.4X faster than Hive on MapReduce and 2.1X to 2.8X than Hive on Tez for the overall TPC-H experiments. Impala is also 8.2X to 10X faster than Hive on MapReduce and about 4.3X faster than Hive on Tez for the TPC-DS inspired experiments. Through detailed analysis of experimental results, we identify the reasons for this performance gap and examine the strengths and limitations of each system.


Benchmarking Scalability and Elasticity of Distributed Database Systems [Experiments and Analysis Paper]
Jörn Kuhlenkamp (KIT), Markus Klems* (KIT), Oliver Röss (KIT)
Distributed database system performance benchmarks are an important source of information for decision makers who must select the right technology for their data management problems. Since important decisions rely on trustworthy experimental data, it is necessary to reproduce experiments and verify the results. We reproduce performance and scalability benchmarking experiments of HBase and Cassandra that have been conducted by previous research and compare the results. The scope of our reproduced experiments is extended with a performance evaluation of Cassandra on different Amazon EC2 infrastructure configurations, and an evaluation of Cassandra and HBase elasticity by measuring scaling speed and performance impact while scaling.
Industrial : Analytics
Location: Diamond 3
Chair: Neoklis Polyzotis


Real-Time Twitter Recommendation: Online Motif Detection in Large Dynamic Graphs
Pankaj Gupta (Twitter),Venu Satuluri (Twitter),Ajeet Grewal (Twitter),Siva Gurumurthy (Twitter),Volodymyr Zhabiuk (Twitter),Quannan Li (Twitter),Jimmy Lin* (Twitter)*
We describe a production Twitter system for generating relevant, personalized, and timely recommendations based on observing the temporally-correlated actions of each user's followings. The system currently serves millions of recommendations daily to tens of millions of mobile users. The approach can be viewed as a specific instance of the novel problem of online motif detection in large dynamic graphs. Our current solution partitions the graph across a number of machines, and with the construction of appropriate data structures, motif detection can be translated into the lookup and intersection of adjacency lists in each partition. We conclude by discussing a generalization of the problem that perhaps represents a new class of data management systems.


Error-bounded Sampling for Analytics on Big Sparse Data
Ying Yan* (Microsoft Research)*,Liang Chen (Microsoft Research),Zheng Zhang (MSRA)
Aggregation queries are at the core of business intelligence and data analytics. In the big data era, many scalable shared-nothing systems have been developed to process aggregation queries over massive amount of data. Microsoft's SCOPE is a well-known instance in this category. Nevertheless, aggregation queries are still expensive, because query processing needs to consume the entire data set, which is often hundreds of terabytes. Data sampling is a technique that samples a small portion of data to process and returns an approximate result with an error bound, thereby reducing the query's execution time. While similar problems were studied in the database literature, we encountered new challenges that disable most of prior efforts: (1) error bounds are dictated by end users and cannot be compromised, (2) data is sparse, meaning data has a limited population but a wide range. For such cases, conventional uniform sampling often yield high sampling rates and thus deliver limited or no performance gains. In this paper, we propose error-bounded stratified sampling to reduce sample size. The technique relies on the insight that we may only reduce the sampling rate with the knowledge of data distributions. The technique has been implemented into Microsoft internal search query platform. Results show that the proposed approach can reduce up to 99% sample size comparing with uniform sampling, and its performance is robust against data volume and other key performance metrics.


Interval Disaggregate: A New Operator for Business Planning
Sang Cha (SAP Labs Korea)*,Chang Song (SAP Labs Korea),Ki Kim (SAP Labs Korea),Cheol Ryu (SAP Labs Korea),Sunho Lee (SAP Labs Korea)
Business planning as well as analytics on top of large-scale database systems is valuable to decision makers, but planning operations known and implemented so far are very basic. In this paper we propose a new planning operation called interval disaggregate, which goes as follows. Suppose that the planner, typically the management of a company, plans sales revenues of its products in the current year. An interval of the expected revenue for each product in the current year is computed from historical data in the database as the prediction interval of linear regression on the data. A total target revenue for the current year is given by the planner. The goal of the interval disaggregate operation is to find an appropriate disaggregation of the target revenue, considering the intervals. We formulate the problem of interval disaggregation more precisely and give solutions for the problem. Multidimensional geometry plays a crucial role in the problem formulation and the solutions. We implemented interval disaggregation into the planning engine of SAP HANA and did experiments on real-world data. Our experiments show that interval disaggregation gives more appropriate solutions with respect to historical data than the known basic disaggregation called referential disaggregation. We also show that interval disaggregation can be combined with the deseasonalization technique when the dataset shows seasonal fluctuations.


Chimera: Large-Scale Classification using Machine Learning, Rules, and Crowdsourcing
AnHai Doan* (Univ. of Wisconsin Madison)*,Chong Sun (WalmartLabs),Narasimhan Rampalli (WalmartLabs)
solution to classify tens of millions of products into 5000+ product types at WalmartLabs. We show that at this scale, many conventional assumptions regarding learning and crowdsourcing break down, and that existing solutions cease to work. We describe how Chimera employs a combination of learning, rules (created by in-house analysts), and crowdsourcing to achieve accurate, continuously improving, and cost-effective classification. We discuss a set of lessons learned for other similar Big Data systems. In particular, we argue that at large scales crowdsourcing is critical, but must be used in combination with learning, rules, and in-house analysts. We also argue that using rules (in conjunction with learning) is a must, and that more research attention should be paid to helping analysts create and manage (tens of thousands of) rules more effectively.
Papers : Parallel and Distributed Systems
Location: Diamond 4
Chair: Gustavo Alonso


Mesa: Geo-Replicated, Near Real-Time, Scalable Data Warehousing
Ashish Gupta* (Google Inc.), Fan Yang (Google Inc.), Jason Govig (Google Inc.), Adam Kirsch (Google Inc.), Kelvin Chan (Google Inc.), Kevin Lai (Google Inc.), Shuo Wu (Google Inc.), Sandeep Dhoot (Google Inc.), Abhilash Kumar (Google Inc.), Ankur Agiwal (Google Inc.), Sanjay Bhansali (Google Inc.), Mingsheng Hong (Google Inc.), Jamie Cameron (Google Inc.), Masood Siddiqi (Google Inc.), David Jones (Google Inc), Jeff Shute (Google Inc.), Andrey Gubarev (Google), Shivakumar Venkataraman (Google Inc.), Divyakant Agrawal (Google Inc.)
Mesa is a highly scalable analytic data warehousing system that stores critical measurement data related to Google's Internet advertising business. Mesa is designed to satisfy a complex and challenging set of user and systems requirements, including near real-time data ingestion and queryability, as well as high availability, reliability, fault tolerance, and scalability for large data and query volumes. Specifically, Mesa handles petabytes of data, processes millions of row updates per second, and serves billions of queries that fetch trillions of rows per day. Mesa is geo-replicated across multiple datacenters and provides consistent and repeatable query answers at low latency, even when an entire datacenter fails. This paper presents the Mesa system and reports the performance and scale that it achieves.


PREDIcT: Towards Predicting the Runtime of Large Scale Iterative Analytics
Adrian Daniel Popescu* (EPFL), Andrey Balmin (GraphSQL), Vuk Ercegovac (Google), Anastasia Ailamaki (EPFL)
Machine learning algorithms are widely used today for analytical tasks such as data cleaning, data categorization, or data filtering. At the same time, the rise of social media motivates recent uptake in large scale graph processing. Both categories of algorithms are dominated by iterative subtasks, i.e., processing steps which are executed repetitively until a convergence condition is met. Optimizing cluster resource allocations among multiple workloadsof iterative algorithms motivates the need for estimating their runtime, which in turn requires: i) predicting the number of iterations, and ii) predicting the processing time of each iteration. As both parameters depend on the characteristics of the dataset and on the convergence function, estimating their values before execution is difficult. This paper proposes PREDIcT, an experimental methodology for predicting the runtime of iterative algorithms. PREDIcT uses sample runsfor capturing the algorithm's convergence trend and per-iteration key input features that are well correlated with the actual processing requirements of the complete input dataset. Using this combination of characteristics we predict the runtime of iterative algorithms, including algorithms with very different runtime patterns among subsequent iterations. Our experimental evaluation of multiple algorithms on scale-free graphs shows a relative prediction error of 10%-30% for predicting runtime, including algorithms with up to 100x runtime variability among consecutive iterations.


Scalable and Adaptive Online Joins
Mohammed ElSeidy* (EPFL), abdallah Elguindy (EPFL), Aleksandar Vitorovic (EPFL), Christoph Koch (EPFL)
Scalable join processing in a parallel shared-nothing environment requires a partitioning policy that evenly distributes the processing load while minimizing the size of state maintained and number of messages communicated. Previous research proposes static partitioning schemes that require statistics beforehand. In an online or streaming environment in which no statistics about the workload are known, traditional static approaches perform poorly. This paper presents a novel parallel online dataflow join operator that supports arbitrary join predicates. The proposed operator continuously adjusts itself to the data dynamics through adaptive dataflow routing and state repartitioning. The operator is resilient to data skew, maintains high throughput rates, avoids blocking behavior during state repartitioning, takes an eventual consistency approach for maintaining its local state, and behaves strongly consistently as a black-box dataflow operator. We prove that the operator ensures a constant competitive ratio 3.75 in data distribution optimality and that the cost of processing an input tuple is amortized constant, taking into account adaptivity costs. Our evaluation demonstrates that our operator outperforms the state-of-the-art static partitioning schemes in resource utilization, throughput, and execution time.


Edelweiss: Automatic Storage Reclamation for Distributed Programming
Neil Conway* (UC Berkeley), Peter Alvaro (UC Berkeley), Emily Andrews (UC Berkeley), Joseph Hellerstein (UC Berkeley)
Event Log Exchange (ELE) is a common programming pattern based on immutable state and messaging. ELE sidesteps traditional challenges in distributed consistency, at the expense of introducing new challenges in designing space reclamation protocols to avoid consuming unbounded storage. We introduce Edelweiss, a sublanguage of Bloom that provides an ELE programming model, yet automatically reclaims space without programmer assistance. We describe techniques to analyze Edelweiss programs and automatically generate application-specific distributed space reclamation logic. We show how Edelweiss can be used to elegantly implement a variety of communication and distributed storage protocols; the storage reclamation code generated by Edelweiss effectively garbage-collects state and often matches hand-written protocols from the literature.


Understanding Insights into the Basic Structure and Essential Issues of Table Placement Methods in Clusters
Yin Huai* (The Ohio State University), Siyuan Ma (Department of Computer Science and Engineering (The Ohio State University), Rubao Lee (The Ohio State University), Owen O'Malley (Hortonworks), Xiaodong Zhang (Department of Computer Science and Engineering (The Ohio State University)
A table placement method is a critical component in big data analytics on distributed systems. It determines the way how data values in a two-dimensional table are organized and stored in the underlying cluster. Based on Hadoop computing environments, several table placement methods have been proposed and implemented. However, a comprehensive and systematic study to understand, to compare, and to evaluate different table placement methods has not been done. Thus, it is highly desirable to gain important insights into the basic structure and essential issues of table placement methods in the context of big data processing infrastructures.In this paper, we present such a study. The basic structure of a data placement method consists of three core operations: row reordering, table partitioning, and data packing. All the existing placement methods are formed by these core operations with variations made by the three key factors: (1) the size of a horizontal logical subset of a table (or the size of a row group), (2) the function of mapping columns to column groups, and (3) the function of packing columns or column groups in a row group into physical blocks. We have designed and implemented a benchmarking tool to provide insights into how variations of each factor affect the I/O performance of reading data of a table stored by a table placement method. Based on our results, we give suggested actions to optimize table reading performance. Results from large-scale experiments have also confirmed that our findings are valid for production workloads. Finally, we present ORC File as a case study to show the effectiveness of our findings and suggested actions.
Papers : Web and Knowledge II
Location: Diamond 5
Chair: Luna Dong


Workload Matters: Why RDF Databases Need a New Design
Gunes Aluc* (University of Waterloo), Tamer Ozsu (University of Waterloo), Khuzaima Daudjee (University of Waterloo)
The Resource Description Framework (RDF) is a standard for conceptually describing data on the Web, and SPARQL is the query language for RDF. As RDF is becoming widely utilized, RDF data management systems are being exposed to more diverse and dynamic workloads. Existing systems are workload-oblivious, and are therefore unable to provide consistently good performance. We propose a vision for a workload-aware and adaptive system. To realize this vision, we re-evaluate relevant existing physical design criteria for RDF and address the resulting set of new challenges.


Scaling Queries over Big RDF Graphs with Semantic Hash Partitioning
Kisung Lee* (Georgia Tech), Ling Liu (Georgia Institute of Technology)
Massive volumes of big RDF data are growing beyond the performance capacity of conventional RDF data management systems operating on a single node. Applications using large RDF data demand efficient data partitioning solutions for supporting RDF data access on a cluster of compute nodes. In this paper we present a novel semantic hash partitioning approach and implement a Semantic HAsh Partitioning-Enabled distributed RDF data management system, called SHAPE. This paper makes three original contributions. First, the semantic hash partitioning approach we propose extends the simple hash partitioning method through direction-based triple groups and direction-based triple replications. The latter enhances the former by controlled data replication through intelligent utilization of data access locality, such that queries over big RDF graphs can be processed with zero or very small amount of inter-machine communication cost. Second, we generate locality-optimized query execution plans that are more efficient than popular multi-node RDF data management systems by effectively minimizing the inter-machine communication cost for query processing. Third but not the least, we provide a suite of locality-aware optimization techniques to further reduce the partition size and cut down on the inter-machine communication cost during distributed query processing. Experimental results show that our system scales well and can process big RDF datasets more efficiently than existing approaches.


Matching Titles with Cross Title Web-Search Enrichment and Community Detection
Vishrawas Gopalakrishnan* (SUNY Buffalo), Nikhil Londhe (SUNY Buffalo), Aidong Zhang (SUNY Buffalo), HUng Ngo (SUNY Buffalo), Rohini Srihari (SUNY Buffalo)
Title matching refers roughly to the following problem. We are given two strings of text obtained from different data sources. The texts refer to some underlying physical entities and the problem is to report whether the two strings refer to the same physical entity or not. There are manifestations of this problem in a variety of domains, such as product or bibliography matching, and location or person disambiguation. We propose a new approach to solving this problem, consisting of two main components. The first component uses Web searches to "enrich'' the given pair of titles: making titles that refer to the same physical entity more similar, and those which do not, much less similar. A notion of similarity is then measured using the second component, where the tokens from the two titles are modelled as vertices of a "social'' network graph. A "strength of ties'' style of clustering algorithm is then applied on this to see whether they form one cohesive "community'' (matching titles), or separately clustered communities (mismatching titles). Experimental results confirm the effectiveness of our approach over existing title matching methods across several input domains.


Aggregate Estimation Over Dynamic Hidden Web Databases
Weimo Liu* (The George Washington University), Saravanan Thirumuruganathan ( University of Texas at Arlington), Nan Zhang (George Washington University), Gautam Das (UT Arlington)
Many web databases are "hidden" behind (i.e., only accessible through) a restrictive, form-like, search interface. Recent studies have shown that it is possible to estimate aggregate query answers over such hidden web databases by issuing a small number of carefully designed search queries through the restrictive web interface. A problem with these existing work, however, is that they all assume the underlying database to be static, while most real-world web databases (e.g., Amazon, eBay) are frequently updated. In this paper, we study the novel problem of estimating aggregates over dynamic hidden web databases while adhering to the stringent query-cost limitation they enforce (e.g., at most 1,000 search queries per day). Theoretical analysis and extensive real-world experiments demonstrate the effectiveness of our proposed algorithms and their superiority over baseline solutions (e.g., the repeated execution of algorithms designed for static web databases).


A Principled Approach to Bridging the Gap between Graph Data and their Schemas
Marcelo Arenas (PUC Chile), Gonzalo Diaz* (PUC Chile), Anastasios Kementsietsidis (IBM Research), Achille Fokoue (IBM T.J. Watson Research Center), Kavitha Srinivas (IBM T.J. Watson Research Center)
Although RDF graph data often come with an associated schema, recent studies have proven that real RDF data rarely conform to their perceived schemas. Since a number of data management decisions, including storage layouts, indexing, and efficient query processing, use schemas to guide the decision making, it is imperative to have an accurate description of the structuredness of the data at hand (how well the data conform to the schema). In this paper, we have approached the study of the structuredness of an RDF graph in a principled way: we propose a framework for specifying structuredness functions, which gauge the degree to which an RDF graph conforms to a schema. In particular, we first define a formal language for specifying structuredness functions with expressions we call rules. This language allows a user to state a rule to which an RDF graph may fully or partially conform. Then we consider the issue of discovering a refinement of a sort (type) by partitioning the dataset into subsets whose structuredness is over a specified threshold. In particular, we prove that the natural decision problem associated to this refinement problem is NP-complete, and we provide a natural translation of this problem into Integer Linear Programming (ILP). Finally, we test this ILP solution with three real world datasets and three different and intuitive rules, which gauge the structuredness in different ways. We show that the rules give meaningful refinements of the datasets, showing that our language can be a powerful tool for understanding the structure of RDF data, and we show that the ILP solution is practical for a large fraction of existing data.
Tutorial : Knowledge Bases in the Age of Big Data Analytics
Location: Bauhinia 1
Chair: Tutorial 3 Chair


Knowledge Bases in the Age of Big Data Analytics
Fabian M. Suchanek, Gerhard Weikum
The proliferation of knowledge-sharing communities such as Wikipedia and the progress in scalable information extraction from Web and text sources has enabled the automatic construction of very large knowledge bases. Recent endeavors of this kind include academic research projects such as DBpedia, KnowItAll, Probase, ReadTheWeb, and YAGO, as well as industrial ones such as Freebase. These projects provide automatically constructed knowledge bases of facts about named entities, their semantic classes, and their mutual relationships. They usually contain millions of entities and hundreds of millions of facts about them. Such world knowledge in turn enables cognitive applications and knowledge-centric services like disambiguating natural-language text, deep question answering, and semantic search for entities and relations in Web and enterprise data. Prominent examples of how knowledge bases can be harnessed include the Google Knowledge Graph and the IBM Watson question answering system. This tutorial presents state-of-the-art methods, recent advances, research opportunities, and open challenges along this avenue of knowledge harvesting and its applications. Particular emphasis will be on the twofold role of knowledge bases for big-data analytics: using scalable distributed algorithms for harvesting knowledge from Web and text sources, and leveraging entity-centric knowledge for deeper interpretation of and better intelligence with big data.
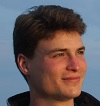
Bio: Fabian M. Suchanek is an associate professor at the Télécom ParisTech University in Paris, France. He obtained his PhD at the Max Planck Institute for Informatics in 2008, which earned him an honorable mention for the ACM SIGMOD Jim Gray Dissertation Award. Later he was a postdoc at Microsoft Research Search Labs in Silicon Valley (in the group of Rakesh Agrawal) and in the WebDam team at INRIA Saclay/France (in the group of Serge Abiteboul), and led an independent Otto Hahn Research Group, funded by the Max Planck Society. Fabian is the main architect of the YAGO ontology, one of the largest public knowledge bases.
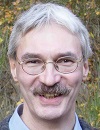
Bio: Gerhard Weikum is a scientific director at the Max Planck Institute for Informatics in Saarbruecken, Germany, where he is leading the department on databases and information systems. He co-authored a comprehensive textbook on transactional systems, received the VLDB 10-Year Award for his work on automatic DB tuning, and is one of the creators of the YAGO knowledge base. Gerhard is an ACM Fellow, a member of several scientific academies in Germany and Europe, and a recipient of a Google Focused Research Award, an ACM SIGMOD Contributions Award, and an ERC Synergy Grant.
Demo 2
Location: Pearl
Chair: Demo 2 Chair


Faster Visual Analytics through Pixel-Perfect Aggregation
Uwe Jugel* (SAP),Zbigniew Jerzak (SAP),Gregor Hackenbroich (SAP),Volker Markl (TU Berlin)
State-of-the-art visual data analysis tools ignore bandwidth limitations. They fetch millions of records of high-volume time series data from an underlying RDBMS to eventually draw only a few thousand pixels on the screen. In this work, we demonstrate a pixel-aware big data visualization system that dynamically adapts the number of data points transmitted and thus the data rate, while preserving pixel-perfect visualizations. We show how to carefully select the data points to fetch for each pixel of a visualization, using a visualization-driven data aggregation that models the visualization process. Defining all required data reduction operators at the query level, our system trades off a few milliseconds of query execution time for dozens of seconds of data transfer time. The results are significantly reduced response times and a near real-time visualization of millions of data points. Using our pixel-aware system, the audience will be able to enjoy the speed and ease of big data visualizations and learn about the scientific background of our system through an interactive evaluation component, allowing the visitor to measure, visualize, and compare competing visualization-related data reduction techniques.


That's All Folks! Llunatic Goes Open Source
Floris Geerts (University of Antwerp),Giansalvatore Mecca* (Università della Basilicata),Paolo Papotti (QCRI),Donatello Santoro (Università della Basilicata)
It is widely recognized that whenever different data sources need to be integrated into a single target database errors and inconsistencies may arise, so that there is a strong need to apply data-cleaning techniques to repair the data. Despite this need, database research has so far investigated mappings and data repairing essentially in isolation. Unfortunately, schema-mappings and data quality rules interact with each other, so that applying existing algorithms in a pipelined way -- i.e., first exchange then data, then repair the result -- does not lead to solutions even in simple settings. We present the Llunatic mapping and cleaning system, the first comprehensive proposal to handle schema mappings and data repairing in a uniform way. Llunatic is based on the intuition that transforming and cleaning data are different facets of the same problem, unified by their declarative nature. This holistic approach allows us to incorporate unique features into the system, such as configurable user interaction and a tunable trade-off between efficiency and quality of the solutions.


HDBTracker: Aggregate Tracking and Monitoring Over Dynamic Web Databases
Weimo Liu* (The George Washington University),Saad Bin Suhaim (The George Washington University),Saravanan Thirumuruganathan (University of Texas At Arlington),Nan Zhang (George Washington University),Gautam Das (UT Arlington),Ali Jaoua (Qatar University)
Numerous web databases, e.g., amazon.com, eBay.com, are "hidden" behind (i.e., accessible only through) their restrictive search and browsing interfaces. This demonstration showcases HDBTracker, a web-based system that reveals and tracks (the changes of) user-specified aggregate queries over such hidden web databases, especially those that are frequently updated, by issuing a small number of search queries through the public web interfaces of these databases. The ability to track and monitor aggregates has applications over a wide variety of domains - e.g., government agencies can track COUNT of openings at online job hunting websites to understand key economic indicators, while businesses can track the AVG price of a product over a basket of e-commerce websites to understand the competitive landscape and/or material costs. A key technique used in HDBTracker is RS-ESTIMATOR, the first algorithm that can efficiently monitor changes to aggregate query answers over a hidden web database.


BSMA: A Benchmark for Analytical Queries over Social Media Data
Fan Xia* (East China Normal University),Ye Li (East China Normal University),Chengcheng Yu (East China Normal University),Haixin Ma (East China Normal University),Haoji Hu (East China Normal University),Weining Qian (East China Normal University)
The demonstration of a benchmark, named as BSMA, for Benchmarking Social Media Analytics, is introduced in this paper. BSMA is designed to benchmark data management systems supporting analytical queries over social media. It is different to existing benchmarks in that: 1) Both real-life data and a synthetic data generator are provided. The real-life dataset contains a social network of 1.6 million users, and all their tweeting and retweeting activities. The data generator can generate both social networks and synthetic timelines that follow data distributions determined by predefined parameters. 2) A set of workloads are provided. The data generator is in responsible for producing updates. A query generator produces queries based on predefined query templates by generating query arguments online. BSMA workloads cover a large amount of queries with graph operations, temporal queries, hotspot queries, and aggregate queries. Furthermore, the argument generator is capable of sampling data items in the timeline following power-law distribution online. 3) A toolkit is provided to measure and report the performance of systems that implement the benchmark. Furthermore, a prototype system based on dataset and workload of BSMA is also implemented. The demonstration will include two parts, i.e. the internals of data and query generator, as well as the performance testing of reference implementations.


Graph-based Data Integration and Business Intelligence with BIIIG
Andre Petermann* (University of Leipzig),Martin Junghanns (University of Leipzig),Robert Mueller (HTWK Leipzig),Erhard Rahm (university of leipzig)
We demonstrate BIIIG (Business Intelligence with Integrated Instance Graphs), a new system for graph-based data integration and analysis. It aims at improving business analytics compared to traditional OLAP approaches by comprehensively tracking relationships between entities and making them available for analysis. BIIIG supports a largely automatic data integration pipeline for metadata and instance data. Metadata from heterogeneous sources are integrated in a so-called Unified Metadata Graph (UMG) while instance data is combined in a single integrated instance graph (IIG). A unique feature of BIIIG is the concept of business transaction graphs, which are derived from the IIG and which reflect all steps involved in a specific business process. Queries and analysis tasks can refer to the entire instance graph or sets of business transaction graphs. In the demonstration, we perform all data integration steps and present analytic queries including pattern matching and graph-based aggregation of business measures.


SeeDB: Automatically Generating Query Visualizations
Manasi Vartak* (MIT),Samuel Madden (MIT CSAIL),Aditya Parameswaran (Stanford University),Neoklis Polyzotis (University of California - Santa Cruz)
Data analysts operating on large volumes of data often rely on visualizations to interpret the results of queries. However, finding the right visualization for a query is a laborious and time-consuming task. We demonstrate SeeDB, a system that partially automates this task: given a query, SeeDB explores the space of all possible visualizations, and automatically identifies and recommends to the analyst those visualizations it finds to be most "interesting" or "useful". In our demonstration, conference attendees will see SeeDB in action for a variety of queries on multiple real-world datasets.


QUEST: An Exploratory Approach to Robust Query Processing
Anshuman Dutt (Indian Institute of Science),Sumit Neelam (Indian Institute of Science),Jayant Haritsa* (Indian Institute of Science Bangalore)
Selectivity estimates for optimizing declarative SQL queries often differ significantly from those actually encountered during query execution, leading to poor plan choices and inflated response times. We recently proposed a conceptually new approach to address this problem wherein the compile-time estimation process is completely eschewed for error-prone selectivities. Instead, these statistics are systematically discovered at run-time through a precisely calibrated sequence of cost-limited executions from a carefully chosen small set of plans, called the plan bouquet. This construction lends itself to guaranteed worst-case performance bounds, and repeatable execution strategies across multiple invocations of a query. A prototype implementation of the plan bouquet technique, called QUEST, has been incorporated on the PostgreSQL engine. In this demo, we showcase the various features of QUEST which result in novel performance guarantees that open up new possibilities for robust query processing.


Redoop Infrastructure for Recurring Big Data Queries
Chuan Lei* (WPI),Zhongfang Zhuang (WPI),Elke Rundensteiner (WPI),Mohamed Eltabakh (Worcester Polytechnic Institute)
This demonstration presents the Redoop system, the first full-fledged MapReduce framework with native support for recurring big data queries. Recurring queries, repeatedly being executed for long periods of time over evolving high-volume data, have become a bedrock component in most large-scale data analytic applications. Redoop is a comprehensive extension to Hadoop that pushes the support and optimization of recurring queries into Hadoop's core functionality. While backward compatible with regular MapReduce jobs, Redoop achieves an order of magnitude better performance than Hadoop for recurring workloads. Redoop employs innovative window-aware optimization techniques for recurring query execution including adaptive window-aware data partitioning, window-aware task scheduling, and inter-window caching mechanisms. We will demonstrate Redoop's capabilities on a compute cluster against real life workloads including click-stream and sensor data analysis.


PackageBuilder: From Tuples to Packages
Matteo Brucato* (UMass Amherst),Rahul Ramakrishna (UMass Amherst),Azza Abouzied (New York University Abu Dhabi UAE),Alexandra Meliou (Umass Amherst)
In this demo, we present PackageBuilder, a system that extends database systems to support package queries. A package is a collection of tuples that individually satisfy base constraints and collectively satisfy global constraints. The need for package support arises in a variety of scenarios: For example, in the creation of meal plans, users are not only interested in the nutritional content of individual meals (base constraints), but also care to specify daily consumption limits and control the balance of the entire plan (global constraints). We introduce PaQL, a declarative SQL-based package query language, and the interface abstractions which allow users to interactively specify package queries and easily navigate through their results. To efficiently evaluate queries, the system employs pruning and heuristics, as well as state-of-the-art constraint optimization solvers. We demonstrate PackageBuilder by allowing attendees to interact with the system's interface, to define PaQL queries and to observe how query evaluation is performed.


Ontology Assisted Crowd Mining
Yael Amsterdamer* (Tel Aviv University),Susan Davidson (University of Pennsylvania),Tova Milo (Tel Aviv University),Slava Novgorodov (Tel Aviv University),Amit Somech (Tel Aviv University)
We present OASSIS (for Ontology ASSISted crowd mining), a prototype system which allows users to declaratively specify their information needs, and mines the crowd for answers. The answers that the system computes are concise and relevant, and represent frequent, significant data patterns. The system is based on (1) a generic model that captures both ontological knowledge, as well as the individual knowledge of crowd members from which frequent patterns are mined; (2) a query language in which users can specify their information needs and types of data patterns they seek; and (3) an efficient query evaluation algorithm, for mining semantically concise answers while minimizing the number of questions posed to the crowd. We will demonstrate OASSIS using a couple of real-life scenarios, showing how users can formulate and execute queries through the OASSIS UI and how the relevant data is mined from the crowd.


SOPS: A System for Efficient Processing of Spatial-Keyword Publish/Subscribe
Lisi Chen* (NTU),Yan Cui (NTU),Gao Cong (Nanyang Technological University),Xin Cao (NTU)
Massive amount of data that are geo-tagged and associated with text information are being generated at an unprecedented scale. These geo-textual data cover a wide range of topics. Users are interested in receiving up-to-date geo-textual objects (e.g., geo-tagged Tweets) such that their locations meet users' need and their texts are interesting to users. For example, a user may want to be updated with tweets near her home on the topic "dengue fever headache." In this demonstration, we present SOPS, the Spatial-Keyword Publish/Subscribe System, that is capable of efficiently processing spatial keyword continuous queries. SOPS supports two types of queries: (1) Boolean Range Continuous (BRC) query that can be used to subscribe the geo-textual objects satisfying a boolean keyword expression and falling in a specified spatial region; (2) Temporal Spatial-Keyword Top-k Continuous (TaSK) query that continuously maintains up-to-date top-k most relevant results over a stream of geo-textual objects. SOPS enables users to formulate their queries and view the real-time results over a stream of geo-textual objects by browser-based user interfaces. On the server side, we propose solutions to efficiently processing a large number of BRC queries (tens of millions) and TaSK queries over a stream of geo-textual objects.


MLJ: Language-Independent Real-Time Search of Tweets Reported by Media Outlets and Journalists
Masumi Shirakawa* (Osaka University),Takahiro Hara (Osaka University),Shojiro Nishio (Osaka University)
In this demonstration, we introduce MLJ (MultiLingual Journalism, http://mljournalism.com), a first Web-based system that enables users to search any topic of latest tweets posted by media outlets and journalists beyond languages. Handling multilingual tweets in real time involves many technical challenges: language barrier, sparsity of words, and real-time data stream. To overcome the language barrier and the sparsity of words, MLJ harnesses CL-ESA, a Wikipedia-based language-independent method to generate a vector of Wikipedia pages (entities) from an input text. To continuously deal with tweet stream, we propose one-pass DP-means, an online clustering method based on DP-means. Given a new tweet as an input, MLJ generates a vector using CL-ESA and classifies it into one of clusters using one-pass DP-means. By interpreting a search query as a vector, users can instantly search clusters containing latest related tweets from the query without being aware of language differences. MLJ as of March 2014 supports nine languages including English, Japanese, Korean, Spanish, Portuguese, German, French, Italian, and Arabic covering 24 countries.
Wednesday Sep 3rd 15:15-18:15
Plenary Poster Session in the East Gallery
Location: Crystal
Chair: Plenary Poster Session in the East Gallery
Plenary Poster Session in the East Gallery
Wednesday Sep 3rd 18:30-22:00
Banquet
Location: Crystal
Chair: Banquet
Banquet
Thursday Sep 4th 08:30-10:00
Award Ceremony: Award distribution for 10 year best paper,early career,and conference best papers
Location: Crystal
Chair: Surajit Chaudhuri
10 year Best Paper Award Talk

Probabilistic Databases: the Long View
Nilesh Dalvi, Dan Suciu
Ten years ago, we studied how to evaluate queries on probabilistic databases. We found something we did not expect: some queries were easy, some were provably hard, and, in restricted cases, one could draw precisely the boundary between them. We called the former queries "safe", the latter "unsafe", and presented these findings in the paper "Efficient Query Evaluation on Probabilistic Databases". Subsequent work by several researchers, including ourselves, have significantly expanded the boundary of safe/unsafe queries to richer languages. Today, ten years later, probabilistic inference over big data sets is becoming a central data management problem, due to the success of large knowledge bases extracted automatically from the Web. One of the most promising approaches to scalable inference is based on the safe plans that we introduced ten years ago. In this talk, we will present our view on how the field has evolved, and what the future holds for Probabilistic Databases.
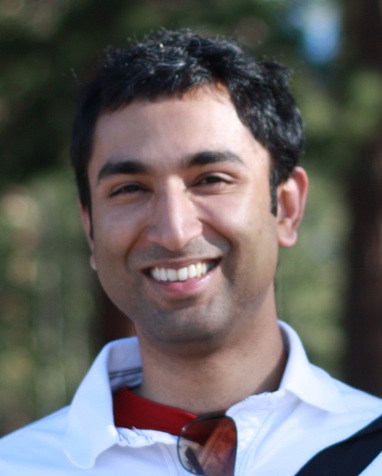
Bio: Dr. Nilesh Dalvi is a Database researcher whose research spans several areas of Data Management including Managing Uncertainty in Databases, Information Integration, Information Extraction and Crowdsourcing. He received his B.S. in Computer Science from IIT Bombay, Mumbai, in 2001, and his Ph.D. from University of Washington, Seattle in 2007. Dr. Dalvi has held the positions of Research Scientist at Yahoo! and Facebook. In 2008, he received an honorable mention for the ACM SIGMOD Best Dissertation Award. He has served as a Program Chair for the International Conference on Very Large Databases, 2012, and has published over 50 articles in the field of Data Management. Dr. Dalvi currently serves as the Chief Scientist at Trooly Inc.
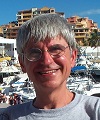
Bio: Dan Suciu is a Professor in Computer Science at the University of Washington. He received his Ph.D. from the University of Pennsylvania in 1995, was a principal member of the technical staff at AT&T Labs and joined the University of Washington in 2000. Suciu is conducting research in data management, with an emphasis on topics related to Big Data and data sharing, such as probabilistic data, data pricing, parallel data processing, data security. He is a co-author of two books Data on the Web: from Relations to Semistructured Data and XML, 1999, and Probabilistic Databases, 2011. He is a Fellow of the ACM, holds twelve US patents, received the best paper award in SIGMOD 2000 and ICDT 2013, the ACM PODS Alberto Mendelzon Test of Time Award in 2010 and in 2012, the 10 Year Most Influential Paper Award in ICDE 2013, and is a recipient of the NSF Career Award and of an Alfred P. Sloan Fellowship. Suciu serves on the VLDB Board of Trustees, and is an associate editor for the VLDB Journal, ACM TOIS, ACM TWEB, and Information Systems and is a past associate editor for ACM TODS. Suciu's PhD students Gerome Miklau and Christopher Re received the ACM SIGMOD Best Dissertation Award in 2006 and 2010 respectively, and Nilesh Dalvi was a runner up in 2008.
Early Career Award Talk


Engineering High-Performance Database Engines
Thomas Neumann, Technische Universitat at Munchen
Developing a database engine is both challenging and rewarding. Database engines are very complex software artifacts that have to scale to large data sizes and large hardware configurations, and developing such systems usually means choosing between different trade-offs at various points of development. This paper gives a survey over two different database engines, the disk-based SPARQL-processing engine RDF-3X, and the relational main-memory engine HyPer. It discusses the design choices that were made during development, and highlights optimization techniques that are important for both systems.
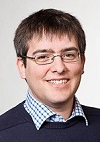
Bio: Thomas Neumann conducts research on database systems, focusing on query optimization and query processing. As part of that research he has build two very successful systems, RDF-3X for efficient processing of large RDF data, and the very fast main-memory database system HyPer. Their development induced many innovative techniques, including advanced join ordering techniques and efficient query compilation approaches. He studied business information systems at the University of Mannheim and received a doctorate in informatics from the same university.Before joining TUM as professor, Neumann was a senior researcher at the Max
List of best papers award recipients(notes:these papers will be presented during regular conference sessions)

The Uncracked Pieces in Database Cracking
Felix Martin Schuhknecht, Alekh Jindal, Jens Dittrich

epiC: an Extensible and Scalable System for Processing Big Data
Dawei Jiang, Gang Chen, Beng Chin Ooi, Kian-Lee Tan, Sai Wu

M4: A Visualization-Oriented Time Series Data Aggregation
Uwe Jugel, Zbigniew Jerzak, Gregor Hackenbroich, Volker Markl

Building Efficient Query Engines in a High-Level Language
Yannis Klonatos, Christoph Koch, Tiark Rompf, Hassan Chafi

On k-Path Covers and their Applications
Stefan Funke, Andre Nusser, Sabine Storandt
Thursday Sep 4th 10:30-12:15
Papers : Graph Data IV
Location: Diamond 1
Chair: Michael Carey


Optimizing Graph Algorithms on Pregel-like Systems
Semih Salihoglu* (Stanford University), Jennifer Widom (Stanford University)
We study the problem of implementing graph algorithms efficiently on Pregel-like systems, which can be surprisingly challenging. Standard graph algorithms in this setting can incur unnecessary inefficiencies such as slow convergence or high communication or computation cost, typically due to structural properties of the input graphs such as large diameters or skew in component sizes. We describe several optimization techniques to address these inefficiencies. Our most general technique is based on the idea of performing some serial computation on a tiny fraction of the input graph, complementing Pregel's vertex-centric parallelism. We base our study on thorough implementations of several fundamental graph algorithms, some of which have, to the best of our knowledge, not been implemented on Pregel-like systems before. The algorithms and optimizations we describe are fully implemented in our open-source Pregel implementation. We present detailed experiments showing that our optimization techniques improve runtime significantly on a variety of very large graph datasets.

Distributed Graph Simulation: Impossibility and Possiblity
Wenfei Fan (University of Edinburgh), Xin Wang (University of Edinburgh), YINGHUI WU* (University of California Santa), Dong Deng (Tsinghua University)


An Effective Encoding Scheme for Spatial RDF Data
John Liagouris (HKU), Nikos Mamoulis* (University of Hong Kong), Panagiotis Bouros (Humboldt-Universitaet zu Berlin), Manolis Terrovitis (IMIS `Athena')
The RDF data model has recently been extended to support representation and querying of spatial information (i.e., locations and geometries), which is associated with RDF entities. Still, there are limited efforts towards extending RDF stores to efficiently support spatial queries, such as range selections (e.g., find entities within a given range) and spatial joins (e.g., find pairs of entities whose locations are close to each other). In this paper, we propose an extension for RDF stores that supports efficient spatial data management. Our contributions include an effective encoding scheme for entities having spatial locations, the introduction of on-the-fly spatial filters and spatial join algorithms, and several optimizations that minimize the overhead of geometry and dictionary accesses. We implemented the proposed techniques as an extension to the open-source RDF-3X engine and we experimentally evaluated them using real RDF knowledge bases. The results show that our system offers robust performance for spatial queries, while introducing little overhead to the original query engine.


Fast Iterative Graph Computation with Block Updates
Wenlei Xie* (Cornell University), Guozhang Wang (Cornell University), David Bindel (Cornell University), Alan Demers (Cornell University), Johannes Gehrke (Cornell University)
Scaling iterative graph processing applications to large graphs is animportant problem. Performance is critical, as data scientists needto execute graph programs many times with varying parameters. Theneed for a high-level, high-performance programming model has inspiredsignificant research on high-level graph programming frameworks.In this paper, we show that the important class of computationally lightgraph applications -- applications that perform little computation pervertex -- has severe scalability problems across multiple cores as theyhit an early ``memory wall'' that limits their speedup. We thenpropose a novel block-oriented computation model where computation isiterated locally on blocks of highly connected nodes, thussignificantly improving the amount of computation per cache miss.Following this model, we describe the design andimplementation of a block-aware graph processingruntime which keeps the familiarvertex-centric programming paradigm while reaping all the benefits ofblock-oriented execution.Our experiments show that block-oriented execution significantlyimproves performance of our framework for several graph applications.


From "Think Like a Vertex" to "Think Like a Graph"
Yuanyuan Tian* (IBM Almaden Research Center), Andrey Balmin (GraphSQL), Severin Andreas Corsten (IBM Germany), Shirish Tatikonda (IBM Research), john McPherson (IBM Research)
To meet the challenge of processing rapidly growing graph and network data created by modern applications, a number of distributed graph processing systems have emerged, such as Pregel and GraphLab. All these systems divide input graphs into partitions, and employ a "think like a vertex" programming model to support iterative graph computation. This vertex-centric model is easy to program and has been proved useful for many graph algorithms. However, this model hides the partitioning information from the users, thus prevents many algorithm-specific optimizations. This often results in longer execution time due to excessive network messages (e.g. in Pregel) or heavy scheduling overhead to ensure data consistency (e.g. in GraphLab). To address this limitation, we propose a new "think like a graph" programming paradigm. Under this graph-centric model, the partition structure is opened up to the users, and can be utilized so that communication within a partition can bypass the heavy message passing or scheduling machinery. We implemented this model in a new system, called Giraph++, based on Apache Giraph, an open source implementation of Pregel. We explore the applicability of the graph-centric model to three categories of graph algorithms, and demonstrate its flexibility and superior performance, especially on well-partitioned data. For example, on a web graph with 118 million vertices and 855 million edges, the graph-centric version of connected component detection algorithm runs 63X faster and uses 204X fewer network messages than its vertex-centric counterpart.


An Experimental Comparison of Pregel-like Graph Processing Systems
Minyang Han* (University of Waterloo), Khuzaima Daudjee (University of Waterloo), Khaled Ammar (University of Waterloo), Tamer Ozsu (University of Waterloo), Xingfang Wang (University of Waterloo), Tianqi Jin (University of Waterloo)
The introduction of Google's Pregel generated much interest in the field of large-scale graph data processing, inspiring the development of Pregel-like systems such as Apache Giraph, GPS, Mizan, and GraphLab, all of which have appeared in the past two years. To gain an understanding of how Pregel-like systems perform, we conduct a study to experimentally compare Giraph, GPS, Mizan, and GraphLab on equal ground by considering graph and algorithm agnostic optimizations and by using several metrics. The systems are compared with four different algorithms (PageRank, single source shortest path, weakly connected components, and distributed minimum spanning tree) on up to 128 Amazon EC2 machines. We find that the system optimizations present in Giraph and GraphLab allow them to perform well. Our evaluation also shows Giraph 1.0.0's considerable improvement since Giraph 0.1 and identifies areas of improvement for all systems.
Papers : Indexing
Location: Diamond 2
Chair: Bin Cui


Adaptive Range Filters for Cold Data: Avoiding Trips to Siberia
Karolina Alexiou (ETH), Donald Kossmann* (ETH), Paul Larson (Microsoft)
Bloom filters are a great technique to test whether a key is notin a set of keys. This paper presents a novel datastructure called ARF. In a nutshell, ARFsare for range queries what Bloom filters are for point queries. Thatis, an ARF can determine whether a set of keys does not containany keys that are part of a specific range. This paper describes theprinciples and methods for efficient implementation ofARFs and presents the results of comprehensive experimentsthat assess the precision, space, and latency of ARFs.Furthermore, thispaper shows how ARFs can be applied to a commercial database systemthat partitions data into hot and cold regions to optimizequeries that involve only hot data.


Lightweight Indexing of Observational Data in Log-Structured Storage
Sheng Wang (National Univ. of Singapore), David Maier (Portland State University), Beng Chin Ooi* (National University of Singapore)
Huge amounts of data are being generated by sensing devices every day, recording the status of objects and the environment. Such observational data is widely used in scientific research. As the capabilities of sensors keep improving, the data produced are drastically expanding in precision and quantity, making it a write-intensive domain. Log-structured storage is capable of providing high write throughput, and hence is a natural choice for managing large-scale observational data. In this paper, we propose an approach to indexing and querying observational data in log-structured storage. Based on key traits of observational data, we design a novel index approach called the CR-index (Continuous Range Index), which provides fast query performance without compromising write throughput. It is a lightweight structure that is fast to construct and often small enough to reside in RAM. Our experimental results show that the CR-index is superior in handling observational data compared to other indexing techniques. While our focus is scientific data, we believe our index will be effective for other applications with similar properties, such as process monitoring in manufacturing.


The Uncracked Pieces in Database Cracking
Felix Martin Schuhknecht* (Saarland University), Alekh Jindal (MIT), Jens Dittrich (Saarland University)
Database cracking has been an area of active research in recent years. The core idea of database cracking is to create indexes adaptively and incrementally as a side-product of query processing. Several works have proposed different cracking techniques for different aspects including updates, tuple-reconstruction, convergence, concurrency-control, and robustness. However, there is a lack of any comparative study of these different methods by an independent group. In this paper, we conduct an experimental study on database cracking. Our goal is to critically review several aspects, identify the potential, and propose promising directions in database cracking. With this study, we hope to expand the scope of database cracking and possibly leverage cracking in database engines other than MonetDB. We repeat several prior database cracking works including the core cracking algorithms as well as three other works on convergence (hybrid cracking), tuple-reconstruction (sideways cracking), and robustness (stochastic cracking) respectively. We evaluate these works and show possible directions to do even better. We further test cracking under a variety of experimental settings, including high selectivity queries, low selectivity queries, and multiple query access patterns. Finally, we compare cracking against different sorting algorithms as well as against different main-memory optimised indexes, including the recently proposed Adaptive Radix Tree (ART). Our results show that: (i) the previously proposed cracking algorithms are repeatable, (ii) there is still enough room to significantly improve the previously proposed cracking algorithms, (iii) cracking depends heavily on query selectivity, (iv) cracking needs to catch up with modern indexing trends, and (v) different indexing algorithms have different indexing signatures.


Efficient Bulk Updates on Multiversion B-trees
Daniar Achakeev* (Philipps-Universität Marburg), Bernhard Seeger (University of Marburg)
Partial persistent index structures support efficient access to current and past versions of objects, while updates are allowed on the current version. The Multiversion B-Tree (MVBT) represents a partially persistent index-structure with both, asymptotic worst-case performance and excellent performance in real life applications. Updates are performed tuple-by-tuple with the same asymptotic performance as for standard B$^+$trees. To the best of our knowledge, there is no efficient algorithm for bulk loading and bulk update of MVBT and other partially persistent index structures. In this paper, we propose the first loading algorithm for MVBT that meets the lower-bound of external sorting. In addition, our approach is also applicable to bulk updates. This is achieved by combining two basic technologies, weight balancing and buffer tree. Our extensive set of experiments confirm the theoretical findings: Our loading algorithm runs considerably faster than performing updates tuple-by-tuple.


Bitlist: New Full-text Index for Low Space Cost and Efficient Keyword Search
Weixiong Rao* (University of Helsinki), Lei Chen (Honk Kong University of Science and Technology), Pan Hui (HKUST (Telekom Innovation Laboratories), Sasu Tarkoma (University of Helsinki)
Nowadays Web search engines are experiencing significant performance challenges caused by a huge amount of Web pages and increasingly larger number of Web users. The key issue for addressing these challenges is to design a compact structure which can index Web documents with low space and meanwhile process keyword search very fast. Unfortunately, the current solutions typically separate the space optimization from the search improvement. As a result, such solutions either save space yet with search inefficiency, or allow fast keyword search but with huge space requirement. In this paper, to address the challenges, we propose a novel structure bitlist with both low space requirement and supporting fast keyword search. Specifically, based on a simple yet very efficient encoding scheme, bitlist uses a single number to encode a set of integer document IDs for low space, and adopts fast bitwise operations for very efficient boolean-based keyword search. Based on real data sets, our extensive experimental results verify that bitlist outperforms the recent proposed solution, inverted list compression [23, 22] by spending 36.71% less space and 61.91% faster processing time, and achieves comparable running time as [8] but with significantly lower space.


Streaming Similarity Search over one Billion Tweets using Parallel Locality-Sensitive Hashing
Narayanan Sundaram* (Intel Corporation), Aizana Turmukhametova (MIT), Nadathur Satish (Intel Corporation), Todd Mostak (Harvard), Piotr Indyk (MIT), Sam Madden (MIT), Pradeep Dubey (Intel Corporation)
Finding nearest neighbors has become an important operation on databases, with applications to text search, multimedia indexing, and many other areas. One popular algorithm for similarity search, especially for high dimensional data (where spatial indexes like kd-trees do not perform well) is Locality Sensitive Hashing (LSH), an approximation algorithm for finding similar objects.In this paper, we describe a new variant of LSH, called Parallel LSH (PLSH) designed to be extremely efficient, capable of scaling out on multiple nodes and multiple cores, and which supports high-throughput streaming of new data. Our approach employs several novel ideas, including: cache-conscious hash table layout, using a 2-level merge algorithm for hash table construction; an efficient algorithm for duplicate elimination during hash-table querying; an insert-optimized hash table structure and efficient data expiration algorithm for streaming data; and a performance model that accurately estimates performance of the algorithm and can be used to optimize parameter settings. We show that on a workload where we perform similarity search on a dataset of 1 Billion tweets, with hundreds of millions of new tweets per day, we can achieve query times of 1-2.5 ms. We show that this is an order of magnitude faster than existing indexing schemes, such as inverted indexes. To the best of our knowledge, this is the fastest implementation of LSH, with table construction times up to 3.7X faster and query times that are 8.3X faster than a basic implementation.
Industrial : Big Data 2
Location: Diamond 3
Chair: Peter Boncz


Changing Engines in Midstream: A Java Stream Computational Model for Big Data Processing
Xueyuan Su* (Oracle Corporation)*,Garret Swart (Oracle Corporation),Brian Goetz (Oracle Corporation),Brian Oliver (Oracle Corporation),Paul Sandoz (Oracle Corporation)
With the addition of lambda expressions and the Stream API in Java 8, Java has gained a powerful and expressive query language that operates over in-memory collections of Java objects, making the transformation and analysis of data more convenient, scalable and efficient. In this paper, we build on Java 8 Stream and add a DistributableStream abstraction that supports federated query execution over an extensible set of distributed compute engines. Each query eventually results in the creation of a materialized result that is returned either as a local object or as an engine defined distributed Java Collection that can be saved and/or used as a source for future queries. Distinctively, DistributableStream supports the changing of compute engines both between and within a query, allowing different parts of a computation to be executed on different platforms. At execution time, the query is organized as a sequence of pipelined stages, each stage potentially running on a different engine. Each node that is part of a stage executes its portion of the computation on the data available locally or produced by the previous stage of the computation. This approach allows for computations to be assigned to engines based on pricing, data locality, and resource availability. Coupled with the inherent laziness of stream operations, this brings great flexibility to query planning and separates the semantics of the query from the details of the engine used to execute it. We currently support three engines, Local, Apache Hadoop MapReduce and Oracle Coherence, and we illustrate how new engines and data sources can be added.


Fast Foreign-Key Detection in Microsoft SQL Server PowerPivot for Excel
Zhimin Chen (Microsoft Research)*,Surajit Chaudhuri (Microsoft Research)
Microsoft SQL Server PowerPivot for Excel, or PowerPivot for short, is an in-memory business intelligence (BI) engine that enables Excel users to interactively create pivot tables over large data sets imported from sources such as relational databases, text files and web data feeds. Unlike traditional pivot tables in Excel that are defined on a single table, PowerPivot allows analysis over multiple tables connected via foreign-key joins. In many cases however, these foreign-key relationships are not known a priori, and information workers are often not be sophisticated enough to define these relationships. Therefore, the ability to automatically discover foreign-key relationships in PowerPivot is valuable, if not essential. The key challenge is to perform this detection interactively and with high precision even when data sets scale to hundreds of millions of rows and the schema contains tens of tables and hundreds of columns. In this paper, we describe techniques for fast foreign-key detection in PowerPivot and experimentally evaluate its accuracy, performance and scale on both synthetic benchmarks and real-world data sets. These techniques have been incorporated into PowerPivot for Excel.


Big Data Small Footprint: The Design of A Low-Power Classifier for Detecting Transportation Modes
Meng-Chieh Yu* (HTC, Studio Engineering)*,Tong Yu (National Taiwan University),ShaoChen Wang (HTC),Chih-Jen Lin (National Taiwan University),Edward Y. Chang (HTC)
Sensors on mobile phones and wearables, and in general sensors on IoT (Internet of Things), bring forth a couple of new challenges to big data research. First, the power consumption for analyzing sensor data must be low, since most wearables and portable devices are power-strapped. Second, the velocity of analyzing big data on these devices must be high, otherwise the limited local storage may overflow. This paper presents our hardware-software co-design of a classifier for wearables to detect a person's transportation mode (i.e., still, walking, running, biking, and on a vehicle). We particularly focus on addressing the big-data small-footprint requirement by designing a classifier that is low in both computational complexity and memory requirement. Together with a sensor-hub configuration, we are able to drastically reduce power consumption by 99%, while maintaining competitive mode-detection accuracy. The data used in the paper is made publicly available for conducting research.


Indexing HDFS Data in PDW: Splitting the data from the index
Vinitha Gankidi (University of Wisconsin, Madison)*,Jignesh Patel (University of Wisconsin),Alan Halverson (Microsoft Jim Gray Systems Lab),David Dewitt (Microsoft Jim Gray Research Lab)
There is a growing interest in making relational DBMSs work synergistically with MapReduce systems. However, there are interesting technical challenges associated with figuring out the right balance between the use and co-deployment of these systems. This paper focuses on one specific aspect of this balance, namely how to leverage the superior indexing and query processing power of a relational DBMS for data that is often more cost-effectively stored in Hadoop/HDFS. We present a method to use conventional B+-tree indices in an RDBMS for data stored in HDFS and demonstrate that our approach is especially effective for highly selective queries.
Papers : Data Analytics
Location: Diamond 4
Chair: Surajit Chaudhuri


Diversity based Relevance Feedback for Time Series Search
Bahaeddin ERAVCI* (Bilkent University), Hakan Ferhatosmanoglu (Bilkent University)
We propose a diversity based relevance feedback approach for time series data to improve the accuracy of search results. We first develop the concept of relevance feedback for time series based on dual-tree complex wavelet (CWT) and SAX based approaches. We enhance the search quality by incorporating diversity in the results presented to the user for feedback. We then propose a method which utilizes the representation type as part of the feedback, as opposed to a human choosing based on a preprocessing or training phase. The proposed methods involve a weighting system which can handle the relevance feedback of important properties for both single and multiple representation cases. Our experiments on a large variety of time series data sets show that the proposed diversity based relevance feedback improves the retrieval performance. Results confirm that representation feedback incorporates item diversity implicitly and achieves good performance even when using simple nearest neighbor as the retrieval method. To the best of our knowledge, this is the first study on diversification of time series search to improve retrieval accuracy as well as representation feedback.


More is Simpler: Effectively and Efficiently Assessing Node-Pair Similarities Based on Hyperlinks
Weiren Yu* (UNSW), Xuemin Lin (University of New South Wales), Wenjie Zhang (The University of New South Wales), Lijun Chang (UNSW), Jian Pei (SFU)
Similarity assessment is one of the core tasks in hyperlink analysis. Recently, with the proliferation of applications, \eg web search and collaborative filtering, SimRank has been a well-studied measure of similarity between two nodes in a graph. It recursively follows the philosophy that ``two nodes are similar if they are referenced (have incoming edges) from similar nodes'', which can be viewed as an aggregation of similarities based on incoming paths. Despite its popularity, SimRank has an undesirable property, \ie ``zero-similarity'': It only accommodates paths with \emph{equal} length from a common ``center'' node. Thus, a large portion of other paths are fully ignored. This paper attempts to remedy this issue. (1) We propose and rigorously justify SimRank*, a revised version of SimRank, which resolves such counter-intuitive ``zero-similarity'' issues while inheriting merits of the basic SimRank philosophy. (2) We show that the series form of SimRank* can be reduced to a fairly succinct and elegant closed form, which looks even simpler than SimRank, yet e Similarity assessment is one of the core tasks in hyperlink analysis. Recently, with the proliferation of applications, \eg web search and collaborative filtering, SimRank has been a well-studied measure of similarity between two nodes in a graph. It recursively follows the philosophy that ``two nodes are similar if they are referenced (have incoming edges) from similar nodes'', which can be viewed as an aggregation of similarities based on incoming paths. Despite its popularity, SimRank has an undesirable property, \ie ``zero-similarity'': It only accommodates paths with \emph{equal} length from a common ``center'' node. Thus, a large portion of other paths are fully ignored. This paper attempts to remedy this issue. (1) We propose and rigorously justify SimRank*, a revised version of SimRank, which resolves such counter-intuitive ``zero-similarity'' issues while inheriting merits of the basic SimRank philosophy. (2) We show that the series form of SimRank* can be reduced to a fairly succinct and elegant closed form, which looks even simpler than SimRank, yet enriches semantics without suffering from increased computational cost. This leads to a fixed-point iterative paradigm of SimRank* in $O(Knm)$ time on a graph of $n$ nodes and $m$ edges for $K$ iterations, which is comparable to SimRank. (3) To further optimize SimRank* computation, we leverage a novel clustering strategy via edge concentration. Due to its NP-hardness, we devise an efficient and effective heuristic to speed up SimRank* computation to $O(Kn\tilde{m})$ time, where $\tilde{m}$ is generally much smaller than $m$. (4) On real and synthetic data, we empirically verify the rich semantics of SimRank*, and demonstrate its high computation efficiency. nriches semantics without suffering from increased computational cost. This leads to a fixed-point iterative paradigm of SimRank* in $O(Knm)$ time on a graph of $n$ nodes and $m$ edges for $K$ iterations, which is comparable to SimRank. (3) To further optimize SimRank* computation, we leverage a novel clustering strategy via edge concentration. Due to its NP-hardness, we devise an efficient and effective heuristic to speed up SimRank* computation to $O(Kn\tilde{m})$ time, where $\tilde{m}$ is generally much smaller than $m$. (4) On real and synthetic data, we empirically verify the rich semantics of SimRank*, and demonstrate its high computation efficiency.


NOMAD: Non-locking, stOchastic Multi-machine algorithm for Asynchronous and Decentralized matrix completion
Hyokun Yun* (Purdue University), Hsiang-Fu Yu (University of Texas), Cho-Jui Hsieh (University of Texas), Vishwanathan S V N (Purdue University), Inderjit Dhillon (University of Texas)
We develop an efficient parallel distributed algorithm for matrix completion, named NOMAD (Non-locking, stOchastic Multi-machine algorithm for Asynchronous and Decentralized matrix completion). NOMAD is a decentralized algorithm with non-blocking communication between processors. One of the key features of NOMAD is that the ownership of a variable is asynchronously transferred between processors in a decentralized fashion. As a consequence it is a lock-free parallel algorithm. In spite of being an asynchronous algorithm, the variable updates of NOMAD are serializable, that is, there is an equivalent update ordering in a serial implementation. NOMAD outperforms synchronous algorithms which require explicit bulk synchronization after every iteration: our extensive empirical evaluation shows that not only does our algorithm perform well in distributed setting on commodity hardware, but also outperforms state-of-the-art algorithms on a HPC cluster both in multi-core and distributed memory settings.


Attraction and Avoidance Detection from Movements
Zhenhui Li* (Penn State University), Bolin Ding (Microsoft Research), Fei Wu (Penn State University), Tobias Kin Hou Lei (Univ. of Illinois at Urbana-Champaign), Roland Kays (North Carolina Museum of Natural Sciences), Margaret Crofoot (University of California Davis)
With the development of positioning technology, movement data has become widely available nowadays. An important task in movement data analysis is to mine the relationships among moving objects based on their spatiotemporal interactions. Among all relationship types, attraction and avoidance are arguably the most natural ones. However, rather surprisingly, there is no existing method that addresses the problem of mining significant attraction and avoidance relationships in a well-defined and unified framework. In this paper, we propose a novel method to measure the significance value of relationship between any two objects by examining the background model of their movements via permutation test. Since permutation test is computationally expensive, two effective pruning strategies are developed to reduce the computation time. Furthermore, we show how the proposed method can be extended to efficiently answer the classic threshold query: given an object, retrieve all the objects in the database that have relationships, whose significance values are above certain threshold, with the query object. Empirical studies on both synthetic data and real movement data demonstrate the effectiveness and efficiency of our method.


Splitter: Mining Fine-Grained Sequential Patterns in Semantic Trajectories
Chao Zhang* (UIUC), Jiawei Han (University of Illinois), Lidan Shou (Zhejiang University), Jiajun Lu (UIUC), Thomas La Porta (PSU)
Driven by the advance of positioning technology and the popularity of location-sharing services, semantic-enriched trajectory data have become unprecedentedly available. The sequential patterns hidden in such data, when properly defined and extracted, can greatly benefit tasks like targeted advertising and urban planning. Unfortunately, classic sequential pattern mining algorithms developed for transactional data cannot effectively mine patterns in semantic trajectories, mainly because the places in the continuous space cannot be regarded as independent items. Instead, similar places need to be grouped to collaboratively form frequent sequential patterns. That said, it remains a challenging task to mine what we call fine-grained sequential patterns, which must satisfy spatial compactness, semantic consistency and temporal continuity simultaneously. We propose Splitter to effectively mine such fine-grained sequential patterns in two steps. In the first step, it retrieves a set of spatially coarse patterns, each attached with a set of trajectory snippets that precisely record the pattern's occurrences in the database. In the second step, Splitter breaks each coarse pattern into fine-grained ones in a top-down manner, by progressively detecting dense and compact clusters in a higher-dimensional space spanned by the snippets. Splitter uses an effective algorithm called weighted snippet shift to detect such clusters, and leverages a divide-and-conquer strategy to speed up the top-down pattern splitting process. Our experiments on both real and synthetic data sets demonstrate the effectiveness and efficiency of Splitter.


GRAMI: Frequent Subgraph and Pattern Mining in a Single Large Graph
Mohammed ElSeidy (EPFL), Ehab Abdelhamid* (KAUST), Spiros Skiadopoulos (University of Peloponnese), Panos Kalnis (King Abdullah University of Science and Technology)
Mining frequent subgraphs is an important operation on graphs; it is defined as finding all subgraphs that appear frequently in a database according to a given frequency threshold. Most existing work assumes a database of many small graphs, but modern applications, such as social networks, citation graphs, or protein-protein interactions in bioinformatics, are modeled as a single large graph. In this paper we present GRAMI, a novel framework for frequent subgraph mining in a single large graph. GRAMI undertakes a novel approach that only finds the minimal set of instances to satisfy the frequency threshold and avoids the costly enumeration of all instances required by previous approaches. We accompany our approach with a heuristic and optimizations that significantly improve performance. Additionally, we present an extension of GRAMI that mines frequent patterns. Compared to subgraphs, patterns offer a more powerful version of matching that captures transitive interactions between graph nodes (like friend of a friend) which are very common in modern applications. Finally, we present CGRAMI, a version supporting structural and semantic constraints, and AGRAMI, an approximate version producing results with no false positives. Our experiments on real data demonstrate that our framework is up to 2 orders of magnitude faster and discovers more interesting patterns than existing approaches.
Papers : Data Integration
Location: Diamond 5
Chair: Yannis Papakonstantinou


Rank Discovery From Web Databases
Saravanan Thirumuruganathan* (University of Texas at Arlington), Nan Zhang (George Washington University), Gautam Das (University of Texas at Arlington)
Many web databases are only accessible through a proprietary search interface which allows users to form a query by entering the desired values for a few attributes. After receiving a query, the system returns the top-k matching tuples according to a pre-determined ranking function. Since the rank of a tuple largely determines the attention it receives from website users, ranking information for any tuple - not just the top-ranked ones - is often of significant interest to third parties such as sellers, customers, market researchers and investors. In this paper, we define a novel problem of rank discovery over hidden web databases. We introduce a taxonomy of ranking functions, and show that different types of ranking functions require fundamentally different approaches for rank discovery. Our technical contributions include principled and efficient randomized algorithms for estimating the rank of a given tuple, as well as negative results which demonstrate the inefficiency of any deterministic algorithm. We show extensive experimental results over real-world databases, including an online experiment at Amazon.com, which illustrates the effectiveness of our proposed techniques.


On Concise Set of Relative Candidate Keys
Shaoxu Song* (Tsinghua University), Lei Chen (Hong Kong University of Science and Technology), Hong Cheng (The Chinese University of Hong Kong)
Matching keys, specifying what attributes to compare and how to compare them for identifying the same real-world entities, are found to be useful in applications like record matching, blocking and windowing [7]. Owing to the complex redundant semantics among matching keys, capturing a proper set of matching keys is highly non-trivial. Analogous to minimal/candidate keys w.r.t. functional dependencies, relative candidate keys (rcks [7], with a minimal number of compared attributes, see a more formal definition in Section 2) can clear up redundant semantics w.r.t. "what attributes to compare". However, we note that redundancy issues may still exist among rcks on the same attributes about "how to compare them". In this paper, we propose to find a concise set of matching keys, which has less redundancy and can still meet the requirements on coverage and validity. Specifically, we study approximation algorithms to efficiently discover a near optimal set. To ensure the quality of matching keys, the returned results are guaranteed to be rcks (minimal on compared attributes), and most importantly, minimal w.r.t. distance restrictions (i.e., redundancy free w.r.t. "how to compare the attributes"). The experimental evaluation demonstrates that our concise rck set is more effective than the existing rck choosing method. Moreover, the proposed pruning methods show up to 2 orders of magnitude improvement w.r.t. time costs on concise rck set discovery.


Incremental Record Linkage
Anja Gruenheid* (ETH Zurich), Luna Dong (google), Divesh Srivastava (AT&T Labs)
Record linkage clusters records such that each cluster corresponds to a single distinct real-world entity. It is a crucial step in data cleaning and data integration. In the big data era, the velocity of data updates is often high, quickly making previous linkage results obsolete. This paper presents an end-to-end framework that can incrementally and efficiently update linkage results when data updates arrive. Our algorithms not only allow merging records in the updates with existing clusters, but also allow leveraging new evidence from the updates to fix previous linkage errors. Experimental results on three real and synthetic data sets show that our algorithms can significantly reduce linkage time without sacrificing linkage quality.


Tracking Entities in the Dynamic World: A Fast Algorithm for Matching Temporal Records
Yueh-Hsuan Chiang* (Univ. of Wisconsin Madison), AnHai Doan (Univ. of Wisconsin Madison), Jeffrey Naughton (Univ of Wisconsin Madison)
Identifying records referring to the same real world entity over time enables longitudinal data analysis. However, difficulties arise from the dynamic nature of the world: the entities described by a temporal data set often evolve their states over time. While the state of the art approach to temporal entity matching achieves high accuracy, this approach is computationally expensive and cannot handle large data sets. In this paper, we present an approach that achieves equivalent matching accuracy but takes far less time. Our key insight is ``static first, dynamic second.'' Our approach first runs an evidence-collection pass, grouping records without considering the possibility of entity evolution, as if the world were ``static.'' Then, it merges clusters from the initial grouping by determining whether an entity might evolve from the state described in one cluster to the state described in another cluster. This intuitively reduces a difficult problem, record matching with evolution, to two simpler problems: record matching without evolution, then ``evolution detection'' among the resulting clusters. Experimental results on several temporal data sets show that our approach provides an order of magnitude improvement in run time over the state-of-the-art approach while producing equivalent matching accuracy.


Online Ordering of Overlapping Data Sources
Mariam Salloum* (UC Riverside), Luna Dong (google), Divesh Srivastava (AT&T Labs), Vassilis Tsotras (UC Riverside)
Data integration systems offer a uniform interface for querying a large number of autonomous and heterogeneous data sources. Ideally, answers are returned as sources are queried and the answer list is updated as more answers arrive. Choosing a good ordering in which the sources are queried is critical for increasing the rate at which answers are returned. However, this problem is challenging since we often do not have complete or precise statistics of the sources, such as their coverage and overlap. It is further exacerbated in the Big Data era, which is witnessing two trends in Deep-Web data: first, obtaining a full coverage of data in a particular domain often requires extracting data from thousands of sources; second, there is often a big variation in overlap between different data sources. In this paper we present OASIS, an Online query Answering System for overlappIng Sources. OASIS has three key components for source ordering. First, the Overlap Estimation component estimates overlaps between sources according to available statistics under the Maximum Entropy principle. Second, the Source Ordering component orders the sources according to the new contribution they are expected to provide, and adjusts the ordering based on statistics collected during query answering. Third, the Statistics Enrichment component selects critical missing statistics to enrich at runtime. Experimental results on both real and synthetic data show high efficiency and scalability of our algorithm.
Tutorial : Enterprise search in the big data era
Location: Bauhinia 1
Chair: Tutorial 4 Chair


Enterprise search in the big data era
Yunyao Li, Ziyang Liu, Huaiyu Zhu
Enterprise search allows users in an enterprise to retrieve desired information through a simple search interface. It is widely viewed as an important productivity tool within an enterprise. While Internet search engines have been highly successful, enterprise search remains notoriously challenging due to a variety of unique challenges, and is being made more so by the increasing heterogeneity and volume of enterprise data. On the other hand, enterprise search also presents opportunities to succeed in ways beyond current Internet search capabilities. This tutorial presents an organized overview of these challenges and opportunities, and reviews the state-of-the-art techniques for building a reliable and high quality enterprise search engine, in the context of the rise of big data.
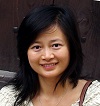
Bio: Yunyao Li is a researcher at IBM Research—Almaden. She has broad interests across multiple disciplines, most notably databases, natural language processing, human-computer interaction, information retrieval, and machine learning. Her current research focuses on enterprise search and scalable declarative text analytics for enterprise applications. She is the owner of several key components in the search engine that is currently powering IBM intranet search. She received her PhD degree in Computer Science and Engineering from the University of Michigan, Ann Arbor in 2007.
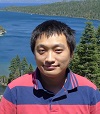
Bio: Ziyang Liu is a researcher at the Data Management department at NEC Laboratories America. His research interests span several topics in data management, including efficient and iterative big data analytics, data pricing, multitenant databases, data usability and effectively searching structured data with keywords. He got his Ph.D. from the School of Computing, Informatics, and Decision Systems Engineering at Arizona State University in 2011. He also received B.S. degree in computer engineering from Harbin Institute of Technology, China, in 2006.
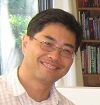
Bio: Huaiyu Zhu is with IBM Research—Almaden. He received his PhD degree in Computational Mathematics and Statistics from Liverpool University. His research interest includes statistical and machine learning techniques in data mining applications, especially in text analytics and large scale enterprise applications. In the past several years his main research focus was on enterprise search.
Demo 3
Location: Pearl
Chair: Demo 3 Chair


Ocelot/HyPE: Optimized Data Processing on Heterogeneous Hardware
Max Heimel* (TU Berlin),Sebastian Breß (Unversity of Magdeburg),Michael Saecker (Parstream GmbH),Bastian Koecher (Technische University Berlin),Volker Markl (TU Berlin),Gunter Saake (Unversity of Magdeburg)
The past years saw the emergence of highly heterogeneous server architectures that feature multiple accelerators in addition to the main processor. Efficiently exploiting these systems for data processing is a challenging research problem that comprises many facets, including how to find an optimal operator placement strategy, how to estimate runtime costs across different hardware architectures, and how to manage the code and maintenance blowup caused by having to support multiple architectures. In prior work, we already discussed solutions to some of these problems: First, we showed that specifying operators in a hardware-oblivious way can prevent code blowup while still maintaining competitive performance when supporting multiple architectures. Second, we presented learning cost functions and several heuristics to efficiently place operators across all available devices. In this demonstration, we provide further insights into this line of work by presenting our combined system Ocelot/HyPE. Our system integrates a hardware-oblivious data processing engine with a learning query optimizer for placement decisions, resulting in a highly adaptive DBMS that is specifically tailored towards heterogeneous hardware environments.


MoveMine2.0: Mining Object Relationships from Movement Data
Zhenhui Li (Penn State University),Fei Wu* (Penn State University),Tobias Kin Hou Lei (UIUC),Jiawei Han (University of Illinois)
The development in positioning technology has enabled us to collect a huge amount of movement data from moving objects, such as people, animals, and vehicles. The data embed rich information about the relationships among moving objects and have applications in many fields, e.g., in ecological study and human behavioral study. Previously, we propose a system MoveMine that integrates several start-of-art movement mining methods. However, it does not include recent methods on relationship pattern mining. Thus, we add substantial new methods and propose a new system, MoveMine 2.0, to support mining of dynamic relationship patterns. Newly added methods focus on two types of pairwise relationship patterns: (i) attraction/avoidance relationship, and (ii) following pattern. A user-friendly interface is designed to support interactive exploration of the result and provide flexibility in tuning the parameters. MoveMine 2.0 is tested on multiple types of real datasets to ensure its practical use. Our system provides useful tools for domain experts to gain insights on real dataset. Meanwhile, it will promote further research in relationship mining from moving objects.


WARP: A Partitioning Framework for Aggressive Data Skipping
Liwen Sun* (UC Berkeley),Sanjay Krishnan (UC Berkeley),Reynold Xin (UC Berkeley),Michael Franklin (UC Berkeley)
We propose to demonstrate a fine-grained partitioning framework that reorganizes the data tuples into small blocks at data loading time. The goal is to enable queries to maximally skip scanning data blocks. The partition framework consists of four steps: (1) workload analysis, which extracts features from a query workload, (2) augmentation, which augments each data tuple with a feature vector, (3) reduce, which succinctly represents a set of data tuples using a set of feature vectors, and (4) partitioning, which performs a clustering algorithm to partition the feature vectors and uses the clustering result to guide the actual data partitioning. Our experiments show that our techniques result in a 3-7x query response time improvement over traditional range partitioning due to more effective data skipping.


Interactive Outlier Exploration in Big Data Streams
Lei Cao* (WPI),Qingyang Wang (WPI),Elke Rundensteiner (WPI)
We demonstrate our VSOutlier system for supporting interactive exploration of outliers in big data streams. VSOutlier not only supports a rich variety of outlier types supported by innovative and efficient outlier detection strategies, but also provides a rich set of interactive interfaces to explore outliers in real time. Using the stock transactions dataset from the US stock market and the moving objects dataset from MITRE, we demonstrate that the VSOutlier system enables the analysts to more efficiently identify, understand, and respond to phenomena of interest in near real-time even when applied to high volume streams.


SQL/AA : Executing SQL on an Asymmetric Architecture
Quoc-Cuong To* (INRIA Rocquencourt UVSQ),Benjamin Nguyen (INRIA Rocquencourt University of Versailles),Philippe Pucheral (INRIA/UVSQ)
Current applications, from complex sensor systems (e.g. quantified self) to online e-markets acquire vast quantities of personal information which usually ends-up on central servers. Decentralized architectures, devised to help individuals keep full control of their data, hinder global treatments and queries, impeding the development of services of great interest. This paper promotes the idea of pushing the security to the edges of applications, through the use of secure hardware devices controlling the data at the place of their acquisition. To solve this problem, we propose secure distributed querying protocols based on the use of a tangible physical element of trust, reestablishing the capacity to perform global computations without revealing any sensitive information to central servers. There are two main problems when trying to support SQL in this context: perform joins and perform aggregations. In this paper, we study the subset of SQL queries without joins and show how to secure their execution in the presence of honest-but-curious attackers.


gMission: A General Spatial Crowdsourcing Platform
Zhao Chen* (HKUST),Rui Fu (HKUST),Ziyuan Zhao (HKUST),Zheng Liu (HKUST),Leihao Xia (HKUST),Lei Chen (Hong Kong University of Science and Technology),Peng Cheng (HKUST),Chen Cao (HKUST),Yongxin Tong (HKUST),CHEN ZHANG (HKUST)
As one of the successful forms of using Wisdom of Crowd, crowdsourcing, has been widely used for many human intrinsic tasks, such as image labeling, natural language understanding, market predication and opinion mining. Meanwhile, with advances in pervasive technology, mobile devices, such as mobile phones and tablets, have become extremely popular. These mobile devices can work as sensors to collect multimedia data(audios, images and videos) and location information. This power makes it possible to implement the new crowdsourcing mode: spatial crowdsourcing. In spatial crowdsourcing, a requester can ask for resources related a specific location, the mobile users who would like to take the task will travel to that place and get the data. Due to the rapid growth of mobile device uses, spatial crowdsourcing is likely to become more popular than general crowdsourcing, such as Amazon Turk and Crowdflower. However, to implement such a platform, effective and efficient solutions for worker incentives, task assignment, result aggregation and data quality control must be developed. In this demo, we will introduce gMission, a general spatial crowdsourcing platform, which features with a collection of novel techniques, including geographic sensing, worker detection, and task recommendation. We introduce the sketch of system architecture and illustrate scenarios via several case analysis.


S-Store: A Streaming NewSQL System for Big Velocity Applications
Ugur Cetintemel (Brown University),Daehyun Kim (Intel Labs),Tim Kraska (Brown University),Samuel Madden (MIT CSAIL),David Maier (Portland State University),John Meehan (Brown University),Andy Pavlo (CMU),Michael Stonebraker (MIT CSAIL),Nesime Tatbul* (Intel)
First-generation streaming systems did not pay much attention to state management via ACID transactions. S-Store is a data management system that combines OLTP transactions with stream processing. To create S-Store, we begin with H-Store, a main-memory transaction processing engine, and add primitives to support streaming. This includes triggers and transaction workflows to implement push-based processing, windows to provide a way to bound the computation, and tables with hidden state to implement scoping for proper isolation. This demo explores the benefits of this approach by showing how a naïve implementation of our benchmarks using only H-Store can yield incorrect results. We also show that by exploiting push-based semantics and our implementation of triggers, we can achieve significant improvement in transaction throughput. We demo two modern applications: (i) leaderboard maintenance for a version of "American Idol", and (ii) a city-scale bicycle rental scenario.


CLEar: A Realtime Online Observatory for Bursty and Viral Events
Runquan Xie* (Singapore Management Universit),Feida Zhu (Singapore Management Universit),Hui Ma (Singapore Management University),Wei Xie (Singapore Management University),Chen Lin (Xiamen University)
We describe our demonstration of CLEar (Clairaudient Ear ), a real-time online platform for detecting, monitoring, summarizing, contextualizing and visualizing bursty and viral events, those triggering a sudden surge of public interest and going viral on micro-blogging platforms. This task is challenging for existing methods as they either use complicated topic models to analyze topics in a off-line manner or define temporal structure of fixed granularity on the data stream for online topic learning, leaving them hardly scalable for real-time stream like that of Twitter. In this demonstration of CLEar, we present a three-stage system: First, we show a real-time bursty event detection module based on a data-sketch topic model which makes use of acceleration of certain stream quantities as the indicators of topic burstiness to trigger efficient topic inference. Second, we demonstrate popularity prediction for the detected bursty topics and event summarization based on clustering related topics detected in successive time periods. Third, we illustrate CLEar’s module for contextualizing and visualizing the event evolution both along time-line and across other news media to offer an easier understanding of the events.


AZDBLab: A Laboratory Information System for a Large-scale Empirical DBMS Study
Young-Kyoon Suh* (University of Arizona),Richard Snodgrass (University of Arizona),Rui Zhang (Teradata)
In the database field, while very strong mathematical and engineering work has been done, the scientific approach has been much less prominent. The deep understanding of query optimizers obtained through the scientific approach can lead to better engineered designs. Unlike other domains, there have been few DBMS-dedicated laboratories, focusing on such scientific investigation. In this demonstration, we present a novel DBMS-oriented research infrastructure, called Arizona Database Laboratory (AZDBLab), to assist database researchers in conducting a large-scale empirical study across multiple DBMSes. For them to test their hypotheses on the behavior of query optimizers, AZDBLab can run and monitor a large-scale experiment with thousands (or millions) of queries on different DBMSes. Furthermore, AZDBLab can help users automatically analyze these queries. In the demo, the audience will interact with AZDBLab through the stand-alone application and the mobile app to conduct such a large-scale experiment for a study. The audience will then run a Tucson Timing Protocol analysis on the finished experiment and then see the analysis (data sanity check and timing) results.


Terrain-Toolkit: A Multi-Functional Tool for Terrain Data
Qi Wang (Zhejiang University),Manohar Kaul (Aarhus University),Cheng Long* (HKUST),Raymond Chi-Wing Wong (Hong Kong University of Science and Technology)
Terrain data is becoming increasingly popular both in industry and in academia. Many tools have been developed for visualizing terrain data. However, we find that (1) they usually accept very few data formats of terrain data only; (2) they do not support terrain simplification well which, as will be shown, is used heavily for query processing in spatial databases; and (3) they do not provide the surface distance operator which is fundamental for many applications based on terrain data. Motivated by this, we developed a tool called Terrain-Toolkit for terrain data which accepts a comprehensive set of data formats, supports terrain simplification and provides the surface distance operator.


FORWARD: Data-Centric UIs using Declarative Templates that Efficiently Wrap Third-Party JavaScript Components
Kian Win Ong* (UCSD),Yannis Papakonstantinou (UC San Diego),Erick Zamora (UCSD)
While Ajax programming and the plethora of JavaScript component libraries enable high-quality UIs in web applications, integrating them with page data is laborious and error-prone as a developer has to handcode incremental modifications with trigger-based programming and manual coordination of data dependencies. The FORWARD web framework simplifies the development of Ajax applications through declarative, state-based templates. This declarative, data-centric approach is characterized by the principle of logical/physical independence, which the database community has often deployed successfully. It enables FORWARD to leverage database techniques, such as incremental view maintenance, updatable views, capability-based component wrappers and cost-based optimization to automate efficient live visualizations. We demonstrate an end-to-end system implementation, including a web-based IDE (itself built in FORWARD), academic and commercial applications built in FORWARD and a wide variety of JavaScript components supported by the declarative templates.
Thursday Sep 4th 12:15-13:45
Sep 4th Lunch
Location: Crystal
Sep 4th Lunch
Thursday Sep 4th 13:45-15:00
Papers : Architecture Systems
Location: Diamond 1
Chair: Ken Salem


Concurrent Analytical Query Processing with GPUs
Kaibo Wang* (The Ohio State University), Kai Zhang (The Ohio State University), Yuan Yuan (The Ohio State University), Siyuan Ma (The Ohio State University), Rubao Lee (The Ohio State University), Xiaoning Ding (Ner Jersey Institute of Technology), Xiaodong Zhang (The Ohio State University)
In current databases, GPUs are used as dedicated accelerators to process each individual query. Sharing GPUs among concurrent queries is not supported, causing serious resource underutilization. Based on the proling of an open-source GPU query engine running commonly used single-query data warehousing workloads, we observe that the utilization of main GPU resources is only up to 25%. The underutilization leads to low system throughput. To address the problem, this paper proposes concurrent query execution as an effective solution. To efficiently share GPUs among concurrent queries for high throughput, the major challenge is to provide software support to control and resolve resource contention incurred by the sharing. Our solution relies on GPU query scheduling and device memory swapping policies to address this challenge. We have implemented a prototype system and evaluated it intensively. The experiment results confirm the effectiveness and performance advantage of our approach. By executing multiple GPU queries concurrently, system throughput can be improved by up to 55% compared with dedicated processing.


Low-Latency Handshake Join
Pratanu Roy* (ETH Zurich), Jens Teubner (TU Dortmund University), Rainer Gemulla (Max-Plack-Institut Saarbrücken)
This work revisits the processing of stream joins on modern hardware architectures. Our work is based on the recently proposed handshake join algorithm, which is a mechanism to parallelize the processing of stream joins in a NUMA-aware and hardware-friendly manner. Handshake join achieves high throughput and scalability, but it suffers from a high latency penalty and a non-deterministic ordering of the tuples in the physical result stream. In this paper, we first characterize the latency behavior of the handshake join and then propose a new low-latency handshake join algorithm, which substantially reduces latency without sacrificing throughput or scalability. We also present a technique to generate punctuated result streams with very little overhead; such punctuations allow the generation of correctly ordered physicial output streams with negligible effect on overall throughput and latency.


Ibex - An Intelligent Storage Engine with Support for Advanced SQL Off-loading
Louis Woods* (ETH Zurich), Gustavo Alonso (Systems Group (ETH Zurich), Zsolt Istvan (ETH Zurich)
Modern data appliances face severe bandwidth bottlenecks when moving vast amounts of data from storage to the query processing nodes. A possible solution to mitigate these bottlenecks is query off-loading to an intelligent storage engine, where partial or whole queries are pushed down to the storage engine. In this paper, we present Ibex, a prototype of an intelligent storage engine that supports off-loading of complex query operators. Besides increasing performance, Ibex also reduces energy consumption, as it uses an FPGA rather than conventional CPUs to implement the off-load engine. Ibex is a hybrid engine, with dedicated hardware that evaluates SQL expressions at line-rate and a software fallback for tasks that the hardware engine cannot handle. Ibex supports GROUP BY aggregation, as well as projection- and selection-based filtering. GROUP BY aggregation has a higher impact on performance but is also a more challenging operator to implement on an FPGA.


When Data Management Systems Meet Approximate Hardware: Challenges and Opportunities
Bingsheng He*, NTU Singapore
Recently, approximate hardware designs have got many research interests in the computer architecture community. The essential idea of approximate hardware is that those hardware components (such as CPU, memory and storage) can trade off the accuracy of results for increased performance, energy consumption, or both. We propose a DBMS ApproxiDB with its design, implementation and optimization aware of the underlying approximate hardware. ApproxiDB will run on a hybrid machine with both approximate hardware and precise hardware (i.e., the conventional hardware without sacrificing the accuracy). With approximate hardware, ApproxiDB can efficiently support the concept of approximate query processing, without the overhead of pre-computed synopses or sampling techniques. More importantly, ApproxiDB is also beneficial to precise query processing, by developing non-trivial hybrid execution mechanisms on both precise and approximate hardware. In this vision paper, we sketch the initial design of ApproxiDB, discuss the technical challenges in building this system and outline an agenda for future research.
Papers : Dissemination
Location: Diamond 2
Chair: Peter Triantafillou


An Efficient Publish/Subscribe Index for E-Commerce Databases
Dongxiang Zhang (NUS), Chee-Yong Chan (National University of Singapore), Kian-Lee Tan* (NUS)
Many of today's publish/subscribe (pub/sub) systems have been designed to cope with a large {\em volume} of subscriptions and high event arrival rate ({\em velocity}). However, in many novel applications (such as e-commerce), there is an increasing {\em variety} of items, each with different attributes. This leads to a very high-dimensional and sparse database that existing pub/sub systems can no longer support effectively. In this paper, we propose an efficient in-memory index that is scalable to the volume and update of subscriptions, the arrival rate of events and the variety of subscribable attributes. The index is also extensible to support complex scenarios such as prefix/suffix filtering and regular expression matching. We conduct extensive experiments on synthetic datasets and two real datasets (AOL query log and Ebay products). The results demonstrate the superiority of our index over state-of-the-art methods: our index incurs orders of magnitude less index construction time, consumes a small amount of memory and performs event matching efficiently.


Delta: Scalable Data Dissemination under Capacity Constraints
Konstantinos Karanasos (IBM Almaden Research Center), Asterios Katsifodimos* (INRIA Saclay), Ioana Manolescu (INRIA (France)
In content-based publish-subscribe (pub/sub) systems, users express their interests as queries over a stream of publications. Scaling up content-based pub/sub to very large numbers of subscriptions is challenging: users are interested in low latency, that is, getting subscription results fast, while the pub/sub system provider is mostly interested in scaling, i.e., being able to serve large numbers of subscribers, with low computational resources utilization. We present a novel approach for scalable content-based pub/sub in the presence of constraints on the available CPU and network resources, implemented within our pub/sub system Delta. We achieve scalability by off-loading some subscriptions from the pub/sub server, and leveraging view-based query rewriting to feed these subscriptions from the data accumulated in others. Our main contribution is a novel algorithm for organizing views in a multi-level dissemination network, exploiting view-based rewriting and pow- erful linear programming capabilities to scale to many views, respect capacity constraints, and minimize latency. The efficiency and effectiveness of our algorithm are confirmed through extensive experiments and a large deployment in a WAN.


Optimal Crowd-Powered Rating and Filtering Algorithms
Aditya Parameswaran* (Stanford University), Stephen Boyd (Stanford), Hector Garcia Molina (Stanford University), Ashish Gupta (Stanford), Neoklis Polyzotis (Univ. of California Santa Cruz), Jennifer Widom (Stanford University)
We focus on crowd-powered filtering, i.e., filtering a large set of items using humans. Filtering is one of the most commonly used building blocks in crowdsourcing applications and systems. While solutions for crowd-powered filtering exist, they make a range of implicit assumptions and restrictions, ultimately rendering them not powerful enough for real-world applications. We describe two approaches to discard these implicit assumptions and restrictions: one, that carefully generalizes prior work, leading to an optimal, but often-times intractable solution, and another, that provides a novel way of reasoning about filtering strategies, leading to a sometimes sub-optimal, but efficiently computable solution (that is provably close to optimal). We demonstrate that our techniques lead to significant reductions in error of up to 30% for fixed cost over prior work in a novel crowdsourcing application: peer evaluation in online courses.


SeeDB: Visualizing Database Queries Efficiently
Aditya Parameswaran* (Stanford University), Neoklis Polyzotis (Univ. of California Santa Cruz), Hector Garcia Molina (Stanford University)
Data scientists rely on visualizations to interpret the data returned by queries, but finding the right visualization remains a manual task that is often laborious. We propose a DBMS, titled SeeDB, that partially automates the task of finding the right visualizations for a query. In a nutshell, given an input query Q, the new DBMS optimizer will explore not only the space of physical plans for Q, but also the space of possible visualizations for the results of Q. The output will comprise a recommendation of potentially "interesting" or "useful" visualizations, where each visualization is coupled with a suitable query execution plan. We discuss the technical challenges in building this system and outline an agenda for future research.
Local Industrial : Analytics
Location: Diamond 3
Chair: Lidan Shou

GEMINI: An Integrative Healthcare Analytics System
Zheng Jye Ling (National University Health System),Quoc Trung Tran (National University of Singapore),Ju Fan (National University of Singapore),Gerald C.H. Koh (National University Health System),Thi Nguyen (National University of Singapore),Chuen Seng Tan (National University Health System),James W. L. Yip (National University Health System),Meihui Zhang (National University of Singapore)

A Personalized Recommendation System for Dating Site
Chaoyue Dai (NetEase Inc.) , Feng Qian (NetEase Inc.), Wei Jiang (NetEase Inc.), Zhoutian Wang (NetEase Inc.), Zenghong Wu (NetEase Inc.)

Design and Implementation of a Real-Time Interactive Analytics System for Large Spatio-Temporal Data
Shiming Zhang (Huawei Noah's Ark Lab)*,Yin Yang (University of Illinois at Urbana-Champaign)*,Wei Fan (Huawei Noah's Ark Lab)*,Marianne Winslett (University of Illinois at Urbana-Champaign)
Papers : Multi-dimensional Access
Location: Diamond 4
Chair: Rui Zhang


SK-LSH : An Efficient Index Structure for Approximate Nearest Neighbor Search
Yingfan Liu (Xidian University), Jiaotao Cui (Xidian University), Helen Huang (University of Queensland), Hui Li (Xidian University), Heng Tao Shen* (The University of Queensland)
Approximate Nearest Neighbor (ANN) search in high dimensional space has become a fundamental paradigm in many applications. Recently, Locality Sensitive Hashing (LSH) and its variants are acknowledged as the most promising solutions to ANN search. However, state-of-the-art LSH approaches suffer from a drawback: accesses to candidate objects require a large number of \emph{random} I/O operations. In order to guarantee the quality of returned results, sufficient objects should be verified, which would consume enormous I/O cost. To address this issue, we propose a novel method, called SortingKeys-LSH (SK-LSH), which reduces the number of page accesses through locally arranging candidate objects. We firstly define a new measure to evaluate the distance between the compound hash keys of two points. A linear order relationship on the set of compound hash keys is then created, and the corresponding data points can be sorted accordingly. Hence, data points that are close to each other according to the distance measure can be stored locally in an index file. During the ANN search, only a limited number of disk pages among few index files are necessary to be accessed for sufficient candidate generation and verification, which not only significantly reduces the response time but also improves the accuracy of the returned results. Our exhaustive empirical study over several real-world data sets demonstrates the superior efficiency and accuracy of SK-LSH for the ANN search, compared with state-of-the-art methods, including LSB, C2LSH and CK-Means.


Earth Mover's Distance based Similarity Search at Scale
Yu Tang* (University of Hong Kong), Leong Hou U (University of Macau), Yilun Cai (University of Hong Kong), Nikos Mamoulis (University of Hong Kong), Reynold Cheng (University of Hong Kong)
Earth Mover's Distance (EMD), as a similarity measure, has received a lot of attention in the fields of multimedia and probabilistic databases, computer vision, image retrieval, machine learning, etc. EMD on multidimensional histograms provides better distinguishability between the objects approximated by the histograms (e.g., images), compared to classic measures like Euclidean distance. Despite its usefulness, EMD has a high computational cost; therefore, a number of effective filtering methods have been proposed, to reduce the pairs of histograms for which the exact EMD has to be computed, during similarity search. Still, EMD calculations in the refinement step remain the bottleneck of the whole similarity search process. In this paper, we focus on optimizing the refinement phase of EMD-based similarity search by (i) adapting an efficient min-cost flow algorithm (SIA) for EMD computation, (ii) proposing a dynamic distance bound, which can be used to terminate an EMD refinement early, and (iii) proposing a dynamic refinement order for the candidates which, paired with a concurrent EMD refinement strategy, reduces the amount of needless computations. Our proposed techniques are orthogonal to and can be easily integrated with the state-of-the-art filtering techniques, reducing the cost of EMD-based similarity queries by orders of magnitude.


Effective Multi-Modal Retrieval based on Stacked Auto-Encoders
Wei Wang (NUS), Beng Chin Ooi* (National University of Singapore), Xiaoyan Yang (Advanced Digital Science Center), Dongxiang Zhang (NUS), Yueting Zhuang (Zhejiang University)
Multi-modal retrieval is emerging as a new search paradigm that enables seamless information retrieval from various types of media. For example, users can simply snap a movie poster to search relevant reviews and trailers. To solve the problem, a set of mapping functions are learned to project high-dimensional features extracted from data of different media types into a common lowdimensional space so that metric distance measures can be applied. In this paper, we propose an effective mapping mechanism based on deep learning (i.e., stacked auto-encoders) for multi-modal retrieval. Mapping functions are learned by optimizing a new objective function, which captures both intra-modal and inter-modal semantic relationships of data from heterogeneous sources effectively. Compared with previous works which require a substantial amount of prior knowledge such as similarity matrices of intramodal data and ranking examples, our method requires little prior knowledge. Given a large training dataset, we split it into minibatches and continually adjust the mapping functions for each batch of input. Hence, our method is memory efficient with respect to the data volume. Experiments on three real datasets illustrate that our proposed method achieves significant improvement in search accuracy over the state-of-the-art methods.


Retrieving Regions of Interest for User Exploration
Xin Cao* (NTU), Gao Cong (Nanyang Technological University), Christian Jensen (Aalborg University), Man Lung Yiu (Hong Kong Polytechnic University)
We consider an application scenario where points of interest (PoIs) each have a web presence and where a web user wants to identify a region that contains relevant PoIs that are relevant to a set of keywords, e.g., in preparation for deciding where to go to conveniently explore the PoIs. Motivated by this, we propose the length-constrained maximum-sum region (LCMSR) query that returns a spatial-network region that is located within a general region of interest, that does not exceed a given size constraint, and that best matches query keywords. Such a query maximizes the total weight of the PoIs in it w.r.t. the query keywords. We show that it is NP-hard to answer this query. We develop an approximation algorithm with a (5 + \epsilon) approximation ratio utilizing a technique that scales node weights into integers. We also propose a more efficient heuristic algorithm and a greedy algorithm. Empirical studies on real data offer detailed insight into the accuracy of the proposed algorithms and show that the proposed algorithms are capable of computing results efficiently and effectively.
Papers : Database Usability I
Location: Diamond 5
Chair: Jun Yang


M4: A Visualization-Oriented Time Series Data Aggregation
Uwe Jugel* (SAP), Zbigniew Jerzak (SAP), Gregor Hackenbroich (SAP), Volker Markl (TU Berlin)
Visual analysis of high-volume time series data is ubiquitous in many industries, including finance, banking, and discrete manufacturing. Contemporary, RDBMS-based systems for visualization of high-volume time series data have difficulty to cope with the hard latency requirements and high ingestion rates of interactive visualizations. Existing solutions for lowering the volume of time series data disregard the semantics of visualizations and result in visualization errors. In this work, we introduce M4, an aggregation-based time series dimensionality reduction technique that provides error-free visualizations at high data reduction rates. Focusing on line charts, as the predominant form of time series visualization, we explain in detail the drawbacks of existing data reduction techniques and how our approach outperforms state of the art, by respecting the process of line rasterization. We describe how to incorporate aggregation-based dimensionality reduction at the query level in a visualization-driven query rewriting system. Our approach is generic and applicable to any visualization system that uses an RDBMS as data source. Using real world data sets from high tech manufacturing, stock markets, and sports analytics domains we demonstrate that our visualization-oriented data aggregation can reduce data volumes by up to two orders of magnitude, while preserving perfect visualizations.


Gestural Query Specification
Arnab Nandi* (Ohio State University), Lilong Jiang (The Ohio State University), Michael Mandel (The Ohio State University)
Direct, ad-hoc interaction with databases has typically been performed over console-oriented conversational interfaces using query languages such as SQL. With the rise in popularity of gestural user interfaces and computing devices that use gestures as their exclusive modes of interaction, database query interfaces require a fundamental rethinking to work without keyboards. We present a novel query specification system that allows the user to query databases using a series of gestures. We present a novel gesture recognition system that uses both the interaction and the state of the database to classify gestural input into relational database queries. We conduct exhaustive systems performance tests and user studies to demonstrate that our system is not only performant and capable of interactive latencies, but it is also more usable, faster to use and more intuitive than existing systems.


The Case for Data Visualization Management Systems
Eugene Wu* (MIT), Leilani Battle (MIT CSAIL), Samuel Madden (MIT CSAIL)
Most visualizations today are produced by retrieving data from a database and using a specialized visualization tool to render it. This decoupled approach results in significant duplication of functionality, such as aggregation and filters, and misses tremendous opportunities for cross-layer optimizations. In this paper, we present the case for an integrated Data Visualization Management System (DVMS) based on a declarative visualization language that fully compiles the end-to-end visualization pipeline into a set of relational algebra queries. Thus the DVMS can be both expressive via the visualization language, and performant by leveraging traditional and visualization-specific optimizations to scale interactive visualizations to massive datasets.


Exemplar Queries: Give me an Example of What You Need
Davide Mottin* (University of Trento), Matteo Lissandrini (University of Trento), Yannis Velegrakis (Yannis Velegrakis), Themis Palpanas (Paris Descartes University)
Search engines are continuously employing advanced techniques that aim to capture user intentions and provide results that go beyond the data that simply satisfy the query conditions. Examples include the personalized results, related searches, similarity search, popular and relaxed queries. In this work we introduce a novel query paradigm that considers a user query as an example of the data in which the user is interested. We call these queries exemplar queries and claim that they can play an important role in dealing with the information deluge. We provide a formal specification of the semantics of such queries and show that they are fundamentally different from notions like queries by example, approximate and related queries. We provide an implementation of these semantics for graph-based data and present an exact solution with a number of optimizations that improve performance without compromising the quality of the answers. We also provide an approximate solution that prunes the search space and achieves considerably better time-performance with minimal or no impact on effectiveness. We experimentally evaluate the effectiveness and efficiency of these solutions with synthetic and real datasets, and illustrate the usefulness of exemplar queries in practice.
Tutorial : Enterprise search in the big data era
Location: Bauhinia 1
Chair: Tutorial 4 Chair


Enterprise search in the big data era
Yunyao Li, Ziyang Liu, Huaiyu Zhu
Enterprise search allows users in an enterprise to retrieve desired information through a simple search interface. It is widely viewed as an important productivity tool within an enterprise. While Internet search engines have been highly successful, enterprise search remains notoriously challenging due to a variety of unique challenges, and is being made more so by the increasing heterogeneity and volume of enterprise data. On the other hand, enterprise search also presents opportunities to succeed in ways beyond current Internet search capabilities. This tutorial presents an organized overview of these challenges and opportunities, and reviews the state-of-the-art techniques for building a reliable and high quality enterprise search engine, in the context of the rise of big data.
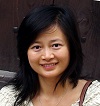
Bio: Yunyao Li is a researcher at IBM Research—Almaden. She has broad interests across multiple disciplines, most notably databases, natural language processing, human-computer interaction, information retrieval, and machine learning. Her current research focuses on enterprise search and scalable declarative text analytics for enterprise applications. She is the owner of several key components in the search engine that is currently powering IBM intranet search. She received her PhD degree in Computer Science and Engineering from the University of Michigan, Ann Arbor in 2007.
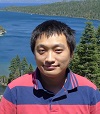
Bio: Ziyang Liu is a researcher at the Data Management department at NEC Laboratories America. His research interests span several topics in data management, including efficient and iterative big data analytics, data pricing, multitenant databases, data usability and effectively searching structured data with keywords. He got his Ph.D. from the School of Computing, Informatics, and Decision Systems Engineering at Arizona State University in 2011. He also received B.S. degree in computer engineering from Harbin Institute of Technology, China, in 2006.
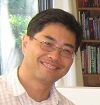
Bio: Huaiyu Zhu is with IBM Research—Almaden. He received his PhD degree in Computational Mathematics and Statistics from Liverpool University. His research interest includes statistical and machine learning techniques in data mining applications, especially in text analytics and large scale enterprise applications. In the past several years his main research focus was on enterprise search.
Demo 1
Location: Pearl
Chair: Demo 1 Chair


X-LiSA: Cross-lingual Semantic Annotation
Lei Zhang*, KIT
The ever-increasing quantities of structured knowledge on the Web and the impending need of multilinguality and cross-linguality for information access pose new challenges but at the same time open up new opportunities for knowledge extraction research. In this regard, cross-lingual semantic annotation has emerged as a topic of major interest and it is essential to build tools that can link words and phrases in unstructured text in one language to resources in structured knowledge bases in any other language. In this paper, we demonstrate X-LiSA, an infrastructure for cross-lingual semantic annotation, which supports both service-oriented and user-oriented interfaces for annotating text documents and web pages in different languages using resources from Wikipedia and Linked Open Data (LOD).


Combining Interaction, Speculative Query Execution and Sampling in the DICE System
Prasanth Jayachandran (The Ohio State University),Karthik Tunga (The Ohio State University),Niranjan Kamat* (The Ohio State University),Arnab Nandi (Ohio State University)
The interactive exploration of data cubes has become a popular application, especially over large datasets. In this paper, we present DICE, a combination of a novel frontend query interface and distributed aggregation backend that enables interactive cube exploration. DICE provides a convenient, practical alternative to the typical offline cube materialization strategy by allowing the user to explore facets of the data cube, trading off accuracy for interactive response-times, by sampling the data. We consider the time spent by the user perusing the results of their current query as an opportunity to execute and cache the most likely followup queries. The frontend presents a novel intuitive interface that allows for sampling-aware aggregations, and encourages interaction via our proposed faceted model. The design of our backend is tailored towards the low-latency user interaction at the frontend, and vice-versa. We discuss the synergistic design behind both the frontend user experience and the backend architecture of DICE; and, present a demonstration that allows the user to fluidly interact with billion-tuple datasets within sub-second interactive response times.


STMaker--A System to Make Sense of Trajectory Data
Han Su* (University of Queensland),Kai Zheng (University of Queensland),KAI ZENG (UCLA),Jiamin Huang (Nanjing University),xiaofang Zhou (University of Queensland)
Widely adoption of GPS-enabled devices generates large amounts of trajectories every day. The raw trajectory data describes the movement history of moving objects by a sequence of longitude, latitude, time-stamp triples, which are nonintuitive for human to perceive the prominent features of the trajectory, such as where and how the moving object travels. In this demo, we present the STMaker system to help users make sense of individual trajectories. Given a trajectory, STMaker can automatically extract the significant semantic behavior of the trajectory, and summarize the behavior by a short human-readable text. In this paper, we first introduce the phrases of generating trajectory summarizations, and then show several real trajectory summarization cases.


Interactive Join Query Inference with JIM
Angela Bonifati (University of Lille INRIA),Radu Ciucanu* (University of Lille INRIA),Slawek Staworko (University of Lille INRIA)
Specifying join predicates may become a cumbersome task in many situations e.g., when the relations to be joined come from disparate data sources, when the values of the attributes carry little or no knowledge of metadata, or simply when the user is unfamiliar with querying formalisms. Such task is recurrent in many traditional data management applications, such as data integration, constraint inference, and database denormalization, but it is also becoming pivotal in novel crowdsourcing applications. We present Jim (Join Inference Machine), a system for interactive join specification tasks, where the user infers an n-ary join predicate by selecting tuples that are part of the join result via Boolean membership queries. The user can label tuples as positive or negative, while the system allows to identify and gray out the uninformative tuples i.e., those that do not add any information to the final learning goal. The tool also guides the user to reach her join inference goal with a minimal number of interactions.


MESA: A Map Service to Support Fuzzy Type Ahead Search over Geo-Textual Data
Yuxin Zheng* (NUS),Zhifeng Bao (University of Tasmania),Lidan Shou (Zhejiang University),Anthony Tung (National University of Singapore)
Geo-textual data are ubiquitous these days. Recent study on spatial keyword search focused on the processing of queries which retrieve objects that match certain keywords within a spatial region. To ensure effective data retrieval, various extensions were done including the tolerance of errors in keyword matching and the search-as-you-type feature using prefix matching. We present MESA, a map application to support different variants of spatial keyword query. In this demonstration, we adopt the autocompletion paradigm that generates the initial query as a prefix matching query. If there are few matching results, other variants are performed as a form of relaxation that reuses the processing done in earlier phases. The types of relaxation allowed include spatial region expansion and exact/approximate prefix/substring matching. MESA adopts the client-server architecture. It provides fuzzy type-ahead search over geo-textual data. The core of MESA is to adopt a unifying search strategy, which incrementally applies the relaxation in an appropriate order to maximize the efficiency of query processing. In addition, MESA equips a user-friendly interface to interact with users and visualize results. MESA also provides customized search to meet the needs of different users.


R3: A Real-time Route Recommendation System
Henan Wang* (Tsinghua University),Guoliang Li (Tsinghua University),Hu Huiqi (Tsinghua University),Chen Shuo (Tsinghua University),Shen Bingwen (Tsinghua University),Wu Hao (SAP Labs),Wen-syan Li (SAP)
Existing route recommendation systems have two main weaknesses. First, they usually recommend the same route for all users and cannot help control traffic jam. Second, they do not take full advantage of real-time traffic to recommend the best routes. To address these two problems, we develop a real-time route recommendation system, called R3, aiming to provide users with the real-time-traffic-aware routes. R3 recommends diverse routes for different users to alleviate the traffic pressure. R3 utilizes historical taxi driving data and real-time traffic data and integrates them together to provide users with real-time route recommendation.


PDQ: Proof-driven Query Answering over Web-based Data
Michael Benedikt* (Oxford University),Julien Leblay (Oxford University),Efthymia Tsamoura (Oxford University)
The data needed to answer queries is often available through Web-based APIs. Indeed, for a given query there may be many Web-based sources which can be used to answer it, with the sources overlapping in their vocabularies, and differing in their access restrictions (required arguments) and cost. We introduce PDQ (Proof-Driven Query Answering), a system for determining a query plan in the presence of web-based sources. It is: constraint-aware -- exploiting relationships between sources to rewrite an expensive query into a cheaper one, access-aware -- abiding by any access restrictions known in the sources, and cost-aware -- making use of any cost information that is available about services. PDQ proceeds by generating query plans from proofs that a query is answerable. We demonstrate the use of PDQ and its effectiveness in generating low-cost plans.


Data In, Fact Out: Automated Monitoring of Facts by FactWatcher
Naeemul Hassan* (University of Texas at Arlington),Afroza Sultana (UNIVERSITY OF TEXAS AT ARLINGT),You Wu (Duke University),Gensheng Zhang (University of Texas at Arlington),Chengkai Li (The University of Texas at Arlington),Jun Yang (Duke University),Cong Yu (Google Research)
Towards computational journalism, we present FactWatcher, a system that helps journalists identify data-backed, attention-seizing facts which serve as leads to news stories. FactWatcher discovers three types of facts, including situational facts, one-of-the-few facts, and prominent streaks, through a unified suite of data model, algorithm framework, and fact ranking measure. Given an append-only database, upon the arrival of a new tuple, FactWatcher monitors if the tuple triggers any new facts. Its algorithms efficiently search for facts without exhaustively testing all possible ones. Furthermore, FactWatcher provides multiple features in striving for an end-to-end system, including fact ranking, fact-to-statement translation and keyword-based fact search.


OceanST: A Distributed Analytic System for Large-scale Spatiotemporal Mobile Broadband Data
Mingxuan Yuan (Noah's Ark Lab),Fei Wang (Huawei Noah's Ark Research Lab),Dongni Ren (Hong Kong University),Ke Deng* (Noah's Ark Research Lab),Jia Zeng (Noah's Ark Lab),Yanhua Li (HUAWEI Noah's Ark Lab),Bing Ni (Huawei Noah's Ark Research Lab),Xiuqiang He (Huawei Noah’s Ark Lab)
With the increasing prevalence of versatile mobile devices and the fast deployment of broadband mobile networks, a huge volume of Mobile Broadband (MBB) data has been generated over time. The MBB data naturally contain rich information of a large number of mobile users, covering a considerable fraction of whole population nowadays, including the mobile applications they are using at different locations and time; the MBB data may present the unprecedentedly large knowledge base of human behavior which has highly recognized commercial and social value. However, the storage, management and analysis of the huge and fast growing volume of MBB data post new and significant challenges to the industrial practitioners and research community. In this demonstration, we present a new, MBB data tailored, distributed analytic system named OceanST which has addressed a series of problems and weaknesses of the existing systems, originally designed for more general purpose and capable to handle MBB data to some extent. OceanST is featured by (i) efficiently loading of ever-growing MBB data, (ii) a bunch of spatiotemporal aggregate queries and basic analysis APIs frequently found in various MBB data application scenarios, and (iii) sampling-based approximate solution with provable accuracy bound to cope with huge volume of MBB data. The demonstration will show the advantage of OceanST in a cluster of 5 machines using 3TB data.
Thursday Sep 4th 15:30-17:00
Papers : Graph Data III: Distance Queries and Top-k Queries
Location: Diamond 1
Chair: Raymond Wong


On the Embeddability of Random Walk Distances
Xiaohan Zhao* (UCSB), Adelbert Chang (UCSB), Atish Das Sarma (eBay Research Labs), Haitao Zheng (UCSB), Ben Y. Zhao (UCSB)
Analysis of large graphs is critical to the ongoing growth of search engines and social networks. One class of queries centers around node affinity, often quantified by random-walk distances between node pairs, including hitting time, commute time, and personalized PageRank (PPR). Despite the potential of these "metrics,'' they are rarely, if ever, used in practice, largely due to extremely high computational costs. In this paper, we investigate methods to scalably and efficiently compute random-walk distances, by "embedding'' graphs and distances into points and distances in geometric coordinate spaces. We show that while existing graph coordinate systems (GCS) can accurately estimate shortest path distances, they produce significant errors when embedding random-walk distances. Based on our observations, we propose a new graph embedding system that explicitly accounts for per-node graph properties that affect random walk. Extensive experiments on a range of graphs show that our new approach can accurately estimate both symmetric and asymmetric random-walk distances. Once a graph is embedded, our system can answer queries between any two nodes in 8 microseconds, orders of magnitude faster than existing methods. Finally, we show that our system produces estimates that can replace ground truth in applications with minimal impact on application output.


A Partition-Based Approach to Structure Similarity Search
Xiang Zhao* (UNSW), Chuan Xiao (Nagoya University), Xuemin Lin (University of New South Wales), Qing Liu (CSIRO), Wenjie Zhang (The University of New South Wales)
Graphs are widely used to model complex data in many applications, such as bioinformatics, chemistry, social networks, pattern recognition, etc. A fundamental and critical query primitive is to efficiently search similar structures in a large collection of graphs. This paper studies the graph similarity queries with edit distance constraints. Existing solutions to the problem utilize fixed-size overlapping substructures to generate candidates, and thus become susceptible to large vertex degrees or large distance thresholds. In this paper, we present a partition-based approach to tackle the problem. By dividing data graphs into variable-size non-overlapping partitions, the edit distance constraint is converted to a graph containment constraint for candidate generation. We develop efficient query processing algorithms based on the new paradigm. A candidate pruning technique and an improved graph edit distance algorithm are also developed to further boost the performance. In addition, a cost-aware graph partitioning technique is devised to optimize the index. Extensive experiments demonstrate our approach significantly outperforms existing approaches.


Schemaless and Structureless Graph Querying
Shengqi Yang* (UCSB), Yinghui Wu (UCSB), Huan Sun (UCSB), Xifeng Yan (University of Santa Barbara)
Querying complex graph databases such as knowledge graphs is a challenging task for non-professional users. Due to their complex schemas and variational information descriptions, it becomes very hard for users to formulate a query that can be properly processed by the existing systems. We argue that for a user-friendly graph query engine, it must support various kinds of transformations such as synonym, abbreviation, and ontology. Furthermore, the derived query results must be ranked in a principled manner. In this paper, we introduce a novel framework enabling schemaless and structureless graph querying (SLQ), where a user need not describe queries precisely as required by most databases. The query engine is built on a set of transformation functions that automatically map keywords and linkages from a query to their matches in a graph. It automatically learns an effective ranking model, without assuming manually labeled training examples, and can efficiently return top ranked matches using graph sketch and belief propagation. The architecture of SLQ is elastic for ''plug-in'' new transformation functions and query logs. Our experimental results show that this new graph querying paradigm is promising: It identifies high-quality matches for both keyword and graph queries over real-life knowledge graphs, and outperforms existing methods significantly in terms of effectiveness and efficiency.


Diversified Top-k Graph Pattern Matching
Wenfei Fan (University of Edinburgh), Xin Wang* (University of Edinburgh), Yinghui Wu (UC Santa Barbara)
Graph pattern matching has been widely used in e.g., social data analysis. A number of matching algorithms have been developed that, given a graph pattern Q and a graph G, compute the set M(Q, G) of matches of Q in G. However, these algorithms often return an excessive number of matches, and are expensive on large real-life social graphs. Moreover, in practice many social queries are to find matches of a specific pattern node, rather than the entire M(Q, G). This paper studies top-k graph pattern matching. (1) We revise graph pattern matching defined in terms of simulation, by supporting a designated output node $u_o$. Given G and Q, it is to find those nodes in M(Q, G) that match $u_o$, instead of M(Q, G). (2) We propose two functions for ranking the matches: a relevance function $\delta_r()$ based on social impact, and a distance function $\delta_d()$ to cover diverse elements. (3) We develop two algorithms for computing top-k matches of $u_o$ based on $\delta_r()$, with the early termination property, i.e., they find top-k matches without computing the entire M(Q, G). (4) We also study diversified top-k matching, a bi-criteria optimization problem based on both $\delta_r()$ and $\delta_d()$. We show that its decision problem is NP-complete. Nonetheless, we provide an approximation algorithm with performance guarantees and a heuristic one with the early termination property. (5) Using real-life and synthetic data, we experimentally verify that our (diversified) top-k matching algorithms are effective, and outperform traditional matching algorithms in efficiency.


Top-K Structural Diversity Search in Large Networks
Xin Huang (The Chinese University of Hong Kong), Hong Cheng* (The Chinese University of Hong Kong), Rong-Hua Li (Guangdong Province Key Laboratory of Popular High Performance Computers), Lu Qin (The Chinese University of Hong Kong), Jeffrey Yu (Chinese University of Hong Kong)
Social contagion depicts a process of information (e.g., fads, opinions, news) diffusion in the online social networks. A recent study reports that in a social contagion process the probability of contagion is tightly controlled by the number of connected components in an individual's neighborhood. Such a number is termed structural diversity of an individual and it is shown to be a key predictor in the social contagion process. Based on this, a fundamental issue in a social network is to find top-k users with the highest structural diversities. In this paper, we, for the first time, study the top-k structural diversity search problem in a large network. Specifically, we develop an effective upper bound of structural diversity for pruning the search space. The upper bound can be incrementally refined in the search process. Based on such upper bound, we propose an efficient framework for top-k structural diversity search. To further speed up the structural diversity evaluation in the search process, several carefully devised heuristic search strategies are proposed. Extensive experimental studies are conducted in 13 real-world large networks, and the results demonstrate the efficiency and effectiveness of the proposed methods.
Papers : Query Processing II
Location: Diamond 2
Chair: Jayant Haritsa


Bounded Conjunctive Queries
Yang Cao* (University of Edinburgh), Wenfei Fan (University of Edinburgh), Wenyuan Yu (Facebook)
A query Q is said to be effectively bounded if for all datasets D, there exists a subset D_Q of D such that Q(D) = Q(D_Q), and the size of D_Q and time for fetching D_Q are independent of the size of D. The need for studying such queries is evident, since it allows us to compute Q(D) by accessing a bounded dataset D_Q, regardless of how big D is. This paper investigates effectively bounded conjunctive queries (SPC) under an access schema A, which specifies indices and cardinality constraints commonly used. We provide characterizations (sufficient and necessary conditions) for determining whether an SPC query Q is effectively bounded under A. We study several problems for deciding whether Q is bounded, and if not, for identifying a minimum set of parameters of Q to instantiate and make Q bounded. We show that these problems range from quadratic-time to NP-complete, and develop efficient (heuristic) algorithms for them. We also provide an algorithm that, given an effectively bounded SPC query Q and an access schema A, generates a query plan for evaluating Q by accessing a bounded amount of data in any (possibly big) dataset. We experimentally verify that our algorithms substantially reduce the cost of query evaluation.


Certain Query Answering in Partially Consistent Databases
Sergio Greco (University of Calabria), Fabian Pijcke (University of Mons), Jef Wijsen* (University of Mons)
A database is called uncertain if two or more tuples of the same relation are allowed to agree on their primary key. Intuitively, such tuples act as alternatives for each other. A repair (or possible world) of such uncertain database is obtained by selecting a maximal number of tuples without ever selecting two tuples of the same relation that agree on their primary key. For a Boolean query q, the problem CERTAINTY(q) takes as input an uncertain database db and asks whether q evaluates to true on every repair of db. In recent years, the complexity of CERTAINTY(q) has been studied under different restrictions on q. These complexity studies have assumed no restrictions on the uncertain databases that are input to CERTAINTY(q). In practice, however, it may be known that these input databases are partially consistent, in the sense that they satisfy some dependencies (e.g., functional dependencies). In this article, we introduce the problem CERTAINTY(q) in the presence of a set Σ of dependencies. The problem CERTAINTY(q,Σ) takes as input an uncertain database db that satisfies Σ, and asks whether every repair of db satisfies q. We focus on the complexity of CERTAINTY(q,Σ) when q is an acyclic conjunctive query without self-join, and Σ is a set of functional dependencies and join dependencies, the latter of a particular form. We provide an algorithm that, given q and Σ, decides whether CERTAINTY(q,Σ) is first-order expressible. Moreover, we show how to effectively construct a first-order definition of CERTAINTY(q,Σ) if it exists.


Computing k-Regret Minimizing Sets
Sean Chester* (University of Victoria), Alex Thomo (University of Victoria), S. Venkatesh (University of Victoria), Sue Whitesides (University of Victoria)
Regret minimizing sets are a recent approach to representing a dataset D by a small subset R of size r of representative data points. The set R is chosen such that executing any top-1 query on R rather than D is minimally perceptible to any user. However, such a subset R may not exist, even for modest sizes, r. In this paper, we introduce the relaxation to k-regret minimizing sets, whereby a top-1 query on R returns a result imperceptibly close to the top-k on D. We show that, in general, with or without the relaxation, this problem is NP-hard. For the specific case of two dimensions, we give an efficient dynamic programming, plane sweep algorithm based on geometric duality to find an optimal solution. For arbitrary dimension, we give an empirically effective, greedy, randomized algorithm based on linear programming. With these algorithms, we can find subsets R of much smaller size that better summarize D, using small values of k larger than 1.


Willingness Optimization for Social Group Activity
Hong-Han Shuai (NTUEE), De-Nian Yang* (Academia Sinica), Philip Yu (Univ. of Illinois at Chicago), Ming-Syan Chen (National Taiwan Univ.)
Studies show that a person is willing to join a social group activity if the activity is interesting, and some close friends will also join the activity as companions. The literature has demonstrated that the interests of a person and social tightness among friends can be effectively derived and mined from social networking websites. However, even with the above two information widely available, nowadays social group activities still need to be coordinated manually, and the process is tedious and time-consuming for users, especially for a large social group activity, due to complicated social connectivity and diversity of possible interests among friends. To address the above important need, this paper proposes to automatically select and recommend potential attendees of a social group activity, which could be very useful for social networking websites as a value-added service. We first formulate a new problem, named Willingness mAximization for Social grOup (WASO). This paper points out that the solution obtained by a greedy algorithm is likely to be trapped in a local optimal solution. Thus, we design a new randomized algorithm to effectively and efficiently solve the problem. Given the computational budgets available, the proposed algorithm is able to optimally allocate the resources and find a solution with an approximation ratio. We implement the proposed algorithm in Facebook, and the user study demonstrates that social groups obtained by the proposed algorithm implemented in Facebook significantly outperform the solutions manually configured by users.
Industrial : Big Data 1
Location: Diamond 3
Chair: Christoph Freytag


MRTuner: A Toolkit to Enable Holistic Optimization for MapReduce Jobs
Juwei Shi* (IBM Research China)*,Jia Zou (IBM Research-China),Jiaheng Lu (RUC),Zhao Cao (IBM Research China),Shi Qiang Li (IBM Research China),Chen Wang (IBM China Research Lab)
MapReduce based data-intensive computing solutions are increasingly deployed as production systems. Unlike Internet companies who invent and adopt the technology from the very beginning, traditional enterprises demand easy-to-use software due to the limited capabilities of administrators. Automatic job optimization software for MapReduce is a promising technique to satisfy such requirements. In this paper, we introduce a toolkit from IBM, called MRTuner, to enable holistic optimization for MapReduce jobs. In particular, we propose a novel Producer-Transporter-Consumer (PTC) model, which characterizes the tradeoffs in the parallel execution among tasks. We also carefully investigate the complicated relations among about twenty parameters, which have significant impact on the job performance. We design an efficient search algorithm to find the optimal execution plan. Finally, we conduct a thorough experimental evaluation on two different types of clusters using the HiBench suite which covers various Hadoop workloads from GB to TB size levels. The results show that the search latency of MRTuner is a few orders of magnitude faster than that of the state-of-the-art cost-based optimizer, and the effectiveness of the optimized execution plan is also significantly improved.


Large-Scale Graph Analytics in Aster 6: Bringing Context to Big Data Discovery
David Simmen* (Teradata Aster)*
Graph analytics is an important big data discovery technique. Applications include identifying influential employees for retention, detecting fraud in a complex interaction network, and determining product affinities by exploiting community buying patterns. Specialized platforms have emerged to satisfy the unique processing requirements of large-scale graph analytics; however, these platforms do not enable graph analytics to be combined with other analytics techniques, nor do they work well with the vast ecosystem of SQL-based business applications. Teradata Aster 6.0 adds support for large-scale graph analytics to its repertoire of analytics capabilities. The solution extends the multi-engine processing architecture with support for bulk synchronous parallel execution, and a specialized graph engine that enables iterative analysis of graph structures. Graph analytics functions written to the vertex-oriented API exposed by the graph engine can be invoked from the context of an SQL query and composed with existing SQL-MR functions, thereby enabling data scientists and business applications to express computations that combine large-scale graph analytics with techniques better suited to a different style of processing. The solution includes a suite of pre-built graph analytic functions adapted for parallel execution.


Summingbird: A Framework for Integrating Batch and Online MapReduce Computations
Oscar Boykin (Twitter),Sam Ritchie (Twitter),Ian O'Connell (Twitter),Jimmy Lin* (Twitter)*
Summingbird is an open-source domain-specific language implemented in Scala and designed to integrate online and batch MapReduce computations in a single framework. Summingbird programs are written using dataflow abstractions such as sources, sinks, and stores, and can run on different execution platforms: Hadoop for batch processing (via Scalding/Cascading) and Storm for online processing. Different execution modes require different bindings for the dataflow abstractions (e.g., HDFS files or message queues for the source) but do not require any changes to the program logic. Furthermore, Summingbird can operate in a hybrid processing mode that transparently integrates batch and online results to efficiently generate up-to-date aggregations over long time spans. The language was designed to improve developer productivity and address pain points in building analytics solutions at Twitter where often, the same code needs to be written twice (once for batch processing and again for online processing) and indefinitely maintained in parallel. Our key insight is that certain algebraic structures provide the theoretical foundation for integrating batch and online processing in a seamless fashion. This means that Summingbird imposes constraints on the types of aggregations that can be performed, although in practice we have not found these constraints to be overly restrictive for a broad range of analytics tasks at Twitter.


DGFIndex for Smart Grid: Enhancing Hive with a Cost-Effective Multidimensional Range Index
Liu Yue* (Chinese Academy of Sciences)*,Songlin Hu (Chinese Academy of Science),Tilmann Rabl (University of Toronto),Wantao Liu (Chinese Academy of Science),Hans-Arno Jacobsen (University of Toronto),Kaifeng Wu (State Grid Electricity Science Research Institute),Jian Chen (Zhejiang Electric Power Corporation)
In Smart Grid applications, as the number of deployed electric smart meters increases, massive amounts of valuable meter data is generated and collected every day. To enable reliable data collection and make business decisions fast, high throughput storage and high-performance analysis of massive meter data become crucial for grid companies. Considering the advantage of high efficiency, fault tolerance, and price-performance of Hadoop and Hive systems, they are frequently deployed as underlying platform for big data processing. However, in real business use cases, these data analysis applications typically involve multidimensional range queries (MDRQ) as well as batch reading and statistics on the meter data. While Hive is high-performance at complex data batch reading and analysis, it lacks efficient indexing techniques for MDRQ. In this paper, we propose DGFIndex, an index structure for Hive that efficiently supports MDRQ for massive meter data. DGFIndex divides the data space into cubes using the grid file technique. Unlike the existing indexes in Hive, which stores all combinations of multiple dimensions, DGFIndex only stores the information of cubes. This leads to smaller index size and faster query processing. Furthermore, with pre-computing user-defined aggregations of each cube, DGFIndex only needs to access the boundary region for aggregation query. Our comprehensive experiments show that DGFIndex can save significant disk space in comparison with the existing indexes in Hive and the query performance with DGFIndex is 2-50 times faster than existing indexes in Hive and HadoopDB for aggregation query, 2-5 times faster than both for non-aggregation query, 2-75 times faster than scanning the whole table in different query selectivity.
Papers : Transaction Processing
Location: Diamond 4
Chair: Sudipto Das


ConfluxDB: Multi-Master Replication for Partitioned Snapshot Isolation Databases
Prima Chairunnanda* (University of Waterloo), Khuzaima Daudjee (University of Waterloo), Tamer Ozsu (University of Waterloo)
Lazy replication with snapshot isolation (SI) has emerged as a popular choice for distributed databases. However, lazy replication often requires execution of update transactions at one (master) site so that it is relatively easy for a total SI order to be determined for consistent installation of updates in the lazily replicated system. We propose a set of techniques that support update transaction execution over multiple partitioned sites, thereby allowing the master to scale. Our techniques determine a total SI order for update transactions over multiple master sites without requiring global coordination in the distributed system, and ensure that updates are installed in this order at all sites to provide consistent and scalable replication with SI. We present ConfluxDB, a PostgreSQL-based implementation of our techniques, and demonstrate its effectiveness through experimental evaluation.


Accordion: Elastic Scalability for Database Systems Supporting Distributed Transactions
Marco Serafini* (Qatar Computing Research Insti), Essam Mansour (Qatar Computing Research Institute), Ashraf Aboulnaga (Qatar Computing Research Institute), Kenneth Salem (Univesity of Waterloo), Taha Rafiq (Amazon.com), Umar Farooq Minhas (IBM Almaden Research Center)
Providing the ability to elastically use more or fewer servers on demand (scale out and scale in) as the load varies is essential for database management systems (DBMSes) deployed on today's distributed computing platforms, such as the cloud. This requires solving the problem of dynamic (online) data placement, which has so far been addressed only for workloads where all transactions are local to one sever. In DBMSes where ACID transactions can access more than one partition, distributed transactions represent a major performance bottleneck. Scaling out and spreading data across a larger number of servers does not necessarily result in a linear increase in the overall system throughput, because transactions that used to access only one server may become distributed. In this paper we present Accordion, a dynamic data placement system for partition-based DBMSes that support ACID transactions (local or distributed). It does so by explicitly considering the affinity between partitions, which indicates the frequency in which they are accessed together by the same transactions. Accordion estimates the capacity of a server by explicitly considering the impact of distributed transactions and affinity on the maximum throughput of the server. It then integrates this estimation in a mixed-integer linear program to explore the space of possible configurations and decide whether to scale out. We implemented Accordion and evaluated it using H-Store, a shared-nothing in-memory DBMS. Our results using the TPC-C and YCSB benchmarks show that Accordion achieves benefits compared to alternative heuristics of up to an order of magnitude reduction in the number of servers used and in the amount of data migrated.


Highly Available Transactions: Virtues and Limitations
Peter Bailis* (UC Berkeley), Aaron Davidson (UC Berkeley), Alan Fekete (University of Sydney), Ali Ghodsi (UC Berkeley/KTH), Joseph Hellerstein (UC Berkeley), Ion Stoica (UC Berkeley)
To minimize network latency and remain online during server failures and network partitions, many modern distributed data storage systems eschew transactional functionality, which provides strong semantic guarantees for groups of multiple operations over multiple data items. In this work, we consider the problem of providing Highly Available Transactions (HATs): transactional guarantees that do not suffer unavailability during system partitions or incur high network latency. We introduce a taxonomy of highly available systems and analyze existing ACID isolation and distributed data consistency guarantees to identify which can and cannot be achieved in HAT systems. This unifies the literature on weak transactional isolation, replica consistency, and highly available systems. We analytically and experimentally quantify the availability and performance benefits of HATs---often two to three orders of magnitude over wide-area networks---and discuss their necessary semantic compromises.


An Evaluation of the Advantages and Disadvantages of Deterministic Database Systems
Kun Ren* (Northwestern Polytechnical Uni), Alexander Thomson (Google), Daniel Abadi (Yale University)
Recent proposals for deterministic database system designs argue that deterministic database systems facilitate replication since the same input can be independently sent to two different replicas without concern for replica divergence. In addition, they argue that determinism yields performance benefits due to (1) the introduction of deadlock avoidance techniques, (2) the reduction (or elimination) of distributed commit protocols, and (3) light-weight locking. However, these performance benefits are not universally applicable, and there exist several disadvantages of determinism, including (1) the additional overhead of processing transactions for which it is not known in advance what data will be accessed, (2) an inability to abort transactions arbitrarily (e.g., in the case of database or partition overload), and (3) the increased latency required by a preprocessing layer that ensures that the same input is sent to every replica. This paper presents a thorough experimental study that carefully investigates both the advantages and disadvantages of determinism, in order to give a database user a more complete understanding of which database to use for a given database workload and cluster configuration.


MaaT: Effective and scalable coordination of distributed transactions in the cloud
Hatem Mahmoud (UC Santa Barbara), Vaibhav Arora* (UCSB), Faisal Nawab (UCSB), Divyakant Agrawal), Amr El Abbadi),)
The past decade has witnessed an increasing adoption of cloud database technology, which provides better scalability, availability, and fault-tolerance via transparent partitioning and replication, and automatic load balacing and failover. However, only a small number of cloud databases provide strong consistency guarantees for distributed transactions, despite decades of research on distributed transaction processing, due to practical challenges that arise in the cloud setting, where failures are the norm, and human administration is minimal. For example, dealing with locks left by transactions initiated by failed machines, and determining a multi-programming level that avoids thrashing without underutilizing available resources, are some of the challenges that arise when using lock-based transaction processing mechanisms in the cloud context. Even in the case of optimistic concurrency control, most proposals in the literature deal with distributed validation but still require the database to acquire locks during two-phase commit when installing updates of a single transaction on multiple machines. Very little theoretical work has been done to entirely eliminate the need for locking in distributed transactions, including locks acquired during two-phase commit. In this paper, we re-design optimistic concurrency control to eliminate any need for locking even for atomic commitment, while handling the practical issues in earlier theoretical work related to this problem. We conduct an extensive experimental study to evaluate our approach against lock-based methods under various setups and workloads, and demonstrate that our approach provides many practical advantages in the cloud context.
Papers : Temporal and Stream Data
Location: Diamond 5
Chair: Yoshi Ishikawa


Differentially Private Event Sequences over Infinite Streams
Georgios Kellaris (HKUST), Stavros Papadopoulos* (Intel Labs & MIT), Xiaokui Xiao (NTU), Dimitris Papadias (HKUST)
Numerous applications require continuous publication of statistics for monitoring purposes, such as real-time traffic analysis, timely disease outbreak discovery, and social trends observation. These statistics may be derived from sensitive user data and, hence, necessitate privacy preservation. A notable paradigm for offering strong privacy guarantees in statistics publishing is e-differential privacy. However, there is limited literature that adapts this concept to settings where the statistics are computed over an infinite stream of "events" (i.e., data items generated by the users), and published periodically. These works aim at hiding a single event over the entire stream. We argue that, in most practical scenarios, sensitive information is revealed from multiple events occurring at contiguous time instances. Towards this end, we put forth the novel notion of w-event privacy over infinite streams, which protects any event sequence occurring in w successive time instants. We first formulate our privacy concept, motivate its importance, and introduce a methodology for achieving it. We next design two instantiations, whose utility is independent of the stream length. Finally, we confirm the practicality of our solutions experimenting with real data.


Discovering Longest-lasting Correlation in Sequence Databases
Yuhong Li (University of macau), Leong Hou U* (University of Macau), Man Lung Yiu (Hong Kong Polytechnic University), Zhiguo Gong (University of Macau)
Most existing work on sequence databases use correlation (e.g., {\it Euclidean distance} and {\it Pearson correlation}) as a core function for various analytical tasks. Typically, it requires users to set a length for the similarity queries. However, there is no steady way to define the proper length on different application needs. In this work we focus on discovering longest-lasting highly correlated subsequences in sequence databases, which is particularly useful in helping those analyses without prior knowledge about the query length. Surprisingly, there has been limited work on this problem. A baseline solution is to calculate the correlations for every possible subsequence combination. Obviously, the brute force solution is not scalable for large datasets. In this work we study a space-constrained index that gives a tight correlation bound for subsequences of similar length and offset by intra-object grouping and inter-object grouping techniques. To the best of our knowledge, this is the first index to support normalized distance metric of arbitrary length subsequences. Extensive experimental evaluation on both real and synthetic sequence datasets verifies the efficiency and effectiveness of our proposed methods.


A Temporal-Probabilistic Database Model for Information Extraction
Maximilian Dylla (Max Planck Institut Informatik), Iris Miliaraki* (Max Planck Institut Informatik), Martin Theobald (University of Antwerp)
Temporal annotations of facts are a key component both for building a high-accuracy knowledge base and for answering queries over the resulting temporal knowledge base with high precision and recall. In this paper, we present a temporal-probabilistic database model for cleaning uncertain temporal facts obtained from information extraction methods. Specifically, we consider a combination of temporal deduction rules, temporal consistency constraints and probabilistic inference based on the common possible-worlds semantics with data lineage, and we study the theoretical properties of this data model. We further develop a query engine which is capable of scaling to very large temporal knowledge bases, with nearly interactive query response times over millions of uncertain facts and hundreds of thousands of grounded rules. Our experiments over two real-world datasets demonstrate the increased robustness of our approach compared to related techniques based on constraint solving via Integer Linear Programming (ILP) and probabilistic inference via Markov Logic Networks (MLNs). We are also able to show that our runtime performance is more than competitive to current ILP solvers and the fastest available, probabilistic but non-temporal, database engines.


High Performance Stream Query Processing With Correlation-Aware Partitioning
Lei Cao* (WPI), Elke Rundensteiner (WPI)
State-of-the-art optimizers produce one single optimal query plan for all stream data, in spite of such a singleton plan typically being sub-optimal or even poor for highly correlated data. Recently a new stream processing paradigm, called multi-route approach, has emerged as a promising approach for tackling this problem. Multi-route first divides data streams into several partitions and then creates a separate query plan for each combination of partitions. Unfortunately current approaches suffer from severe shortcomings, in particular, the lack of an effective partitioning strategy and the prohibitive query optimization expense. In this work we propose the first practical multi-route optimizer named \textit{\underline{c}orrelation-aware \underline{m}ulti-\underline{r}oute stream query optimizer} (or CMR) that solves both problems. By exploiting both intra- and inter-stream correlations of streams, CMR produces effective partitions without having to undertake repeated expensive query plan generation. The produced partitions not only are best served by distinct optimal query plans, but also leverage the partition-driven pruning opportunity. Experimental results with both synthetic and real life stream data confirm that CMR outperforms the state-of-the-art solutions up to an order of magnitude in both the query optimization time and the run-time execution performance.


Continuous Matrix Approximation on Distributed Data
Mina Ghashami* (University of Utah), Jeff Phillips (University of Utah), Feifei Li (University of Utah)
Tracking and approximating data matrices in streaming fashion is a fundamental challenge. The problem requires more care and attention when data comes from multiple distributed sites, each receiving a stream of data. This paper considers the problem of "tracking approximations to a matrix" in the distributed streaming model. In this model, there are $m$ distributed sites each observing a distinct stream of data (where each element is a row of a distributed matrix) and has a communication channel with a coordinator, and the goal is to track an $\epsilon$-approximation to the norm of the matrix along any direction. To that end, we present novel algorithms to address the matrix approximation problem. Our algorithms maintain a smaller matrix $B$, as an approximation to a distributed streaming matrix $A$, such that for any unit vector $x$: $| \|A x\|^2 - \|B x\|^2 | \leq \epsilon \|A\|_F^2$. Our algorithms work in streaming fashion and incur small communication, which is critical for distributed computation. Our best method is deterministic and uses only $O((m/\epsilon) \log(\beta N))$ communication, where $N$ is the size of stream (at the time of the query) and $\beta$ is an upper-bound on the squared norm of any row of the matrix. In addition to proving all algorithmic properties theoretically, extensive experiments with real large datasets demonstrate the efficiency of these protocols.
Tutorial : Uncertain Entity Resolution
Location: Bauhinia 1
Chair: Tutorial 5 Chair


Uncertain Entity Resolution
Avigdor Gal
Entity resolution is a fundamental problem in data integration dealing with the combination of data from different sources to a unified view of the data. Entity resolution is inherently an uncertain process because the decision to map a set of records to the same entity cannot be made with certainty unless these are identical in all of their attributes or have a common key. In the light of recent advancement in data accumulation, management, and analytics landscape (known as big data) the tutorial re-evaluates the entity resolution process and in particular looks at best ways to handle data veracity. The tutorial ties entity resolution with recent advances in probabilistic database research, focusing on sources of uncertainty in the entity resolution process.
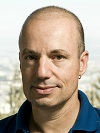
Bio: Avigdor Gal is an associate professor at Faculty of Industrial Engineering & Management, Technion. He is an expert on information systems. His research focuses on effective methods of integrating data from multiple and diverse sources, which affect the way businesses and consumers seek information over the Internet. His current work zeroes in on data integration-the task of providing communication between databases, and connecting such communication to real-world concepts. Another line of research involves the identification of complex events such as flu epidemics, biological attacks, and breaches in computer security, and its application to disaster and crisis management. He has applied his research to European and American projects in government, eHealth, and the integration of business documents. Prof. Gal has published more than 100 papers in leading professional journals (e.g. Journal of the ACM (JACM), ACM Transactions on Database Systems (TODS), IEEE Transactions on Knowledge and Data Engineering (TKDE), ACM Transactions on Internet Technology (TOIT), and the VLDB Journal) and conferences (ICDE, BPM, DEBS, ER, CoopIS) and books (Schema Matching and Mapping). He authored the book Uncertain schema Matching in 2011, serves in various editorial capacities for periodicals including the Journal on Data Semantics (JoDS), Encyclopedia of Database Systems and Computing, and has helped organize professional workshops and conferences nearly every year since 1998. He has won the IBM Faculty Award each year from 2002-2004, several Technion awards for teaching, the 2011-13 Technion-Microsoft Electronic Commerce Research Award, and the 2012 Yanai Award for Excellence in Academic Education, and others.
Demo 4
Location: Pearl
Chair: Demo 4 Chair


SPIRE: Supporting Parameter-Driven Interactive Rule Mining and Exploration
Xika Lin* (Worcester Polytechnic Institut),Abhishek Mukherji (Worcester Polytechnic Institute),Elke Rundensteiner (Worcester Polytechnic Institute),Matthew Ward (Worcester Polytechnic Institute)
We demonstrate our SPIRE technology for supporting interactive mining of both positive and negative rules at the speed of thought. It is often misleading to learn only about positive rules, yet extremely revealing to find strongly supported negative rules. Key technical contributions of SPIRE including region-wise abstractions of rules, positive-negative rule relationship analysis, rule redundancy management and rule visualization supporting novel exploratory queries will be showcased. The audience can interactively explore complex rule relationships in a visual manner, such as comparing negative rules with their positive counterparts, that would otherwise take prohibitive time. Overall, our SPIRE system provides data analysts with rich insights into rules and rule relationships while significantly reducing manual effort and time investment required.


An Integrated Development Environment for Faster Feature Engineering
Michael Cafarella (University of Michigan),Michael Anderson* (University of Michigan),Yixing Jiang (University of Michigan),Guan Wang (University of Michigan),Bochun Zhang (University of Michigan)
The application of machine learning to large datasets has become a core component of many important and exciting software systems being built today. The extreme value in these trained systems is tempered, however, by the difficulty of constructing them. As shown by the experience of Google, Netflix, IBM, and many others, a critical problem in building trained systems is that of feature engineering. High-quality machine learning features are crucial for the system’s performance but are difficult and time-consuming for engineers to develop. Data-centric developer tools that improve the productivity of feature engineers will thus likely have a large impact on an important area of work. We have built a demonstration integrated development environment for feature engineers. It accelerates one particular step in the feature engineering development cycle: evaluating the effectiveness of novel feature code. In particular, it uses an index and runtime execution planner to process raw data objects (e.g., Web pages) in order of descending likelihood that the data object will be relevant to the user’s feature code. This demonstration IDE allows the user to write arbitrary feature code, evaluate its impact on learner quality, and observe exactly how much faster our technique performs compared to a baseline system.


Pronto: A Software-Defined Networking based System for Performance Management of Analytical Queries on Distributed Data Stores
Pengcheng Xiong* (NEC Labs),Hakan Hacigumus (NEC Labs)
Nowadays data analytics applications are accessing more and more data from distributed data stores, creating large amount of data traffic on the network. Therefore, distributed analytic queries are prone to suffer from bad performance in terms of query execution time when they encounter a network resource contention, which is quite common in a shared network. Typical distributed query optimizers do not have a way to solve this problem because historically they have been treating the network underneath as a black-box: they are unable to monitor it, let alone to control it. However, we are entering a new era of software-defined networking (SDN), which provides visibility into and control of the network's state for the applications including distributed database systems. In this demonstration, we present a system, called Pronto that leverages the SDN capabilities for a distributed query processor to achieve performance improvement and differentiation for analytical queries. The system is the real implementation of our recently developed methods on commercial SDN products. The demonstration shows the shortcomings of a distributed query optimizer, which treats the underlying network as a black box, and the advantages of the SDN-based approach by allowing the users to selectively explore various relevant and interesting settings in a distributed query processing environment.


Getting Your Big Data Priorities Straight: A Demonstration of Priority-based QoS using Social-network-driven Stock Recommendation
Rui Zhang* (IBM Almaden),Reshu Jain (IBM Research - Almaden),Prasenjit Sarkar (IBM Research - Almaden)
As we come to terms with various big data challenges, one vital issue remains largely untouched. That is the optimal multiplexing and prioritization of different big data applications sharing the same underlying infrastructure, for example, a public cloud platform. Given these demanding applications and the necessary practice to avoid over-provisioning, resource contention between applications is inevitable. Priority must be given to important applications (or sub workloads in an application) in these circumstances. This demo highlights the compelling impact prioritization could make, using an example application that recommends promising combinations of stocks to purchase based on relevant Twitter sentiment. The application consists of a batch job and an interactive query, ran simultaneously. Our underlying solution provides a unique capability to identify and differentiate application workloads throughout a complex big data platform. Its current implementation is based on Apache Hadoop and the IBM GPFS distributed storage system. The demo showcases the superior interactive query performance achievable by prioritizing its workloads and thereby avoiding I/O bandwidth contention. The query time is 3.6 x better compared to no prioritization. Such a performance is within 0.3% of that of an idealistic system where the query runs without contention. The demo is conducted on around 3 months of Twitter data, pertinent to the S & P 100 index, with about 4 x 10^12 potential stock combinations considered.


Vertexica: Your Relational Friend for Graph Analytics!
Alekh Jindal* (MIT),Praynaa Rawlani (MIT),Samuel Madden (MIT CSAIL)
In this paper, we present Vertexica, a graph analytics tools on top of a relational database, which is user friendly and yet highly efficient. Instead of constraining programmers to SQL, Vertexica offers a popular vertex-centric query interface, which is more natural for analysts to express many graph queries. The programmers simply provide their vertex-compute functions and Vertexica takes care of efficiently executing them in the standard SQL engine. The advantage of using Vertexica is its ability to leverage the relational features and enable much more sophisticated graph analysis. These include expressing graph algorithms which are difficult in vertex-centric but straightforward in SQL and the ability to compose end-to-end data processing pipelines, including pre- and post- processing of graphs as well as combining multiple algorithms for deeper insights. Vertexica has a graphical user interface and we outline several demonstration scenarios including, interactive graph analysis, complex graph analysis, and continuous and time series analysis.


NScale: Neighborhood-centric Analytics on Large Graphs
Abdul Quamar* (University of Maryland),Amol Deshpande (University of Maryland),Jimmy Lin (University of Maryland)
There is an increasing interest in executing rich and complex analysis tasks over large-scale graphs, many of which require processing and reasoning about a large number of multi-hop neighborhoods or subgraphs in the graph. Examples of such tasks include ego network analysis, motif counting in biological networks, finding social circles, personalized recommendations, link prediction, anomaly de- tection, analyzing influence cascades, and so on. These tasks are not well served by existing vertex-centric graph processing frameworks whose computation and execution models limit the user program to directly access the state of a single vertex, resulting in high communication, scheduling, and memory overheads in executing such tasks. Further, most existing graph processing frameworks also typically ignore the challenges in extracting the relevant portions of the graph that an analysis task is interested in, and loading it onto distributed memory. In this demonstration proposal, we describe NSCALE, a novel end-to-end graph processing framework that enables the distributed execution of complex neighborhood-centric analytics over large- scale graphs in the cloud. NSCALE enables users to write programs at the level of neighborhoods or subgraphs. NSCALE uses Apache YARN for efficient and fault-tolerant distribution of data and computation; it features GEL, a novel graph extraction and loading phase, that extracts the relevant portions of the graph and loads them into distributed memory using as few machines as possible. NSCALE utilizes novel techniques for the distributed execution of user computation that minimize memory consumption by exploit- ing overlap among the neighborhoods of interest. A comprehensive experimental evaluation shows orders-of-magnitude improvements in performance and total cost over vertex-centric approaches.


DPSynthesizer: Differentially Private Data Synthesizer for Privacy Preserving Data Sharing
Haoran Li* (Emory University),Li Xiong (Emory University),Xiaoqian Jiang (UC San Diego),Lifan Zhang (Emory University)
Differential privacy has recently emerged in private statistical data release as one of the strongest privacy guarantees. However, to this date there is no open-source tools for releasing synthetic data in place of the original data under differential privacy. We propose DPSynthesizer, a toolkit for differentially private data synthesization. The core of DPSynthesizer is DPCopula which is designed for high-dimensional data. DPCopula computes a differentially private copula function from which we can sample synthetic data. Copula functions are used to describe the dependence between multivariate random vectors and allow us to build the multivariate joint distribution using one-dimensional marginal distributions. DPSynthesizer also implements a set of state-of-the-art methods for building differentially private histograms from which synthetic data can be generated. We will demonstrate the system using DPCopula as well as other methods with various data sets, showing the feasibility, utility, efficiency of various methods.


SPOT: Locating Social Media Users Based on Social Network Context
Zhi Liu* (University of North Texas),Yan Huang (University of North Texas),Longbo Kong (University of North Texas)
A tremendous amount of information is being shared everyday on social media sites such as Facebook, Twitter or Google+. But only a small portion of users provide their location information, which can be helpful in targeted advertisement and many other services. In this demo we present our large scale user location estimation system, SPOT, which showcase different location estimating models on real world data sets. The demo shows three different location estimation algorithms: a friend-based, a social closeness-based, and an energy and local social coefficient based. The first algorithm is a baseline and the other two new algorithms utilize social closeness information which was traditionally treated as a binary friendship. The two algorithms are based on the premise that friends are different and close friends can help to estimate location better. The demo will also show that all three algorithms benefit from a confidence-based iteration method. The demo is web-based. A user can specify different settings, explore the estimation results on a map, and observe the statistical information, e.g. accuracy and average friends used in the estimation, dynamically. The demo provides two datasets: Twitter (148,860 located users) and Gowalla (99,563 located users). Furthermore, a user can filter users with certain features, e.g. with more than 100 friends, to see how the estimating models work on a particular case. The estimated and real locations of those users as well as their friends will be displayed on the map.


RASP-QS: Efficient and Confidential Query Services in the Cloud
Zohreh Alavi (Wright State University),James Powers (Wright State University),Jiayue Wang (Wright State University),Keke Chen* (Wright State University)
Hosting data query services in public clouds is an attractive solution for its great scalability and significant cost savings. However, data owners also have concerns on data privacy due to the lost control of the infrastructure. This demonstration shows a prototype for efficient and confidential range/kNN query services built on top of the random space perturbation (RASP) method. The RASP approach provides a privacy guarantee practical to the setting of cloud-based computing, while enabling much faster query processing compared to the encryption-based approach. This demonstration will allow users to more intuitively understand the technical merits of the RASP approach via interactive exploration of the visual interface.


Thoth: Towards Managing a Multi-System Cluster
Mayuresh Kunjir* (Duke University),Prajakta Kalmegh (Duke University),Shivnath Babu (Duke University)
Following the `no one size fits all' philosophy, active research in big data platforms is focusing on creating an environment for multiple `one-size' systems to co-exist and co-operate in the same cluster. Consequently, it has now become imperative to provide an \textit{integrated management} solution that provides a database-centric view of the underlying multi-system environment. We outline the proposal of DBMS+, a database management platform over multiple `one-size' systems. Our prototype implementation of DBMS+, called Thoth, adaptively chooses a \textit{best-fit} system based on application requirements. In this demonstration, we propose to showcase Thoth DM, a data management framework for Thoth which consists of a data collection pipeline utility, data consolidation and dispatcher module, and a warehouse for storing this data. We further introduce the notion of apps; an app is a utility that registers with Thoth and interfaces with its warehouse to provide core database management functionalities like dynamic provisioning of resources, designing a multi-system-aware optimizer, tuning of configuration parameters on each system, data storage, and layout schemes. We will demonstrate Thoth in action over Hive, Hadoop, Shark, Spark, and the Hadoop Distributed File System. This demonstration will focus on the following apps: (i) Dashboard for administration and control that will let the audience monitor and visualize a database-centric view of the multi-system cluster, and (ii) Data Layout Recommender apps will allow searching for the optimal data layout in the multi-system setting.
Friday Sep 5th 09:00-18:00
BPOE: Big data benchmarks, Performance Optimization, and Emerging hardware
Location: Diamond 1
Chair: Jianfeng Zhan

Fifth workshop on Big Data Benchmarks, Performance Optimization, and Emerging Hardware (BPOE-5)
Jianfeng Zhan, Chinese Academy of Sciences
PDA@IOT: Workshop on Personal Data Analytics in the Internet of Things
Location: Diamond 2
Chair: Vassilis Christophides, R&I Paris Center, Technicolor, France Themis Palpanas, Paris Descartes University, France

Workshop on Personal Data Analytics in the Internet of Things (PDA@IOT 2014)
CHRISTOPHIDES Vassilis (ICS-FORTH Greece),Themis Palpanas (University of Trento),)
BeRSys & SSW: Benchmarking RDF Systems & Semantic Search Over The Web
Location: Diamond 3
Chair: Irini Fundulaki,Ioan Toma,Ioana Manolescu & Roberto De Virgilio

2nd International Workshop on Benchmarking RDF Systems (BeRSys 2014)
Irini Fundulaki (ICS-FORTH Greece),Ioan Toma (University of Innsbruck),Ioana Manolescu (Inria Saclay France)

4th international workshop on semantic search over the web (SSW 2014)
Roberto De Virgilio, Yahoo
TPCTC: TPC Technology Conference on Performance Evaluation & Benchmarking
Location: Diamond 5
Chair: Raghunath Nambiar, Cisco, USA;Meikel Poess, Oracle, USA;

Sixth TPC Technology Conference on Performance Evaluation & Benchmarking (TPCTC 2014)
Raghunath Nambiar, CISCO