![]() |
![]() |
![]() |
![]() |
Monday Aug 31st 08:00-09:00
Light Breakfast
Location: Grand Promenade
Monday Aug 31st 09:00-10:30
Big-O(Q) Session 1
Location: Kings 1

Workshop on Big-Graphs Online Querying (Big-O(Q) 2015)
Arijit Khan (ETH Zurich), Prasenjit Mitra (Qatar Computing Research Institute), Cong Yu (Google Research)
IMDM Session 1
Location: Kings 2

Third International Workshop on In-Memory Data Management and Analytics (IMDM 2015)
Justin Levandoski (Microsoft Research), Andy Pavlo (Carnegie Mellon University), Arun Jagatheesan (Samsung R&D Center), Thomas Neumann (Technische Universität München)
ADMS Session 1
Location: Kings 3

Sixth International Workshop on Accelerating Data Management Systems Using Modern Processor and Storage Architectures (ADMS 2015)
Rajesh Bordawekar (IBM T.J. Watson Research Center), Buğra Gedik (Bilkent University), Tirthankar Lahiri (Oracle), Christian A. Lang (Acelot Inc)
TPCTC Session 1
Location: Queens 4

Seventh TPC Technology Conference on Performance Evaluation & Benchmarking (TPCTC 2015)
Raghunath Nambiar (CISCO), Meikel Poess (Oracle)
BIRTE Session 1
Location: Queens 5

9th International Workshop on Business Intelligence for the Real Time Enterprise (BIRTE 2015)
Meichun Hsu (Hewlett-Packard), Malu Castellanos (Hewlett-Packard), Panos K Chrysanthis (University of Pittsburgh)
PhD Workshop Session 1
Location: Queens 6

PhD Workshop
Jennie Duggan (Northwestern University), Rachel Pottinger (University of British Columbia)
Monday Aug 31st 10:30-11:00
Refreshments: Coffee Break
Location: Grand Promenade
Monday Aug 31st 11:00-12:30
Big-O(Q) Session 2
Location: Kings 1

Workshop on Big-Graphs Online Querying (Big-O(Q) 2015)
Arijit Khan (ETH Zurich), Prasenjit Mitra (Qatar Computing Research Institute), Cong Yu (Google Research)
IMDM Session 2
Location: Kings 2

Third International Workshop on In-Memory Data Management and Analytics (IMDM 2015)
Justin Levandoski (Microsoft Research), Andy Pavlo (Carnegie Mellon University), Arun Jagatheesan (Samsung R&D Center), Thomas Neumann (Technische Universität München)
ADMS Session 2
Location: Kings 3

Sixth International Workshop on Accelerating Data Management Systems Using Modern Processor and Storage Architectures (ADMS 2015)
Rajesh Bordawekar (IBM T.J. Watson Research Center), Buğra Gedik (Bilkent University), Tirthankar Lahiri (Oracle), Christian A. Lang (Acelot Inc)
TPCTC Session 2
Location: Queens 4

Seventh TPC Technology Conference on Performance Evaluation & Benchmarking (TPCTC 2015)
Raghunath Nambiar (CISCO), Meikel Poess (Oracle)
BIRTE Session 2
Location: Queens 5

9th International Workshop on Business Intelligence for the Real Time Enterprise (BIRTE 2015)
Meichun Hsu (Hewlett-Packard), Malu Castellanos (Hewlett-Packard), Panos K Chrysanthis (University of Pittsburgh)
PhD Workshop Session 2
Location: Queens 6

PhD Workshop
Jennie Duggan (Northwestern University), Rachel Pottinger (University of British Columbia)
Monday Aug 31st 12:30-14:00
Lunch
Location: Lagoon Lanai
Monday Aug 31st 14:00-15:00
Big-O(Q) Session 3
Location: Kings 1

Workshop on Big-Graphs Online Querying (Big-O(Q) 2015)
Arijit Khan (ETH Zurich), Prasenjit Mitra (Qatar Computing Research Institute), Cong Yu (Google Research)
IMDM Session 3
Location: Kings 2

Third International Workshop on In-Memory Data Management and Analytics (IMDM 2015)
Justin Levandoski (Microsoft Research), Andy Pavlo (Carnegie Mellon University), Arun Jagatheesan (Samsung R&D Center), Thomas Neumann (Technische Universität München)
ADMS Session 3
Location: Kings 3

Sixth International Workshop on Accelerating Data Management Systems Using Modern Processor and Storage Architectures (ADMS 2015)
Rajesh Bordawekar (IBM T.J. Watson Research Center), Buğra Gedik (Bilkent University), Tirthankar Lahiri (Oracle), Christian A. Lang (Acelot Inc)
TPCTC Session 3
Location: Queens 4

Seventh TPC Technology Conference on Performance Evaluation & Benchmarking (TPCTC 2015)
Raghunath Nambiar (CISCO), Meikel Poess (Oracle)
BIRTE Session 3
Location: Queens 5

9th International Workshop on Business Intelligence for the Real Time Enterprise (BIRTE 2015)
Meichun Hsu (Hewlett-Packard), Malu Castellanos (Hewlett-Packard), Panos K Chrysanthis (University of Pittsburgh)
PhD Workshop Session 3
Location: Queens 6

PhD Workshop
Jennie Duggan (Northwestern University), Rachel Pottinger (University of British Columbia)
Monday Aug 31st 15:00-15:30
Refreshments: Coffee Break
Location: Grand Promenade
Monday Aug 31st 15:30-18:00
Big-O(Q) Session 4
Location: Kings 1

Workshop on Big-Graphs Online Querying (Big-O(Q) 2015)
Arijit Khan (ETH Zurich), Prasenjit Mitra (Qatar Computing Research Institute), Cong Yu (Google Research)
IMDM Session 4
Location: Kings 2

Third International Workshop on In-Memory Data Management and Analytics (IMDM 2015)
Justin Levandoski (Microsoft Research), Andy Pavlo (Carnegie Mellon University), Arun Jagatheesan (Samsung R&D Center), Thomas Neumann (Technische Universität München)
ADMS Session 4
Location: Kings 3

Sixth International Workshop on Accelerating Data Management Systems Using Modern Processor and Storage Architectures (ADMS 2015)
Rajesh Bordawekar (IBM T.J. Watson Research Center), Buğra Gedik (Bilkent University), Tirthankar Lahiri (Oracle), Christian A. Lang (Acelot Inc)
TPCTC Session 4
Location: Queens 4

Seventh TPC Technology Conference on Performance Evaluation & Benchmarking (TPCTC 2015)
Raghunath Nambiar (CISCO), Meikel Poess (Oracle)
BIRTE Session 4
Location: Queens 5

9th International Workshop on Business Intelligence for the Real Time Enterprise (BIRTE 2015)
Meichun Hsu (Hewlett-Packard), Malu Castellanos (Hewlett-Packard), Panos K Chrysanthis (University of Pittsburgh)
PhD Workshop Session 4
Location: Queens 6

PhD Workshop
Jennie Duggan (Northwestern University), Rachel Pottinger (University of British Columbia)
Monday Aug 31st 19:00-21:00
Welcome Buffet Reception
Location: Lagoon Lanai
Chair: Sam Madden
Tuesday Sep 1st 07:30-08:30
Light Breakfast
Location: Grand Promenade
Tuesday Sep 1st 08:30-10:15
Industrial Keynote: Juan Loaiza;Academic Keynote: Anastasia Ailamaki
Location: Monarchy Ballroom
Chair: Volker Markl


Engineering Database Hardware and Software Together
Juan Loaiza, Oracle
Since its inception, Oracle’s database software primarily ran on customer configured off-the-shelf hardware. A decade ago, the architecture of conventional systems started to become a bottleneck and Oracle developed the Oracle Exadata Database Machine to optimize the full hardware and software stack for database workloads. Exadata is based on a scale-out architecture of database servers and storage servers that optimizes both OLTP and Analytic workloads while hosting hundreds of databases simultaneously on the same system. By using database specific protocols for storage and networking we bypass limitations imposed by conventional network and storage layers. Exadata is now deployed at thousands of Enterprises including 4 of the 5 largest banks, telecoms, and retailers for varied workloads such as inter-bank funds transfers, e-commerce, ERP, Cloud SaaS applications, and petabyte data warehouses. Five years ago, Oracle initiated a project to extend our database stack beyond software and systems and into the architecture of the microprocessor itself. The goal of this effort is to dramatically improve the performance, reliability and cost effectiveness of a new generation of database machines. The new SPARC M7 processor is the first step. The M7 is an extraordinarily fast conventional processor with 32-cores per socket and an extremely high bandwidth memory system. Added to it’s conventional processing capabilities are 32 custom on-chip database co-processors that run database searches at full memory bandwidth rates, and decompress data in real-time to increase memory bandwidth and capacity. Further, the M7 implements innovative fine-grained memory protection to secure sensitive business data. In the presentation we will describe how Oracle’s engineering teams integrate software and hardware at all levels to achieve breakthrough performance, reliability, and security for the database and rest of the modern data processing stack.
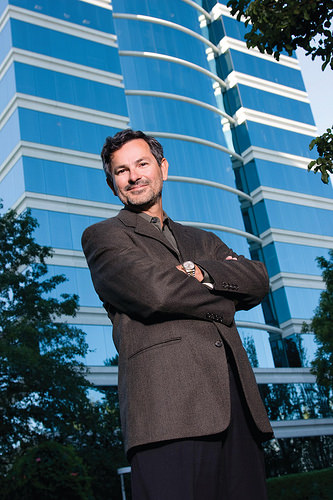
Bio: As Senior Vice President of Systems Technology at Oracle, Juan Loaiza is in charge of developing the mission-critical capabilities of Oracle Database, including data and transaction management, high availability, performance, in-memory processing, enterprise replication, and Oracle Exadata. Mr. Loaiza joined the Oracle Database development organization in 1988. Mr. Loaiza holds BS and MS degrees in computer science from the Massachusetts Institute of Technology.


Databases and Hardware: The Beginning and Sequel of a Beautiful Friendship
Anastasia Ailamaki, EPFL
Top-level performance has been the target of 40 years of VLDB research and the holy grail of many a database system. In data management, system performance is defined as acceptable response time and throughput on critical-path operations, ideally with scalability guarantees. Performance is improved with top-of-the line research on fast data management algorithms; their efficiency, however, is contingent on seamless collaboration between the database software and hardware and storage devices. In 1980, target was to minimize disk accesses; in 2000, memory replaced disks in terms of access costs. Nowadays performance is synonymous to scalability; and scalability, in turn, translates into sustainable and predictable use of hardware resources in the face of embarrassing parallelism and deep storage hierarchies while minimizing energy needs — a multidimensionally challenging goal. I will discuss the work done in the past four decades to tighten the interaction between the database software and underlying hardware and explain why, as application and microarchitecture roadmaps evolve, the effort of maintaining smooth collaboration blossoms into a multitude of interesting research questions with direct technological impact.
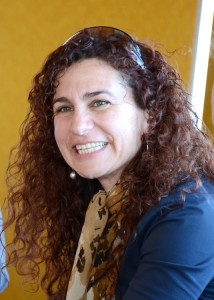
Bio: Anastasia Ailamaki is a Professor of Computer and Communication Sciences at the Ecole Polytechnique Federale de Lausanne (EPFL) in Switzerland. Her research interests are in data-intensive systems and applications, and in particular (a) in strengthening the interaction between the database software and emerging hardware and I/O devices, and (b) in automating data management to support computationally-demanding, data-intensive scientific applications. She has received an ERC Consolidator Award (2013), a Finmeccanica endowed chair from the Computer Science Department at Carnegie Mellon (2007), a European Young Investigator Award from the European Science Foundation (2007), an Alfred P. Sloan Research Fellowship (2005), eight best-paper awards in database, storage, and computer architecture conferences (2001-2012), and an NSF CAREER award (2002). She holds a Ph.D. in Computer Science from the University of Wisconsin-Madison in 2000. She is the vice chair of the ACM SIGMOD community, a senior member of the IEEE, and has served as a CRA-W mentor. She is a member of the Global Agenda Council for Data, Society and Development of the World Economic Forum.
Tuesday Sep 1st 10:15-10:45
Refreshments: Coffee Break
Location: Grand Promenade
Tuesday Sep 1st 10:45-12:15
Research 1: Big Data Systems Analysis
Location: Kings 1
Chair: Philippe Cudre-Mauroux


Shared Execution of Recurring Workloads in MapReduce
Chuan Lei (Worcester Polytechnic Institute), Zhongfang Zhuang (Worcester Polytechnic Institute), Elke Rundensteiner (Worcester Polytechnic Institute (US), Mohamed Eltabakh (Worcester Polytechnic Institute)
With the increasing complexity of data-intensive MapReduce workloads, Hadoop must often accommodate hundreds or even thousands of recurring analytics queries that periodically execute over frequently updated datasets, e.g., latest stock transactions, new log files, or recent news feeds. For many applications, such recurring queries come with user-specified service-level agreements (SLAs), commonly expressed as the maximum allowed latency for producing results before their merits decay. The recurring nature of these emerging workloads combined with their SLA constraints make it challenging to share and optimize their execution. While some recent efforts on multi-job optimization in the MapReduce context have emerged, they focus on sharing work among ad-hoc jobs on static datasets. Unfortunately, these sharing techniques neither take the recurring nature of the queries into account nor guarantee the satisfaction of SLA requirements. In this work, we propose the first scalable multi-query sharing engine tailored for recurring workloads in the MapReduce infrastructure, called Helix. First, Helix deploys sliced window alignment techniques to reveal sharing opportunities among recurring queries without introducing additional MapReduce overhead. Based on the aligned slices, Helix leverages an integrated model of sharing groups and execution ordering to efficiently produce an optimized shared execution plan for all recurring queries in a single pass. Our experimental results over real-world datasets confirm that Helix significantly outperforms the state-of-art techniques by an order of magnitude.


A Performance Study of Big Data on Small Nodes
Dumitrel Loghin (NUS), Bogdan Tudor (NUS), Hao Zhang (NUS), Beng Chin Ooi (NUS), Yong Meng Teo (NUS)
The continuous increase in volume, variety and velocity of Big Data exposes datacenter resource scaling to an energy utilization problem. Traditionally, datacenters employ x86-64 (big) server nodes with power usage of tens to hundreds of Watts. But lately, low-power (small) systems originally developed for mobile devices have seen significant improvements in performance. These improvements could lead to the adoption of such small systems in servers, as announced by major industry players. In this context, we systematically conduct a performance study of Big Data execution on small nodes in comparison with traditional big nodes, and present insights that would be useful for future development. We run Hadoop MapReduce, MySQL and in-memory Shark workloads on clusters of ARM big.LITTLE boards and Intel Xeon server systems. We evaluate execution time, energy usage and total cost of running the workloads on self-hosted ARM and Xeon nodes. Our study shows that there is no one size fits all rule for judging the efficiency of executing Big Data workloads on small and big nodes. But small memory size, low memory and I/O bandwidths, and software immaturity concur in canceling the lower-power advantage of ARM servers. We show that I/O-intensive MapReduce workloads are more energy-efficient to run on Xeon nodes. In contrast, database query processing is always more energy-efficient on ARM servers, at the cost of slightly lower throughput. With minor software modifications, CPU-intensive MapReduce workloads are almost four times cheaper to execute on ARM servers.


Understanding the Causes of Consistency Anomalies in Apache Cassandra
Hua Fan (University of Waterloo), Aditya Ramaraju (University of Waterloo), Marlon McKenzie (University of Waterloo), Wojciech Golab (University of Waterloo), Bernard Wong (University of Waterloo)
A recent paper on benchmarking eventual consistency showed that when a constant workload is applied against Cassandra, the staleness of values returned by read operations exhibits interesting but unexplained variations when plotted against time. In this paper we reproduce this phenomenon and investigate in greater depth the low-level mechanisms that give rise to stale reads. We show that the staleness spikes exhibited by Cassandra are strongly correlated with garbage collection, particularly the "stop-the-world" phase which pauses all application threads in a Java virtual machine. We show experimentally that the staleness spikes can be virtually eliminated by delaying read operations artificially at servers immediately after a garbage collection pause. In our experiments this yields more than a 98% reduction in the number of consistency anomalies that exceed 5ms, and has negligible impact on throughput and latency.


Compaction management in distributed key-value datastores
Muhammad Yousuf Ahmad (McGill University), Bettina Kemme (McGill)
Compactions are a vital maintenance mechanism used by datastores based on the log-structured merge-tree to counter the continuous buildup of data files under update-intensive workloads. While compactions help keep read latencies in check over the long run, this comes at the cost of significantly degraded read performance over the course of the compaction itself. In this paper, we offer an in-depth analysis of compaction-related performance overheads and propose techniques for their mitigation. We offload large, expensive compactions to a dedicated compaction server to allow the datastore server to better utilize its resources towards serving the actual workload. Moreover, since the newly compacted data is already cached in the compaction server's main memory, we fetch this data over the network directly into the datastore server's local cache, thereby avoiding the performance penalty of reading it back from the filesystem. In fact, pre-fetching the compacted data from the remote cache prior to switching the workload over to it can eliminate local cache misses altogether. Therefore, we implement a smarter warmup algorithm that ensures that all incoming read requests are served from the datastore server's local cache even as it is warming up. We have integrated our solution into HBase, and using the YCSB and TPC-C benchmarks, we show that our approach significantly mitigates compaction-related performance problems. We also demonstrate the scalability of our solution by distributing compactions across multiple compaction servers.


Fuzzy Joins in MapReduce: An Experimental Study
Ben Kimmett (University of Victoria), Venkatesh Srinivasan (University of Victoria), Alex Thomo (University of Victoria)
We report experimental results for the MapReduce algorithms proposed by Afrati, Das Sarma, Menestrina, Parameswaran and Ullman in ICDE'12 to compute fuzzy joins of binary strings using Hamming Distance. Their algorithms come with complete theoretical analysis, however, no experimental evaluation is provided. They argue that there is a tradeoff between communication cost and processing cost, and that there is a skyline of the proposed algorithms; i.e. none dominates another. We observe via experiments that the algorithms' actual behavior is more clear-cut than what the theoretical analysis suggests. We provide detailed experimental results and insights that show the different facets of each algorithm.
Research 2: Caching and Indexing
Location: Kings 2
Chair: Jens Dittrich


Sharing Buffer Pool Memory in Multi-Tenant Relational Database-as-a-Service
Vivek Narasayya (Microsoft), Ishai Menache (Microsoft Research), Mohit Singh (Microsoft Research), Feng Li (Microsoft Research), Manoj Syamala (Microsoft Research), Surajit Chaudhuri (Microsoft)
Relational database-as-a-service (DaaS) providers need to rely on multi-tenancy and resource sharing among tenants, since statically reserving resources for a tenant is not cost effective. A major consequence of resource sharing is that the performance of one tenant can be adversely affected by resource demands of other co-located tenants. One such resource that is essential for good performance of a tenant’s workload is buffer pool memory. In this paper, we study the problem of how to effectively share buffer pool memory in multi-tenant relational DaaS. We first develop an SLA framework that defines and enforces accountability of the service provider to the tenant even when buffer pool memory is not statically reserved on behalf of the tenant. Next, we present a novel buffer pool page replacement algorithm (MT-LRU) that builds upon theoretical concepts from weighted online caching, and is designed for multi-tenant scenarios involving SLAs and overbooking. MT-LRU generalizes the LRU-K algorithm which is commonly used in relational database systems. We have prototyped our techniques inside a commercial DaaS engine and extensive experiments demonstrate the effectiveness of our solution.


Optimal Probabilistic Cache Stampede Prevention
Andrea Vattani (Goodreads Amazon Inc), Flavio Chierichetti (Sapienza), Keegan Lowenstein (Bugsnag Inc.)
When a frequently-accessed cache item expires, multiple requests to that item can trigger a cache miss and start regenerating that same item at the same time. This phenomenon, known as cache stampede, severely limits the performance of databases and web servers. A natural countermeasure to this issue is to let the processes that perform such requests to randomly ask for a regeneration before the expiration time of the item. In this paper we give optimal algorithms for performing such probabilistic early expirations. Our algorithms are theoretically optimal and have much better performances than other solutions used in real-world applications.


Indexing Highly Dynamic Hierarchical Data
Jan Finis (TU München), Robert Brunel (Technische Universität München), Alfons Kemper (TU München), Thomas Neumann (TU München), Norman May (SAP AG), Franz Faerber (SAP SE)
Maintaining and querying hierarchical data in a relational database system is an important task in many business applications. This task is especially challenging when considering dynamic use cases with a high rate of complex, possibly skewed structural updates. Labeling schemes are widely considered the indexing technique of choice for hierarchical data, and many different schemes have been proposed. However, they cannot handle dynamic use cases well due to various problems which we investigate in this paper. We therefore propose our dynamic Order Indexes, which offer competitive query performance, unprecedented update efficiency, and robustness for highly dynamic workloads.


BF-Tree: Approximate Tree Indexing
Manos Athanassoulis (EPFL), Anastasia Ailamaki (EPFL)
The increasing volume of time-based generated data and the shift in storage technologies suggest that we might need to reconsider indexing. Several workloads - like social and service monitoring - often include attributes with implicit clustering because of their time-dependent nature. In addition, solid state disks (SSD) (using flash or other low-level technologies) emerge as viable competitors of hard disk drives (HDD). Capacity and access times of storage devices create a trade-off between SSD and HDD. Slow random accesses in HDD have been replaced by efficient random accesses in SSD, but their available capacity is one or more orders of magnitude more expensive than the one of HDD. Indexing, however, is designed assuming HDD as secondary storage, thus minimizing random accesses at the expense of capacity. Indexing data using SSD as secondary storage requires treating capacity as a scarce resource. To this end, we introduce approximate tree indexing, which employs probabilistic data structures (Bloom filters) to trade accuracy for size and produce smaller, yet powerful, tree indexes, which we name Bloom filter trees (BF-Trees). BF-Trees exploit pre-existing data ordering or partitioning to offer competitive search performance. We demonstrate, both by an analytical study and by experimental results, that by using workload knowledge and reducing indexing accuracy up to some extent, we can save substantially on capacity when indexing on ordered or partitioned attributes. In particular, in experiments with a synthetic workload, approximate indexing offers 2.22x-48x smaller index footprint with competitive response times, and in experiments with TPCH and a monitoring real-life dataset from an energy company, it offers 1.6x-4x smaller index footprint with competitive search times as well.
Industrial 1: Crowdsourcing, Data Cleaning, and Using Textual Knowledge Bases
Location: Kings 3
Chair: Kyuseok Shim


Argonaut: Macrotask Crowdsourcing for Complex Data Processing
Daniel Haas (UC Berkeley),Jason Ansel (GoDaddy),Lydia Gu (GoDaddy),Adam Marcus (Unlimited Labs)
Crowdsourced workflows are used in research and industry to solve a variety of tasks. The databases community has used crowd workers in query operators/optimization and for tasks such as entity resolution. Such research utilizes microtasks where crowd workers are asked to answer simple yes/no or multiple choice questions with little training. Typically, microtasks are used with voting algorithms to combine redundant responses from multiple crowd workers to achieve result quality. Microtasks are powerful, but fail in cases where larger context (e.g., domain knowledge) or significant time investment is needed to solve a problem, for example in large-document structured data extraction. In this paper, we consider context-heavy data processing tasks that may require many hours of work, and refer to such tasks as macrotasks. Leveraging the infrastructure and worker pools of existing crowdsourcing platforms, we automate macrotask scheduling, evaluation, and pay scales. A key challenge in macrotask-powered work, however, is evaluating the quality of a worker’s output, since ground truth is seldom available and redundancy-based quality control schemes are impractical. We present Argonaut, a framework that improves macrotask powered work quality using a hierarchical review. Argonaut uses a predictive model of worker quality to select trusted workers to perform review, and a predictive model of task quality to decide which tasks to review. Finally, Argonaut identifies the ideal trade-off between a single phase of review and multiple phases of review given a constrained review budget in order to maximize overall output quality. We evaluate an industrial use of Argonaut to power a structured data extraction pipeline that has utilized over half a million hours of crowd worker input to complete millions of tasks. We show that Argonaut can capture up to 118% more errors than random spot-check reviews in review budget-constrained environments with up to two review layers.


FIT to monitor feed quality
Tamraparni Dasu (AT&T Labs-Research), Vladislav Shkapenyuk (AT&T Labs-Research), Divesh Srivastava (AT&T Labs-Research), Deborah Swayne (AT&T Labs-Research)
While there has been significant focus on collecting and managing data feeds, it is only now that attention is turning to their quality. In this paper, we propose a principled approach to online data quality monitoring in a dynamic feed environment. Our goal is to alert quickly when feed behavior deviates from expectations. We make contributions in two distinct directions. First, we propose novel enhancements to permit a publish-subscribe approach to incorporate data quality modules into the DFMS architecture. Second, we propose novel temporal extensions to standard statistical techniques to adapt them to online feed monitoring for outlier detection and alert generation at multiple scales along three dimensions: aggregation at multiple time intervals to detect at varying levels of sensitivity; multiple lengths of data history for varying the speed at which models adapt to change; and multiple levels of monitoring delay to address lagged data arrival. FIT, or Feed Inspection Tool, is the result of a successful implementation of our approach. We present several case studies outlining the effective deployment of FIT in real applications along with user testimonials.


ConfSeer: Leveraging Customer Support Knowledge Bases for Automated Misconfiguration Detection
Rahul Potharaju (Microsoft), Joseph Chan (Microsoft), Luhui Hu (Microsoft), Cristina Nita-Rotaru (Purdue University), Mingshi Wang (Microsoft), Liyan Zhang Microsoft), Navendu Jain (Microsoft Research)
We introduce ConfSeer, an automated system that detects potential configuration issues or deviations from identified best practices by leveraging a knowledge base (KB) of technical solutions. The intuition is that these KB articles describe the configuration problems and their fixes so if the system can accurately understand them, it can automatically pinpoint both the errors and their resolution. Unfortunately, finding an accurate match is difficult because (a) the KB articles are written in natural language text, and (b) configuration files typically contain a large number of parameters and their value settings thus “expert-driven” manual troubleshooting is not scalable. While there are several state-of-the-art techniques proposed for individual tasks such as keyword matching, concept determination and entity resolution, none offer a practical end-to-end solution to detecting problems in machine configurations. In this paper, we describe our experiences building ConfSeer using a novel combinations of ideas from natural language processing, information retrieval and interactive learning. ConfSeer powers the recommendation engine behind Microsoft Operations Management Suite that proposes fixes for software configuration errors. The system has been running in production for about a year to proactively find misconfigurations on tens of thousands of servers. Our evaluation of ConfSeer against an expert-defined rule-based commercial system, an expert survey and web search engines shows that it achieves 80%-97.5% accuracy and incurs low runtime overheads.
Research 3: Data Mining
Location: Queens 4
Chair: Mourad Ouzzani


SRS: Solving c-Approximate Nearest Neighbor Queries in High Dimensional Euclidean Space with a Tiny Index
Yifang Sun (University of New South Wales), Wei Wang (University of New South Wales), Jianbin Qin (University of New South Wales), Ying Zhang (University of Technology Sydney), Xuemin Lin (University of New South Wales)
Nearest neighbor searches in high-dimensional space have many important applications in domains such as data mining, and multimedia databases. The problem is challenging due to the phenomenon called “curse of dimensionality”. An alternative solution is to consider algorithms that returns a c-approximate nearest neighbor (c-ANN) with guaranteed probabilities. Locality Sensitive Hashing (LSH) is among the most widely adopted method, and it achieve high efficiency both in theory and practice. However, it is known to require an extremely high amount of space for indexing, hence limiting its scalability. In this paper, we propose several surprisingly simple methods to answer c-ANN queries with theoretical guarantees requiring only a single tiny index. Our methods are highly flexible and support a variety of functionalities, such as finding the exact nearest neighbor with any given probability. In the experiment, our methods demonstrate superior performance against the state-of-the-art LSH-based methods, and scale up well to 1 billion high-dimensional points on a single commodity PC.


Rare Time Series Motif Discovery from Unbounded Streams
Nurjahan Begum (UC Riverside), Eamonn Keogh (UC Riverside)
The detection of time series motifs, which are approximately repeated subsequences in time series streams, has been shown to have great utility as a subroutine in many higher-level data mining algorithms. However, this detection becomes much harder in cases where the motifs of interest are vanishingly rare or when faced with a never-ending stream of data. In this work we investigate algorithms to find such rare motifs. We demonstrate that under reasonable assumptions we must abandon any hope of an exact solution to the motif problem as it is normally defined; however, we introduce algorithms that allow us to solve the underlying problem with high probability.


Beyond Itemsets: Mining Frequent Featuresets over Structured Items
Saravanan Thirumuruganathan (University of Texas at Arlingt), Habibur Rahman (University of Texas at Arlington), Sofiane Abbar (Qatar Computing Research Institute), Gautam Das (University of Texas at Arlington)
We assume a dataset of transactions generated by a set of users over structured items where each item could be described through a set of features. In this paper, we are interested in identifying the frequent featuresets (set of features) by mining item transactions. For example, in a news website, items correspond to news articles, the features are the named-entities/topics in the articles and an item transaction would be the set of news articles read by a user within the same session. We show that mining frequent featuresets over structured item transactions is a novel problem and show that straightforward extensions of existing frequent itemset mining techniques provide unsatisfactory results. This is due to the fact that while users are drawn to each item in the transaction due to a subset of its features, the transaction by itself does not provide any information about such underlying preferred features of users. In order to overcome this hurdle, we propose a featureset uncertainty model where each item transaction could have been generated by various featuresets with different probabilities. We describe a novel approach to transform item transactions into uncertain transaction over featuresets and estimate their probabilities using constrained least squares based approach. We propose diverse algorithms to mine frequent featuresets. Our experimental evaluation provides a comparative analysis of the different approaches proposed.


Mining Revenue-Maximizing Bundling Configuration
Loc Do (Singapore Management University), Hady W. Lauw (Singapore Management Univ), Ke Wang (Simon Fraser University)
With greater prevalence of social media, there is an increasing amount of user-generated data revealing consumer preferences for various products and services. Businesses seek to harness this wealth of data to improve their marketing strategies. Bundling, or selling two or more items for one price is a highly-practiced marketing strategy. In this paper, we address the bundle configuration problem from the data-driven perspective. Given a set of items in a seller's inventory, we seek to determine which items should belong to which bundle so as to maximize the total revenue, by mining consumer preferences data. We show that this problem is NP-hard when bundles are allowed to contain more than two items. Therefore, we describe an optimal solution for bundle sizes up to two items, and propose two heuristic solutions for bundles of any larger size. We investigate the effectiveness and the efficiency of the proposed algorithms through experimentations on real-life rating-based preferences data.


ALID: Scalable Dominant Cluster Detection
Lingyang Chu (Institute of Computing Technology UCAS), Shuhui Wang (Institute of Computing Technology UCAS), Siyuan Liu (Carnegie Mellon University), Qingming Huang (University of Chinese Academy of Sciences), Jian Pei (Simon Fraser University)
Detecting dominant clusters is important in many analytic applications. The state-of-the-art methods find dense subgraphs on the affinity graph as the dominant clusters. However, the time and space complexity of those methods are dominated by the construction of the affinity graph, which is quadratic with respect to the number of data points, and thus impractical on large data sets. To tackle the challenge, in this paper, we apply Evolutionary Game Theory (EGT) and develop a scalable algorithm, Approximate Localized Infection Immunization Dynamics (ALID). The major idea is to perform Localized Infection Immunization Dynamics (LID) to find dense subgraph within local range of the affinity graph. LID is further scaled up with guaranteed high efficiency and detection quality by an estimated Region of Interest (ROI) and a carefully designed Candidate Infective Vertex Search method (CIVS). ALID only constructs small local affinity graphs and has a time complexity of $\mathcal{O}(C(a^*+\delta)n)$ and a space complexity of $\mathcal{O}(a^*(a^*+\delta))$, where $a^*$ is the size of the largest dominant cluster and $C\ll{n}$ and $\delta\ll{n}$ are small constants. We demonstrate by extensive experiments on both synthetic data and real world data that ALID achieves state-of-the-art detection quality with much lower time and space cost on single machine. We also demonstrate the encouraging parallelization performance of ALID by implementing the Parallel ALID (PALID) on Apache Spark. PALID processes 50 million SIFT data points in 2.29 hours, achieving a speedup ratio of 7.51 with 8 executors.
Research 4: Graph Mining
Location: Queens 5
Chair: Kamal Karlapalem


Leveraging Graph Dimensions in Online Graph Search
Yuanyuan Zhu (Wuhan University), Jeffrey Xu Yu (The Chinese University of Hong Kong), Lu Qin (University of Technology (Sydney)
Graphs have been widely used due to its expressive power to model complicated relationships. However, given a graph database DG = {g1, g2, ... , gn}, it is challenging to process graph queries since a basic graph query usually involves costly graph operations such as maximum common subgraph and graph edit distance computation, which are NP-hard. In this paper, we study a novel DS-preserved mapping which maps graphs in a graph database DG onto a multidimensional space MG under a structural dimension M using a mapping function phi(). The DS-preserved mapping preserves two things: distance and structure. By the distance-preserving, it means that any two graphs gi and gj in DG must map to two data objects phi(gi) and phi(gj) in MG, such that the distance, d(phi(gi), phi(gj)), between phi(gi) and phi(gj) in MG approximates the graph dissimilarity delta(gi, gj) in DG. By the structure-preserving, it further means that for a given unseen query graph q, the distance between q and any graph gi in DG needs to be preserved such that delta(q, gi) can approximate d(phi(q), phi(gi)). We discuss the rationality of using graph dimension M for online graph processing, and show how to identify a small set of subgraphs to form M efficiently. We propose an iterative algorithm DSPM to compute the graph dimension, and discuss its optimization techniques. We also give an approximate algorithm DSPMap in order to handle a large graph database. We conduct extensive performance studies on both real and synthetic datasets to evaluate the top-k similarity query which is to find top-k similar graphs from DG for a query graph, and show the effectiveness and efficiency of our approaches.


Event Pattern Matching over Graph Streams
Chunyao Song (University of Massachusetts (Lowell), Tingjian Ge (University of Massachusetts (Lowell), Cindy Chen (University of Massachusetts (Lowell), Jie Wang University of Massachusetts (Lowell,)
A graph is a fundamental and general data structure underlying all data applications. Many applications today call for the management and query capabilities directly on graphs. Real time graph streams, as seen in road networks, social and communication networks, and web requests, are such applications. Event pattern matching requires the awareness of graph structures, which is different from traditional complex event processing. It also requires a focus on the dynamicity of the graph, time order constraints in patterns, and online query processing, which deviates significantly from previous work on subgraph matching as well. We study the semantics and efficient online algorithms for this important and intriguing problem, and evaluate our approaches with extensive experiments over real world datasets in four different domains.


An Efficient Similarity Search Framework for SimRank over Large Dynamic Graphs
Yingxia Shao (Peking University), Bin Cui (Peking University), Lei Chen (Hong Kong University of Science and Technology), Mingming Liu (Peking University), Xing Xie (Microsoft Research)
SimRank is an important measure of vertex-pair similarity according to the structure of graphs. The similarity search based on SimRank is an important operation for identifying similar vertices in a graph and has been employed in many data analysis applications. Nowadays, graphs in the real world become much larger and more dynamic. The existing solutions for similarity search are expensive in terms of time and space cost. None of them can efficiently support similarity search over large dynamic graphs. In this paper, we propose a novel two-stage random-walk sampling framework (TSF) for SimRank-based similarity search (e.g., top-$k$ search). In the preprocessing stage, TSF samples a set of one-way graphs to index raw random walks in a novel manner within $\mathcal{O}(NR_g)$ time and space, where $N$ is the number of vertices and $R_g$ is the number of one-way graphs. The one-way graph can be efficiently updated in accordance with the graph modification, thus TSF is well suited to dynamic graphs. During the query stage, TSF can search similar vertices fast by naturally pruning unqualified vertices based on the connectivity of one-way graphs. Furthermore, with additional $R_q$ samples, TSF can estimate the SimRank score with probability $1-2e^{-2\epsilon^{2}\frac{R_gR_q}{(1-c)^2}}$ if the error of approximation is bounded by $1-\epsilon$. Finally, to guarantee the scalability of TSF, the one-way graphs can also be compactly stored on the disk when the memory is limited. Extensive experiments have demonstrated that TSF can handle dynamic billion-edge graphs with high performance.


Growing a Graph Matching from a Handful of Seeds
Ehsan Kazemi (EPFL), Seyed Hamed Hassani (ETHZ), Matthias Grossglauser (EPFL)
In many graph--mining problems, two networks from different domains have to be matched. In the absence of reliable node attributes, graph matching has to rely on only the link structures of the two networks, which amounts to a generalization of the classic graph isomorphism problem. Graph matching has applications in social--network reconciliation and de-anonymization, protein--network alignment in biology, and computer vision. The most scalable graph--matching approaches use ideas from percolation theory, where a matched node pair ``infects'' neighbouring pairs as additional potential matches. This class of matching algorithm requires an initial seed set of known matches to start the percolation. The size and correctness of the matching is very sensitive to the size of the seed set. In this paper, we give a new graph--matching algorithm that can operate with a much smaller seed set than previous approaches, with only a small increase in matching errors. We characterize a phase transition in matching performance as a function of the seed set size, using a random bigraph model and ideas from bootstrap percolation theory. We also show the excellent performance in matching several real large-scale social network, using only a handful of seeds.


Association Rules with Graph Patterns
Wenfei Fan (University Edinburgh and Beihang University), Xin Wang (Southwest Jiaotong University), Yinghui Wu (Washington State University), Jingbo Xu,University Edinburgh and Beihang University)
We propose graph-pattern association rules (GPARs) for social media marketing. Extending association rules for itemsets, GPARs help us discover regularities between entities in social graphs, and identify potential customers by exploring social influence. We propose topological support and confidence measures for GPARs. We study the problem of discovering top-k diversified GPARs. While this problem is NP-hard, we develop a parallel algorithm with accuracy bound. We also study the problem of identifying potential customers with GPARs. While it is also NP-hard, we provide a parallel scalable algorithm that guarantees a polynomial speedup over sequential algorithms with the increase of processors. Using real-life and synthetic graphs, we experimentally verify the scalability and effectiveness of the algorithms.
Tutorial 1: A Time Machine for Information: Looking Back to Look Forward
Location: Queens 6


A Time Machine for Information: Looking Back to Look Forward
Xin Luna Dong, Wang-Chiew Tan
There has been growing interest in harnessing the information available on the web to develop a comprehensive understanding of the history of entities and facts. In this tutorial, we will ambitiously refer to a system that supports exploration of such temporal information as a time machine for information. We discuss real examples and use cases that motivate the need to incorporate the temporal aspects of data. We then survey and present existing work on extraction, linkage, and integration that are central to the development of any information time machine. While one goal of this tutorial is to disseminate the above described material, a parallel goal is to motivate the audience, through our tutorial, to pursue research in the direction of managing and integrating temporal data. We hope that the research community and the industry will become more engaged in this line of research and move towards the ultimate goal of building a time machine that records and preserves history accurately, and helps people “look back” and so as to “look forward”.
Demo 1: Data Mining, Graph, Text, and Semi-structured Data
Location: Kona 4
Chair: Tianli Mo


Evaluating SPARQL Queries on Massive RDF Datasets
Razen Harbi (King Abdullah University of Science and Technology), Ibrahim Abdelaziz (King Abdullah University of Science and Technology), Panos Kalnis (King Abdullah University of Science and Technology), Nikos Mamoulis (University of Ioannina)
Distributed RDF systems partition data across multiple com- puter nodes. Partitioning is typically based on heuristics that minimize inter-node communication and it is performed in an initial, data pre-processing phase. Therefore, the re- sulting partitions are static and do not adapt to changes in the query workload; as a result, existing systems are unable to consistently avoid communication for queries that are not favored by the initial data partitioning. Furthermore, for very large RDF knowledge bases, the partitioning phase be- comes prohibitively expensive, leading to high startup costs. In this paper, we propose AdHash, a distributed RDF system which addresses the shortcomings of previous work. First, AdHash initially applies lightweight hash partitioning, which drastically minimizes the startup cost, while favoring the parallel processing of join patterns on subjects, with- out any data communication. Using a locality-aware plan- ner, queries that cannot be processed in parallel are evalu- ated with minimal communication. Second, AdHash moni- tors the data access patterns and adapts dynamically to the query load by incrementally redistributing and replicating frequently accessed data. As a result, the communication cost for future queries is drastically reduced or even elimi- nated. Our experiments with synthetic and real data verify that AdHash (i) starts faster than all existing systems, (ii) processes thousands of queries before other systems become online, and (iii) gracefully adapts to the query load, being able to evaluate queries on billion-scale RDF data in sub- seconds. In this demonstration, audience can use a graphi- cal interface of AdHash to verify its performance superiority compared to state-of-the-art distributed RDF systems.


Demonstration of Santoku: Optimizing Machine Learning over Normalized Data
Arun Kumar (University of Wisconsin-Madiso), Mona Jalal (University of Wisconsin-Madison), Boqun Yan (University of Wisconsin-Madison), Jeffrey Naughton (University Wisconsin-Madison), Jignesh Patel (University of Wisconsin-Madison)
Advanced analytics is a booming area in the data man- agement industry and a hot research topic. Almost all toolkits that implement machine learning (ML) al- gorithms assume that the input is a single table, but most relational datasets are not stored as single tables due to normalization. Thus, analysts often join tables to obtain a denormalized table. Also, analysts typ- ically ignore any functional dependencies among fea- tures because ML toolkits do not support them. In both cases, time is wasted in learning over data with redundancy. We demonstrate Santoku, a toolkit to help analysts improve the performance of ML over normal- ized data. Santoku applies the idea of factorized learn- ing and automatically decides whether to denormalize or push ML computations through joins. Santoku also exploits database dependencies to provide automatic in- sights that could help analysts with exploratory feature selection. It is usable as a library in R, which is a pop- ular environment for advanced analytics. We demon- strate the benefits of Santoku in improving ML perfor- mance and helping analysts with feature selection.


PRISM: Concept-preserving Summarization of Top-K Social Image Search Results
Boon-Siew Seah (Nanyang Technological University), Sourav S Bhowmick (Nanyang Technological University), Aixin Sun (Nanyang Technological University)
Most existing tag-based social image search engines present search results as a ranked list of images, which cannot be consumed by users in a natural and intuitive manner. In this demonstration, we present a novel concept-preserving image search results summa- rization system called prism. prism exploits both visual features and tags of the search results to generate high quality summary, which not only breaks the results into visually and semantically coherent clusters but it also maximizes the coverage of the original top-k search results. It first constructs a visual similarity graph where the nodes are images in the top-k search results and the edges repre- sent visual similarities between pairs of images. This graph is opti- mally decomposed and compressed into a set of concept-preserving subgraphs based on a set of summarization criteria. One or more exemplar images from each subgraph is selected to form the exem- plar summary of the result set. We demonstrate various innovative features of prism and the promise of superior quality summary con- struction of social image search results.


SPARTex: A Vertex-Centric Framework for RDF Data Analytics
Ibrahim Abdelaziz (King Abdullah University of Science and Technology), Razen Harbi (King Abdullah University of Science and Technology), Semih Salihoglu (Stanford University), Panos Kalnis (King Abdullah University of Science and Technology), Nikos Mamoulis (University of Ioannina)
A growing number of applications require combining SPARQL queries with generic graph search on RDF data. However, the lack of procedural capabilities in SPARQL makes it inappropriate for graph analytics. Moreover, RDF engines focus on SPARQL query evaluation whereas graph management frameworks perform only generic graph computations. In this work, we bridge the gap by introducing SPARTex, an RDF analytics framework based on the vertex-centric computation model. In SPARTex, user-defined ver- tex centric programs can be invoked from SPARQL as stored pro- cedures. SPARTex allows the execution of a pipeline of graph algo- rithms without the need for multiple reads/writes of input data and intermediate results. We use a cost-based optimizer for minimiz- ing the communication cost. SPARTex evaluates queries that com- bine SPARQL and generic graph computations orders of magnitude faster than existing RDF engines. We demonstrate a real system prototype of SPARTex running on a local cluster using real and syn- thetic datasets. SPARTex has a real-time graphical user interface that allows the participants to write regular SPARQL queries, use our proposed SPARQL extension to declaratively invoke graph al- gorithms or combine/pipeline both SPARQL querying and generic graph analytics.


I2RS: A Distributed Geo-Textual Image Retrieval and Recommendation System
Lu Chen (Zhejiang University), Yunjun Gao (Zhejiang University), Zhihao Xing (Zhejiang University), Christian Jensen (Aalborg University), Gang Chen (Zhejiang University)
Massive amounts of geo-tagged and textually annotated images are provided by online photo services such as Flickr and Zommr. However, most existing image retrieval engines only consider text annotations. We present I2RS, a system that allows users to view geo-textual images on Google Maps, find hot topics within a spe- cific geographic region and time period, retrieve images similar to a query image, and receive recommended images that they might be interested in. I2RS is a distributed geo-textual image retrieval and recommendation system that employs SPB-trees to index geo- textual images, and that utilizes metric similarity queries, includ- ing top-m spatio-temporal range and k nearest neighbor queries, to support geo-textual image retrieval and recommendation. The system adopts the browser-server model, whereas the server is deployed in a distributed environment that enables efficiency and scalability to huge amounts of data and requests. A rich set of 100 million geo-textual images crawled from Flickr is used to demon- strate that, I2RS can return high-quality answers in an interactive way and support efficient updates for high image arrival rates.


Reformulation-based query answering in RDF: alternatives and performance
Damian Bursztyn (INRIA), Francois Goasdoue (University of Rennes 1), Ioana Manolescu (INRIA)
Answering queries over Semantic Web data, i.e., RDF graphs, must account for both explicit data and implicit data, en- tailed by the explicit data and the semantic constraints hold- ing on them. Two main query answering techniques have been devised, namely Saturation-based (Sat) which precom- putes and adds to the graph all implicit information, and Reformulation-based (Ref) which reformulates the query based on the graph constraints, so that evaluating the refor- mulated query directly against the explicit data (i.e., with- out considering the constraints) produces the query answer. While Sat is well known, Ref has received less attention so far. In particular, reformulated queries often perform poorly if the query is complex. Our demonstration show- cases a large set of Ref techniques, including but not limited to one we proposed recently. The audience will be able to 1. test them against different datasets, constraints and queries, as well as different well-established systems, 2. analyze and understand the performance challenges they raise, and 3. al- ter the scenarios to visualize the impact on performance. In particular, we show how a cost-based Ref approach allows avoiding reformulation performance pitfalls.


TreeScope: Finding Structural Anomalies In Semi-Structured Data
Shanshan Ying (ADSC), Flip Korn, Barna Saha (University of Massachusetts Amherst), Divesh Srivastava (AT&T Labs-Research)
Semi-structured data are prevalent on the web, with formats such as XML and JSON soaring in popularity due to their generality, flex- ibility and easy customization. However, these very same features make semi-structured data prone to a range of data quality errors, from errors in content to errors in structure. While the former has been well studied, little attention has been paid to structural errors. In this demonstration, we present TREESCOPE, which analyzes semi-structured data sets with the goal of automatically identifying structural anomalies from the data. Our techniques learn robust structural models that have high support, to identify potential errors in the structure. Identified structural anomalies are then concisely summarized to provide plausible explanations of the potential er- rors. The goal of this demonstration is to enable an interactive ex- ploration of the process of identifying and summarizing structural anomalies in semi-structured data sets.


PERSEUS: An Interactive Large-Scale Graph Mining and Visualization Tool
Danai Koutra (Carnegie Mellon University), Di Jin (Carnegie Mellon University), Yuanchi Ning (Uber Technologies Inc.), Christos Faloutsos (Carnegie Mellon University)
Given a large graph with several millions or billions of nodes and edges, such as a social network, how can we explore it efficiently and find out what is in the data? In this demo we present Perseus, a large-scale system that enables the comprehensive analysis of large graphs by supporting the coupled summarization of graph properties and structures, guiding attention to outliers, and allowing the user to inter- actively explore normal and anomalous node behaviors. Specifically, Perseus provides for the following opera- tions: 1) It automatically extracts graph invariants (e.g., degree, PageRank, real eigenvectors) by performing scalable, online batch processing on Hadoop; 2) It interactively visualizes univariate and bivariate distributions for those in- variants; 3) It summarizes the properties of the nodes that the user selects; 4) It efficiently visualizes the induced sub- graph of a selected node and its neighbors, by incrementally revealing its neighbors. In our demonstration, we invite the audience to interact with Perseus to explore a variety of multi-million-edge so- cial networks including a Wikipedia vote network, a friend- ship/foeship network in Slashdot, and a trust network based on the consumer review website Epinions.com.


Virtual eXist-db: Liberating Hierarchical Queries from the Shackles of Access Path Dependence
Curtis Dyreson (Utah State University), Sourav S Bhowmick (Nanyang Technological University), Ryan Grapp (Utah State University)
XQuery programs can be hard to write and port to new data collections because the path expressions in a query are dependent on the hierarchy of the data. We propose to demonstrate a system to liberate query writers from this dependence. A plug-and-play query contains a specification of what data the query needs in order to evaluate. We implemented virtual eXist-db to support plug-and- play XQuery queries. Our system adds a virtualDoc function that lets a programmer sketch the hierarchy needed by the query, which may well be different than what the data has, and logically (not physically) transforms the data (with information loss guarantees) to the hierarchy specified by the virtualDoc. The demonstration will consist of a sequence of XQuery queries using a virtual hierarchy, including queries suggested by the audience. We will also demonstrate a GUI tool to construct a virtual hierarchy.


FLORIN - A System to Support (Near) Real-Time Applica-tions on User Generated Content on Daily News
Qingyuan Liu (Temple University), Eduard Dragut (Temple University), Arjun Mukherjee (University of Houston), Weiyi Meng (Binghamton University)
In this paper, we propose a system, FLORIN, which provides support for near real-time applications on user generated content on daily news. FLORIN continuously crawls news outlets for articles and user comments accompanying them. It attaches the articles and comments to daily event stories. It identifies the opinionated content in user comments and performs named entity recognition on news articles. All these pieces of information are organized hierarchically and exportable to other applications. Multiple applications can be built on this data. We have implemented a sentiment analysis system that runs on top of it.


A Framework for Clustering Uncertain Data
Erich Schubert (Ludwig-Maximilians-Universität Munich), Alexander Koos (Ludwig-Maximilians-Universität München), Tobias Emrich (Ludwig-Maximilians-Universität Munich), Andreas Züfle (Ludwig-Maximilians-Universität München), Klaus Schmid (Ludwig-Maximilians-Universität München), Arthur Zimek (Ludwig-Maximilians-Universität Munich)
The challenges associated with handling uncertain data, in particular with querying and mining, are finding increasing attention in the research community. Here we focus on clustering uncertain data and describe a general framework for this purpose that also allows to visualize and understand the impact of uncertainty—using different uncertainty models—on the data mining results. Our framework constitutes release 0.7 of ELKI (http://elki.dbs.ifi.lmu.de/) and thus comes along with a plethora of implementations of algorithms, distance measures, indexing techniques, evaluation measures and visualization components.

Query-oriented summarization of RDF graphs
Sejla Cebiric (INRIA), Francois Goasdoue (University of Rennes 1), Ioana Manolescu (INRIA)


Universal-DB: Towards Representation Independent Graph Analytics
Yodsawalai Chodpathumwan (University of Illinois), Amirhossein Aleyassin (University of Illinois), Arash Termehchy (Oregon State University), Yizhou Sun (Northeastern University)
Graph analytics algorithms leverage quantiable structural properties of the data to predict interesting concepts and relationships. The same information, however, can be rep- resented using many dierent structures and the structural properties observed over particular representations do not necessarily hold for alternative structures. Because these algorithms tend to be highly eective over some choices of structure, such as that of the databases used to validate them, but not so eective with others, graph analytics has largely remained the province of experts who can nd the desired forms for these algorithms. We argue that in order to make graph analytics usable, we should develop systems that are eective over a wide range of choices of structural organizations. We demonstrate Universal-DB an entity sim- ilarity and proximity search system that returns the same answers for a query over a wide range of choices to represent the input database.


Tornado: A Distributed Spatio-Textual Stream Processing System
Ahmed Mahmood (Purdue University), Ahmed Aly (Purdue University), Thamir Qadah (Purdue University), El Kindi Rezig (Purdue University), Anas Daghistani (Purdue University), Amgad Madkour (Purdue University), Ahmed Abdelhamid (Purdue University), Mohamed Hassan (Purdue University), Walid Aref (Purdue University (USA), Saleh Basalamah (Umm Al-Qura University)
The widespread use of location-aware devices together with the increased popularity of micro-blogging applications (e.g., Twitter) led to the creation of large streams of spatio-textual data. In order to serve real-time applications, the processing of these large-scale spatio-textual streams needs to be distributed. However, existing distributed stream processing systems (e.g., Spark and Storm) are not optimized for spatial/textual content. In this demonstration, we introduce Tornado, a distributed in-memory spatio-textual stream processing server that extends Storm. To efficiently process spatio-textual streams, Tornado introduces a spatio-textual indexing layer to the architecture of Storm. The indexing layer is adaptive, i.e., dynamically re-distributes the processing across the system according to changes in the data distribution and/or query workload. In addition to keywords, higher-level textual concepts are identified and are semantically matched against spatio-textual queries. Tornado provides data deduplication and fusion to eliminate redun- dant textual data. We demonstrate a prototype of Tornado running against real Twitter streams, where the users can register continuous or snapshot spatio-textual queries using a map-assisted query-interface.


S+EPP: Construct and Explore Bisimulation Summaries, plus Optimize Navigational Queries; all on Existing SPARQL Systems
Mariano Consens (University of Toronto), Valeria Fionda (University of Calabria), Shahan Khatchadourian (University of Toronto), Giuseppe Pirrò (ICAR-CNR)
We demonstrate S+EPPs, a system that provides fast construction of bisimulation summaries using graph analytics platforms, and then enhances existing SPARQL engines to support summary-based exploration and navigational query optimization. The construction component adds a novel optimization to a parallel bisimulation algorithm implemented on a multi-core graph processing framework. We show that for several large, disk resident, real world graphs, full sum- mary construction can be completed in roughly the same time as the data load. The query translation component supports Extended Property Paths (EPPs), an enhancement of SPARQL 1.1 property paths that can express a significantly larger class of navigational queries. EPPs are implemented via rewritings into a widely used SPARQL subset. The optimization component can (transparently to users) translate EPPs defined on instance graphs into EPPs that take advantage of bisimulation summaries. S+EPPs combines the query and optimization translations to enable summary-based optimization of graph traversal queries on top of off-the-shelf SPARQL processors. The demonstration showcases the construction of bisimulation summaries of graphs (ranging from millions to billions of edges), together with the exploration benefits and the navigational query speedups obtained by leveraging summaries stored alongside the original datasets.


GraphGen: Exploring Interesting Graphs in Relational Data
Konstantinos Xirogiannopoulos (University of Maryland at College Park), Udayan Khurana (University of Maryland at College Park), Amol Deshpande (University of Maryland at College Park)
Analyzing interconnection structures among the data through the use of graph algorithms and graph analytics has been shown to provide tremendous value in many application domains. However, graphs are not the primary choice for how most data is currently stored, and users who want to employ graph analytics are forced to extract data from their data stores, construct the requisite graphs, and then use a specialized engine to write and execute their graph analysis tasks. This cumbersome and costly process not only raises barriers in using graph analytics, but also makes it hard to explore and identify hidden or implicit graphs in the data. Here we demonstrate a system, called GRAPHGEN, that enables users to declaratively specify graph extraction tasks over relational databases, visually explore the extracted graphs, and write and execute graph algorithms over them, either directly or using existing graph libraries like the widely used NetworkX Python library. We also demonstrate how unifying the extraction tasks and the graph algorithms enables significant optimizations that would not be possible otherwise.


StarDB: A Large-Scale DBMS for Strings
Majed Sahli (King Abdullah University of Science and Technology), Essam Mansour (QCRI), Panos Kalnis (King Abdullah University of Science and Technology)
Strings and applications using them are proliferating in science and business. Currently, strings are stored in file systems and processed using ad-hoc procedural code. Exist- ing techniques are not flexible and cannot efficiently handle complex queries or large datasets. In this paper, we demonstrate StarDB, a distributed database system for analytics on strings. StarDB hides data and system complexities and allows users to focus on analytics. It uses a comprehensive set of parallel string operations and provides a declarative query language to solve complex queries. StarDB automatically tunes itself and runs with over 90% efficiency on supercomputers, public clouds, clusters, and workstations. We test StarDB using real datasets that are 2 orders of magnitude larger than the datasets reported by previous works.
Tuesday Sep 1st 12:15-13:30
Lunch
Location: Lagoon Lanai
Tuesday Sep 1st 13:30-15:00
Turing Award Lecture: Michael Stonebraker
Location: Monarchy Ballroom
Chair: David DeWitt

The Land Sharks are on the Squawk Box (How Riding a Bicycle across America and Building Postgres Have a Lot in Common)
Michael Stonebraker, MIT
This Turing Award talk intermixes a bicycle ride across America during the summer of 1988 with the design, construction and commercialization of Postgres during the late 80’s and early 90’s. Striking parallels are observed, leading to a discussion of what it takes to build a new DBMS. Also, indicated are the roles that perseverance and serendipity played in both endeavors.
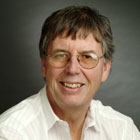
Bio: Michael Stonebraker is an Adjunct Professor of Computer Science at MIT and recipient of the 2014 A.M. Turing Award from the ACM for his fundamental contributions to the concepts and practices underlying modern database systems. He specializes in database management systems and data integration, and has been a pioneer of database research and technology for more than 40 years. He is the author of scores of papers in this area. He was the main architect of the INGRES relational DBMS, the object-relational DBMS POSTGRES, and the federated data system, Mariposa; and principal architect of the C-Store column store database, H-Store main-memory OLTP engine, and SciDB array engine. He has started nine start-up companies to commercialize these database technologies and, more recently, Big Data technologies (Vertica, VoltDB, Paradigm4, Tamr). He is a member of the National Academy of Engineering and the American Academy of Arts and Sciences.
Tuesday Sep 1st 15:00-15:30
Refreshments: Coffee Break
Location: Grand Promenade
Tuesday Sep 1st 15:30-17:00
Research 5: Graph Processing 1
Location: Kings 1
Chair: Wook-Shin Han


Efficient Top-K SimRank-based Similarity Join
Wenbo Tao (Tsinghua University), Minghe Yu (Tsinghua University), Guoliang Li (Tsinghua University)
SimRank is a popular and widely-adopted measure on the similarity between nodes in a graph. It is time and space consuming to compute the SimRank values for all pairs of nodes, especially for large graphs. In real-world applications, users are only interested in the most similar pairs. To address this problem, in this paper we study the top-k SimRank-based similarity join problem, which finds $k$ most similar pairs of nodes with the largest SimRank similarities among all possible pairs. To the best of our knowledge, this is the first attempt to address this problem. We encode each node as a vector by summarizing its near neighbors and transfer the calculation of the SimRank similarity between two nodes to computing the dot product between the corresponding two vectors. We devise an efficient two-step framework to compute top-$k$ similar pairs using the vectors. For large graphs, exact algorithms cannot meet the high-performance requirement, and we also devise an approximate algorithm which can efficiently identify the top-$k$ similar pairs under user-specified accuracy requirement. Experiments on both real and synthetic datasets show our method achieves high performance and good scalability.


MOCgraph: Scalable Distributed Graph Processing Using Message Online Computing
Chang Zhou (Peking University), jun Gao (Peking University), Binbin Sun (Huawei), Jeffrey Xu Yu (The Chinese University of Hong Kong)
Existing distributed graph processing frameworks, e.g., Pregel, Giraph, GPS and GraphLab, mainly exploit main memory to support flexible graph operations for efficiency. Due to the complexity of graph analytics, huge memory space is required especially for those graph analytics that spawn large intermediate results. Existing frameworks may terminate abnormally or degrade performance seriously when the memory is exhausted or the external storage has to be used. In this paper, we propose MOCgraph, a scalable distributed graph processing framework to reduce the memory footprint and improve the scalability, based on message online computing. MOCgraph consumes incoming messages in a streaming manner, so as to handle larger graphs or more complex analytics with the same memory capacity. MOCgraph also exploits message online computing with external storage to provide an efficient out-of-core support. We implement MOCgraph on top of Apache Giraph, and test it against several representative graph algorithms on large graph datasets. Experiments illustrate that MOCgraph is efficient and memory-saving, especially for graph analytics with large intermediate results.


The More the Merrier: Efficient Multi-Source Graph Traversal
Manuel Then (Technische Universität München), Moritz Kaufmann (Technische Universität München), Fernando Chirigati (New York University), Tuan-Anh Hoang-Vu (New York University), Kien Pham (New York University), Alfons Kemper (TUM), Thomas Neumann (TU Munich (Germany), Huy Vo (New York University)
Graph analytics on social networks, Web data, and communication networks has been widely used in a plethora of applications. Many graph analytics algorithms are based on breadth-first search (BFS) graph traversal, which is not only time-consuming for large datasets but also involves much redundant computation when executed multiple times from different start vertices. In this paper, we propose Multi-Source BFS (MS-BFS), an algorithm that is designed to run multiple concurrent BFSs over the same graph on a single CPU core while scaling up as the number of cores increases. MS-BFS leverages the properties of small-world networks, which apply to many real-world graphs, and enables efficient graph traversal that: (i) shares common computation across concurrent BFSs; (ii) greatly reduces the number of random memory accesses; and (iii) does not incur synchronization costs. We demonstrate how a real graph analytics application—all-vertices closeness centrality—can be efficiently solved with MS-BFS. Furthermore, we present an extensive experimental evaluation with both synthetic and real datasets, including Twitter and Wikipedia, showing that MS-BFS provides almost linear scalability with respect to the number of cores and excellent scalability for increasing graph sizes, outperforming state-of-the-art BFS algorithms by more than one order of magnitude when running a large number of BFSs.


Efficient Partial-Pairs SimRank Search on Large Networks
Weiren Yu (Imperial College London), Julie McCann (Imperial College London)
The assessment of node-to-node similarities based on graph topology arises in a myriad of applications, e.g., web search. SimRank is a notable measure of this type, with the intuition that ``two nodes are similar if their in-neighbors are similar''. While most existing work retrieving SimRank only considers all-pairs SimRank $s(\star,\star)$ and single-source SimRank $s(\star,j)$ (scores between every node and query $j$), there are appealing applications for \emph{partial-pairs} SimRank, e.g., similarity join. Given two node subsets $A$ and $B$ in a graph, partial-pairs SimRank assessment aims to retrieve only ${\{s(a,b)\}}_{\forall a \in A, \forall b \in B }$. However, the best-known solution appears not self-contained since it hinges on the premise that the SimRank scores with node-pairs in an $h$-go cover set must be given beforehand. This paper focuses on efficient assessment of partial-pairs SimRank in a self-contained manner. (1) We devise a novel ``seed germination'' model that computes partial-pairs SimRank in $O(k |E| \min\{|A|,|B|\})$ time and $O(|E| + k |V|)$ memory for $k$ iterations on a graph of $|V|$ nodes and $|E|$ edges. (2) We further eliminate unnecessary edge access to improve the time of partial-pairs SimRank to $O(m \min\{|A|,|B|\})$, where $m \le \min\{k|E|, {\Delta}^{2k}\}$, and $\Delta$ is the maximum degree. (3) We show that our partial-pairs SimRank model also can handle the computations of all-pairs and single-source SimRanks. (4) We empirically verify that our algorithms are (a) 38x faster than the best-known competitors, and (b) memory-efficient, allowing scores to be assessed accurately on graphs with tens of millions of links.


Exploiting Vertex Relationships in Speeding up Subgraph Isomorphism over Large Graphs
Xuguang Ren (Griffith University), Junhu Wang (Griffith University)
Subgraph Isomorphism is a fundamental problem in graph data processing. Most existing subgraph isomorphism algorithms are based on a backtracking framework which computes the solutions by incrementally matching all query vertices to candidate data vertices. However, we observe that extensive duplicate computation exists in these algorithms, and such duplicate computation can be avoided by exploiting relationships between data vertices. Motivated by this, we propose a novel approach, BoostIso, to reduce duplicate computation. Our extensive experiments with real datasets show that, after integrating our approach, most existing subgraph isomorphism algorithms can be speeded up significantly, especially for some graphs with intensive vertex relationships, where the improvement can be up to several orders of magnitude.
Research 6: Information Integration
Location: Kings 2
Chair: David Maier


Preference-aware Integration of Temporal Data
Bogdan Alexe (IBM Almaden Research Center), Mary Roth (UCSC and IBM Research), Wang-Chiew Tan (UCSC)
A complete description of an entity is rarely contained in a single data source, but rather, it is often distributed across different data sources. Applications based on personal electronic health records, sentiment analysis, and financial records all illustrate that significant value can be derived from integrated, consistent, and query-able profiles of entities from different sources. Even more so, such integrated profiles are considerably enhanced if temporal information from different sources is carefully accounted for. We develop a simple and yet versatile operator, called PRAWN, that is typically called as a final step of an entity integration workflow. PRAWN is capable of consistently integrating and resolving temporal conflicts in data that may contain multiple dimensions of time based on a set of preference rules specified by a user (hence the name PRAWN for preference-aware union). In the event that not all conflicts can be resolved through preferences, one can enumerate each possible consistent interpretation of the result returned by PRAWN at a given time point through a polynomial-delay algorithm. In addition to providing algorithms for implementing PRAWN, we study and establish several desirable properties of PRAWN. First, PRAWN produces the same temporally integrated outcome, modulo representation of time, regardless of the order in which data sources are integrated. Second, PRAWN can be customized to integrate temporal data for different applications by specifying application-specific preference rules. Third, we show experimentally that our implementation of PRAWN is feasible on both “small” and “big” data platforms in that it is efficient in both storage and execution time. Finally, we demonstrate a fundamental advantage of PRAWN: we illustrate that standard query languages can be immediately used to pose useful temporal queries over the integrated and resolved entity repository.


Optimizing the Chase: Scalable Data Integration under Constraints
George Konstantinidis (USC), Jose-Luis Ambite (USC)
We are interested in scalable data integration and data exchange under constraints/dependencies. In data exchange the problem is how to materialize a target database instance, satisfying the source-to-target and target dependencies, that provides the certain answers. In data integration, the problem is how to rewrite a query over the target schema into a query over the source schemas that provides the certain answers. In both these problems we make use of the chase algorithm, the main tool to reason with dependencies. Our first contribution is to introduce the frugal chase, which produces smaller universal solutions than the standard chase, still remaining polynomial in data complexity. Our second contribution is to use the frugal chase to scale up query answering using views under LAV weakly acyclic target constraints, a useful language capturing RDF/S. The latter problem can be reduced to query rewriting using views without constraints by chasing the source-to-target mappings with the target constraints. We construct a compact graph-based representation of the mappings and the constraints and develop an efficient algorithm to run the frugal chase on this representation. We show experimentally that our approach scales to large problems, speeding up the compilation of the dependencies into the mappings by close to 2 and 3 orders of magnitude, compared to the standard and the core chase, respectively. Compared to the standard chase, we improve online query rewriting time by a factor of 3, while producing equivalent, but smaller, rewritings of the original query.


Supervised Meta-blocking
George Papadakis (IMIS Research Center "Athena"), George Papastefanatos (IMIS Research Center "Athena"), Georgia Koutrika (HP Labs)
Entity Resolution matches mentions of the same entity. Being an expensive task for large data, its performance can be improved by blocking, i.e., grouping similar entities and comparing only entities in the same group. Blocking improves the run-time of Entity Resolution, but it still involves unnecessary comparisons that limit its performance. Meta-blocking is the process of restructuring a block collection in order to prune such comparisons. Existing unsupervised meta-blocking methods use simple pruning rules, which offer a rather coarse-grained filtering technique that can be conservative (i.e., keeping too many unnecessary comparisons) or aggressive (i.e., pruning good comparisons). In this work, we introduce supervised meta-blocking techniques that learn classification models for distinguishing promising comparisons. For this task, we propose a small set of generic features that combine a low extraction cost with high discriminatory power. We show that supervised meta-blocking can achieve high performance with small training sets that can be manually created. We analytically compare our supervised approaches with baseline and competitor methods over 10 large-scale datasets, both real and synthetic.


Enriching Data Imputation with Extensive Similarity Neighbors
Shaoxu Song (Tsinghua University), Aoqian Zhang (Tsinghua University), Lei Chen (Hong Kong University of Science and Technology), Jianmin Wang (Tsinghua University)
Incomplete information often occur along with many database applications, e.g., in data integration, data cleaning or data exchange. The idea of data imputation is to fill the miss- ing data with the values of its neighbors who share the same information. Such neighbors could either be identified certainly by editing rules or statistically by relational de- pendency networks. Unfortunately, owing to data sparsity, the number of neighbors (identified w.r.t. value equality) is rather limited, especially in the presence of data values with variances. In this paper, we argue to extensively en- rich similarity neighbors by similarity rules with tolerance to small variations. More fillings can thus be acquired that the aforesaid equality neighbors fail to reveal. To fill the missing values more, we study the problem of maximizing the missing data imputation. Our major contributions in- clude (1) the np-hardness analysis on solving and approx- imating the problem, (2) exact algorithms for tackling the problem, and (3) efficient approximation with performance guarantees. Experiments on real and synthetic data sets demonstrate that the filling accuracy can be improved.
Industrial 2: Big Data Systems
Location: Kings 3
Chair: Guy Lohman


Gobblin: Unifying Data Ingestion for Hadoop
Lin Qiao (LinkedIn), Yinan Li (LinkedIn), Sahil Takiar (LinkedIn), Ziyang Liu (LinkedIn), Narasimha Veeramreddy (LinkedIn), Min Tu (LinkedIn), Ying Dai (LinkedIn), Issac Buenrostro (LinkedIn), Kapil Surlaker (LinkedIn), Shirshanka Das (LinkedIn), Chavdar Botev (LinkedIn)
We present Gobblin, a generic data-ingestion framework at LinkedIn which was open sourced as of February 2015. The development of Gobblin was mainly driven by the fact that LinkedIn's data sources have become increasingly heterogeneous. Data is constantly obtained and written into online data storage systems or streaming systems, including Espresso, Kafka, Voldemort, Oracle, MySQL, RocksDB and a number of other data stores and event logging systems. Such data include member profiles, connections, posts and many other activities. These data sources crop terabytes worth of data every day, and most of these data needs to be loaded into our Hadoop clusters to feed business- or consumer-oriented analysis. We used to develop a separate data ingestion pipeline for each data source, and at one point we were running over a dozen different types of pipes. Having this many different data ingestion pipelines is like re-implementing the HashMap every time we need to use HashMap with a different type argument. Moreover, these pipelines were developed by several different teams. It is not hard to imagine the non-scalability of this approach, and the issues it brought in terms of maintenance, usability, data format conversion, data quality, and metadata management. Similar pains have been shared with us from engineers at other companies. Gobblin aims to eventually replace most or all of these ingestion pipelines with a generic data ingestion framework, which is easily configurable to ingest data from several different types of sources (covering a large number of real use cases), and easily extensible for new data sources and use cases.


Schema-Agnostic Indexing with Azure DocumentDB
Dharma Shukla (Microsoft), Shireesh Thota (Microsoft), Karthik Raman (Microsoft), Madhan Gajendran (Microsoft), Ankur Shah (Microsoft), Sergii Ziuzin (Microsoft), Krishnan Sundaram (Microsoft), Miguel Gonzalez Guajardo (Microsoft), Anna Wawrzyniak (Microsoft), Samer Boshra (Microsoft), Renato Ferreira (Microsoft), Mohamed Nassar (Microsoft), Michael Koltachev (Microsoft), Ji Huang (Microsoft), Sudipta Sengupta (Microsoft), Justin Levandoski (Microsoft), David Lomet (Microsoft)
Azure DocumentDB is Microsoft’s multi-tenant distributed database service for managing JSON documents at Internet scale. DocumentDB is now generally available to Azure developers. In this paper, we describe the DocumentDB indexing subsystem. DocumentDB indexing enables automatic indexing of documents without requiring a schema or secondary indices. Uniquely, DocumentDB provides real-time consistent queries in the face of very high rates of document updates. As a multi-tenant service, DocumentDB is designed to operate within extremely frugal resource budgets while providing predictable performance and robust resource isolation to its tenants. This paper describes the DocumentDB capabilities, including document representation, query language, document indexing approach, core index support, and early production experiences.


Scaling Spark in the Real World: Performance and Usability
Michael Armbrust (Databricks Inc), Tathagata Das (Databricks Inc), Aaron Davidson (Databricks Inc), Ali Ghodsi (Databricks Inc), Andrew Or (Databricks Inc), Josh Rosen (Databricks Inc), Ion Stoica (UC Berkeley), Patrick Wendell (Databricks Inc), Reynold Xin (Databricks Inc), Matei Zaharia (MIT CSAIL)
Apache Spark is one of the most widely used open source processing engines for big data, with rich language-integrated APIs and a wide range of libraries. Over the past two years, our group has worked to bring Spark to a variety of organizations, through consulting relationships and a hosted service, Databricks Cloud. In this talk, we describe the main challenges and requirements that appeared in taking Spark to a wider variety of users, and usability and performance improvements we have made to the engine in response.
Research 7: Query Interfaces and Languages
Location: Queens 4
Chair: Bernhard Mitschang


Answering Why-not Questions on Reverse Top-k Queries
Yunjun Gao (Zhejiang University), Qing Liu (Zhejiang University), Gang Chen (Zhejiang University), Baihua Zheng (Singapore Management University), Linlin Zhou (Zhejiang University)
Why-not questions, which aim to seek clarifications on the miss-ing tuples for query results, have recently received considerable attention from the database community. In this paper, we system-atically explore why-not questions on reverse top-k queries, ow-ing to its importance in multi-criteria decision making. Given an initial reverse top-k query and a missing/why-not weighting vec-tor set Wm that is absent from the query result, why-not questions on reverse top-k queries explain why Wm does not appear in the query result and provide suggestions on how to refine the initial query with minimum penalty to include Wm in the refined query result. We first formalize why-not questions on reverse top-k que-ries and reveal their semantics, and then propose a unified frame-work called WQRTQ to answer why-not questions on both mono-chromatic and bichromatic reverse top-k queries. Our framework offers three solutions, namely, (i) modifying a query point q, (ii) modifying a why-not weighting vector set Wm and a parameter k, and (iii) modifying q, Wm, and k simultaneously, to cater for dif-ferent application scenarios. Extensive experimental evaluation using both real and synthetic data sets verifies the effectiveness and efficiency of the presented algorithms.


SnapToQuery: Providing Interactive Feedback during Exploratory Query Specification
Lilong Jiang (Ohio State University), Arnab Nandi (Ohio State University)
A critical challenge in the data exploration process is discovering and issuing the "right" query, especially when the space of possible queries is large. This problem of exploratory query specification is exacerbated by the use of interactive user interfaces driven by mouse, touch, or next-generation, three-dimensional, motion capture-based devices; which, are often imprecise due to jitter and sensitivity issues. In this paper, we propose SnapToQuery, a novel technique that guides users through the query space by providing interactive feedback during the query specification process by "snapping" to the user's likely intended queries. These intended queries can be derived from prior query logs, or from the data itself, using methods described in this paper. In order to provide interactive response times over large datasets, we propose two data reduction techniques when snapping to these queries. Performance experiments demonstrate that our algorithms help maintain an interactive experience while allowing for accurate guidance. User studies over three kinds of devices(mouse, touch, and motion capture) show that SnapToQuery can help users specify queries quicker and more accurately; resulting in a query specification time speedup of 1.4x for mouse and touch-based devices and 2.2x for motion capture-based devices.


Constructing an Interactive Natural Language Interface for Relational Databases
Fei Li (University of Michigan), H V Jagadish (University of Michigan)
Natural language has been the holy grail of query interface designers, but has generally been considered too hard to work with, except in limited specific circumstances. In this paper, we describe the architecture of an interactive natural language query interface for relational databases. Through a carefully limited interaction with the user, we are able to correctly interpret complex natural language queries, in a generic manner across a range of domains. By these means, a logically complex English language sentence is correctly translated into a SQL query, which may include aggregation, nesting, and various types of joins, among other things, and can be evaluated against an RDBMS. We have constructed a system, NaLIR (Natural Language Interface for Relational databases), embodying these ideas. Our experimental assessment, through user studies, demonstrates that NaLIR is good enough to be usable in practice: even naive users are able to specify quite complex ad-hoc queries.


A Natural Language Interface for Querying General and Individual Knowledge
Yael Amsterdamer (Tel Aviv University), Anna Kukliansky (Tel Aviv University), Tova Milo (Tel Aviv University)
Many real-life scenarios require the joint analysis of general knowledge, which includes facts about the world, with individual knowledge, which relates to the opinions or habits of individuals. Recently developed crowd mining platforms, which were designed for such tasks, are a major step towards the solution. However, these platforms require users to specify their information needs in a formal, declarative language, which may be too complicated for naive users. To make the joint analysis of general and individual knowledge accessible to the public, it is desirable to provide an interface that translates the user questions, posed in natural language (NL), into the formal query languages that crowd mining platforms support. While the translation of NL questions to queries over conventional databases has been studied in previous work, a setting with mixed individual and general knowledge raises unique challenges. In particular, to support the distinct query constructs associated with these two types of knowledge, the NL question must be partitioned and translated using different means; yet eventually all the translated parts should be seamlessly combined to a well-formed query. To account for these challenges, we design and implement a modular translation framework that employs new solutions along with state-of-the art NL parsing tools. The results of our experimental study, involving real user questions on various topics, demonstrate that our framework provides a high-quality translation for many questions that are not handled by previous translation tools.


Possible and Certain SQL Keys
Henning Köhler (Massey University), Sebastian Link (The University of Auckland), Xiaofang Zhou (The University of Queensland)
Driven by the dominance of the relational model, the requirements of modern applications, and the veracity of data, we revisit the fundamental notion of a key in relational databases with NULLs. In SQL database systems primary key columns are NOT NULL by default. NULL columns may occur in unique constraints which only guarantee uniqueness for tuples which do not feature null markers in any of the columns involved, and therefore serve a different function than primary keys. We investigate the notions of possible and certain keys, which are keys that hold in some or all possible worlds that can originate from an SQL table, respectively. Possible keys coincide with the unique constraint of SQL, and thus provide a semantics for their syntactic definition in the SQL standard. Certain keys extend primary keys to include NULL columns, and thus form a sufficient and necessary condition to identify tuples uniquely, while primary keys are only sufficient for that purpose. In addition to basic characterization, axiomatization, and simple discovery approaches for possible and certain keys, we investigate the existence and construction of Armstrong tables, and describe an indexing scheme for enforcing certain keys. Our experiments show that certain keys with NULLs do occur in real-world databases, and that related computational problems can be solved efficiently. Certain keys are therefore semantically well-founded and able to maintain data quality in the form of Codd's entity integrity rule while handling the requirements of modern applications, that is, higher volumes of incomplete data from different formats.
Research 8: Social Computing and Recommendations
Location: Queens 5
Chair: Mahashweta Das


D2P: Distance-Based Differential Privacy in Recommenders
Rachid Guerraoui (EPFL), Anne-Marie Kermarrec (INRIA), Rhicheek Patra (EPFL), Mahsa Taziki (EPFL)
The upsurge in the number of web users over the last two decades has resulted in a significant growth of online information. This information growth calls for recommenders that personalize the information proposed to each individual user. Nevertheless, personalization also opens major privacy concerns. This paper presents D2P, a novel protocol that ensures a strong form of differential privacy, which we call distance-based differential privacy, and which is particularly well suited to recommenders. D2P avoids revealing exact user profiles by creating altered profiles where each item is replaced with another one at some distance. We evaluate D2P analytically and experimentally on MovieLens and Jester datasets and compare it with other private and non-private recommenders.


Show Me the Money: Dynamic Recommendations for Revenue Maximization
Wei Lu (University of British Columbia), Shanshan Chen (University of British Columbia), Keqian Li (University of British Columbia), Laks V. S. Lakshmanan (University of British Columbia)
Recommender Systems (RS) play a vital role in applications such as e-commerce and on-demand content streaming. Research on RS has mainly focused on the customer perspective, i.e., accurate prediction of user preferences and maximization of user utilities. As a result, most existing techniques are not explicitly built for revenue maximization, the primary business goal of enterprises. In this work, we explore and exploit a novel connection between RS and the profitability of a business. As recommendations can be seen as an information channel between a business and its customers, it is interesting and important to investigate how to make strategic dynamic recommendations leading to maximum possible revenue. To this end, we propose a novel revenue model that takes into account a variety of factors including prices, valuations, saturation effects, and competition amongst products. Under this model, we study the problem of finding revenue-maximizing recommendation strategies over a finite time horizon. We show that this problem is NP-hard, but approximation guarantees can be obtained for a slightly relaxed version, by establishing an elegant connection to matroid theory. Given the prohibitively high complexity of the approximation algorithm, we also design intelligent heuristics for the original problem. Finally, we conduct extensive experiments on two real and synthetic datasets and demonstrate the efficiency, scalability, and effectiveness our algorithms, and that they significantly outperform several intuitive baselines.


Finish Them!: Pricing Algorithms for Human Computation
Yihan Gao (UIUC), Aditya Parameswaran (Massachusetts Institute of Technology)
Given a batch of human computation tasks, a commonly ignored aspect is how the price (i.e., the reward paid to human workers) of these tasks must be set or varied in order to meet latency or cost constraints. Often, the price is set up-front and not modified, leading to either a much higher monetary cost than needed (if the price is set too high), or to a much larger latency than expected (if the price is set too low). Leveraging a pricing model described in prior work, we develop algorithms to optimally set and then vary price over time in order to (a) meet a user-specified deadline while minimizing total monetary cost (b) meet a user-specified monetary budget constraint while minimizing total elapsed time. We leverage techniques from decision theory (specifically, Markov Decision Processes) for both these problems, and demonstrate that our techniques lead to up to 30% reduction in cost over schemes proposed in prior work. Furthermore, we develop techniques to speed-up the computation, enabling users to leverage the price setting algorithms on-the-fly.


TransactiveDB: Tapping into Collective Human Memories
Michele Catasta (EPFL), Alberto Tonon (University of Fribourg), Djellel Eddine Difallah (University of Fribourg), Gianluca Demartini (eXascale Infolab), Karl Aberer (EPFL), Philippe Cudré-Mauroux (University of Fribourg)
Database Management Systems (DBMSs) have been rapidly evolving in the recent years, exploring ways to store multi-structured data or to involve human processes during query execution. In this paper, we outline a future avenue for DBMSs supporting transactive memory queries that can only be answered by a collection of individuals connected through a given interaction graph. We present TransactiveDB and its ecosystem, which allow users to pose queries in order to reconstruct collective human memories. We describe a set of new transactive operators including TUnion, TFill, TJoin, and TProjection. We also describe how TransactiveDB leverages transactive operators---by mixing query execution, social network analysis and human computation---in order to effectively and efficiently tap into the memories of all targeted users.


Worker Skill Estimation in Team-Based Tasks
Habibur Rahman (University of Texas at Arlington), Saravanan Thirumuruganathan (University of Texas at Arlingt), Senjuti Basu Roy (UW), Sihem Amer-Yahia (LIG), Gautam Das (University of Texas at Arlington)
Many emerging applications such as collaborative editing, multi-player games, or fan-subbing require to form a team of experts to accomplish a task together. Existing research has investigated how to assign workers to such team-based tasks to ensure the best outcome assuming the skills of individual workers to be known. In this work, we investigate how to estimate individual worker's skill based on the outcome of the team-based tasks they have undertaken. We consider two popular {\em skill aggregation functions} and estimate that the skill of a worker, which is either a {\em deterministic value or a probability distribution}. We propose efficient solutions for worker skill estimation using continuous and discrete optimization techniques. We present comprehensive experiments and validate the scalability and effectiveness of our proposed solutions using multiple real-world datasets.
Tutorial 2: On Uncertain Graphs Modeling and Queries
Location: Queens 6


On Uncertain Graphs Modeling and Queries
Arijit Khan, Lei Chen
Large-scale, highly-interconnected networks pervade both our society and the natural world around us. Uncertainty, on the other hand, is inherent in the underlying data due to a variety of reasons, such as noisy measurements, lack of precise information needs, inference and prediction models, or explicit manipulation, e.g., for privacy purposes. Therefore, uncertain, or probabilistic, graphs are increasingly used to represent noisy linked data in many emerging application scenarios, and they have recently become a hot topic in the database research community. While many classical graph algorithms such as reachability and shortest path queries become #P-complete, and hence, more expensive in uncertain graphs; various complex queries are also emerging over uncertain networks, such as pattern matching, information diffusion, and influence maximization queries. In this tutorial, we discuss the sources of uncertain graphs and their applications, uncertainty modeling, as well as the complexities and algorithmic advances on uncertain graphs processing in the context of both classical and emerging graph queries. We emphasize the current challenges and highlight some future research directions.
Demo 2: Information Retrieval, Data Quality, and Provenance
Location: Kona 4
Chair: Tianli Mo


A Topic-based Reviewer Assignment System
Ngai Meng Kou (University of Macau), Leong Hou U (University of Macau), Nikos Mamoulis (University of Hong Kong), Yuhong Li (University of Macau), Ye Li (University of Macau), Zhiguo Gong (University of Macau)
Peer reviewing is a widely accepted mechanism for assessing the quality of submitted articles to scientific conferences or journals. Conference management systems (CMS) are used by conference organizers to invite appropriate reviewers and assign them to submitted papers. Typical CMS rely on paper bids entered by the reviewers and apply simple matching algorithms to compute the paper assignment. In this paper, we demonstrate our Reviewer Assignment System (RAS), which has advanced features compared to broadly used CMSs. First, RAS automatically extracts the profiles of reviewers and submissions in the form of topic vectors. These profiles can be used to automatically assign reviewers to papers without relying on a bidding process, which can be tedious and error-prone. Second, besides supporting classic assignment models (e.g., stable marriage and optimal assignment), RAS includes a recently published assignment model by our research group, which maximizes, for each paper, the coverage of its topics by the profiles of its reviewers. The features of the demonstration include (1) automatic extraction of paper and reviewer profiles, (2) assignment computation by different models, and (3) visualization of the results by different models, in order to assess their effectiveness.


Data Profiling with Metanome
Thorsten Papenbrock (Hasso-Plattner-Institute), Tanja Bergmann (Hasso-Plattner-Institute), Moritz Finke (Hasso-Plattner-Institute), Jakob Zwiener (Hasso-Plattner-Institute), Felix Naumann (Hasso-Plattner-Institute)
Data profiling is the discipline of discovering metadata about given datasets. The metadata itself serve a variety of use cases, such as data integration, data cleansing, or query optimization. Due to the importance of data profiling in practice, many tools have emerged that support data scientists and IT professionals in this task. These tools provide good support for profiling statistics that are easy to compute, but they are usually lacking automatic and efficient discovery of complex statistics, such as inclusion dependencies, unique column combinations, or functional dependencies. We present Metanome, an extensible profiling platform that in- corporates many state-of-the-art profiling algorithms. While Meta- nome is able to calculate simple profiling statistics in relational data, its focus lies on the automatic discovery of complex metadata. Metanome’s goal is to provide novel profiling algorithms from research, perform comparative evaluations, and to support developers in building and testing new algorithms. In addition, Metanome is able to rank profiling results according to various metrics and to visualize the, at times, large metadata sets.


Provenance for SQL through Abstract Interpretation: Value-less, but Worthwhile
Tobias Müller (U Tübingen), Torsten Grust (U Tübingen)
We demonstrate the derivation of fine-grained where- and why-provenance for a rich dialect of SQL that includes recursion, (correlated) subqueries, windows, grouping/aggregation, and the RDBMS’s library of built-in functions. The approach relies on ideas that originate in the programming language community—program slicing and abstract interpretation, in particular. A two-stage process first records a query’s control flow decisions and locations of data access before it derives provenance without consultation of the actual data values (rendering the method largely “value-less”). We will bring an interactive demonstrator that uses this provenance information to make input/output dependencies in real-world SQL queries tangible.


SAASFEE: Scalable Scientific Workflow Execution Engine
Marc Bux (Humboldt-Universität zu Berlin), Jörgen Brandt (Humboldt-Universität zu Berlin), Carsten Lipka (Humboldt-Universität zu Berlin), Kamal Hakimzadeh (KTH Royal Institute of Technology), Jim Dowling (KTH Royal Institute of Technology), Ulf Leser (Humboldt Universität zu Berlin)
Across many fields of science, primary data sets like sensor read-outs, time series, and genomic sequences are analyzed by complex chains of specialized tools and scripts exchanging intermediate results in domain-specific file formats. Scientific workflow management systems (SWfMSs) support the development and execution of these tool chains by providing workflow specification languages, graphical editors, fault-tolerant execution engines, etc. However, many SWfMSs are not prepared to handle large data sets because of inadequate support for distributed computing. On the other hand, most SWfMSs that do support distributed computing only allow static task execution orders. We present SAASFEE, a SWfMS which runs arbitrarily complex work- flows on Hadoop YARN. Workflows are specified in Cuneiform, a functional workflow language focusing on parallelization and easy integration of existing software. Cuneiform workflows are executed on Hi-WAY, a higher-level scheduler for running workflows on YARN. Distinct features of SAASFEE are the ability to execute iterative workflows, an adaptive task scheduler, re-executable provenance traces, and compatibility to selected other workflow systems. In the demonstration, we present all components of SAASFEE using real-life workflows from the field of genomics.


QOCO: A Query Oriented Data Cleaning System with Oracles
Moria Bergman (Tel Aviv University), Tova Milo (Tel Aviv University), Slava Novgorodov (Tel Aviv University), Wang-Chiew Tan (University of California Santa Cruz)
As key decisions are often made based on information contained in a database, it is important for the database to be as complete and correct as possible. For this reason, many data cleaning tools have been developed to automatically resolve inconsistencies in databases. However, data cleaning tools provide only best-effort results and usually cannot eradicate all errors that may exist in a database. Even more importantly, existing data cleaning tools do not typically address the problem of determining what information is missing from a database. To tackle these problems, we present QOCO, a novel query oriented cleaning system that leverages materialized views that are defined by user queries as a trigger for identifying the remaining incorrect/missing information. Given a user query, QOCO inter- acts with domain experts (which we model as oracle crowds) to identify potentially wrong or missing answers in the result of the user query, as well as determine and correct the wrong data that is the cause for the error(s). We will demonstrate QOCO over a World Cup Games database, and illustrate the interaction between QOCO and the oracles. Our demo audience will play the role of oracles, and we show how QOCO’s underlying operations and optimization mechanisms can effectively prune the search space and minimize the number of questions that need to be posed to accelerate the cleaning process.


Collaborative Data Analytics with DataHub
Anant Bhardwaj (MIT), Amol Deshpande (University of Maryland), Aaron Elmore (University of Chicago), David Karger (MIT),Sam Madden (MIT), Aditya Parameswaran (University of Illinois at Urbana Champaign), Harihar Subramanyam (MIT), Eugene Wu (Columbia), Rebecca Zhang (MIT)
While there have been many solutions proposed for storing and analyzing large volumes of data, all of these solutions have limited support for collaborative data analytics, especially given the many individuals and teams are simultaneously analyzing, modifying and exchanging datasets, employing a number of heterogeneous tools or languages for data analysis, and writing scripts to clean, preprocess, or query data. We demonstrate DataHub, a unified platform with the ability to load, store, query, collaboratively analyze, interactively visualize, interface with external applications, and share datasets. We will demonstrate the following aspects of the DataHub platform: (a) flexible data storage, sharing, and native version- ing capabilities: multiple conference attendees can concurrently update the database and browse the different versions and inspect conflicts; (b) an app ecosystem that hosts apps for various data- processing activities: conference attendees will be able to effortlessly ingest, query, and visualize data using our existing apps; (c) thrift-based data serialization permits data analysis in any combination of 20+ languages, with DataHub as the common data store: conference attendees will be able to analyze datasets in R, Python, and Matlab, while the inputs and the results are still stored in DataHub. In particular, conference attendees will be able to use the DataHub notebook — an IPython-based notebook for analyzing data and storing the results of data analysis.


Mindtagger: A Demonstration of Data Labeling in Knowledge Base Construction
Jaeho Shin (Stanford University), Christopher Re (Stanford University), Mike Cafarella (University of Michigan)
End-to-end knowledge base construction systems using statistical inference are enabling more people to automatically extract high-quality domain-specific information from un- structured data. As a result of deploying DeepDive framework across several domains, we found new challenges in debugging and improving such end-to-end systems to construct high-quality knowledge bases. DeepDive has an iterative development cycle in which users improve the data. To help our users, we needed to develop principles for analyzing the system’s error as well as provide tooling for inspecting and labeling various data products of the system. We created guidelines for error analysis modeled after our colleagues’ best practices, in which data labeling plays a critical role in every step of the analysis. To enable more productive and systematic data labeling, we created Mindtagger, a versatile tool that can be configured to support a wide range of tasks. In this demonstration, we show in detail what data labeling tasks are modeled in our error analysis guidelines and how each of them is performed using Mindtagger.


Annotating Database Schemas to Help Enterprise Search
Eli Cortez (Microsoft), Philip Bernstein (Microsoft), Yeye He (Microsoft Research), Lev Novik (Microsoft)
In large enterprises, data discovery is a common problem faced by users who need to find relevant information in relational databases. In this scenario, schema annotation is a useful tool to enrich a database schema with descriptive keywords. In this paper, we demonstrate Barcelos, a system that automatically annotates corporate databases. Unlike existing annotation approaches that use Web oriented knowledge bases, Barcelos mines enterprise spreadsheets to find candidate annotations. Our experimental evaluation shows that Barcelos produces high quality annotations; the top-5 have an average precision of 87%.


KATARA: Reliable Data Cleaning with Knowledge Bases and Crowdsourcing
Xu Chu (University of Waterloo), John Morcos (University of Waterloo), Ihab Ilyas (University of Waterloo), Mourad Ouzzani (QCRI), Paolo Papotti (QCRI), Nan Tang (QCRI), Yin Ye (Google)
Data cleaning with guaranteed reliability is hard to achieve without accessing external sources, since the truth is not necessarily discoverable from the data at hand. Furthermore, even in the presence of external sources, mainly knowledge bases and humans, effectively leveraging them still faces many challenges, such as aligning heterogeneous data sources and decomposing a complex task into simpler units that can be consumed by humans. We present Katara, a novel end-to-end data cleaning system powered by knowledge bases and crowdsourcing. Given a table, a kb, and a crowd, Katara (i) interprets the table semantics w.r.t. the given kb; (ii) identifies correct and wrong data; and (iii) generates top-k possible repairs for the wrong data. Users will have the opportunity to experience the following features of Katara: (1) Easy specification: Users can define a Katara job with a browser-based specification; (2) Pattern validation: Users can help the system to resolve the ambiguity of different table patterns (i.e., table semantics) discovered by Katara; (3) Data annotation: Users can play the role of internal crowd workers, helping Katara annotate data. Moreover, Katara will visualize the annotated data as correct data validated by the kb, correct data jointly validated by the kb and the crowd, or erroneous tuples along with their possible repairs.


Gain Control over your Integration Evaluations
Patricia Arocena (University of Toronto), Radu Ciucanu (University of Lille (INRIA), Boris Glavic (IIT), Renee Miller (University Toronto)
Integration systems are typically evaluated using a few real-world scenarios (e.g., bibliographical or biological datasets) or using synthetic scenarios (e.g., based on star-schemas or other patterns for schemas and constraints). Reusing such evaluations is a cumbersome task because their focus is usually limited to showcasing a specific feature of an approach. This makes it difficult to compare integration solutions, understand their generality, and understand their performance for different application scenarios. Based on this observation, we demonstrate some of the requirements for develop- ing integration benchmarks. We argue that the major abstractions used for integration problems have converged in the last decade which enables the application of robust empirical methods to integration problems (from schema evolution, to data exchange, to answering queries using views and many more). Specifically, we demonstrate that schema mappings are the main abstraction that now drives most integration solutions and show how a metadata generator can be used to create more credible evaluations of the performance and scalability of data integration systems. We will use the demonstration to evangelize for more robust, shared empirical evaluations of data integration systems.


Janiform Intra-Document Analytics for Reproducible Research
Jens Dittrich (Saarland University), Patrick Bender (Saarland University)
Peer-reviewed publication of research papers is a cornerstone of science. However, one of the many issues of our publication culture is that our publications only publish a summary of the final result of a long project. This means that we put well-polished graphs de- scribing (some) of our experimental results into our publications. However, the algorithms, input datasets, benchmarks, raw result datasets, as well as scripts that were used to produce the graphs in the first place are rarely published and typically not available to other researchers. Often they are only available when personally asking the authors. In many cases, however, they are not available at all. This means from a long workflow that led to producing a graph for a research paper, we only publish the final result rather than the entire workflow. This is unfortunate and has been criticized in various scientific communities. In this demo we argue that one part of the problem is our dated view on what a “document” and hence “a publication” is, should, and can be. As a remedy, we introduce portable database files (PDbF). These files are janiform, i.e. they are at the same time a standard static pdf as well as a highly dynamic (offline) HTML-document. PDbFs allow you to access the raw data behind a graph, perform OLAP-style analysis, and reproduce your own graphs from the raw data — all of this within a portable document. We demo a tool allowing you to create PDbFs smoothly from within LATEX. This tool allows you to preserve the workflow of raw measurement data to its final graph- ical output through all processing steps. Notice that this pdf al- ready showcases our technology: rename this file to “.html” and see what happens (currently we support the desktop versions of Firefox, Chrome, and Safari). But please: do not try to rename this file to “.ova” and mount it in VirtualBox.


EFQ: Why-Not Answer Polynomials in Action
Katerina Tzompanaki (Université Paris Sud), Nicole Bidoit (Université Paris Sud - INRIA), Melanie Herschel (University of Stuttgart)
One important issue in modern database applications is supporting the user with efficient tools to debug and fix queries because such tasks are both time and skill demanding. One particular problem is known as Why-Not question and focusses on the reasons for missing tuples from query results. The EFQ platform demonstrated here has been designed in this context to efficiently leverage Why- Not Answers polynomials, a novel approach that provides the user with complete explanations to Why-Not questions and allows for automatic, relevant query refinements.


Error Diagnosis and Data Profiling with Data X-Ray
Xiaolan Wang (University of Massachusetts Amherst), Mary Feng (University of Massachusetts Amherst and University of Iowa), Yue Wang (University of Massachusetts Amherst), Xin Luna Dong (Google Inc), Alexandra Meliou (University of Massachusetts Amherst)
The problem of identifying and repairing data errors has been an area of persistent focus in data management research. However, while traditional data cleaning techniques can be effective at identifying several data discrepancies, they disregard the fact that many errors are systematic, inherent to the process that produces the data, and thus will keep occurring unless the root cause is identified and corrected. In this demonstration, we will present a large-scale diagnostic framework called DATAXRAY. Like a medical X-ray that aids the diagnosis of medical conditions by revealing problems underneath the surface, DATAXRAY reveals hidden connections and common properties among data errors. Thus, in contrast to traditional clean- ing methods, which treat the symptoms, our system investigates the underlying conditions that cause the errors. The core of DATAXRAY combines an intuitive and principled cost model derived by Bayesian analysis, and an efficient, highly- parallelizable diagnostic algorithm that discovers common proper- ties among erroneous data elements in a top-down fashion. Our system has a simple interface that allows users to load different datasets, to interactively adjust key diagnostic parameters, to explore the derived diagnoses, and to compare with solutions produced by alternative algorithms. Through this demonstration, participants will understand (1) the characteristics of good diagnoses, (2) how and why errors occur in real-world datasets, and (3) the distinctions with other related problems and approaches.


A Demonstration of TripleProv: Tracking and Querying Provenance over Web Data
Marcin Wylot (University of Fribourg), Philippe Cudré-Mauroux (University of Fribourg), Paul Groth (Elsevir Labs)
The proliferation of heterogeneous Linked Data on the Web poses new challenges to database systems. In particular, the capacity to store, track, and query provenance data is becoming a pivotal feature of modern triple stores. In this demonstration, we present TripleProv: a new system extending a native RDF store to efficiently handle the storage, tracking and querying of provenance in RDF data. In the following, we give an overview of our approach providing a reliable and understandable specification of the way results were derived from the data and how particular pieces of data were combined to answer the query. Subsequently, we present techniques enabling to tailor queries with provenance data. Finally, we describe our demonstration and how the attendees will be able to interact with our system during the conference.


WADaR: Joint Wrapper and Data Repair
Stefano Ortona (University of Oxford), Giorgio Orsi (University of Oxford), Marcello Buoncristiano (Universita della Basilicata), Tim Furche (University of Oxford)
Web scraping (or wrapping) is a popular means for acquiring data from the web. Recent advancements have made scalable wrapper-generation possible and enabled data acquisition processes involving thousands of sources. This makes wrapper analysis and maintenance both needed and challenging as no scalable tools exists that support these tasks. We demonstrate WADaR, a scalable and highly auto- mated tool for joint wrapper and data repair. WADaR uses off-the-shelf entity recognisers to locate target entities in wrapper-generated data. Markov chains are used to deter- mine structural repairs, that are then encoded into suitable repairs for both the data and corresponding wrappers. We show that WADaR is able to increase the quality of wrapper-generated relations between 15% and 60%, and to fully repair the corresponding wrapper without any knowledge of the original website in more than 50% of the cases.


Wisteria: Nurturing Scalable Data Cleaning Infrastructure
Daniel Haas (UC Berkeley), Sanjay Krishnan (UC Berkeley), Jiannan Wang (UC Berkeley), Michael Franklin (UC Berkeley), Eugene Wu (Columbia University)
Analysts report spending upwards of 80% of their time on problems in data cleaning. The data cleaning process is inherently iterative, with evolving cleaning workflows that start with basic exploratory data analysis on small samples of dirty data, then refine analysis with more sophisticated/expensive cleaning operators (e.g., crowd- sourcing), and finally apply the insights to a full dataset. While an analyst often knows at a logical level what operations need to be done, they often have to manage a large search space of physical operators and parameters. We present Wisteria, a system designed to support the iterative development and optimization of data clean- ing workflows, especially ones that utilize the crowd. Wisteria separates logical operations from physical implementations, and driven by analyst feedback, suggests optimizations and/or replace- ments to the analyst’s choice of physical implementation. We high- light research challenges in sampling, in-flight operator replace- ment, and crowdsourcing. We overview the system architecture and these techniques, then provide a demonstration designed to show- case how Wisteria can improve iterative data analysis and cleaning. The code is available at: http://www.sampleclean.org.
Tuesday Sep 1st 17:15-19:00
Reception and Poster Session 1
Location: Kohala Ballroom
Chair: Tianli Mo
Shared Execution of Recurring Workloads in MapReduce
Chuan Lei - Zhongfang Zhuang - Elke Rundensteiner - Mohamed Eltabakh
A Performance Study of Big Data on Small Nodes
Dumitrel Loghin - Bogdan Tudor - Hao Zhang - Beng Chin Ooi - Yong Meng Teo
Understanding the Causes of Consistency Anomalies in Apache Cassandra
Hua Fan - Aditya Ramaraju - Marlon McKenzie - Wojciech Golab - Bernard Wong
Compaction management in distributed key-value datastores
Muhammad Yousuf Ahmad - Bettina Kemme
Fuzzy Joins in MapReduce: An Experimental Study
Ben Kimmett - Venkatesh Srinivasan - Alex Thomo
Sharing Buffer Pool Memory in Multi-Tenant Relational Database-as-a-Service
Vivek Narasayya - Ishai Menache - Mohit Singh - Feng Li - Manoj Syamala - Surajit Chaudhuri
Optimal Probabilistic Cache Stampede Prevention
Andrea Vattani - Flavio Chierichetti - Keegan Lowenstein
Indexing Highly Dynamic Hierarchical Data
Jan Finis - Robert Brunel - Alfons Kemper - Thomas Neumann - Norman May - Franz Faerber
BF-Tree: Approximate Tree Indexing
Manos Athanassoulis - Anastasia Ailamaki
SRS: Solving c-Approximate Nearest Neighbor Queries in High Dimensional Euclidean Space with a Tiny Index
Yifang Sun - Wei Wang - Jianbin Qin - Ying Zhang - Xuemin Lin
Rare Time Series Motif Discovery from Unbounded Streams
Nurjahan Begum - Eamonn Keogh
Beyond Itemsets: Mining Frequent Featuresets over Structured Items
Saravanan Thirumuruganathan - Habibur Rahman - Sofiane Abbar - Gautam Das
Mining Revenue-Maximizing Bundling Configuration
Loc Do - Hady W. Lauw - Ke Wang
ALID: Scalable Dominant Cluster Detection
Lingyang Chu - Shuhui Wang - Siyuan Liu - Qingming Huang - Jian Pei
Leveraging Graph Dimensions in Online Graph Search
Yuanyuan Zhu - Jeffrey Xu Yu - Lu Qin
Event Pattern Matching over Graph Streams
Chunyao Song - Tingjian Ge - Cindy Chen - Jie Wang
An Efficient Similarity Search Framework for SimRank over Large Dynamic Graphs
Yingxia Shao - Bin Cui - Lei Chen - Mingming Liu - Xing Xie
Growing a Graph Matching from a Handful of Seeds
Ehsan Kazemi - Seyed Hamed Hassani - Matthias Grossglauser
Association Rules with Graph Patterns
Wenfei Fan - Xin Wang - Yinghui Wu - Jingbo Xu
Efficient Top-K SimRank-based Similarity Join
Wenbo Tao - Minghe Yu - Guoliang Li
MOCgraph: Scalable Distributed Graph Processing Using Message Online Computing
Chang Zhou - jun Gao - Binbin Sun - Jeffrey Xu Yu
The More the Merrier: Efficient Multi-Source Graph Traversal
Manuel Then - Moritz Kaufmann - Fernando Chirigati - Tuan-Anh Hoang-Vu - Kien Pham - Alfons Kemper - Thomas Neumann - Huy Vo
Efficient Partial-Pairs SimRank Search on Large Networks
Weiren Yu - Julie McCann
Exploiting Vertex Relationships in Speeding up Subgraph Isomorphism over Large Graphs
Xuguang Ren - Junhu Wang
Preference-aware Integration of Temporal Data
Bogdan Alexe - Mary Roth - Wang-Chiew Tan
Optimizing the Chase: Scalable Data Integration under Constraints
George Konstantinidis - Jose-Luis Ambite
Supervised Meta-blocking
George Papadakis - George Papastefanatos - Georgia Koutrika
Enriching Data Imputation with Extensive Similarity Neighbors
Shaoxu Song - Aoqian Zhang - Lei Chen - Jianmin Wang
Answering Why-not Questions on Reverse Top-k Queries
Yunjun Gao - Qing Liu - Gang Chen - Baihua Zheng - Linlin Zhou
SnapToQuery: Providing Interactive Feedback during Exploratory Query Specification
Lilong Jiang - Arnab Nandi
Constructing an Interactive Natural Language Interface for Relational Databases
Fei Li - H. V. Jagadish
A Natural Language Interface for Querying General and Individual Knowledge
Yael Amsterdamer - Anna Kukliansky - Tova Milo
Possible and Certain SQL Keys
Henning Kohler - Sebastian Link - Xiaofang Zhou
D2P: Distance-Based Differential Privacy in Recommenders
Rachid Guerraoui - Anne-Marie Kermarrec - Rhicheek Patra - Mahsa Taziki
Show Me the Money: Dynamic Recommendations for Revenue Maximization
Wei Lu - Shanshan Chen - Keqian Li - Laks V. S. Lakshmanan
Finish Them!: Pricing Algorithms for Human Computation
Yihan Gao - Aditya Parameswaran
TransactiveDB: Tapping into Collective Human Memories
Michele Catasta - Alberto Tonon - Djellel Eddine Difallah - Gianluca Demartini - Karl Aberer - Philippe Cudre-Mauroux
Worker Skill Estimation in Team-Based Tasks
Habibur Rahman - Saravanan Thirumuruganathan - Senjuti Basu Roy - Sihem Amer-Yahia - Gautam Das
Scalable Subgraph Enumeration in MapReduce
Longbin Lai - Lu Qin - Xuemin Lin - Lijun Chang
FrogWild! -- Fast PageRank Approximations on Graph Engines
Ioannis Mitliagkas - Michael Borokhovich - Alexandros Dimakis - Constantine Caramanis
Pregel Algorithms for Graph Connectivity Problems with Performance Guarantees
Da Yan - James Cheng - Kai Xing - Yi Lu - Wilfred Ng - Yingyi Bu
Blogel: A Block-Centric Framework for Distributed Computation on Real-World Graphs
Da Yan - James Cheng - Yi Lu - Wilfred Ng
LogGP: A Log-based Dynamic Graph Partitioning Method
Ning Xu - Lei Chen - Bin Cui
Coordination Avoidance in Database Systems
Peter Bailis - Alan Fekete - Michael Franklin - Ali Ghodsi - Joseph Hellerstein - Ion Stoica
A Scalable Search Engine for Mass Storage Smart Objects
Nicolas Anciaux - Saliha Lallali - Iulian Sandu Popa - Philippe Pucheral
Schema Management for Document Stores
Lanjun Wang - Oktie Hassanzadeh - Shuo Zhang - Juwei Shi - Limei Jiao - Jia Zou - Chen Wang
Supporting Scalable Analytics with Latency Constraints
Boduo Li - Yanlei Diao - Prashant Shenoy
Principles of Dataset Versioning: Exploring the Recreation/Storage Tradeoff
Souvik Bhattacherjee - Amit Chavan - Silu Huang - Amol Deshpande - Aditya Parameswaran
Inferring Continuous Dynamic Social Influence and Personal Preference for Temporal Behavior Prediction
Jun Zhang - Chaokun Wang - Jianmin Wang - Jeffrey Xu Yu
Influential Community Search in Large Networks
Rong-Hua LI - Lu Qin - Jeffrey Xu Yu - Rui Mao
Linearized and Single-Pass Belief Propagation
Wolfgang Gatterbauer - Stephan Gunnemann - Danai Koutra - Christos Faloutsos
Online Topic-Aware Influence Maximization
Shuo Chen - Ju Fan - Guoliang Li - Jianhua Feng - Kian-Lee Tan - Jinhui Tang
Walk, Not Wait: Faster Sampling Over Online Social Networks
Azade Nazi - Zhuojie Zhou - Saravanan Thirumuruganathan - Nan Zhang - Gautam Das
Work-Efficient Parallel Skyline Computation for the GPU
Kenneth Bogh - Sean Chester - Ira Assent
Memory-Efficient Hash Joins
R. Barber - G. Lohman - I. Pandis - V. Raman - R. Sidle - G. Attaluri - N. Chainani - S. Lightstone - D. Sharpe
MRCSI: Compressing and Searching String Collections with Multiple References
Sebastian Wandelt - Ulf Leser
Trill: A High-Performance Incremental Query Processor for Diverse Analytics
Badrish Chandramouli - Jonathan Goldstein - Mike Barnett - Robert DeLine - John Platt - James Terwilliger - John Wernsing
Rapid Sampling for Visualizations with Ordering Guarantees
Albert Kim - Eric Blais - Aditya Parameswaran - Piotr Indyk - Sam Madden - Ronitt Rubinfeld
Argonaut: Macrotask Crowdsourcing for Complex Data Processing
Adam Marcus - Lydia Gu - Daniel Haas - Jason Ansel
FIT to monitor feed quality
Tamraparni Dasu - Vladislav Shkapenyuk - Divesh Srivastava - Deborah Swayne
ConfSeer: Leveraging Customer Support Knowledge Bases for Automated Misconfiguration Detection
Rahul Potharaju - Navendu Jain
Gobblin: Unifying Data Ingestion for Hadoop
Lin Qiao - Kapil Surlaker - Shirshanka Das - Chavdar Botev - Yinan Li - Sahil Takiar - Henry Cai - Narasimha Veeramreddy - Min Tu - Ziyang Liu - Ying Dai
Schema-Agnostic Indexing with Azure DocumentDB
Dharma Shukla - Shireesh Thota - Karthik Raman - Madhan Gajendran - Ankur Shah - Sergii Ziuzin - Krishnan Sundaram - Miguel Gonzalez Guajardo - Anna Wawrzyniak - Samer Boshra - Renato Ferreira - Mohamed Nassar - Michael Koltachev - Ji Huang - Sudipta Sengupta - Justin Levandoski - David Lomet
Scaling Spark in the Real World
Michael Armbrust - Tathagata Das - Aaron Davidson - Ali Ghodsi - Andrew Or - Josh Rosen - Ion Stoica - Patrick Wendell - Reynold Xin - Matei Zaharia
JetScope: Reliable and Interactive Analytics at Cloud Scale
Eric Boutin - Jaliya Ekanayake - Anna Korsun - Jingren Zhou
Towards Scalable Real-time Analytics: An Architecture for Scale-out of OLxP Workloads
Jeffrey Pound - Anil Goel - Nathan Auch - Franz Faerber - Francis Gropengiesser - Christian Mathis - Thomas Bodner - Wolfgang Lehner - Scott MacLean - Peter Bumbulis
Real-Time Analytical Processing with SQL Server
Paul Larson - Adrian Birka - Eric Hanson - Weiyun Huang - Michal Novakiewicz - Vassilis Papadimos
The Dataflow Model: A Practical Approach to Balancing Correctness, Latency, and Cost in Massive-Scale, Unbounded, Out-of-Order Data Processing
Tyler Akidau - Robert Bradshaw - Craig Chambers - Slava Chernyak - Rafael Fernandez-Moctezuma - Reuven Lax - Sam McVeety - Daniel Mills - Frances Perry - Eric Schmidt - Sam Whittle
Live Programming Support in the LogicBlox System
Todd Green - Dan Olteanu - Geoffrey Washburn
Indexing and Selecting Hierarchical Business Logic
Anja Gruenheid - Alessandra Loro - Donald Kossman - Damien Profeta - Philippe Beaudequin
Distributed Architecture of Oracle Database In-memory
Niloy Mukherjee - Shasank Chavan - Maria Colgan - Dinesh Das - Mike Gleeson - Sanket Hase - Allison Holloway - Hui Jin - Jesse Kamp - Kartk Kulkarni - Tirthankar Lahiri - Juan Loaiza - Neil Macnaughton - Vineet Marwah - Andy Witkowski - Jiaqi Yan - Mohamed Zait
Gorilla: Facebook's Fast, Scalable, In-Memory Time Series Database
Justin Teller - Scott Franklin - Tuomas Pelkonen - Paul Cavallaro
Query Optimization in Oracle 12c Database In-Memory
Dinesh Das - Jiaqi Yan - Mohamed Zait - Satya Valluri - Nirav Vyas - Ramarajan Krishnamachari - Prashant Gaharwar - Jesse Kamp - Niloy Mukherjee
Building a Replicated Logging System with Apache Kafka
Guozhang Wang - Joel Koshy - Sriram Subramanian - Kartik Paramasivam - Mammad Zadeh - Neha Narkhede - Jun Rao - Jay Kreps - Joe Stein
Optimization of Common Table Expressions in MPP Database Systems
Amr El-Helw - Venkatesh Raghavan - Mohamed Soliman - George Caragea - Zhongxian Gu - Michalis Petropoulos
One Trillion Edges: Graph Processing at Facebook-Scale
Avery Ching - Dionysios Logothetis - Sergey Edunov - Maja Kabiljo - Sambavi Muthukrishnan
Differential Privacy in Telco Big Data Platform
Xueyang Hu - Mingxuan Yuan - Jianguo Yao - Yu Deng - Lei Chen - Haibing Guan - Jia Zeng
Efficient Evaluation of Object-Centric Exploration Queries for Visualization
You Wu - Boulos Harb - Jun Yang - Cong Yu
ACME: A Parallel Cloud-Oriented System for Extracting Frequent Patterns from a Very Long Sequence
Majed Sahli
Efficient Distributed Subgraph Similarity Matching
Ye Yuan
Efficient k-Closest Pair Queries in General Metric Spaces
Yunjun Gao
Task-Assignment Optimization in Knowledge Intensive Crowdsourcing
Senjuti Basu Roy
Data Profiling - A Survey
Felix Naumann
Data Generation for Testing and Grading SQL Queries
Bikash Chandra/S Sudarshan
Wednesday Sep 2nd 07:30-08:30
Light Breakfast
Location: Grand Promenade
Wednesday Sep 2nd 08:30-10:00
Industrial Keynote: Todd Walter; Academic Keynote: Magdalena Balazinska
Location: Monarchy Ballroom
Chair: Chen Li


Big Plateaus of Big Data on the Big Island
Todd Walter, Teradata
In ancient texts, 40 was a magic number. It meant “a lot” or “a long time”. 40 years represented the time it took for a new generation to arise. A look back at 40 years of VLDB suggests this applies to database researchers as well – the young researchers of the early VLDBs are now the old folks of the database world and a new generation is creating afresh. Over this period many plateaus of “Big Data” have challenged the database community and been conquered. But there is still no free lunch – database research is really the science of trade-offs many of which are no different today than 40 years ago. And of course the evolution of hardware technology continues to swing the trade-off pendulum while enabling new plateaus to be reached. Todd will take a look back at customer big data plateaus of the past. He will look at where we are today, then use his crystal ball and the lessons of the past to extrapolate the next several plateaus – how they will be the same and how will they be different. Along the way we will have a little fun with some VLDB and Teradata history.
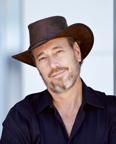
Bio: Todd Walter is the Chief Technologist for Teradata across the Americas region. With substantive expertise in big data, database engineering and systems architecture, he works closely with Teradata customers, colleagues, and alliance partners to evaluate and prioritize initiatives — and implement data strategy and analytics. As a pragmatic visionary, Walter helps customer business analysts as well as technologists better understand all of the astonishing possibilities of big data and analytics in view of emerging as well as existing capabilities of information infrastructures. Todd works with organizations of all sizes and levels of experience, from start-ups to Fortune 100 companies at the leading edge of adopting big data, data warehouse and analytics technologies. Walter has been with Teradata for nearly 28 years, contributing significantly to Teradata's unique design features and functionality. He holds more than a dozen Teradata patents and is a Teradata Fellow, the highest technical award granted by the company. Todd served for more than ten years as Chief Technical Officer of Teradata Labs, responsible for vision, strategy and technical leadership of the Teradata product line before taking on his current strategic consulting role.


Big Data Research: Will Industry Solve all the Problems?
Magdalena Balazinska, University of Washington
The need for effective tools for big data data management and analytics continues to grow. While the ecosystem of tools is expanding many research problems remain open: they include challenges around efficient processing, flexible analytics, ease of use, and operation as a service. Many new systems and much innovation, however, come from industry (or from academic projects that quickly became big players in industry). An important question for our community is whether industry will solve all the problems or whether there is a place for academic research in big data and what is that place. In this talk, we will first look back at the past 40 years of VLDB research and will then discuss some recent research results and open problems.
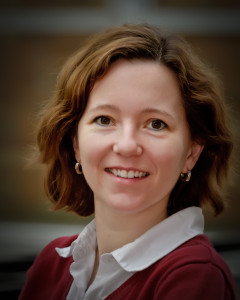
Bio: Magdalena Balazinska is an Associate Professor in the department of Computer Science and Engineering at the University of Washington and the Jean Loup Baer Professor of Computer Science and Engineering. She’s the director of the IGERT PhD Program in Big Data and Data Science. She’s also a Senior Data Science Fellow of the University of Washington eScience Institute. Magdalena’s research interests are in the field of database management systems. Her current research focuses on big data management, scientific data management, and cloud computing. Magdalena holds a Ph.D. from the Massachusetts Institute of Technology (2006). She is a Microsoft Research New Faculty Fellow (2007), received an NSF CAREER Award (2009), a 10-year most influential paper award (2010), an HP Labs Research Innovation Award (2009 and 2010), a Rogel Faculty Support Award (2006), a Microsoft Research Graduate Fellowship (2003-2005), and multiple best-paper awards.
Wednesday Sep 2nd 10:00-10:30
Refreshments: Coffee Break
Location: Grand Promenade
Wednesday Sep 2nd 10:30-12:00
Research 9: Graph Processing 2
Location: Kings 1
Chair: Peter Triantafillou


Scalable Subgraph Enumeration in MapReduce
Longbin Lai (UNSW), Lu Qin (University of Technology (Sydney), Xuemin Lin (University of New South Wales), Lijun Chang (University of New South Wales)
Subgraph enumeration, which aims to find all the subgraphs of a large data graph that are isomorphic to a given pattern graph, is a fundamental graph problem with a wide range of applications. However, existing sequential algorithms for subgraph enumeration fall short in handling large graphs due to the involvement of computationally intensive subgraph isomorphism operations. Thus, some recent researches focus on solving the problem using MapReduce. Nevertheless, exiting MapReduce approaches are not scalable to handle very large graphs since they either produce a huge number of partial results or consume a large amount of memory. Motivated by this, in this paper, we propose a new algorithm TwinTwigJoin based on a left-deep-join framework in MapReduce, in which the basic join unit is a TwinTwig (an edge or two incident edges of a node). We show that in the Erdos-Renyi random-graph model, TwinTwigJoin is instance optimal in the left-deep-join framework under reasonable assumptions, and we devise an algorithm to compute the optimal join plan. Three optimization strategies are explored to improve our algorithm. Furthermore, we discuss how our approach can be adapted in the power-law random-graph model. We conduct extensive performance studies in several real graphs, one of which contains billions of edges. Our approach significantly outperforms existing solutions in all tests.


FrogWild! -- Fast PageRank Approximations on Graph Engines
Ioannis Mitliagkas (UT Austin), Michael Borokhovich (UT Austin), Alexandros Dimakis (UT Austin), Constantine Caramanis (UT Austin)
We propose FrogWild, a novel algorithm for fast approximation of high PageRank vertices. Our algorithm can be seen as a quantized version of power iteration that performs multiple parallel random walks over a directed graph. One important innovation is that we introduce a modification to the GraphLab framework that only partially synchronizes mirror vertices. We show that this partial synchronization creates dependencies between the random walks used to estimate PageRank. Our main theoretical innovation is the analysis of the correlations introduced by this partial synchronization process and a bound establishing that our approximation is close to the true PageRank vector. We implement our algorithm in GraphLab and compare it against the default PageRank implementation. We show that our algorithm is very fast, performing each iteration in less than one second on the Twitter graph and can be up to $7$x faster compared to the standard GraphLab PageRank implementation.


Pregel Algorithms for Graph Connectivity Problems with Performance Guarantees
Da Yan (HKUST), James Cheng (CUHK), Kai Xing (HKUST), Yi Lu (CUHK), Wilfred Ng, Yingyi Bu (UC Irvine)
Graphs in real life applications are often huge, such as the Web graph and various social networks. These massive graphs are often stored and processed in distributed sites. In this paper, we study graph algorithms that adopt Google's Pregel, an iterative vertex-centric framework for graph processing in the Cloud. We first identify a set of desirable properties of an efficient Pregel algorithm, such as linear space, communication and computation cost per iteration, and logarithmic number of iterations. We define such an algorithm as a practical Pregel algorithm (PPA). We then propose PPAs for computing connected components (CCs), biconnected components (BCCs) and strongly connected components (SCCs). The PPAs for computing BCCs and SCCs use the PPAs of many fundamental graph problems as building blocks, which are of interest by themselves. Extensive experiments over large real graphs verified the efficiency of our algorithms.


LogGP: A Log-based Dynamic Graph Partitioning Method
Ning Xu, Lei Chen (Hong Kong University of Science and Technology), Bin Cui (Peking University)
With the increasing availability and scale of graph data from Web 2.0, graph partitioning becomes one of efficient pre-processing techniques to balance the computing workload. Since the cost of partitioning the entire graph is strictly prohibitive, there are some recent tentative works towards streaming graph partitioning which can run faster, be easily paralleled, and be incrementally updated. Unfortunately, the experiments show that the running time of each partitioning is still unbalanced due to the variation of workload access pattens during the supersteps. In addition, the one-pass streaming partitioning result is not always satisfactory for the algorithms' local view of the graph. In this paper, we present LogGP, a log-based graph partitioning system that records, analyzes and reuses the historical statistical information to refine the partitioning result. LogGP can be used as a middle-ware and deployed to many state-of-the-art paralleled graph processing systems easily. LogGP utilizes the historical partitioning results to generate a hyper-graph and uses a novel hyper-graph streaming partitioning approach to generate a better initial streaming graph partitioning result. During the execution, the system uses running logs to optimize graph partitioning which prevents performance degradation. Moreover, LogGP can dynamically repartition the massive graphs in accordance with the structural changes. Extensive experiments conducted on a moderate size of computing cluster with real-world graph datasets demonstrate the superiority of our approach against the state-of-the-art solutions.


Blogel: A Block-Centric Framework for Distributed Computation on Real-World Graphs
Da Yan (HKUST), James Cheng (CUHK), Yi Lu (CUHK), Wilfred Ng, The Hong Kong University of Science and Technology)
The rapid growth in the volume of many real-world graphs (e.g., social networks, web graphs, and spatial networks) has led to the development of various vertex-centric distributed graph computing systems in recent years. However, real-world graphs from different domains have very different characteristics, which often create bottlenecks in vertex-centric parallel graph computation. We identify three such important characteristics from a wide spectrum of real-world graphs, namely (1)skewed degree distribution, (2)large diameter, and (3)(relatively) high density. Among them, only (1) has been studied by existing systems, but many real-world power-law graphs also exhibit the characteristics of~(2) and~(3). In this paper, we propose a block-centric framework, called Blogel, which naturally handles all the three adverse graph characteristics. Blogel programmers may think like a block and develop efficient algorithms for various graph problems. We propose parallel algorithms to partition an arbitrary graph into blocks efficiently, and block-centric programs are then run over these blocks. Our experiments on large real-world graphs verified that Blogel is able to achieve orders of magnitude performance improvements over the state-of-the-art distributed graph computing systems.
Research 10: Novel DB Architectures
Location: Kings 2
Chair: Sharad Mehrotra


Coordination Avoidance in Database Systems
Peter Bailis (UC Berkeley), Alan Fekete (University of Sydney), Michael Franklin (UC Berkeley), Ali Ghodsi (UC Berkeley), Joseph Hellerstein (UC Berkeley), Ion Stoica (UC Berkeley)
Minimizing coordination, or blocking communication between concurrently executing operations, is key to maximizing scalability, availability, and high performance in database systems. However, uninhibited coordination-free execution can compromise application correctness, or consistency. When is coordination necessary for correctness? The classic use of serializable transactions is sufficient to maintain correctness but is not necessary for all applications, sacrificing potential scalability. In this paper, we develop a formal framework, invariant confluence, that determines whether an application requires coordination for correct execution. By operating on application-level invariants over database states (e.g., integrity constraints), invariant confluence analysis provides a necessary and sufficient condition for safe, coordination-free execution. When programmers specify their application invariants, this analysis allows databases to coordinate only when anomalies that might violate invariants are possible. We analyze the invariant confluence of common invariants and operations from real-world database systems (i.e., integrity constraints) and applications and show that many are invariant confluent and therefore achievable without coordination. We apply these results to a proof-of-concept coordination-avoiding database prototype and demonstrate sizable performance gains compared to serializable execution, notably a 25-fold improvement over prior TPC-C New-Order performance on a 200 server cluster.


A Scalable Search Engine for Mass Storage Smart Objects
Nicolas Anciaux (INRA and University of Versailles Saint-Quentin), Saliha Lallali (INRIA and University of Versailles Saint-Quentin), Iulian Sandu Popa (University of Versailles), Philippe Pucheral (INRIA and University of Versailles Saint-Quentin)
This paper presents a new embedded search engine designed for smart objects. Such devices are generally equipped with extremely low RAM and large Flash storage capacity. To tackle these conflicting hardware constraints, conventional search engines privilege either insertion or query scalability but cannot meet both requirements at the same time. Moreover, very few solutions support document deletions and updates in this context. In this paper, we introduce three design principles, namely Write-Once Partitioning, Linear Pipelining and Background Linear Merging, and show how they can be combined to produce an embedded search engine reconciling high insert/delete/update rate and query scalability. We have implemented our search engine on a development board having a hardware configuration representative for smart objects and have conducted extensive experiments using two representative datasets. The experimental results demonstrate the scalability of the approach and its superiority compared to state of the art methods.


Principles of Dataset Versioning: Exploring the Recreation/Storage Tradeoff
Souvik Bhattacherjee (University of Maryland (College Park), Amit Chavan (University of Maryland at College Park), Silu Huang (University of Illinois at Urbana-Champaign), Amol Deshpande (University of Maryland at College Park), Aditya Parameswaran,University of Illinois at Urbana-Champaign)
The relative ease of collaborative data science and analysis has led to a proliferation of many thousands or millions of versions of the same datasets in many scientific and commercial domains, acquired or constructed at various stages of data analysis across many users, and often over long periods of time. Managing, storing, and recreating these dataset versions is a non-trivial task. The fundamental challenge here is the storage-recreation trade-off: the more storage we use, the faster it is to recreate or retrieve versions, while the less storage we use, the slower it is to recreate or retrieve versions. Despite the fundamental nature of this problem, there has been a surprisingly little amount of work on it. In this paper, we study this trade-off in a principled manner: we formulate six problems under various settings, trading off these quantities in various ways, demonstrate that most of the problems are intractable, and propose a suite of inexpensive heuristics drawing from techniques in delay-constrained scheduling, and spanning tree literature, to solve these problems. We have built a prototype version management system, that aims to serve as a foundation to our DATAHUB system for facilitating collaborative data science. We demonstrate, via extensive experiments, that our proposed heuristics provide efficient solutions in practical dataset versioning scenarios.


Schema Management for Document Stores
Lanjun Wang (IBM Research-China), Oktie Hassanzadeh (IBM Research), Shuo Zhang (IBM Research-China), Juwei Shi (IBM Research-China), Limei Jiao, Jia Zou (IBM Research-China), Chen Wang (Tsinghua University)
Document stores that provide the efficiency of a schema-less interface are widely used by developers in mobile and cloud applications. However, the simplicity developers achieved controversially leads to complexity for data management due to lack of a schema. In this paper, we present a schema management framework for document stores. This framework discovers and persists schemas of JSON records in a repository, and also supports queries and schema summarization. The major technical challenge comes from varied structures of records caused by the schema-less data model and schema evolution. In the discovery phase, we apply a canonical form based method and propose an algorithm based on equivalent sub-trees to group equivalent schemas efficiently. Together with the algorithm, we propose a new data structure, eSiBu-Tree, to store schemas and support queries. In order to present a single summarized representation for heterogenous schemas in records, we introduce the concept of ``skeleton'', and propose to use it as a relaxed form of the schema, which captures a small set of core attributes. Finally, extensive experiments based on real data sets demonstrate the efficiency of our proposed schema discovery algorithms, and practical use cases in real-world data exploration and integration scenarios are presented to illustrate the effectiveness of using skeletons in these applications.


Supporting Scalable Analytics with Latency Constraints
Boduo Li (University of Massachusetts Amherst), Yanlei Diao (University of Massachusetts Amherst), Prashant Shenoy (University of Massachusetts Amherst)
Recently there has been a significant interest in building big data analytics systems that can handle both "big data" and "fast data". Our work is strongly motivated by recent real-world use cases that point to the need for a general, unified data processing framework to support analytical queries with different latency requirements. Toward this goal, we start with an analysis of existing big data systems to understand the causes of high latency. We then propose an extended architecture with mini-batches as granularity for computation and shuffling, and augment it with new model-driven resource allocation and runtime scheduling techniques to meet user latency requirements while maximizing throughput. Results from real-world workloads show that our techniques, implemented in Incremental Hadoop, reduce its latency from tens of seconds to sub-second, with 2x-5x increase in throughput. Our system also outperforms state-of-the-art distributed stream systems, Storm and Spark Streaming, by 1-2 orders of magnitude when combining latency and throughput.
Industrial 3: Real-time and Interactive Analytics
Location: Kings 3
Chair: Fatma Ozcan


JetScope: Reliable and Interactive Analytics at Cloud Scale
Eric Boutin (Microsoft), Paul Brett), Microsoft), Xiaoyu Chen), Microsoft), Jaliya Ekanayake (Microsoft), Tao Guan (Microsoft), Anna Korsun (Microsoft), Zhicheng Yin (Microsoft), Nan Zhang (Microsoft), Jingren Zhou (Microsoft)
Interactive, reliable, and rich data analytics at cloud scale is a key capability to support low latency data exploration and experimentation over terabytes of data for a wide range of business scenarios. Besides the challenges in massive scalability and low latency distributed query processing, it is imperative to achieve all these requirements with effective fault tolerance and efficient recovery, as failures and fluctuations are the norm in such a distributed environment. We present a cloud scale interactive query processing system, called JetScope, developed at Microsoft. The system has a SQL-like declarative scripting language and delivers massive scalability and high performance through advanced optimizations. In order to achieve low latency, the system leverages various access methods, optimizes delivering first rows, and maximizes network and scheduling efficiency. The system also provides a fine-grained fault tolerance mechanism which is able to efficiently detect and mitigate failures without significantly impacting the query latency and user experience. JetScope has been deployed to hundreds of servers in production at Microsoft, serving a few million queries every day.


Towards Scalable Real-time Analytics: An Architecture for Scale-out of OLxP Workloads
Anil Goel (SAP Labs),Jeffrey Pound (SAP Labs), Nathan Auch (SAP Labs), Peter Bumbulis (SAP Labs), Scott MacLean (SAP Labs), Franz Faerber (SAP SE), Francis Gropengiesser (SAP SE), Christian Mathis (SAP SE), Thomas Bodner (SAP SE), Wolfgang Lehner (TU Dresden)
We present an overview of our work on the SAP HANA Scale-out Extension, a novel distributed database architecture designed to support large scale analytics over real-time data. This platform permits high performance OLAP with massive scale-out capabilities, while concurrently allowing OLTP workloads. This dual capability enables analytics over real-time changing data and allows fine grained user-specified service level agreements (SLAs) on data freshness. We advocate the decoupling of core database components such as query processing, concurrency control, and persistence, a design choice made possible by advances in high-throughput low-latency networks and storage devices. We provide full ACID guarantees and build on a logical timestamp mechanism to provide MVCC-based snapshot isolation, while not requiring synchronous updates of replicas. Instead, we use asynchronous update propagation guaranteeing consistency with timestamp validation. We provide a view into the design and development of a large scale data management platform for real-time analytics, driven by the needs of modern enterprise customers.


Real-Time Analytical Processing with SQL Server
Paul Larson (Microsoft), Adrian Birka (Microsoft), Eric Hanson (Microsoft), Weiyun Huang (Microsoft), Michal Novakiewicz (Microsoft), Vassilis Papadimos (Microsoft)
Over the last two releases SQL Server has integrated two specialized engines into the core system: the Apollo column store engine for analytical workloads and the Hekaton in-memory engine for high-performance OLTP workloads. There is an increasing demand for real-time analytics, that is, for running analytical queries and reporting on the same system as transaction processing so as to have access to the freshest data. SQL Server 2016 will include enhancements to column store indexes and in-memory tables that significantly improve performance on such hybrid work-loads. This paper describes four such enhancements: column store indexes on in-memory tables, making secondary column store indexes on disk-based tables updatable, allowing B-tree indexes on primary column store indexes, and further speeding up the column store scan operator.
Research 11: Social Network Analysis
Location: Queens 4
Chair: Laks Lakshmanan


Inferring Continuous Dynamic Social Influence and Personal Preference for Temporal Behavior Prediction
Jun Zhang (Tsinghua University), Chaokun Wang (Tsinghua University), Jianmin Wang (Tsinghua University), Jeffrey Xu Yu (The Chinese University of Hong Kong (Hong Kong)
It is always attractive and challenging to explore the intricate behavior data and uncover people's motivations, preference and habits, which can greatly benefit many tasks including link prediction, item recommendation, etc. Traditional work usually studies people's behaviors without time information in a static or discrete manner, assuming the underlying factors stay invariant in a long period. However, we believe people's behaviors are dynamic, and the contributing factors including the social influence and personal preference for behaviors are varying continuously over time. Such continuous dynamics convey important knowledge about people's behavior patterns; ignoring them would lead to inaccurate models. In this work, we address the continuous dynamic modeling of temporal behaviors. To model the fully continuous temporal dynamics of behaviors and the underlying factors, we propose the DP-Space, a dynamic preference probability space, which can capture their smooth variation in various shapes over time with flexible basis functions. Upon that we propose a generative dynamic behavior model, ConTyor, which considers the temporal item-adoption behaviors as joint effect of dynamic social influence and varying personal preference over continuous time. We also develop effective inference methods for ConTyor and present its applications. We conduct a comprehensive experimental study using real-world datasets to evaluate the effectiveness of our model and the temporal modeling. Results verify that ConTyor outperforms existing state-of-the-art static and temporal models in behavior predictions. Moreover, in our detailed study on temporal modeling, we show that temporal modeling is superior to static approaches and modeling over continuous time is further better than that over discrete time. We also demonstrate that the ancient behavior data can still become important and beneficial if modeled well.


Influential Community Search in Large Networks
Rong-Hua LI (CUHK), Lu Qin (University of Technology (Sydney), Jeffrey Xu Yu (The Chinese University of Hong Kong), Rui Mao (Shenzhen University)
Community search is a problem of finding densely connected subgraphs that satisfy the query conditions in a network, which has attracted much attention in recent years. However, all the previous studies on community search do not consider the influence of a community. In this paper, we introduce a novel community model called $k$-influential community based on the concept of $k$-core, which can capture the influence of a community. Based on the new community model, we propose a linear-time online search algorithm to find the top-$r$ $k$-influential communities in a network. To further speed up the influential community search algorithm, we devise a linear-space index structure which supports efficient search of the top-$r$ $k$-influential communities in optimal time. We also propose an efficient algorithm to maintain the index when the network is frequently updated. We conduct extensive experiments on 7 real-world large networks, and the results demonstrate the efficiency and effectiveness of the proposed methods.


Linearized and Single-Pass Belief Propagation
Wolfgang Gatterbauer (Carnegie Mellon University), Stephan Günnemann (Carnegie Mellon University), Danai Koutra (Carnegie Mellon University), Christos Faloutsos (Carnegie Mellon University)
How can we tell when accounts are fake or real in a social network? And how can we tell which accounts belong to liberal, conservative or centrist users? Often, we can answer such questions and label nodes in a network based on the labels of their neighbors and appropriate assumptions of homophily ("birds of a feather fock together") or heterophily ("opposites attract"). One of the most widely used methods for this kind of inference is Belief Propagation (BP) which iteratively propagates the information from a few nodes with explicit labels throughout a network until convergence. A well-known problem with BP, however, is that there are no known exact guarantees of convergence in graphs with loops. This paper introduces Linearized Belief Propagation (LinBP), a linearization of BP that allows a closed-form solution via intuitive matrix equations and, thus, comes with exact convergence guarantees. It handles homophily, heterophily, and more general cases that arise in multi-class settings. Plus, it allows a compact implementation in SQL. The paper also introduces Single-pass Belief Propagation (SBP), a localized (or "myopic") version of LinBP that propagates information across every edge at most once and for which the final class assignments depend only on the nearest labeled neighbors. In addition, SBP allows fast incremental updates in dynamic networks. Our runtime experiments show that LinBP and SBP are orders of magnitude faster than standard BP, while leading to almost identical node labels.


Online Topic-Aware Influence Maximization
Shuo Chen (Tsinghua University), Ju Fan (National University of Singapore), Guoliang Li (Tsinghua University), Jianhua Feng (Tsinghua University), Kian-Lee Tan (National University of Singapore), Jinhui Tang (National University of Singapore)
Influence maximization, whose objective is to select $k$ users (called seeds) from a social network such that the number of users influenced by the seeds (called influence spread) is maximized, has attracted significant attention from both the academic and industrial communities, due to its widespread applications, such as viral marketing and rumor control. However, in real-world social networks, users have their own interests (which can be represented as topics) and are more likely to be influenced by their friends (or friends' friends) with similar topics. We can increase the influence spread by taking into consideration topics in influence maximization. To address this problem, we study topic-aware influence maximization, which, given a topic-aware influence maximization (\timlgl) query, finds $k$ seeds from a social network such that the topic-aware influence spread of the $k$ seeds is maximized. Our goal is to enable online \timlgl queries. Since the topic-aware influence maximization problem is NP-hard and computing the topic-aware influence spread is \#P-hard, we focus on devising efficient algorithms to achieve instant performance while keeping a high influence spread. We first propose a best-effort algorithm with $1-\frac{1}{e}$ approximation ratio, which estimates an upper bound of the topic-aware influence of each user and utilizes the bound to prune a large number of users with small influence. We devise effective techniques to estimate tighter upper bounds. We then propose a faster topic-sample-based algorithm with $\epsilon\cdot (1-\frac{1}{e})$ approximation ratio for any $\epsilon\in(0,1]$, which materializes the influence spread of some topic-distribution samples and utilizes the materialized information to avoid computing the actual influence of users with small influences. Experimental results on real-world datasets show that our methods significantly outperform baseline approaches in efficiency while keeping nearly the same influence spread.


Walk, Not Wait: Faster Sampling Over Online Social Networks
Azade Nazi (University of Texas at Arlington), Zhuojie Zhou (George Washington University), Saravanan Thirumuruganathan (University of Texas at Arlingt), Nan Zhang (George Washington University), Gautam Das (University of Texas at Arlington)
In this paper, we introduce a novel, general purpose, technique for faster sampling of nodes over an online social network. Specifically, unlike traditional random walks which wait for the convergence of sampling distribution to a predetermined target distribution - a waiting process that incurs a high query cost - we develop WALK-ESTIMATE, which starts with a much shorter random walk, and then proactively estimate the sampling probability for the node taken before using acceptance-rejection sampling to adjust the sampling probability to the predetermined target distribution. We present a novel backward random walk technique which provides provably unbiased estimations for the sampling probability, and demonstrate the superiority of WALK-ESTIMATE over traditional random walks through theoretical analysis and extensive experiments over real world online social networks.
Research 12: Query Processing 1
Location: Queens 5
Chair: Dan Olteanu


Work-Efficient Parallel Skyline Computation for the GPU
Kenneth Bøgh (Århus Universitet), Sean Chester (Århus Universitet), Ira Assent (Århus Universitet)
The skyline operator returns records in a dataset that provide optimal trade-offs of multiple dimensions. State-of-the-art skyline computation involves complex tree traversals, data-ordering, and conditional branching to minimize the number of point-to-point comparisons. Meanwhile, GPGPU computing offers the potential for parallelizing skyline computation across thousands of cores. However, attempts to port skyline algorithms to the GPU have prioritized throughput and failed to outperform sequential algorithms. In this paper, we introduce a new skyline algorithm, designed for the GPU, that uses a global, static partitioning scheme. With the partitioning, we can permit controlled branching to exploit transitive relationships and avoid most point-to-point comparisons. The result is a non-traditional GPU algorithm, SkyAlign, that prioritizes work-efficiency and respectable throughput, rather than maximal throughput, to achieve orders of magnitude faster performance.


Memory-Efficient Hash Joins
Gopi Attaluri (IBM Software Group), Ronald Barber (IBM Research-Almaden), Naresh Chainani (IBM Software Group), Sam Lightstone (IBM Software Group), Guy Lohman (IBM Research-Almaden), Ippokratis Pandis (IBM Research-Almaden), Vijayshankar Raman (IBM Research-Almaden), Dave Sharpe (IBM Software Group), Richard Sidle (IBM Research-Almaden)
We present new hash tables for joins, and a hash join based on these, that consumes far less memory and is usually faster than recently published in-memory joins. Our hash join is not restricted to outer tables that fit wholly in memory. Key to this hash join is a new concise hash table (CHT), a linear probing hash table that has 100% fill factor, and uses a sparse bitmap with embedded population counts to almost entirely avoid collisions. This bitmap also serves as a Bloom filter for use in multi-table joins. We study the random access characteristics of hash joins, and renew the case for non-partitioned hash joins. We introduce a variant of partitioned joins in which only the build is partitioned, but the probe is not, as this is more efficient for large outer tables than traditional partitioned joins. This also avoids partitioning costs during the probe, while at the same time allowing parallel build without latching overheads. Additionally, we present a variant of CHT, called a concise array table (CAT), that can be used when the key domain is moderately dense. CAT is collision-free and avoids storing join keys in the hash table. We perform a detailed comparison of CHT and CAT against leading in-memory hash joins. Our experiments show that we can reduce the memory usage by one to three orders of magnitude, while also being competitive in performance.


MRCSI: Compressing and Searching String Collections with Multiple References
Sebastian Wandelt (HU Berlin), Ulf Leser (HU Berlin)
Efficiently storing and searching collections of similar strings, such as large populations of genomes or long change histories of documents from Wikis, is a timely and challenging problem. Several recent proposals could drastically reduce space requirements by exploiting the similarity between strings in so-called reference-based compression. However, these indexes are usually not searchable any more, i.e., in these methods search efficiency is sacrificed for storage efficiency. We propose Multi-Reference Compressed Search Indexes (MRCSI) as a framework for efficiently compressing dissimilar string collections. In contrast to previous works which can use only a single reference for compression, MRCSI (a) uses multiple references for achieving increased compression rates, where the reference set need not be specified by the user but is determined automatically, and (b) supports efficient approximate string searching with edit distance constraints. We prove that finding the smallest MRCSI is NP-hard. We then propose three heuristics for computing MRCSIs achieving increasing compression ratios. Compared to state-of-the-art competitors, our methods target an interesting and novel sweet-spot between high compression ratio versus search efficiency.


Trill: A High-Performance Incremental Query Processor for Diverse Analytics
Badrish Chandramouli (Microsoft Research), Jonathan Goldstein (Microsoft Research), Mike Barnett (Microsoft Research), Robert DeLine (Microsoft Research), Danyel Fisher (Microsoft Research), John Platt (Microsoft Research), James Terwilliger (Microsoft Research), John Wernsing (Microsoft Research)
This paper introduces Trill – a new query processor for analytics. Trill fulfills a combination of three requirements for a query processor to serve the diverse big data analytics space: (1) Query Model: Trill is based on a tempo-relational model that enables it to handle streaming and relational queries with early results, across the latency spectrum from real-time to offline; (2) Fabric and Language Integration: Trill is architected as a high-level language library that supports rich data-types and user libraries, and integrates well with existing distribution fabrics and applications; and (3) Performance: Trill’s throughput is high across the latency spectrum. For streaming data, Trill’s throughput is 2-4 orders of magnitude higher than comparable streaming engines. For offline relational queries, Trill’s throughput is comparable to a major modern commercial columnar DBMS. Trill uses a streaming batched-columnar data representation with a new dynamic compilation-based system architecture that addresses all these requirements. In this paper, we describe Trill’s new design and architecture, and report experimental results that demonstrate Trill’s high performance across diverse analytics scenarios. We also describe how Trill’s ability to support diverse analytics has resulted in its adoption across many usage scenarios at Microsoft.


Rapid Sampling for Visualizations with Ordering Guarantees
Albert Kim (MIT), Eric Blais (MIT), Aditya Parameswaran (MIT and U Illinois (UIUC)), Piotr Indyk (MIT), Sam Madden (MIT), Ronitt Rubinfeld (MIT and Tel Aviv University)
Visualizations are frequently used as a means to understand trends and gather insights from datasets, but often take a long time to generate. In this paper, we focus on the problem of rapidly generating approximate visualizations while preserving crucial visual proper- ties of interest to analysts. Our primary focus will be on sampling algorithms that preserve the visual property of ordering; our techniques will also apply to some other visual properties. For instance, our algorithms can be used to generate an approximate visualization of a bar chart very rapidly, where the comparisons between any two bars are correct. We formally show that our sampling algorithms are generally applicable and provably optimal in theory, in that they do not take more samples than necessary to generate the visualizations with ordering guarantees. They also work well in practice, correctly ordering output groups while taking orders of magnitude fewer samples and much less time than conventional sampling schemes.
Tutorial 3: Structured Analytics in Social Media
Location: Queens 6


Structured Analytics in Social Media
Mahashweta Das, Gautam Das
The rise of social media has turned the Web into an online community where people connect, communicate, and collaborate with each other. Structured analytics in social media is the process of discovering the structure of the relationships emerging from this social media use. It focuses on identifying the users involved, the activities they undertake, the actions they perform, and the items (e.g., movies, restaurants, blogs, etc.) they create and interact with. There are two key challenges facing these tasks: how to organize and model social media content, which is often unstructured in its raw form, in order to employ structured analytics on it; and how to employ analytics algorithms to capture both explicit link-based relationships and implicit behavior-based relationships. In this tutorial, we systemize and summarize the research so far in analyzing social interactions between users and items in the Web from data mining and database perspectives. We start with a general overview of the topic, including discourse to various exciting and practical applications. Then, we discuss the state-of-art for modeling the data, formalizing the mining task, developing the algorithmic solutions, and evaluating on real datasets. We also emphasize open problems and challenges for future research in the area of structured analytics and social media.
Demo 3: Systems, User Interfaces, and Visualization
Location: Kona 4
Chair: Tianli Mo


FP-Hadoop: Efficient Execution of Parallel Jobs Over Skewed Data
Miguel Liroz-Gistau (INRIA), Reza Akbarinia (INRIA), Patrick Valduriez (INRIA)
Big data parallel frameworks, such as MapReduce or Spark have been praised for their high scalability and performance, but show poor performance in the case of data skew. There are important cases where a high percentage of processing in the reduce side ends up being done by only one node. In this demonstration, we illustrate the use of FP-Hadoop, a system that efficiently deals with data skew in MapReduce jobs. In FP-Hadoop, there is a new phase, called inter- mediate reduce (IR), in which blocks of intermediate values, constructed dynamically, are processed by intermediate reduce workers in parallel, by using a scheduling strategy. Within the IR phase, even if all intermediate values belong to only one key, the main part of the reducing work can be done in parallel using the computing resources of all available workers. We implemented a prototype of FP-Hadoop, and conducted extensive experiments over synthetic and real datasets. We achieve excellent performance gains compared to native Hadoop, e.g. more than 10 times in reduce time and 5 times in total execution time. During our demonstration, we give the users the possibility to execute and compare job executions in FP-Hadoop and Hadoop. They can retrieve general information about the job and the tasks and a summary of the phases. They can also visually compare different configurations to explore the difference between the approaches.


SDB: A Secure Query Processing System with Data Interoperability
Zhian He (Hong Kong Polytechnic University), WaiKit Wong (Hang Seng Management College), Ben Kao (University of Hong Kong), David W. Cheung (University of Hong Kong), Rongbin Li (University of Hong Kong), Siu Ming Yiu (University of Hong Kong), Eric Lo (Polytecnic University of Hong Kong)
We address security issues in a cloud database system which em- ploys the DBaaS model — a data owner (DO) exports data to a cloud database service provider (SP). To provide data security, sensitive data is encrypted by the DO before it is uploaded to the SP. Compared to existing secure query processing systems like CryptDB [7] and MONOMI [8], in which data operations (e.g., comparison or addition) are supported by specialized encryption schemes, our demo system, SDB, is implemented based on a set of data- interoperable secure operators, i.e., the output of an operator can be used as input of another operator. As a result, SDB can sup- port a wide range of complex queries (e.g., all TPC-H queries) efficiently. In this demonstration, we show how our SDB prototype supports secure query processing on complex workload like TPC-H. We also demonstrate how our system protects sensitive in- formation from malicious attackers.


A Demonstration of HadoopViz: An Extensible MapReduce-based System for Visualizing Big Spatial Data
Ahmed Eldawy (University of Minnesota), Mohamed Mokbel (University of Minnesota), Christopher Jonathan (University of Minnesota)
This demonstration presents HadoopViz; an extensible MapReduce-based system for visualizing Big Spatial Data. HadoopViz has two main unique features that distinguish it from other techniques. (1) It provides an extensible interface that allows users to visualize various types of data by defining five abstract functions, without delving into the details of the MapReduce algorithms. We show how it is used to create four types of visualizations, namely, scatter plot, road network, frequency heat map, and temperature heat map. (2) HadoopViz is capable of generating big images with giga-pixel resolution by employing a three-phase approach of partitioning, rasterize, and merging. HadoopViz generates single and multi-level images, where the latter allows users to zoom in/out to get more/less details. Both types of images are generated with a very high resolution using the extensible and scalable framework of HadoopViz.


A Demonstration of the BigDAWG Polystore System
Aaron Elmore (MIT), Jennie Duggan (Northwestern), Michael Stonebraker (MIT), Manasi Vartak (MIT), Sam Madden (MIT), Vijay Gadepally (MIT), Jeremy Kepner (MIT), Timothy Mattson (Intel), Jeff Parhurst (Intel), Stavros Papadopoulos (MIT), Nesime Tatbul (Intel Labs and MIT), Magdalena Balazinska (Univsersity of Washington), Bill Howe (University of Washington), Jeffrey Heer (University of Washington), David Maier (Portland State University), Tim Kraska (Brown), Ugur Cetintemel (Brown University), Stan Zdonik (Brown University)
This paper presents BigDAWG, a reference implementation of a new architecture for “Big Data” applications. Such applications not only call for large-scale analytics, but also for real-time streaming support, smaller analytics at interactive speeds, data visualization, and cross-storage-system queries. Guided by the principle that “one size does not fit all”, we build on top of a variety of storage engines, each designed for a specialized use case. To illustrate the promise of this approach, we demonstrate its effective- ness on a hospital application using data from an intensive care unit (ICU). This complex application serves the needs of doctors and re- searchers and provides real-time support for streams of patient data. It showcases novel approaches for querying across multiple storage engines, data visualization, and scalable real-time analytics.


RINSE: Interactive Data Series Exploration with ADS+
Kostas Zoumpatianos (University of Trento), Stratos Idreos (Harvard), Themis Palpanas (Paris Descartes University)
Numerous applications continuously produce big amounts of data series, and in several time critical scenarios analysts need to be able to query these data as soon as they be- come available. An adaptive index data structure, ADS+, which is specifically tailored to solve the problem of index- ing and querying very large data series collections has been recently proposed as a solution to this problem. The main idea is that instead of building the complete index over the complete data set up-front and querying only later, we inter- actively and adaptively build parts of the index, only for the parts of the data on which the users pose queries. The net effect is that instead of waiting for extended periods of time for the index creation, users can immediately start exploring the data series. In this work, we present a demonstration of ADS+; we introduce RINSE, a system that allows users to experience the benefits of the ADS+ adaptive index through an intuitive web interface. Users can explore large datasets and find patterns of interest, using nearest neighbor search. They can draw queries (data series) using a mouse, or touch screen, or they can select from a predefined list of data se- ries. RINSE can scale to large data sizes, while drastically reducing the data to query delay: by the time state-of-the- art indexing techniques finish indexing 1 billion data series (and before answering even a single query), adaptive data series indexing can already answer 3 ∗ 105 queries.


Smart Drill-Down: A New Data Exploration Operator
Manas Joglekar (Stanford University), Hector Garcia-Molina (Stanford University), Aditya Parameswaran (University of Illinois at Urbana Champaign)
We present a data exploration system equipped with smart drill- down, a novel operator for interactively exploring a relational table to discover and summarize “interesting” groups of tuples. Each such group of tuples is represented by a rule. For instance, the rule (a, b, ⋆, 1000) tells us that there are a thousand tuples with value a in the first column and b in the second column (and any value in the third column). Smart drill-down presents an analyst with a list of rules that together describe interesting aspects of the table. The analyst can tailor the definition of interesting, and can interactively apply smart drill-down on an existing rule to explore that part of the table. In the demonstration, conference attendees will be able to use the data exploration system equipped with smart drill-down, and will be able to contrast smart drill-down to traditional drill-down, for various interestingness measures, and resource constraints.


VIIQ: auto-suggestion enabled visual interface for interactive graph query formulation
Nandish Jayaram (University of Texas at Arlingt), Sidharth Goyal (University of Texas at Arlington), Chengkai Li (University of Texas at Arlington)
We present VIIQ (pronounced as wick), an interactive and iterative visual query formulation interface that helps users construct query graphs specifying their exact query intent. Heterogeneous graphs are increasingly used to represent complex relationships in schema- less data, which are usually queried using query graphs. Existing graph query systems offer little help to users in easily choosing the exact labels of the edges and vertices in the query graph. VIIQ helps users easily specify their exact query intent by providing a visual interface that lets them graphically add various query graph com- ponents, backed by an edge suggestion mechanism that suggests edges relevant to the user’s query intent. In this demo we present: 1) a detailed description of the various features and user-friendly graphical interface of VIIQ, 2) a brief description of the edge sug- gestion algorithm, and 3) a demonstration scenario that we intend to show the audience.


VINERy: A Visual IDE for Information Extraction
Yunyao Li (IBM Research-Almaden), Elmer Kim (Treasuer Data (Inc.), Marc Touchette (IBM Silicon Valley Lab), Ramiya Venkatachalam (IBM Silicon Valley Lab), Hao Wang (IBM Silicon Valley Lab)
Information Extraction (IE) is the key technology enabling analytics over unstructured and semi-structured data. Not surprisingly, it is becoming a critical building block for a wide range of emerging applications. To satisfy the rising demands for information extraction in real-world applications, it is crucial to lower the barrier to entry for IE development and enable users with general computer science background to develop higher quality extractors. In this demonstration1, we present VINERY, an intuitive yet expressive visual IDE for information extraction. We show how it supports the full cycle of IE development without requiring a single line of code and enables a wide range of users to develop high quality IE extractors with minimal efforts. The extractors visually built in VINERY are automatically translated into semantically equivalent extractors in a state-of-the-art declarative language for IE. We also demonstrate how the auto-generated extractors can then be imported into a conventional Eclipse-based IDE for further enhancement. The results of our user studies indicate that VINERY is a significant step forward in facilitating extractor development for both expert and novice IE developers.


GIS navigation boosted by column stores
Foteini Alvanaki (CWI), Romulo Goncalves (Netherlands eScience Center), Milena Ivanova (NuoDB), Martin Kersten (CWI), Kostis Kyzirakos (CWI)
Earth observation sciences, astronomy, and seismology have large data sets which have inherently rich spatial and geospatial information. In combination with large collections of semantically rich objects which have a large number of thematic properties, they form a new source of knowledge for urban planning, smart cities and natural resource management. Modeling and storing these properties indicating the relationships between them is best handled in a relational database. Furthermore, the scalability requirements posed by the latest 26-attribute light detection and ranging (LI- DAR) data sets are a challenge for file-based solutions. In this demo we show how to query a 640 billion point data set using a column store enriched with GIS functionality. Through a lightweight and cache conscious secondary index called Imprints, spatial queries performance on a flat table storage is comparable to traditional file-based solutions. All the results are visualised in real time using QGIS.


AIDE: An Automatic User Navigation System for Interactive Data Exploration
Yanlei Diao (University of Massachusetts Amherst), Kyriaki Dimitriadou (Brandeis university), Zhan Li (Brandeis University), Wenzhao Liu (University of Massachusetts Amherst), Olga Papaemmanouil (Brandeis University), Kemi Peng (Brandeis University), Liping Peng (University of Massachusetts Amherst)
Data analysts often engage in data exploration tasks to discover interesting data patterns, without knowing exactly what they are looking for. Such exploration tasks can be very labor-intensive because they often require the user to review many results of ad-hoc queries and adjust the predicates of subsequent queries to balance the trade-off between collecting all interesting information and reducing the size of returned data. In this demonstration we introduce AIDE , a system that automates these exploration tasks. AIDE steers the user towards interesting data areas based on her relevance feedback on database samples, aiming to achieve the goal of identifying all database objects that match the user interest with high efficiency. In our demonstration, conference attendees will see AIDE in action for a variety of exploration tasks on real-world datasets.


A Demonstration of AQWA: Adaptive Query-Workload-Aware Partitioning of Big Spatial Data
Ahmed Aly (Purdue University), Ahmed Abdelhamid (Purdue University), Ahmed Mahmood,Purdue University), Walid Aref (Purdue University), Mohamed Hassan (Purdue University), Hazem Elmeleegy (Turn Inc), Mourad Ouzzani (Qatar Computing Research Institute)
The ubiquity of location-aware devices, e.g., smartphones and GPS devices, has led to a plethora of location-based services in which huge amounts of geotagged information need to be efficiently pro- cessed by large-scale computing clusters. This demo presents AQWA, an adaptive and query-workload-aware data partitioning mechanism for processing large-scale spatial data. Unlike existing cluster-based systems, e.g., SpatialHadoop, that apply static parti- tioning of spatial data, AQWA has the ability to react to changes in the query-workload and data distribution. A key feature of AQWA is that it does not assume prior knowledge of the query-workload or data distribution. Instead, AQWA reacts to changes in both the data and the query-workload by incrementally updating the partitioning of the data. We demonstrate two prototypes of AQWA deployed over Hadoop and Spark. In both prototypes, we process spatial range and k-nearest-neighbor (kNN, for short) queries over large- scale spatial datasets, and we exploit the performance of AQWA under different query-workloads.


DATASPREAD: Unifying Databases and Spreadsheets
Mangesh Bendre (University of Illinois at Urbana-Champaign), Bofan Sun (University of Illinois at Urbana-Champaign), Ding Zhang (University of Illinois at Urbana-Champaign), Xinyan Zhou (University of Illinois at Urbana-Champaign), Kevin Chang (University of Illinois at Urbana-Champaign), Aditya Parameswaran (University of Illinois at Urbana-Champaign)
Spreadsheet software is often the tool of choice for ad-hoc tabular data management, processing, and visualization, especially on tiny data sets. On the other hand, relational database systems offer significant power, expressivity, and efficiency over spreadsheet software for data management, while lacking in the ease of use and ad-hoc analysis capabilities. We demonstrate DATASPREAD, a data exploration tool that holistically unifies databases and spread- sheets. It continues to offer a Microsoft Excel-based spreadsheet front-end, while in parallel managing all the data in a back-end database, specifically, PostgreSQL. DATASPREAD retains all the advantages of spreadsheets, including ease of use, ad-hoc analysis and visualization capabilities, and a schema-free nature, while also adding the advantages of traditional relational databases, such as scalability and the ability to use arbitrary SQL to import, filter, or join external or internal tables and have the results appear in the spreadsheet. DATASPREAD needs to reason about and reconcile differences in the notions of schema, addressing of cells and tuples, and the current “pane” (which exists in spreadsheets but not in traditional databases), and support data modifications at both the front-end and the back-end. Our demonstration will center on our first and early prototype of the DATASPREAD, and will give the attendees a sense for the enormous data exploration capabilities offered by unifying spreadsheets and databases.

CODD: A Dataless Approach to Big Data Testing
Ashoke S (Indian Institute of Science), Jayant Haritsa (IISc)


Vizdom: Interactive Analytics through Pen and Touch
Andrew Crotty (Brown University), Alex Galakatos (Brown University), Emanuel Zgraggen (Brown University), Carsten Binnig (Brown University), Tim Kraska (Brown University)
Machine learning (ML) and advanced statistics are impor- tant tools for drawing insights from large datasets. How- ever, these techniques often require human intervention to steer computation towards meaningful results. In this demo, we present Vizdom, a new system for interactive analytics through pen and touch. Vizdom’s frontend allows users to visually compose complex workflows of ML and statis- tics operators on an interactive whiteboard, and the back- end leverages recent advances in workflow compilation tech- niques to run these computations at interactive speeds. Ad- ditionally, we are exploring approximation techniques for quickly visualizing partial results that incrementally refine over time. This demo will show Vizdom’s capabilities by allowing users to interactively build complex analytics work- flows using real-world datasets.


DBSeer: Pain-free Database Administration through Workload Intelligence
Dong Young Yoon (University of Michigan Ann Arbor), Barzan Mozafari (University of Michigan Ann Arbor),Douglas Brown (Teradata Inc.)
The pressing need for achieving and maintaining high performance in database systems has made database administration one of the most stressful jobs in information technology. On the other hand, the increasing complexity of database systems has made qualified database administrators (DBAs) a scarce resource. DBAs are now responsible for an array of demanding tasks; they need to (i) provi- sion and tune their database according to their application require- ments, (ii) constantly monitor their database for any performance failures or slowdowns, (iii) diagnose the root cause of the perfor- mance problem in an accurate and timely fashion, and (iv) take prompt actions that can restore acceptable database performance. However, much of the research in the past years has focused on improving the raw performance of the database systems, rather than improving their manageability. Besides sophisticated consoles for monitoring performance and a few auto-tuning wizards, DBAs are not provided with any help other than their own many years of experience. Typically, their only resort is trial-and-error, which is a tedious, ad-hoc and often sub-optimal solution. In this demonstration, we present DBSeer, a workload intelligence framework that exploits advanced machine learning and causal- ity techniques to aid DBAs in their various responsibilities. DBSeer analyzes large volumes of statistics and telemetry data collected from various log files to provide the DBA with a suite of rich functionalities including performance prediction, performance diagno- sis, bottleneck explanation, workload insight, optimal admission control, and what-if analysis. In this demo, we showcase various features of DBSeer by predicting and analyzing the performance of a live database system. Will also reproduce a number of realistic performance problems in the system, and allow the audience to use DBSeer to quickly diagnose and resolve their root cause.

Sharing and Reproducing Database Applications
Quan Pham (University of Chicago), Severin Thaler (University of Chicago), Tanu Malik (University of Chicago), Ian Foster (University of Chicago), Boris Glavic (IIT)
Wednesday Sep 2nd 12:00-13:30
Lunch
Location: Lagoon Lanai
Wednesday Sep 2nd 13:30-15:00
Research 13: Graph Processing 3
Location: Kings 1
Chair: Mohamed Mokbel


TOP: A Framework for Enabling Algorithmic Optimizations for Distance-Related Problems
Yufei Ding (North Carolina State University), Xipeng Shen (North Carolina State University), Madanlal Musuvathi (Microsoft Research), Todd Mytkowicz (Microsoft Research)
Computing distances among data points is an essential part of many important algorithms in data analytics, graph analysis, and other domains. In each of these domains, developers have spent significant manual effort optimizing algorithms, often through novel applications of the triangle equality, in order to minimize the number of distance computations in the algorithms. In this work, we observe that many algorithms across these domains can be generalized as an instance of a generic distance-related abstraction. Based on this abstraction, we derive seven principles for correctly applying the triangular inequality to optimize distance-related algorithms. Guided by the findings, we develop Triangular Optimizer (TOP), the first software framework that is able to automatically produce optimized algorithms that either matches or outperforms manually designed algorithms for solving distance-related problems. TOP achieves up to 237x speedups and 2.5X on average.


SCAN++: Efficient Algorithm for Finding Clusters, Hubs and Outliers on Large-scale Graphs
Hiroaki Shiokawa (NTT), Yasuhiro Fujiwara (NTT), Makoto Onizuka (Osaka University)
Graph clustering is one of the key techniques for understanding the structures present in graphs. Besides cluster detection, identifying hubs and outliers is also a key task, since they have important roles to play in graph data mining. The structural clustering algorithm SCAN, proposed by Xu et al., is successfully used in many application because it not only detects densely connected nodes as clusters but also identifies sparsely connected nodes as hubs or outliers. However, it is difficult to apply SCAN to large-scale graphs due to its high time complexity. This is because it evaluates the density for all adjacent nodes included in the given graphs. In this paper, we propose a novel graph clustering algorithm named SCAN++. In order to reduce time complexity, we introduce new data structure of directly two-hop-away reachable node set (DTAR). DTAR is the set of two-hop-away nodes from a given node that are likely to be in the same cluster as the given node. SCAN++ employs two approaches for efficient clustering by using DTARs without sacrificing clustering quality. First, it reduces the number of the density evaluations by computing the density only for the adjacent nodes such as indicated by DTARs. Second, by sharing a part of the density evaluations for DTARs, it offers efficient density evaluations of adjacent nodes. As a result, SCAN++ detects exactly the same clusters, hubs, and outliers from large-scale graphs as SCAN with much shorter computation time. Extensive experiments on both real-world and synthetic graphs demonstrate the performance superiority of SCAN++ over existing approaches.


GraphTwist: Fast Iterative Graph Computation with Two-tier Optimizations
Yang Zhou (Georgia Institute of Technolog), Ling Liu (Georgia Institute of Technology), Kisung Lee (Georgia Institute of Technology), Qi Zhang (Georgia Institute of Technology)
Large-scale real-world graphs are known to have highly skewed vertex degree distribution and highly skewed edge weight distribution. Existing vertex-centric iterative graph computation models suffer from a number of serious problems: (1) poor performance of parallel execution due to inherent workload imbalance at vertex level; (2) inefficient CPU resource utilization due to short execution time for low-degree vertices compared to the cost of in-memory or on-disk vertex access; and (3) incapability of pruning insignificant vertices or edges to improve the computational performance. In this paper, we address the above technical challenges by designing and implementing a scalable, efficient, and provably correct two-tier graph parallel processing system, GraphTwist. At storage and access tier, GraphTwist maximizes parallel efficiency by employing three graph parallel abstractions for partitioning a big graph by slice, strip or dice based partitioning techniques. At computation tier, GraphTwist presents two utility-aware pruning strategies: slice pruning and cut pruning, to further improve the computational performance while preserving the computational utility defined by graph applications. Theoretic analysis is provided to quantitatively prove that iterative graph computations powered by utility-aware pruning techniques can achieve a very good approximation with bounds on the introduced error.


A Scalable Distributed Graph Partitioner
Daniel Margo (Harvard University), Margo Seltzer (Harvard University)
We present Scalable Host-tree Embeddings for Efficient Partitioning (Sheep), a distributed graph partitioning algorithm capable of handling graphs that far exceed main memory. Sheep produces high quality edge partitions an order of magnitude faster than both state of the art offline (e.g., METIS) and streaming partitioners (e.g., Fennel). Sheep's partitions are independent of the input graph distribution, which means that graph elements can be assigned to processing nodes arbitrarily without affecting the partition quality. Sheep transforms the input graph into a strictly smaller elimination tree via a distributed map-reduce operation. By partitioning this tree, Sheep finds an upper-bounded communication volume partitioning of the original graph. We describe the Sheep algorithm and analyze its space-time requirements, partition quality, and intuitive characteristics and limitations. We compare Sheep to contemporary partitioners and demonstrate that Sheep creates competitive partitions, scales to larger graphs, and has better runtime.


Keys for Graphs
Wenfei Fan (University of Edinburgh), Zhe Fan (University of Edinburgh), Chao Tian (University of Edinburgh), Xin Luna Dong (Google Inc)
Keys for graphs aim to uniquely identify entities represented by vertices in a graph. We propose a class of keys that are recursively defined in terms of graph patterns, and are interpreted with subgraph isomorphism. Extending conventional keys for relations and XML, these keys find applications in object identification, knowledge fusion and social network reconciliation. As an application, we study the entity matching problem that, given a graph G and a set \Sigma of keys, is to find all pairs of entities (vertices) in G that are identified by keys in \Sigma. We show that the problem is intractable, and cannot be parallelized in logarithmic rounds. Nonetheless, we provide two parallel scalable algorithms for entity matching, in MapReduce and a vertex-centric asynchronous model. Using real-life and synthetic data, we experimentally verify the effectiveness and scalability of the algorithms.
Research 14: Novel Hardware Architectures
Location: Kings 2
Chair: Paul Larson


Scaling Up Concurrent Main-Memory Column-Store Scans: Towards Adaptive NUMA-aware Data and Task Placement
Iraklis Psaroudakis (EPFL), Tobias Scheuer (SAP SE), Norman May (SAP AG), Abdelkader Sellami (SAP SE), Anastassia Ailamaki (EPFL)
Main-memory column-stores are called to efficiently use modern non-uniform memory access (NUMA) architectures to service a high number of clients on big data. The efficient usage of NUMA architectures depends on the data placement and scheduling strategy of the column-store. The majority of column-stores chooses a static strategy that typically involves partitioning all data across the NUMA architecture, and employing a stealing-based task scheduler. In this paper, we identify and implement different alternative strategies for data placement and task scheduling for the case of concurrent scans. We compare these strategies with an extensive sensitivity analysis and quantify their trade-offs. Our most significant findings include that unnecessary partitioning can hurt throughput by up to 70%, and that stealing tasks can hurt the throughput of memory-intensive workloads by up to 58%. Based on the implications of our analysis, we envision a design that can adapt the data placement and task scheduling strategy to the workload.


In-Memory Performance for Big Data
Goetz Graefe (HP Labs), Haris Volos (HP Labs), Hideaki Kimura (HP Labs), Harumi Kuno (HP Labs), Joseph Tucek (HP Labs), Mark Lillibridge (HP Labs), Alistair Veitch (Google)
When a working set fits into memory, the overhead imposed by the buffer pool renders traditional databases non-competitive with in-memory designs that sacrifice the benefits of a buffer pool. However, despite the large memory available with modern hardware, data skew, shifting workloads, and complex mixed workloads make it difficult to guarantee that a working set will fit in memory. Hence, some recent work has focused on enabling in-memory databases to protect performance when the working data set almost fits in memory. Contrary to those prior efforts, we enable buffer pool designs to match in-memory performance while supporting the "big data" workloads that continue to require secondary storage, thus providing the best of both worlds. We introduce here a novel buffer pool design that adapts pointer swizzling for references between system objects (as opposed to application objects), and uses it to practically eliminate buffer pool overheads for memory-resident data. Our implementation and experimental evaluation demonstrate that we achieve graceful performance degradation when the working set grows to exceed the buffer pool size, and graceful improvement when the working set shrinks towards and below the memory and buffer pool sizes.


Profiling R on a Contemporary Processor
Shriram Sridharan (University of Wisconsin-Madison), Jignesh Patel (University of Wisconsin-Madison)
R is a popular data analysis language, but there is scant experimental data characterizing the run-time profile of R programs. This paper addresses this limitation by systematically cataloging where time is spent when running R programs. Our evaluation using four different workloads shows that when analyzing large datasets, R programs a) spend more than 85% of their time in processor stalls, which leads to slower execution times, b) trigger the garbage collector frequently, which leads to higher memory stalls, and c) create a large number of unnecessary temporary objects that causes R to swap to disk quickly even for datasets that are far smaller than the available main memory. Addressing these issues should allow R programs to run faster than they do today, and allow R to be used for analyzing even larger datasets. As outlined in this paper, the results presented in this paper motivate a number of future research investigations in the database, architecture, and programming language communities. All data and code that is used in this paper (which includes the R programs, and changes to the R source code for instrumentation) can be found at: http://quickstep.cs.wisc.edu/dissecting-R/.


Deployment of Query Plans on Multicores
Jana Giceva (ETH Zurich), Gustavo Alonso (ETH Zurich), Timothy Roscoe (ETH Zurich), Tim Harris (Oracle labs)
Efficient resource scheduling of multithreaded software on multicore hardware is difficult given the many parameters involved and the hardware heterogeneity of existing systems. In this paper we explore the efficient deployment of query plans over a multicore machine. We focus on shared query systems, and implement the proposed ideas using SharedDB. The goal of the paper is to explore how to deliver maximum performance and predictability, while minimizing resource utilization when deploying query plans on multicore machines. We propose to use resource activity vectors to characterize the behavior of individual database operators. We then present a novel deployment algorithm which uses these vectors together with dataflow information from the query plan to optimally assign relational operators to physical cores. Experiments demonstrate that this approach significantly reduces resource requirements while preserving performance and is robust across different server architectures.


Faster Set Intersection with SIMD instructions by Reducing Branch Mispredictions
Hiroshi Inoue (IBM Research-Tokyo and University of Tokyo), Moriyoshi Ohara (IBM Research-Tokyo), Kenjiro Taura (University of Tokyo)
Set intersection is one of the most important operations for many applications such as Web search engines or database management systems. This paper describes our new algorithm to efficiently find set intersections with sorted arrays on modern processors with SIMD instructions and high branch misprediction penalties. Our algorithm efficiently exploits SIMD instructions and can drastically reduce branch mispredictions. Our algorithm extends a merge-based algorithm by reading multiple elements, instead of just one element, from each of two input arrays and compares all of the pairs of elements from the two arrays to find the elements with the same values. The key insight for our improvement is that we can reduce the number of costly hard-to-predict conditional branches by advancing a pointer by more than one element at a time. Although this algorithm increases the total number of comparisons, we can execute these comparisons more efficiently using the SIMD instructions and gain the benefits of the reduced branch misprediction overhead. Our algorithm is suitable to replace existing standard library functions, such as std::set_intersection in C++, thus accelerating many applications, because the algorithm is simple and requires no preprocessing to generate additional data structures. We implemented our algorithm on Xeon and POWER7+. The experimental results show our algorithm outperforms the std::set_intersection implementation delivered with gcc by up to 5.2x using SIMD instructions and by up to 2.1x even without using SIMD instructions for 32-bit and 64-bit integer datasets. Our SIMD algorithm also outperformed an existing algorithm that can leverage SIMD instructions.
Industrial 4: Novel Approaches to Modern Data Processing
Location: Kings 3
Chair: John Cieslewicz


The Dataflow Model: A Practical Approach to Balancing Correctness, Latency, and Cost in Massive-Scale, Unbounded, Out-of-Order Data Processing
Tyler Akidau (Google), Robert Bradshaw (Google), Craig Chambers (Google), Slava Chernyak (Google), Rafael Fernández-Moctezuma (Google), Reuven Lax (Google), Sam McVeety (Google), Daniel Mills (Google), Frances Perry (Google), Eric Schmidt (Google), Sam Whittle (Google)
Unbounded, unordered, global-scale datasets are increasingly common in day-to-day business (e.g. Web logs, mobile usage statistics, and sensor networks). At the same time, consumers of these datasets have evolved sophisticated requirements, such as event-time ordering and windowing by features of the data themselves, in addition to an insatiable hunger for faster answers. Meanwhile, practicality dictates that one can never fully optimize along all dimensions of correctness, latency, and cost for these types of input. As a result, data processing practitioners are left with the quandary of how to reconcile the tensions between these seemingly competing propositions, often resulting in disparate implementations and systems. We propose that a fundamental shift of approach is necessary to deal with these evolved requirements in modern data processing. We as a field must stop trying to groom unbounded datasets into finite pools of information that eventually become complete, and instead live and breathe under the assumption that we will never know if or when we have seen all of our data, only that new data will arrive, old data may be retracted, and the only way to make this problem tractable is via principled abstractions that allow the practitioner the choice of appropriate tradeoffs along the axes of interest: correctness, latency, and cost. In this paper, we present one such approach, the Dataflow Model, along with a detailed examination of the semantics it enables, an overview of the core principles that guided its design, and a validation of the model itself via the real-world experiences that led to its development.


Live Programming Support in the LogicBlox System: A MetaLogiQL Approach
Todd Green (LogicBlox Inc.), Dan Olteanu (LogicBlox Inc.), Geoffrey Washburn (LogicBlox Inc.)
The emerging category of self-service enterprise applications motivates support for ``live programming'' in the database, where the user's iterative data exploration triggers changes to installed application code and its output in real time. This paper discusses the technical challenges in supporting live programming in the database and presents the solution implemented in the LogicBlox commercial system. The workhorse architectural component is a ``meta-engine'' that incrementally maintains metadata representing application code, guides its compilation into an internal representation in the database kernel, and orchestrates maintenance of materialized views based on those changes. Our approach mirrors LogicBlox's declarative programming model and describes the maintenance of application code using declarative meta-rules; the meta-engine is essentially a ``bootstrap'' version of the database engine proper. Beyond live programming, the meta-engine turns out effective for a range of static analysis and optimization tasks. Outside of the database context, we speculate that our design may even provide a novel means of building incremental compilers for general-purpose programming languages.


Indexing and Selecting Hierarchical Business Logic
Alessandra Loro (Palantir Technologies), Anja Gruenheid (ETH Zurich), Donald Kossman (ETH Zurich and Microsoft Research), Damien Profeta (S.A.S. Amadeus), Philippe Beaudequin (S.A.S. Amadeus)
Business rule management is the task of storing and maintaining company-specific decision rules and business logic that is queried frequently by application users. These rules can impede efficient query processing when they require the business rule engine to resolve semantic hierarchies. To address this problem, this work discusses hierarchical indexes that are performance and storage-conscious. In the first part of this work, we develop a tree-based hierarchical structure that represents client-defined semantic hierarchies as well as two variants of this structure that improve performance and main memory allocation. The second part of our work focuses on selecting the top rules out of those retrieved from the index. We formally define a priority score-based decision scheme that allows for a conflict-free rule system and efficient rule ranking. Additionally, we introduce a weight-based lazy merging technique for rule selection. All of these techniques are evaluated with real world and synthetic data sets
Research 15: Query Optimization
Location: Queens 4
Chair: Jonathan Goldstein


Resource Bricolage for Parallel Database Systems
Jiexing Li (Google Inc), Jeffrey Naughton (University of Wisconsin-Madison), Rimma Nehme (Microsoft Jim Gray Systems Lab)
Running parallel database systems in an environment with heterogeneous resources has become increasingly common, due to cluster evolution and increasing interest in moving applications into public clouds. For database systems running in a heterogeneous cluster, the default uniform data partitioning strategy may overload some of the slow machines while at the same time it may under-utilize the more powerful machines. Since the processing time of a parallel query is determined by the slowest machine, such an allocation strategy may result in a significant query performance degradation. We take a first step to address this problem by introducing a technique we call resource bricolage that improves database performance in heterogeneous environments. Our approach quantifies the performance differences among machines with various resources as they process workloads with diverse resource requirements. We formalize the problem of minimizing workload execution time and view it as an optimization problem, and then we employ linear programming to obtain a recommended data partitioning scheme. We verify the effectiveness of our technique with an extensive experimental study on a commercial database system.


Multi-Objective Parametric Query Optimization
Immanuel Trummer (EPFL), Christoph Koch (EPFL)
Classical query optimization compares query plans according to one cost metric and associates each plan with a constant cost value. In this paper, we introduce the Multi-Objective Parametric Query Optimization (MPQ) problem where query plans are compared according to multiple cost metrics and the cost of a given plan according to a given metric is modeled as a function that depends on multiple parameters. The cost metrics may for instance include execution time or monetary fees; a parameter may represent the selectivity of a query predicate that is unspecified at optimization time. MPQ generalizes parametric query optimization (which allows multiple parameters but only one cost metric) and multi-objective query optimization (which allows multiple cost metrics but no parameters). We formally analyze the novel MPQ problem and show why existing algorithms are inapplicable. We present a generic algorithm for MPQ and a specialized version for MPQ with piecewise-linear plan cost functions. We prove that both algorithms find all relevant query plans and experimentally evaluate the performance of our second algorithm in a Cloud computing scenario.


Querying with Access Patterns and Integrity Constraints
Michael Benedikt (Oxford University), Julien Leblay (Oxford University), Efi Tsamoura (Oxford University)
Traditional query processing involves a search for plans formed by applying algebraic operators on top of primitives representing access to relations in the input query. But many querying scenarios involve two interacting issues that complicate the search. On the one hand, the search space may be limited by access restrictions associated with the interfaces to datasources, which require certain parameters to be given as inputs. On the other hand, the search space may be extended through the presence of integrity constraints that relate sources to each other, allowing for plans that do not match the structure of the user query. In this paper we present the first optimization approach that attacks both these difficulties within a single framework, presenting a system in which classical cost-based join optimization is extended to support both access-restrictions and constraints. Instead of iteratively exploring subqueries of the input query, our optimizer explores a space of proofs that witness the answering of the query, where each proof has a direct correspondence with a query plan.


Uncertainty Aware Query Execution Time Prediction
Wentao Wu (University of Wisconsin-Madison), Xi Wu (University of Wisconsin-Madison), Hakan Hacigumus (NEC Labs America), Jeffrey Naughton (University of Wisconsin-Madison)
Predicting query execution time is a fundamental issue underlying many database management tasks. Existing predictors rely on information such as cardinality estimates and system performance constants that are difficult to know exactly. As a result, accurate prediction still remains elusive for many queries. However, existing predictors provide a single, point estimate of the true execution time, but fail to characterize the uncertainty in the prediction. In this paper, we take a first step towards providing uncertainty information along with query execution time predictions. We use the query optimizer's cost model to represent the query execution time as a function of the selectivities of operators in the query plan as well as the constants that describe the cost of CPU and I/O operations in the system. By treating these quantities as random variables rather than constants, we show that with low overhead we can infer the distribution of likely prediction errors. We further show that the estimated prediction errors by our proposed techniques are strongly correlated with the actual prediction errors.


Join Size Estimation Subject to Filter Conditions
David Vengerov (Oracle Labs), Andre Menck (Oracle Corp.), Mohamed Zait (Oracle Corp),Sunil Chakkappen (Oracle Corp)
In this paper, we present a new algorithm for estimating the size of equality join of multiple database tables. The proposed algorithm, Correlated Sampling, constructs a small space synopsis for each table, which can then be used to provide a quick estimate of the join size of this table with other tables subject to dynamically specified predicate filter conditions, possibly specified over multiple columns (attributes) of each table. This algorithm makes a single pass over the data and is thus suitable for streaming scenarios. We compare this algorithm analytically to two other previously known sampling approaches (independent Bernoulli Sampling and End-Biased Sampling) and to a novel sketch-based approach. We also compare these four algorithms experimentally and show that results fully correspond to our analytical predictions based on derived expressions for the estimator variances, with Correlated Sampling giving the best estimates in a large range of situations.
Research 16: Crowdsourcing and Social Network Analysis
Location: Queens 5
Chair: Yi Chen


Scaling Up Crowd-Sourcing to Very Large Datasets: A Case for Active Learning
Barzan Mozafari (University of Michigan), Purna Sarkar (UC Berkeley), Michael Franklin (UC Berkeley), Michael Jordan (UC Berkeley), Sam Madden (MIT)
Crowd-sourcing has become a popular means of acquiring labeled data for many tasks where humans are more accurate than computers, such as image tagging, entity resolution, and sentiment analysis. However, due to the time and cost of human labor, solutions that rely solely on crowd-sourcing are often limited to small datasets (i.e., a few thousand items). This paper proposes algorithms for integrating machine learning into crowd-sourced databases in order to combine the accuracy of human labeling with the speed and cost-effectiveness of machine learning classifiers. By using active learning as our optimization strategy for labeling tasks in crowd-sourced databases, we can minimize the number of questions asked to the crowd, allowing crowd-sourced applications to scale (i.e., label much larger datasets at lower costs). Designing active learning algorithms for a crowd-sourced database poses many practical challenges: such algorithms need to be generic, scalable, and easy to use, even for practitioners who are not machine learning experts. We draw on the theory of nonparametric bootstrap to design, to the best of our knowledge, the first active learning algorithms that meet all these requirements. Our results, on 3 real-world datasets collected with Amazon’s Mechanical Turk, and on 15 UCI datasets, show that our methods on average ask 1-2 orders of magnitude fewer questions than the baseline, and 4.5-44x fewer than existing active learning algorithms.


Hear the Whole Story: Towards the Diversity of Opinion in Crowdsourcing Markets
Ting Wu (Hong Kong University of Science and Technology), Lei Chen (Hong Kong University of Science and Technology), Pan Hui (Hong Kong University of Science and Technology), CHEN ZHANG (Hong Kong University of Science and Technology), Weikai Li (Hong Kong University of Science and Technology)
Recently, the popularity of crowdsourcing has brought a new opportunity for engaging human intelligence in the process of data analysis. Crowdsourcing provides a fundamental mechanism for enabling online workers to participate tasks that are either too difficult to be solved solely by computers or too expensive to employ experts to perform. In the field of social science, four elements are required to form a wise crowd - Diversity of Opinion, Independence, Decentralization and Aggregation. However, while the other three elements are already studied and implemented in current crowdsourcing platforms, the `Diversity of Opinion' has not been functionally enabled. In this paper, we address the algorithmic optimizations towards the \textit{diversity of opinion} of crowdsourcing marketplaces. From a computational perspective, in order to build a wise crowd, we are interested in quantitatively modeling the diversity, and take it into consideration for constructing a crowd. In a crowdsourcing marketplace, we usually encounter two basic paradigms for worker selection: selecting workers for a given task ; and building a crowd waiting for tasks to come. As results, we propose Task-driven Model (T-Model) and Similarity-driven (S-Model) for both of the paradigms. Under both of the models, we propose efficient and effective algorithms to enlist a budgeted number of workers, and maximize the diversity. We have verified the solutions with extensive experiments on both synthetic datasets and real data sets.


Where To: Crowd-Aided Path Selection
Chen Zhang (Hong Kong University of Science and Technology), Yongxin Tong (Hong Kong University of Science and Technology), Lei Chen (Hong Kong University of Science and Technology)
With the widespread use of geo-positioning services (GPS), GPS-based navigation systems have become ever more of an integral part of our daily lives. GPS-based navigation systems usually suggest multiple paths for any given pair of source and target, leaving users perplexed when trying to select the best one among them, namely the problem of \textit{best path selection}. Too many suggested paths may jeopardize the usability of the recommendation data, and decrease user satisfaction. Although existing studies have already partially relieved this problem through integrating historical traffic logs or updating traffic conditions periodically, their solutions neglect the potential contribution of human experience. In this paper, we resort to crowdsourcing to ease the pain of the best path selection. The first step of appropriately using the crowd is to ask proper questions. For the best path selection problem, simple questions (e.g. binary voting) over compete paths cannot be directly applied to road networks due to their being too complex for crowd workers. Thus, this paper makes the first contribution by designing two types of questions, namely Routing Query (RQ) and Binary Routing Query (BRQ), to ask the crowd to decide which direction to take at each road intersection. Furthermore, we propose a series of efficient algorithms to dynamically manage the questions in order to reduce the selection hardness within a limited budget. Finally, we compare the proposed methods against two baselines, and the effectiveness and efficiency of our proposals are verified by the results from simulations and experiments on a real-world crowdsourcing platform.


Reliable Diversity-Based Spatial Crowdsourcing by Moving Workers
Peng Cheng (Hong Kong University of Science and Technology), Xiang Lian (University of Texas Rio Grande Valley), Zhao Chen (Hong Kong University of Science and Technology), Rui Fu (Hong Kong University of Science and Technology), Lei Chen (Hong Kong University of Science and Technology), Jinsong Han (Xi'an Jiaotong University), Jizhong Zhao (Xi'an Jiaotong University)
With the rapid development of mobile devices and the crowdsourcig platforms, the spatial crowdsourcing has attracted much attention from the database community, specifically, spatial crowdsourcing refers to sending a location-based request to workers according to their positions. In this paper, we consider an important spatial crowdsourcing problem, namely reliable diversity-based spatial crowdsourcing (RDB-SC), in which spatial tasks (such as taking videos/photos of a landmark or firework shows, and checking whether or not parking spaces are available) are time-constrained, and workers are moving towards some directions. Our RDB-SC problem is to assign workers to spatial tasks such that the completion reliability and the spatial/temporal diversities of spatial tasks are maximized. We prove that the RDB-SC problem is NP-hard and intractable. Thus, we propose three effective approximation approaches, including greedy, sampling, and divide-and-conquer algorithms. In order to improve the efficiency, we also design an effective cost-model-based index, which can dynamically maintain moving workers and spatial tasks with low cost, and efficiently facilitate the retrieval of RDB-SC answers. Through extensive experiments, we demonstrate the efficiency and effectiveness of our proposed approaches over both real and synthetic data sets.


Learning User Preferences By Adaptive Pairwise Comparison
Li Qian (Facebook), Jinyang Gao (National University of Singapo), H V Jagadish (University of Michigan Ann Arbor)
Users make choices among multi-attribute objects in a data set in a variety of domains including used car purchase, job search and hotel room booking. Individual users sometimes have strong preferences between objects, but these preferences may not be universally shared by all users. If we can cast these preferences as derived from a quantitative user-specific preference function, then we can predict user preferences by learning their preference function, even though the preference function itself is not directly observable, and may be hard to express. In this paper we study the problem of quantitative preference learning with pairwise comparisons on a set of entities with multiple attributes. We formalize the problem into two subproblems, namely preference estimation and comparison selection. We propose an innovative approach to estimate the preference, and introduce a binary search strategy to adaptively select the comparisons. We introduce the concept of an orthogonal query to support this adaptive selection, as well as a novel S-tree index to enable efficient evaluation of orthogonal queries. We integrate these components into a system for inferring user preference with adaptive pairwise comparisons. Our experiments and user study demonstrate that our adaptive system significantly outperforms the naive random selection system on both real data and synthetic data, with either simulated or real user feedback. We also show our preference learning approach is much more effective than existing approaches, and our S-tree can be constructed efficiently and perform orthogonal query at interactive speeds.
Tutorial 4: SQL-on-Hadoop Systems (1/2)
Location: Queens 6


SQL-on-Hadoop Systems (1/2)
Daniel Abadi, Shivnath Babu, Fatma Ozcan, Ippokratis Pandis
In this tutorial, we will examine the SQL-on-Hadoop systems along various dimensions. One important aspect is their data storage. Some of these systems support all native Hadoop formats, and do not impose any propriety data formats, and keep the data open to all applications running on the same platform. While there are some database hybrid solutions, such as HAWQ, HP Haven, and Vortex, that store their propriety data formats in HDFS. Most often, these systems are also able to run SQL queries over native HDFS formats, but do not provide the same level of performance. Some SQL-on-Hadoop systems provide their own SQL-specific run-times, such as Impala, Big SQL, and Presto, while others exploit a general purpose run-time such as Hive (MapReduce and Tez) and SparkSQL (Spark). Another important aspect is the support for schema flexibility and complex data types. Almost all of these systems support complex data types, such as arrays and structs. But, only a few, such as Drill and Hadapt with Sinew [13], are able to work with schemaless data.
Panel 1: 40-years VLDB
Location: Kona 1-2-3
Chair: Pat Selinger

40-years VLDB
Phil Bernstein (Microsoft),Michael Brodie (MIT and retired Chief Scientist Verizon IT),Don Chamberlin (retired IBM Fellow),Alfons Kemper (Technical University Munich),Michael Stonebraker (MIT and serial entrepreneur), Pat Selinger (Paradata)
In this panel, we will sweep across 40 years of VLDB with stories and anecdotes about people and technology, the amazing adoption of relational databases, what we focused on and thought important, compared to what really turned out to be important? What was it like to be in on the birth of the field? When did we realize that this could be something big? What problems were missed or ignored and caused us regrets? Given your expertise and knowledge of the field, what predictions do you have for VLDB opportunities in the future? The audience should expect to find something interesting not only to those who traveled this journey with us but also to attendees who weren’t there at the time and may not have even been born. This is a rare opportunity for you to hear from the people who were there and hear their perspectives on the future.
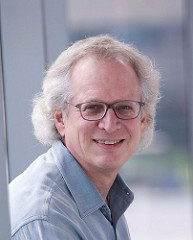
Bio: Philip A. Bernstein is a Distinguished Scientist at Microsoft Research. Over the past 35 years, he has been a product architect at Microsoft and Digital Equipment Corp., a professor at Harvard University and Wang Institute of Graduate Studies, and a VP Software at Sequoia Systems. He has published over 150 papers and two books on the theory and implementation of database systems, especially on transaction processing and metadata management. His latest work focuses on database systems and object-oriented middleware for distributed computing, and integration of heterogeneous data in the enterprise and on the web. He is an ACM Fellow, a winner of the ACM SIGMOD Innovations Award, and a member of the National Academy of Engineering. He received a B.S. from Cornell and M.Sc. and Ph.D. from University of Toronto. His home page is: http://research.microsoft.com/~philbe
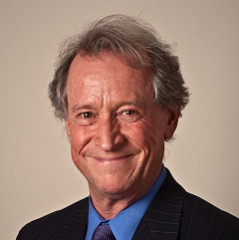
Bio: Dr. Brodie has over 40 years experience in research and industrial practice in databases, distributed systems, integration, artificial intelligence, and multi-disciplinary problem solving. He is concerned with the Big Picture aspects of information ecosystems including business, economic, social, application, and technical. Dr. Brodie is a Research Scientist, Computer Science and Artificial Intelligence Laboratory, Massachusetts Institute of Technology; advises startups; serves on Advisory Boards of national and international research organizations; and is an adjunct professor at the National University of Ireland, Galway and at the University of Technology, Sydney. For over 20 years he served as Chief Scientist of IT, Verizon, a Fortune 15 company, responsible for advanced technologies, architectures, and methodologies for IT strategies and for guiding industrial scale deployments of emerging technologies. His current research and applied interests include Big Data, Data Science, data curation at scale and a related start up Tamr.com. He has served on several National Academy of Science committees. Dr. Brodie holds a PhD in Databases from the University of Toronto and a Doctor of Science (honoris causa) from the National University of Ireland.
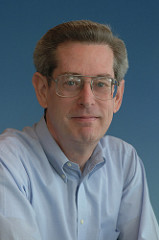
Bio: Don Chamberlin is co-inventor, with Ray Boyce, of SQL, the world’s most widely-used database query language. He was also one of the managers of System R, the IBM research project that produced the first implementation of SQL. More recently, Don represented IBM on the W3C working group that developed XQuery, a query language for XML data. Don received his B.S. degree from Harvey Mudd College and his Ph.D. from Stanford University. He has been named a Fellow of IBM, ACM, IEEE, and the Computer History Museum, and has received the ACM Software Systems Award and the SIGMOD Innovations Award. For several years Don has contributed problems to the annual ACM International Collegiate Programming Contest. He has also served as an adjunct professor of computer science at University of California, Santa Cruz, and at Santa Clara University. Don is currently retired and is dividing his time among learning, traveling, volunteer activities, and enjoying his grandchildren.
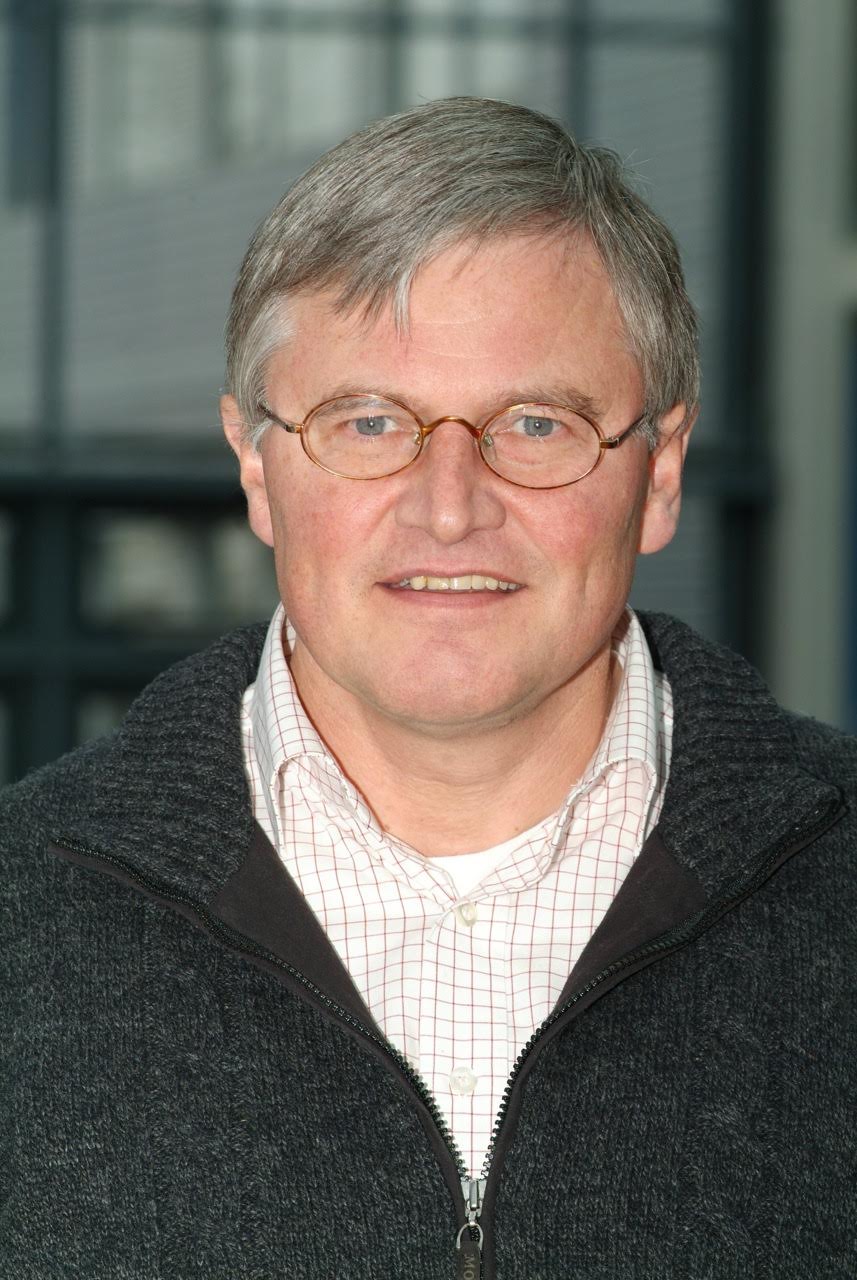
Bio: Alfons Kemper's research field is database systems engineering. He explores ways to optimize information systems for operational and scientific applications as a way to combat the data explosion. His main areas of interest are optimization concepts for distributed information structures, data integration methods and, in particular, main memory-based database systems. Together with his colleague Thomas Neumann he leads the HyPer main-memory database project (hyper-db.com) at Technische Universität München. HyPer is one of the first hybrid database systems that offers high-performance OLTP as well as OLAP in parallel on the same database state. After studying computer science at the University of Dortmund from 1977 to 1980, he moved to the University of Southern California, Los Angeles. While there, he obtained his Master of Science and doctorate. Upon his return to Germany, he completed his lecturer qualification at the University of Karlsruhe. His first professorship was conferred by RWTH Aachen. After many years as Director of the Chair of Database Systems at the University of Passau, TUM offered him a position in 2004. From 2006 to 2010, he was Dean of the Department of Informatics at TUM. His textbook on database systems, published by deGruyter and now in its 10th edition, is a best-seller in German-speaking countries and is used in most universities and colleges.
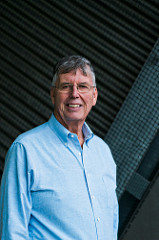
Bio: Dr. Stonebraker has been a pioneer of data base research and technology for more than a quarter of a century. He was the main architect of the INGRES relational DBMS, and the object-relational DBMS, POSTGRES. These prototypes were developed at the University of California at Berkeley where Stonebraker was a Professor of Computer Science for twenty five years. More recently at M.I.T. he was a co-architect of the Aurora/Borealis stream processing engine, the C-Store column-oriented DBMS, the H-Store transaction processing engine, the SciDB array DBMS, and the Data Tamer data curation system. Presently he serves as Chief Technology Officer of Paradigm4 and Tamr, Inc. Professor Stonebraker was awarded the ACM System Software Award in 1992 for his work on INGRES. Additionally, he was awarded the first annual SIGMOD Innovation award in 1994, and was elected to the National Academy of Engineering in 1997. He was awarded the IEEE John Von Neumann award in 2005, and is presently an Adjunct Professor of Computer Science at M.I.T, where he is co-director of the Intel Science and Technology Center focused on big data.
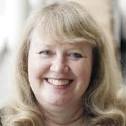
Bio: Dr. Pat Selinger is the Chief Technology Officer at Paradata (Paradata.io) where she is working on challenging problems in data harmonization, curation, provenance, and entity resolution. Prior to joining Paradata, she worked at IBM Research. She is a world-renowned pioneer in relational database management and inventor of the technique of cost-based query. She was a key member of the original System R team that created the first relational database research prototype. She also established and led IBM’s Database Technology Institute, considered one of the most successful examples of a fast technology pipeline from research to development and personally has technical contributions in the areas of database optimization, data parallelism, distributed data, and unstructured data management. Dr. Selinger was appointed an IBM Fellow in 1994 and is an ACM Fellow, a member of the National Academy of Engineering, and a Fellow of the American Academy of Arts and Sciences. Dr. Selinger has also received the ACM Systems Software Award for her work on System R and has received the SIGMOD Innovation Award.
Wednesday Sep 2nd 15:00-15:30
Refreshments: Coffee Break
Location: Grand Promenade
Wednesday Sep 2nd 15:30-17:00
Research 17: Graph Processing Systems
Location: Kings 1
Chair: Tilmann Rabl


Pregelix: Big(ger) Graph Analytics on A Dataflow Engine
Yingyi Bu (UC Irvine), Vinayak Borkar (X15 Software Inc), Jianfeng Jia (UC Irvine), Michael Carey (UC Irvine), Tyson Condie (UCLA)
There is a growing need for distributed graph processing systems that are capable of gracefully scaling to very large graph datasets. Unfortunately, this challenge has not been easily met due to the intense memory pressure imposed by process-centric, message passing designs that many graph processing systems follow. Pregelix is a new open source distributed graph processing system that is based on an iterative dataflow design that is better tuned to handle both in-memory and out-of-core workloads. As such, Pregelix offers improved performance characteristics and scaling properties over current open source systems (e.g., we have seen up to 15x speedup compared to Apache Giraph and up to 35x speedup compared to distributed GraphLab), and more effective use of available machine resources to support Big(ger) Graph Analytics.


Large-Scale Distributed Graph Computing Systems: An Experimental Evaluation
Yi Lu (CUHK), James Cheng (CUHK), Da Yan (HKUST), Huanhuan Wu (CUHK)
With the prevalence of graph data in real-world applications (e.g., social networks, mobile phone networks, web graphs, etc.) and their ever-increasing size, many distributed graph computing systems have been developed in recent years to process and analyze massive graphs. Most of these systems adopt Pregel's vertex-centric computing model, while various techniques have been proposed to address the limitations in the Pregel framework. However, there is a lack of comprehensive comparative analysis to evaluate the performance of various systems and their techniques, making it difficult for users to choose the best system for their applications. We conduct extensive experiments to evaluate the performance of existing systems on graphs with different characteristics and on algorithms with different design logic. We also study the effectiveness of various techniques adopted in existing systems, and the scalability of the systems. The results of our study reveal the strengths and limitations of existing systems, and provide valuable insights for users, researchers and system developers.


Fast Failure Recovery in Distributed Graph Processing Systems
Yanyan Shen (National University of Singapore), Gang Chen (Zhejiang University), H V Jagadish (University of Michigan Ann Arbor), Wei Lu (Renmin University), Beng Chin Ooi (National University of Singapore), Bogdan Tudor (National University of Singapore)
Distributed graph processing systems increasingly require many compute nodes to cope with the requirements imposed by contemporary graph-based Big Data applications. However, increasing the number of compute nodes increases the chance of node failures. Therefore, provisioning an efficient failure recovery strategy is critical for distributed graph processing systems. This paper proposes a novel recovery mechanism for distributed graph processing systems that parallelizes the recovery process. The key idea is to partition the part of the graph that is lost during a failure among a subset of the remaining nodes. To do so, we augment the existing checkpoint-based and log-based recovery schemes with a partitioning mechanism that is sensitive to the total computation and communication cost of the recovery process. Our implementation on top of the widely used Giraph system outperforms checkpoint-based recovery by up to 30x on a cluster of 40 compute nodes.


Giraph Unchained: Barrierless Asynchronous Parallel Execution in Pregel-like Graph Processing Systems
Minyang Han (University of Waterloo), Khuzaima Daudjee (University of Waterloo)
The bulk synchronous parallel (BSP) model used by synchronous graph processing systems allows algorithms to be easily implemented and reasoned about. However, BSP can suffer from poor performance due to stale messages and frequent global synchronization barriers. Asynchronous computation models have been proposed to alleviate these overheads but existing asynchronous systems that implement such models have limited scalability or retain frequent global barriers, and do not always support graph mutations or algorithms with multiple computation phases. We propose barrierless asynchronous parallel (BAP), a new computation model that reduces both message staleness and global synchronization. This enables BAP to overcome the limitations of existing asynchronous models while retaining support for graph mutations and algorithms with multiple computation phases. We present GiraphUC, which implements our BAP model in the open source distributed graph processing system Giraph, and evaluate our system at scale with large real-world graphs on 64 EC2 machines. We show that GiraphUC provides across-the-board performance improvements of up to $5\times$ faster over synchronous systems and up to an order of magnitude faster than asynchronous systems. Our results demonstrate that the BAP model provides efficient and transparent asynchronous execution of algorithms that are programmed synchronously.


GraphMat: High performance graph analytics made productive
Narayanan Sundaram (Intel Labs), Nadathur Satish (Intel Labs), Mostofa Ali Patwary (Intel Labs), Subramanya Dulloor (Intel Labs), Michael Anderson (Intel Labs), Satya Gautam Vadlamudi (Intel Labs), Dipankar Das (Intel Labs), Pradeep Dubey (Intel Labs)
Given the growing importance of large-scale graph analytics, there is a need to improve the performance of graph analysis frameworks without compromising on productivity. GraphMat is our solution to bridge this gap between a user-friendly graph analytics framework and native, hand-optimized code. GraphMat functions by taking vertex programs and mapping them to high performance sparse matrix operations in the backend. We thus get the productivity benefits of a vertex programming framework without sacrificing performance. GraphMat is a single-node multicore graph framework written in C++ which has enabled us to write a diverse set of graph algorithms with the same effort compared to other vertex programming frameworks. GraphMat performs 1.1-7X faster than high performance frameworks such as GraphLab, CombBLAS and Galois. GraphMat also matches the performance of MapGraph, a GPU-based graph framework, despite running on a CPU platform with significantly lower compute and bandwidth resources. It achieves better multicore scalability (13-15X on 24 cores) than other frameworks and is 1.2X off native, hand-optimized code on a variety of graph algorithms. Since GraphMat performance depends mainly on a few scalable and well-understood sparse matrix operations, GraphMat can naturally benefit from the trend of increasing parallelism in future hardware.
Research 18: Novel Hardware Architectures 2
Location: Kings 2
Chair: Jayant Haritsa


In-Cache Query Co-Processing on Coupled CPU-GPU Architectures
Jiong He (NTU), Shuhao Zhang (NTU), Bingsheng He (NTU)
Recently, there have been some emerging processor designs that the CPU and the GPU (Graphics Processing Unit) are integrated in a single chip and share Last Level Cache (LLC). However, the main memory bandwidth of such coupled CPU-GPU architectures can be much lower than that of a discrete GPU. As a result, current GPU query co-processing paradigms can severely suffer from memory stalls. In this paper, we propose a novel in-cache query co-processing paradigm for main memory On-Line Analytical Processing (OLAP) databases on coupled CPU-GPU architectures. Specifically, we adapt CPU-assisted prefetching to minimize cache misses in GPU query co-processing and CPU-assisted decompression to improve query execution performance. Furthermore, we develop a cost model guided adaptation mechanism for distributing the workload of prefetching, decompression, and query evaluations between CPU and GPU. We implement a system prototype and evaluate it on two recent AMD APUs A8 and A10. The experimental results show that 1) in-cache query co-processing can effectively improve the performance of state-of -the-art GPU co-processing paradigm by up to 30% and 33% on A8 and A10, respectively, and 2) our workload distribution adaption mechanism can significantly improve the query performance by 36% and 40% on A8 and A10, respectively.


NVRAM-aware Logging in Transaction Systems
Jian Huang (Georgia Tech), Karsten Schwan (Georgia Tech), Moinuddin Qureshi (Georgia Tech)
Emerging byte-addressable, non-volatile memory technologies (NVRAM) like phase-change memory can increase the capacity of future memory systems by orders of magnitude. Compared to systems that rely on disk storage, NVRAM-based systems promise significant improvements in performance for key applications like online transaction processing (OLTP). Unfortunately, NVRAM systems suffer from two drawbacks: their asymmetric read-write performance and the notable higher cost of the new memory technologies compared to disk. This paper investigates the cost-effective use of NVRAM in transaction systems. It shows that using NVRAM only for the logging subsystem (NV-Logging) provides much higher transactions per dollar than simply replacing all disk storage with NVRAM. Specifically, for NV-Logging, we show that the software overheads associated with centralized log buffers cause performance bottlenecks and limit scaling. The per-transaction logging methods described in the paper help avoid these overheads, enabling concurrent logging for multiple transactions. Experimental results with a faithful emulation of future NVRAM-based servers using the TPCC, TATP, and TPCB benchmarks show that NV-Logging improves throughput by 1.42 - 2.72x over the costlier option of replacing all disk storage with NVRAM. Results also show that NV-Logging performs 1.21 - 6.71x better than when logs are placed into the PMFS NVRAM-optimized file system. Compared to state-of-the-art distributed logging, NV-Logging delivers 20.4% throughput improvements.


Improving Main Memory Hash Joins on Intel Xeon Phi Processors: An Experimental Approach
Saurabh Jha (Nanyang Technological University), Bingsheng He (Nanyang Technological University), Mian Lu (A*STAR IHPC), Xuntao Cheng (Nanyang Technological University), Phung Huynh Huynh (A*STAR IHPC)
Modern processor technologies have driven new designs and implementations in main-memory hash joins. Recently, Intel Many Integrated Core (MIC) co-processors (commonly known as Xeon Phi) embrace emerging x86 single-chip many-core techniques. Compared with contemporary multi-core CPUs, Xeon Phi has quite different architectural features: wider SIMD instructions, many cores and hardware contexts, as well as lower-frequency in-order cores. In this paper, we experimentally revisit the state-of-the-art hash join algorithms on Xeon Phi co-processors. In particular, we study two camps of hash join algorithms: hardware-conscious ones that advocate careful tailoring of the join algorithms to underlying hardware architectures and hardware-oblivious ones that omit such careful tailoring. For each camp, we study the impact of architectural features and software optimizations on Xeon Phi in comparison with results on multi-core CPUs. Our experiments show two major findings on Xeon Phi, which are quantitatively different from those on multi-core CPUs. First, the impact of architectural features and software optimizations has quite different behavior on Xeon Phi in comparison with those on the CPU, which calls for new optimization and tuning on Xeon Phi. Second, hardware oblivious algorithms can outperform hardware conscious algorithms on a wide parameter window. These two findings further shed light on the design and implementation of query processing on new-generation single-chip many-core technologies.


REWIND: Recovery Write-Ahead System for In-Memory Non-Volatile Data-Structures
Andreas Chatzistergiou (University of Edinburgh), Marcelo Cintra (Intel), Stratis Viglas (University of Edinburgh)
Recent non-volatile memory (NVM) technologies, such as PCM, STT-MRAM and ReRAM, can act as both main memory and storage. This has led to research into NVM programming models, where persistent data structures remain in memory and are accessed directly through CPU loads and stores. Existing mechanisms for transactional updates are not appropriate in such a setting as they are optimized for block-based storage. We present REWIND, a user-mode library approach to managing transactional updates directly from user code written in an imperative general-purpose language. REWIND relies on a custom persistent in-memory data structure for the log that supports recoverable operations on itself. The scheme also employs a combination of non-temporal updates, persistent memory fences, and lightweight logging. Experimental results on synthetic transactional workloads and TPC-C show the overhead of REWIND compared to its non-recoverable equivalent to be within a factor of only 1.5 and 1.39 respectively. Moreover, REWIND outperforms state-of-the-art approaches for data structure recoverability as well as general purpose and NVM-aware DBMS-based recovery schemes by up to two orders of magnitude.


Persistent B+-Trees in Non-Volatile Main Memory
Shimin Chen (Chinese Academy of Sciences), Qin Jin (Renmin University)
Computer systems in the near future are expected to have Non-Volatile Main Memory (NVMM), enabled by a new generation of Non-Volatile Memory (NVM) technologies, such as Phase Change Memory (PCM), STT-MRAM, and Memristor. The non-volatility property has the promise to persist in-memory data structures for instantaneous failure recovery. However, realizing such promise requires a careful design to ensure that in-memory data structures are in known consistent states after failures. This paper studies persistent in-memory B+-Trees as B+-Trees are widely used in database and data-intensive systems. While traditional techniques, such as undo-redo logging and shadowing, support persistent B+-Trees, we find that they incur drastic performance overhead because of extensive NVM writes and CPU cache flush operations. PCM-friendly B+-Trees with unsorted leaf nodes help mediate this issue, but the remaining overhead is still large. In this paper, we propose write atomic B+-Trees (wB+-Trees), a new type of main-memory B+-Trees, that aim to reduce such overhead as much as possible. wB+-Tree nodes employ a small indirect slot array and/or a bitmap so that most insertions and deletions do not require the movement of index entries. In this way, wB+-Trees can achieve node consistency either through atomic writes in the nodes or by redo-only logging. We model fast NVM using DRAM on a real machine and model PCM using a cycle-accurate simulator. Experimental results show that compared with previous persistent B+-Tree solutions, wB+-Trees achieve up to 8.8x speedups on DRAM-like fast NVM and up to 27.1x speedups on PCM for insertions and deletions while maintaining good search performance. Moreover, we replaced Memcached's internal hash index with tree indices. Our real machine Memcached experiments show that wB+-Trees achieve up to 3.8X improvements over previous persistent tree structures with undo-redo logging or shadowing.
Industrial 5: In-memory Data Management
Location: Kings 3
Chair: Amr El-Helw


Distributed Architecture of Oracle Database In-memory
Niloy Mukherjee (Oracle Corporation), Shasank Chavan (Oracle Corporation), Maria Colgan (Oracle Corporation), Dinesh Das (Oracle Corporation), Mike Gleeson (Oracle Corporation), Sanket Hase (Oracle Corporation), Allison Holloway (Oracle Corporation), Hui Jin (Oracle Corporation), Jesse Kamp (Oracle Corporation), Kartk Kulkarni (Oracle Corporation), Tirthankar Lahiri (Oracle Corporation), Juan Loaiza (Oracle Corporation), Neil Macnaughton (Oracle Corporation), Vineet Marwah (Oracle Corporation), Andy Witkowski (Oracle Corporation), Jiaqi Yan (Oracle Corporation), Mohamed Zait (Oracle Corporation)
Over the last few years, the information technology industry has witnessed revolutions in multiple dimensions. Increasing ubiquitous sources of data have posed two connected challenges to data management solutions – processing unprecedented volumes of data, and providing ad-hoc real-time analysis in mainstream production data stores without compromising regular transactional workload performance. In parallel, computer hardware systems are scaling out elastically, scaling up in the number of processors and cores, and increasing main memory capacity extensively. The data processing challenges combined with the rapid advancement of hardware systems has necessitated the evolution of a new breed of main-memory databases optimized for mixed OLTAP environments and designed to scale. The Oracle RDBMS In-memory Option (DBIM) is an industry-first distributed dual format architecture that allows a database object to be stored in columnar format in main memory highly optimized to break performance barriers in analytic query workloads, simultaneously maintaining transactional consistency with the corresponding OLTP optimized row-major format persisted in storage and accessed through database buffer cache. In this paper, we present the distributed, highly-available, and fault-tolerant architecture of the Oracle DBIM that enables the RDBMS to transparently scale out in a database cluster, both in terms of memory capacity and query processing throughput. We believe that the architecture is unique among all mainstream in-memory databases. It allows complete application-transparent, extremely scalable and automated distribution of Oracle RDBMS objects in-memory across a cluster, as well as across multiple NUMA nodes within a single server. It seamlessly provides distribution awareness to the Oracle SQL execution framework through affinitized fault-tolerant parallel execution within and across servers without explicit optimizer plan changes or query rewrites.


Gorilla: A Fast, Scalable, In-Memory Time Series Database
Justin Teller (Facebook), Scott Franklin (Facebook), Tuomas Pelkonen (Facebook), Paul Cavallaro (Facebook)
Large-scale internet services aim to remain highly available and responsive in the presence of unexpected failures. Providing this service often requires monitoring and analyzing tens of millions of measurements per second across a large number of systems, and one particularly effective solution is to store and query such measurements in a time series database (TSDB). A key challenge in the design of TSDBs is how to strike the right balance between efficiency, scalability, and reliability. In this paper we introduce Gorilla, Facebook's in-memory TSDB. Our insight is that users of monitoring systems do not place much emphasis on individual data points but rather on aggregate analysis, and recent data points are of much higher value than older points to quickly detect and diagnose the root cause of an ongoing problem. Gorilla optimizes for remaining highly available for writes and reads, even in the face of failures, at the expense of possibly dropping small amounts of data on the write path. To improve query efficiency, we aggressively leverage compression techniques such as delta-of-delta timestamps and XOR'd floating point values to reduce Gorilla's storage footprint by $10$x. This allows us to store Gorilla's data in memory, reducing query latency by $73$x and improving query throughput by $14$x when compared to a traditional database (HBase)-backed time series data. This performance improvement has unlocked new monitoring and debugging tools, such as time series correlation search and more dense visualization tools. Gorilla also gracefully handles failures from a single-node to entire regions with little to no operational overhead.


Query Optimization in Oracle 12c Database In-Memory
Dinesh Das (Oracle), Jiaqi Yan (Oracle), Mohamed Zait (Oracle Corp), Satyanarayana Valluri,EPFL), Nirav Vyas (Oracle), Ramarajan Krishnamachari (Oracle), Prashant Gaharwar (Oracle), Jesse Kamp (Oracle), Niloy Mukherjee (Oracle Corporation)
Traditional on-disk row major tables have been the dominant storage mechanism in relational databases for decades. Over the last decade, however, with explosive growth in data volume and demand for faster analytics, has come the recognition that a different data representation is needed. There is widespread agreement that in-memory column-oriented databases are best suited to meet the realities of this new world. Oracle 12c Database In-memory, the industry’s first dual-format database, allows existing row major on-disk tables to have complementary in-memory columnar representations. The new storage format brings new data processing techniques and query execution algorithms and thus new challenges for the query optimizer. Execution plans that are optimal for one format may be sub-optimal for the other. In this paper, we describe the changes made in the query optimizer to generate execution plans optimized for the specific format – row major or columnar – that will be scanned during query execution. With enhancements in several areas – statistics, cost model, query transformation, access path and join optimization, parallelism, and cluster-awareness – the query optimizer plays a significant role in unlocking the full promise and performance of Oracle Database In-Memory.
Research 19: Social Network Analysis
Location: Queens 4
Chair: Felix Naumann


Robust Local Community Detection: On Free Rider Effect and Its Elimination
Yubao Wu (Case Western Reserve University), Ruoming Jin (Kent State University), Jing Li (Case Western Reserve University), Xiang Zhang (Case Western Reserve University)
Given a large network, local community detection aims at finding the community that contains a set of query nodes and also maximizes (minimizes) a goodness metric. This problem has recently drawn intense research interest. Various goodness metrics have been proposed. However, most existing metrics tend to include irrelevant subgraphs in the detected local community. We refer to such irrelevant subgraphs as free riders. We systematically study the existing goodness metrics and provide theoretical explanations on why they may cause the free rider effect. We further develop a query biased node weighting scheme to reduce the free rider effect. In particular, each node is weighted by its proximity to the query node. We define a query biased density metric to integrate the edge and node weights. The query biased densest subgraph, which has the largest query biased density, will shift to the neighborhood of the query nodes after node weighting. We then formulate the query biased densest connected subgraph (QDC) problem, study its complexity, and provide efficient algorithms to solve it. We perform extensive experiments on a variety of real and synthetic networks to evaluate the effectiveness and efficiency of the proposed methods.


Viral Marketing Meets Social Advertising: Ad Allocation with Minimum Regret
Cigdem Aslay (University Pompeu Fabra), Wei Lu (University of British Columbia), Francesco Bonchi (Yahoo Labs), Amit Goyal (Twitter), Laks Lakshmanan (University of British Columbia)
Social advertisement is one of the fastest growing sectors in the digital advertisement landscape: ads in the form of promoted posts are shown in the feed of users of a social networking platform, along with normal social posts; if a user clicks on a promoted post, the host (social network owner) is paid a fixed amount from the advertiser. In this context, allocating users to ads is typically performed by maximizing click-through-rate, i.e., the likelihood that the user will click on the ad. However, this simple strategy fails to leverage the fact the ads can propagate virally through the network, from endorsing users to their followers. In this paper, we study the problem of allocating users to ads through the viral-marketing lens. Advertisers approach the host with a budget in return for the marketing campaign service provided by the host. We show that allocation that takes into account the propensity of ads for viral propagation can achieve significantly better performance. However, uncontrolled virality could be undesirable for the host as it creates room for exploitation by the advertisers: hoping to tap uncontrolled virality, an advertiser might declare a lower budget for its marketing campaign, aiming at the same large outcome with a smaller cost. This creates a challenging trade-off: on the one hand, the host aims at leveraging virality and the network effect to improve advertising efficacy, while on the other hand the host wants to avoid giving away free service due to uncontrolled virality. We formalize this as the problem of ad allocation with minimum regret, which we show is \NPhard and inapproximable w.r.t. any factor. However, we devise an algorithm that provides approximation guarantees w.r.t. the total budget of all advertisers. We develop a scalable version of our approximation algorithm, which we extensively test on three real data sets, confirming that our algorithm delivers high quality solutions, is scalable, and significantly outperforms several natural baselines.


Community Detection in Social Networks: An In-depth Benchmarking Study with a Procedure-Oriented Framework
Meng Wang (Tsinghua University), Chaokun Wang (Tsinghua University), Jeffrey Xu Yu (The Chinese University of Hong Kong), Jun Zhang (Tsinghua University)
Revealing the latent community structure, which is crucial to understanding the features of networks, is an important problem in network and graph analysis. During the last decade, many approaches have been proposed to solve this challenging problem in diverse ways, i.e. different measures or data structures. Unfortunately, experimental reports on existing techniques fell short in validity and integrity since many comparisons were not based on a unified code base or merely discussed in theory. We engage in an in-depth benchmarking study of community detection in social networks. We formulate a generalized community detection procedure and propose a procedure-oriented framework for benchmarking. This framework enables us to evaluate and compare various approaches to community detection systematically and thoroughly under identical experimental conditions. Upon that we can analyze and diagnose the inherent defect of existing approaches deeply, and further make effective improvements correspondingly. We have re-implemented ten state-of-the-art representative algorithms upon this framework and make comprehensive evaluations of multiple aspects, including the efficiency evaluation, performance evaluations, sensitivity evaluations, etc. We discuss their merits and faults in depth, and draw a set of take-away interesting conclusions. In addition, we present how we can make diagnoses for these algorithms resulting in significant improvements.


Leveraging History for Faster Sampling of Online Social Networks
Zhuojie Zhou (George Washington University), Nan Zhang (George Washington University), Gautam Das (University of Texas at Arlington)
With a vast amount of data available on online social networks, how to enable efficient analytics over such data has been an increasingly important research problem. Given the sheer size of such social networks, many existing studies resort to sampling techniques that draw random nodes from an online social network through its restrictive web/API interface. While these studies differ widely in analytics tasks supported and algorithmic design, almost all of them use the exact same underlying technique of random walk - a Markov Chain Monte Carlo based method which iteratively transits from one node to its random neighbor. Random walk fits naturally with this problem because, for most online social networks, the only query we can issue through the interface is to retrieve the neighbors of a given node (i.e., no access to the full graph topology). A problem with random walks, however, is the ``burn-in'' period which requires a large number of transitions/queries before the sampling distribution converges to a stationary value that enables the drawing of samples in a statistically valid manner. In this paper, we consider a novel problem of speeding up the fundamental design of random walks (i.e., reducing the number of queries it requires) without changing the stationary distribution it achieves - thereby enabling a more efficient ``drop-in'' replacement for existing sampling-based analytics techniques over online social networks. Technically, our main idea is to leverage the history of random walks to construct a higher-ordered Markov chain. We develop two algorithms, Circulated Neighbors and Groupby Neighbors Random Walk (CNRW and GNRW) and rigidly prove that, no matter what the social network topology is, CNRW and GNRW offer better efficiency than baseline random walks while achieving the same stationary distribution. We demonstrate through extensive experiments on real-world social networks and synthetic graphs the superiority of our techniques over the existing ones.


Real-time Targeted Influence Maximization for Online Advertisements
Yuchen Li (National University of Singapo), Dongxiang Zhang (National University of Singapore), Kian-Lee Tan (National University of Singapore)
Advertising in social network has become a multi-billion-dollar industry. A main challenge is to identify key influencers who can effectively contribute to the dissemination of information. Although the influence maximization problem, which finds a seed set of $k$ most influential users based on certain propagation models, has been well studied, it is not target-aware and cannot be directly applied to online advertising. In this paper, we propose a new problem, named Keyword-Based Targeted Influence Maximization (KB-TIM), to find a seed set that maximizes the expected influence over users who are relevant to a given advertisement. To solve the problem, we propose a sampling technique based on weighted reverse influence set and achieve an approximation ratio of $(1-1/e-\varepsilon)$. To meet the instant-speed requirement, we propose two disk-based solutions that improve the query processing time by two orders of magnitude over the state-of-the-art solutions, while keeping the theoretical bound. Experiments conducted on two real social networks confirm our theoretical findings as well as the efficiency. Given an advertisement with $5$ keywords, it takes only $2$ seconds to find the most influential users in a social network with billions of edges.
Research 20: Ranking and Top-K
Location: Queens 5
Chair: Raymond Wong


Top-k Nearest Neighbor Search In Uncertain Data Series
Michele Dallachiesa (University of Trento), Themis Palpanas (Paris Descartes University), Ihab Ilyas (QCRI)
Many real applications consume data that is intrinsically uncertain, noisy and error-prone. In this study, we investigate the problem of finding the top-k nearest neighbors in uncertain data series, which occur in several different domains. We formalize the top-k nearest neighbor problem for uncertain data series, and describe a model for uncertain data series that captures both uncertainty and correlation. This distinguishes our approach from prior work that compromises the accuracy of the model by assuming independence of the value distribution at neighboring time-stamps. We introduce the Holistic-PkNN algorithm, which uses novel metric bounds for uncertain series and an efficient refinement strategy to reduce the overall number of required probability estimates. We evaluate our proposal under a variety of settings using a combination of synthetic and 45 real datasets from diverse domains. The results demonstrate the significant advantages of the proposed approach.


Scaling Manifold Ranking Based Image Retrieval
Yasuhiro Fujiwara (NTT), Go Irie (NTT), Shari Kuroyama (California Institute of Technology), Makoto Onizuka (Osaka University)
Manifold Ranking is a graph-based ranking algorithm being successfully applied to retrieve images from multimedia databases. Given a query image, Manifold Ranking computes the ranking scores of images in the database by exploiting the relationships among them expressed in the form of a graph. Since Manifold Ranking effectively utilizes the global structure of the graph, it is significantly better at finding intuitive results compared with current approaches. Fundamentally, Manifold Ranking requires an inverse matrix to compute ranking scores and so needs O(n^3) time, where n is the number of images. Manifold Ranking, unfortunately, does not scale to support databases with large numbers of images. Our solution, Mogul, is based on two ideas: (1) It efficiently computes ranking scores by sparse matrices, and (2) It skips unnecessary score computations by estimating upper bounding scores. These two ideas reduce the time complexity of Mogul to O(n) from O(n^3) of the inverse matrix approach. Experiments show that Mogul is much faster and gives significantly better retrieval quality than a state-of-the-art approximation approach.


Optimal Enumeration: Efficient Top-k Tree Matching
Lijun Chang (University of New South Wales), Xuemin Lin (University of New South Wales), Wenjie Zhang (UNSW), Jeffrey Xu Yu (The Chinese University of Hong Kong (Hong Kong), Ying Zhang (University of Technology (Sydney), Lu Qin (University of Technology (Sydney)
Driven by many real applications, graph pattern matching has attracted a great deal of attention recently. Consider that a twig-pattern matching may result in an extremely large number of matches in a graph; this may not only confuse users by providing too many results but also lead to high computational costs. In this paper, we study the problem of top-$k$ tree pattern matching; that is, given a rooted tree $T$, compute its top-$k$ matches in a directed graph $G$ based on the twig-pattern matching semantics. We firstly present a novel and optimal enumeration paradigm based on the principle of Lawler's procedure. We show that our enumeration algorithm runs in $O (n_T + \log k)$ time in each round where $n_T$ is the number of nodes in $T$. Considering that the time complexity to output a match of $T$ is $O(n_T)$ and $n_T \geq \log k$ in practice, our enumeration technique is optimal. Moreover, the cost of generating top-$1$ match of $T$ in our algorithm is $O (m_R)$ where $m_R$ is the number of edges in the transitive closure of a data graph $G$ involving all relevant nodes to $T$. $O (m_R)$ is also optimal in the worst case without pre-knowledge of $G$. Consequently, our algorithm is optimal with the running time $O (m_R + k (n_T + \log k))$ in contrast to the time complexity $O (m_R \log k + k n_T (\log k + d_T))$ of the existing technique where $d_T$ is the maximal node degree in $T$. Secondly, a novel priority based access technique is proposed, which greatly reduces the number of edges accessed and results in a significant performance improvement. Finally, we apply our techniques to the general form of top-$k$ graph pattern matching problem (i.e., query is a graph) to improve the existing techniques. Comprehensive empirical studies demonstrate that our techniques may improve the existing techniques by orders of magnitude.


Generating Top-k Packages via Preference Elicitation
Min Xie (University of British Columbia), Laks V. S. Lakshmanan (University of British Columbia), Peter Wood (Birkbeck (University of London)
There are several applications, such as play lists of songs or movies, and shopping carts, where users are interested in finding top-k packages, consisting of sets of items. In response to this need, there has been a recent flurry of activity around extending classical recommender systems (RS), which are effective at recommending individual items, to recommend packages, or sets of items. The few recent proposals for package RS suffer from one of the following drawbacks: they either rely on hard constraints which may be difficult to be specified exactly by the user or on returning Pareto-optimal packages which are too numerous for the user to sift through. To overcome these limitations, we propose an alternative approach for finding personalized top-k packages for users, by capturing users' preferences over packages using a linear utility function which the system learns. Instead of asking a user to specify this function explicitly, which is unrealistic, we explicitly model the uncertainty in the utility function and propose a preference elicitation-based framework for learning the utility function through feedback provided by the user. We propose several sampling-based methods which, given user feedback, can capture the updated utility function. We develop an efficient algorithm for generating top-k packages using the learned utility function, where the rank ordering respects any of a variety of ranking semantics proposed in the literature. Through extensive experiments on both real and synthetic datasets, we demonstrate the efficiency and effectiveness of the proposed system for finding top-k packages.


Rank aggregation with ties: Experiments and Analysis
Bryan Brancotte (Université Paris Sud), Bo Yang (Wuhan University (Wu), Guillaume Blin (University of Bordeaux), Sarah Cohen-Boulakia (Université Paris Sud), Sylvie Hamel (Université de Montréal)
The problem of aggregating multiple rankings into one consensus ranking is an active research topic especially in the database community. Various studies have implemented methods for rank aggregation and may have come up with contradicting conclusions upon which algorithms work best. Comparing such results is cumbersome, as the original studies mixed different approaches and used very different evaluation data sets and metrics. Additionally, in real applications, the rankings to be aggregated may not be permutations where elements are strictly ordered, but they may have ties where some elements are placed at the same position. However, most of the studies have not considered rank aggregation with ties. This paper introduces the first large study of algorithms for rank aggregation with ties. More precisely, (i) we review the major rank aggregation algorithms and show how ties in the input rankings can be or cannot be handled; (ii) we propose the first implementation to compute the exact solution of the Rank Aggregation with ties problem based on linear programming; (iii) we evaluate algorithms for rank aggregation with ties on a very large panel of both real and carefully generated synthetic datasets; (iv) we provide guidance on the algorithms to be favored depending on dataset features.
Tutorial 5: SQL-on-Hadoop Systems (2/2)
Location: Queens 6


SQL-on-Hadoop Systems (2/2)
Daniel Abadi, Shivnath Babu, Fatma Ozcan, Ippokratis Pandis
In this tutorial, we will examine the SQL-on-Hadoop systems along various dimensions. One important aspect is their data storage. Some of these systems support all native Hadoop formats, and do not impose any propriety data formats, and keep the data open to all applications running on the same platform. While there are some database hybrid solutions, such as HAWQ, HP Haven, and Vortex, that store their propriety data formats in HDFS. Most often, these systems are also able to run SQL queries over native HDFS formats, but do not provide the same level of performance. Some SQL-on-Hadoop systems provide their own SQL-specific run-times, such as Impala, Big SQL, and Presto, while others exploit a general purpose run-time such as Hive (MapReduce and Tez) and SparkSQL (Spark). Another important aspect is the support for schema flexibility and complex data types. Almost all of these systems support complex data types, such as arrays and structs. But, only a few, such as Drill and Hadapt with Sinew [13], are able to work with schemaless data.
Demo 1: Data Mining, Graph, Text, and Semi-structured Data
Location: Kona 4
Chair: Tianli Mo


Evaluating SPARQL Queries on Massive RDF Datasets
Razen Harbi (King Abdullah University of Science and Technology), Ibrahim Abdelaziz (King Abdullah University of Science and Technology), Panos Kalnis (King Abdullah University of Science and Technology), Nikos Mamoulis (University of Ioannina)
Distributed RDF systems partition data across multiple com- puter nodes. Partitioning is typically based on heuristics that minimize inter-node communication and it is performed in an initial, data pre-processing phase. Therefore, the re- sulting partitions are static and do not adapt to changes in the query workload; as a result, existing systems are unable to consistently avoid communication for queries that are not favored by the initial data partitioning. Furthermore, for very large RDF knowledge bases, the partitioning phase be- comes prohibitively expensive, leading to high startup costs. In this paper, we propose AdHash, a distributed RDF system which addresses the shortcomings of previous work. First, AdHash initially applies lightweight hash partitioning, which drastically minimizes the startup cost, while favoring the parallel processing of join patterns on subjects, with- out any data communication. Using a locality-aware plan- ner, queries that cannot be processed in parallel are evalu- ated with minimal communication. Second, AdHash moni- tors the data access patterns and adapts dynamically to the query load by incrementally redistributing and replicating frequently accessed data. As a result, the communication cost for future queries is drastically reduced or even elimi- nated. Our experiments with synthetic and real data verify that AdHash (i) starts faster than all existing systems, (ii) processes thousands of queries before other systems become online, and (iii) gracefully adapts to the query load, being able to evaluate queries on billion-scale RDF data in sub- seconds. In this demonstration, audience can use a graphi- cal interface of AdHash to verify its performance superiority compared to state-of-the-art distributed RDF systems.


Demonstration of Santoku: Optimizing Machine Learning over Normalized Data
Arun Kumar (University of Wisconsin-Madiso), Mona Jalal (University of Wisconsin-Madison), Boqun Yan (University of Wisconsin-Madison), Jeffrey Naughton (University Wisconsin-Madison), Jignesh Patel (University of Wisconsin-Madison)
Advanced analytics is a booming area in the data man- agement industry and a hot research topic. Almost all toolkits that implement machine learning (ML) al- gorithms assume that the input is a single table, but most relational datasets are not stored as single tables due to normalization. Thus, analysts often join tables to obtain a denormalized table. Also, analysts typ- ically ignore any functional dependencies among fea- tures because ML toolkits do not support them. In both cases, time is wasted in learning over data with redundancy. We demonstrate Santoku, a toolkit to help analysts improve the performance of ML over normal- ized data. Santoku applies the idea of factorized learn- ing and automatically decides whether to denormalize or push ML computations through joins. Santoku also exploits database dependencies to provide automatic in- sights that could help analysts with exploratory feature selection. It is usable as a library in R, which is a pop- ular environment for advanced analytics. We demon- strate the benefits of Santoku in improving ML perfor- mance and helping analysts with feature selection.


PRISM: Concept-preserving Summarization of Top-K Social Image Search Results
Boon-Siew Seah (Nanyang Technological University), Sourav S Bhowmick (Nanyang Technological University), Aixin Sun (Nanyang Technological University)
Most existing tag-based social image search engines present search results as a ranked list of images, which cannot be consumed by users in a natural and intuitive manner. In this demonstration, we present a novel concept-preserving image search results summa- rization system called prism. prism exploits both visual features and tags of the search results to generate high quality summary, which not only breaks the results into visually and semantically coherent clusters but it also maximizes the coverage of the original top-k search results. It first constructs a visual similarity graph where the nodes are images in the top-k search results and the edges repre- sent visual similarities between pairs of images. This graph is opti- mally decomposed and compressed into a set of concept-preserving subgraphs based on a set of summarization criteria. One or more exemplar images from each subgraph is selected to form the exem- plar summary of the result set. We demonstrate various innovative features of prism and the promise of superior quality summary con- struction of social image search results.


SPARTex: A Vertex-Centric Framework for RDF Data Analytics
Ibrahim Abdelaziz (King Abdullah University of Science and Technology), Razen Harbi (King Abdullah University of Science and Technology), Semih Salihoglu (Stanford University), Panos Kalnis (King Abdullah University of Science and Technology), Nikos Mamoulis (University of Ioannina)
A growing number of applications require combining SPARQL queries with generic graph search on RDF data. However, the lack of procedural capabilities in SPARQL makes it inappropriate for graph analytics. Moreover, RDF engines focus on SPARQL query evaluation whereas graph management frameworks perform only generic graph computations. In this work, we bridge the gap by introducing SPARTex, an RDF analytics framework based on the vertex-centric computation model. In SPARTex, user-defined ver- tex centric programs can be invoked from SPARQL as stored pro- cedures. SPARTex allows the execution of a pipeline of graph algo- rithms without the need for multiple reads/writes of input data and intermediate results. We use a cost-based optimizer for minimiz- ing the communication cost. SPARTex evaluates queries that com- bine SPARQL and generic graph computations orders of magnitude faster than existing RDF engines. We demonstrate a real system prototype of SPARTex running on a local cluster using real and syn- thetic datasets. SPARTex has a real-time graphical user interface that allows the participants to write regular SPARQL queries, use our proposed SPARQL extension to declaratively invoke graph al- gorithms or combine/pipeline both SPARQL querying and generic graph analytics.


I2RS: A Distributed Geo-Textual Image Retrieval and Recommendation System
Lu Chen (Zhejiang University), Yunjun Gao (Zhejiang University), Zhihao Xing (Zhejiang University), Christian Jensen (Aalborg University), Gang Chen (Zhejiang University)
Massive amounts of geo-tagged and textually annotated images are provided by online photo services such as Flickr and Zommr. However, most existing image retrieval engines only consider text annotations. We present I2RS, a system that allows users to view geo-textual images on Google Maps, find hot topics within a spe- cific geographic region and time period, retrieve images similar to a query image, and receive recommended images that they might be interested in. I2RS is a distributed geo-textual image retrieval and recommendation system that employs SPB-trees to index geo- textual images, and that utilizes metric similarity queries, includ- ing top-m spatio-temporal range and k nearest neighbor queries, to support geo-textual image retrieval and recommendation. The system adopts the browser-server model, whereas the server is deployed in a distributed environment that enables efficiency and scalability to huge amounts of data and requests. A rich set of 100 million geo-textual images crawled from Flickr is used to demon- strate that, I2RS can return high-quality answers in an interactive way and support efficient updates for high image arrival rates.


Reformulation-based query answering in RDF: alternatives and performance
Damian Bursztyn (INRIA), Francois Goasdoue (University of Rennes 1), Ioana Manolescu (INRIA)
Answering queries over Semantic Web data, i.e., RDF graphs, must account for both explicit data and implicit data, en- tailed by the explicit data and the semantic constraints hold- ing on them. Two main query answering techniques have been devised, namely Saturation-based (Sat) which precom- putes and adds to the graph all implicit information, and Reformulation-based (Ref) which reformulates the query based on the graph constraints, so that evaluating the refor- mulated query directly against the explicit data (i.e., with- out considering the constraints) produces the query answer. While Sat is well known, Ref has received less attention so far. In particular, reformulated queries often perform poorly if the query is complex. Our demonstration show- cases a large set of Ref techniques, including but not limited to one we proposed recently. The audience will be able to 1. test them against different datasets, constraints and queries, as well as different well-established systems, 2. analyze and understand the performance challenges they raise, and 3. al- ter the scenarios to visualize the impact on performance. In particular, we show how a cost-based Ref approach allows avoiding reformulation performance pitfalls.


TreeScope: Finding Structural Anomalies In Semi-Structured Data
Shanshan Ying (ADSC), Flip Korn, Barna Saha (University of Massachusetts Amherst), Divesh Srivastava (AT&T Labs-Research)
Semi-structured data are prevalent on the web, with formats such as XML and JSON soaring in popularity due to their generality, flex- ibility and easy customization. However, these very same features make semi-structured data prone to a range of data quality errors, from errors in content to errors in structure. While the former has been well studied, little attention has been paid to structural errors. In this demonstration, we present TREESCOPE, which analyzes semi-structured data sets with the goal of automatically identifying structural anomalies from the data. Our techniques learn robust structural models that have high support, to identify potential errors in the structure. Identified structural anomalies are then concisely summarized to provide plausible explanations of the potential er- rors. The goal of this demonstration is to enable an interactive ex- ploration of the process of identifying and summarizing structural anomalies in semi-structured data sets.


PERSEUS: An Interactive Large-Scale Graph Mining and Visualization Tool
Danai Koutra (Carnegie Mellon University), Di Jin (Carnegie Mellon University), Yuanchi Ning (Uber Technologies Inc.), Christos Faloutsos (Carnegie Mellon University)
Given a large graph with several millions or billions of nodes and edges, such as a social network, how can we explore it efficiently and find out what is in the data? In this demo we present Perseus, a large-scale system that enables the comprehensive analysis of large graphs by supporting the coupled summarization of graph properties and structures, guiding attention to outliers, and allowing the user to inter- actively explore normal and anomalous node behaviors. Specifically, Perseus provides for the following opera- tions: 1) It automatically extracts graph invariants (e.g., degree, PageRank, real eigenvectors) by performing scalable, online batch processing on Hadoop; 2) It interactively visualizes univariate and bivariate distributions for those in- variants; 3) It summarizes the properties of the nodes that the user selects; 4) It efficiently visualizes the induced sub- graph of a selected node and its neighbors, by incrementally revealing its neighbors. In our demonstration, we invite the audience to interact with Perseus to explore a variety of multi-million-edge so- cial networks including a Wikipedia vote network, a friend- ship/foeship network in Slashdot, and a trust network based on the consumer review website Epinions.com.


Virtual eXist-db: Liberating Hierarchical Queries from the Shackles of Access Path Dependence
Curtis Dyreson (Utah State University), Sourav S Bhowmick (Nanyang Technological University), Ryan Grapp (Utah State University)
XQuery programs can be hard to write and port to new data collections because the path expressions in a query are dependent on the hierarchy of the data. We propose to demonstrate a system to liberate query writers from this dependence. A plug-and-play query contains a specification of what data the query needs in order to evaluate. We implemented virtual eXist-db to support plug-and- play XQuery queries. Our system adds a virtualDoc function that lets a programmer sketch the hierarchy needed by the query, which may well be different than what the data has, and logically (not physically) transforms the data (with information loss guarantees) to the hierarchy specified by the virtualDoc. The demonstration will consist of a sequence of XQuery queries using a virtual hierarchy, including queries suggested by the audience. We will also demonstrate a GUI tool to construct a virtual hierarchy.


FLORIN - A System to Support (Near) Real-Time Applica-tions on User Generated Content on Daily News
Qingyuan Liu (Temple University), Eduard Dragut (Temple University), Arjun Mukherjee (University of Houston), Weiyi Meng (Binghamton University)
In this paper, we propose a system, FLORIN, which provides support for near real-time applications on user generated content on daily news. FLORIN continuously crawls news outlets for articles and user comments accompanying them. It attaches the articles and comments to daily event stories. It identifies the opinionated content in user comments and performs named entity recognition on news articles. All these pieces of information are organized hierarchically and exportable to other applications. Multiple applications can be built on this data. We have implemented a sentiment analysis system that runs on top of it.


A Framework for Clustering Uncertain Data
Erich Schubert (Ludwig-Maximilians-Universität Munich), Alexander Koos (Ludwig-Maximilians-Universität München), Tobias Emrich (Ludwig-Maximilians-Universität Munich), Andreas Züfle (Ludwig-Maximilians-Universität München), Klaus Schmid (Ludwig-Maximilians-Universität München), Arthur Zimek (Ludwig-Maximilians-Universität Munich)
The challenges associated with handling uncertain data, in particular with querying and mining, are finding increasing attention in the research community. Here we focus on clustering uncertain data and describe a general framework for this purpose that also allows to visualize and understand the impact of uncertainty—using different uncertainty models—on the data mining results. Our framework constitutes release 0.7 of ELKI (http://elki.dbs.ifi.lmu.de/) and thus comes along with a plethora of implementations of algorithms, distance measures, indexing techniques, evaluation measures and visualization components.

Query-oriented summarization of RDF graphs
Sejla Cebiric (INRIA), Francois Goasdoue (University of Rennes 1), Ioana Manolescu (INRIA)


Universal-DB: Towards Representation Independent Graph Analytics
Yodsawalai Chodpathumwan (University of Illinois), Amirhossein Aleyassin (University of Illinois), Arash Termehchy (Oregon State University), Yizhou Sun (Northeastern University)
Graph analytics algorithms leverage quantiable structural properties of the data to predict interesting concepts and relationships. The same information, however, can be rep- resented using many dierent structures and the structural properties observed over particular representations do not necessarily hold for alternative structures. Because these algorithms tend to be highly eective over some choices of structure, such as that of the databases used to validate them, but not so eective with others, graph analytics has largely remained the province of experts who can nd the desired forms for these algorithms. We argue that in order to make graph analytics usable, we should develop systems that are eective over a wide range of choices of structural organizations. We demonstrate Universal-DB an entity sim- ilarity and proximity search system that returns the same answers for a query over a wide range of choices to represent the input database.


Tornado: A Distributed Spatio-Textual Stream Processing System
Ahmed Mahmood (Purdue University), Ahmed Aly (Purdue University), Thamir Qadah (Purdue University), El Kindi Rezig (Purdue University), Anas Daghistani (Purdue University), Amgad Madkour (Purdue University), Ahmed Abdelhamid (Purdue University), Mohamed Hassan (Purdue University), Walid Aref (Purdue University (USA), Saleh Basalamah (Umm Al-Qura University)
The widespread use of location-aware devices together with the increased popularity of micro-blogging applications (e.g., Twitter) led to the creation of large streams of spatio-textual data. In order to serve real-time applications, the processing of these large-scale spatio-textual streams needs to be distributed. However, existing distributed stream processing systems (e.g., Spark and Storm) are not optimized for spatial/textual content. In this demonstration, we introduce Tornado, a distributed in-memory spatio-textual stream processing server that extends Storm. To efficiently process spatio-textual streams, Tornado introduces a spatio-textual indexing layer to the architecture of Storm. The indexing layer is adaptive, i.e., dynamically re-distributes the processing across the system according to changes in the data distribution and/or query workload. In addition to keywords, higher-level textual concepts are identified and are semantically matched against spatio-textual queries. Tornado provides data deduplication and fusion to eliminate redun- dant textual data. We demonstrate a prototype of Tornado running against real Twitter streams, where the users can register continuous or snapshot spatio-textual queries using a map-assisted query-interface.


S+EPP: Construct and Explore Bisimulation Summaries, plus Optimize Navigational Queries; all on Existing SPARQL Systems
Mariano Consens (University of Toronto), Valeria Fionda (University of Calabria), Shahan Khatchadourian (University of Toronto), Giuseppe Pirrò (ICAR-CNR)
We demonstrate S+EPPs, a system that provides fast construction of bisimulation summaries using graph analytics platforms, and then enhances existing SPARQL engines to support summary-based exploration and navigational query optimization. The construction component adds a novel optimization to a parallel bisimulation algorithm implemented on a multi-core graph processing framework. We show that for several large, disk resident, real world graphs, full sum- mary construction can be completed in roughly the same time as the data load. The query translation component supports Extended Property Paths (EPPs), an enhancement of SPARQL 1.1 property paths that can express a significantly larger class of navigational queries. EPPs are implemented via rewritings into a widely used SPARQL subset. The optimization component can (transparently to users) translate EPPs defined on instance graphs into EPPs that take advantage of bisimulation summaries. S+EPPs combines the query and optimization translations to enable summary-based optimization of graph traversal queries on top of off-the-shelf SPARQL processors. The demonstration showcases the construction of bisimulation summaries of graphs (ranging from millions to billions of edges), together with the exploration benefits and the navigational query speedups obtained by leveraging summaries stored alongside the original datasets.


GraphGen: Exploring Interesting Graphs in Relational Data
Konstantinos Xirogiannopoulos (University of Maryland at College Park), Udayan Khurana (University of Maryland at College Park), Amol Deshpande (University of Maryland at College Park)
Analyzing interconnection structures among the data through the use of graph algorithms and graph analytics has been shown to provide tremendous value in many application domains. However, graphs are not the primary choice for how most data is currently stored, and users who want to employ graph analytics are forced to extract data from their data stores, construct the requisite graphs, and then use a specialized engine to write and execute their graph analysis tasks. This cumbersome and costly process not only raises barriers in using graph analytics, but also makes it hard to explore and identify hidden or implicit graphs in the data. Here we demonstrate a system, called GRAPHGEN, that enables users to declaratively specify graph extraction tasks over relational databases, visually explore the extracted graphs, and write and execute graph algorithms over them, either directly or using existing graph libraries like the widely used NetworkX Python library. We also demonstrate how unifying the extraction tasks and the graph algorithms enables significant optimizations that would not be possible otherwise.


StarDB: A Large-Scale DBMS for Strings
Majed Sahli (King Abdullah University of Science and Technology), Essam Mansour (QCRI), Panos Kalnis (King Abdullah University of Science and Technology)
Strings and applications using them are proliferating in science and business. Currently, strings are stored in file systems and processed using ad-hoc procedural code. Exist- ing techniques are not flexible and cannot efficiently handle complex queries or large datasets. In this paper, we demonstrate StarDB, a distributed database system for analytics on strings. StarDB hides data and system complexities and allows users to focus on analytics. It uses a comprehensive set of parallel string operations and provides a declarative query language to solve complex queries. StarDB automatically tunes itself and runs with over 90% efficiency on supercomputers, public clouds, clusters, and workstations. We test StarDB using real datasets that are 2 orders of magnitude larger than the datasets reported by previous works.
Wednesday Sep 2nd 18:00-21:00
Banquet
Location: Palace Lawn
Chair: Banquet Chair
Thursday Sep 3rd 07:30-08:30
Light Breakfast
Location: Grand Promenade
Thursday Sep 3rd 08:30-10:00
Award Ceremony: Award distribution for 10 year best paper,early career,and conference best papers
Location: Monarchy Ballroom
Chair: Surajit Chaudhuri
Award Ceremony
Thursday Sep 3rd 10:00-10:30
Refreshments: Coffee Break
Location: Grand Promenade
Thursday Sep 3rd 10:30-12:00
Research 21: Spatial Databases
Location: Kings 1
Chair: Walid Aref


Trajectory Simplification: On Minimizing the Direction-based Error
Cheng Long (Hong Kong University of Science and Technology), Raymond Chi-Wing Wong (Hong Kong University of Science and Technology), H V Jagadish University of Michigan Ann Arbor,)
Trajectory data is central to many applications with moving objects. Raw trajectory data is usually very large, and so is simplified before it is stored and processed. Many trajectory simplification notions have been proposed, and among them, the direction-preserving trajectory simplification (DPTS) which aims at protecting the direction information has been shown to perform quite well. However, existing studies on DPTS require users to specify an error tolerance which users might not know how to set properly in some cases (e.g., the error tolerance could only be known at some future time and simply setting one error tolerance does not meet the needs since the simplified trajectories would usually be used in many different applications which accept different error tolerances). In these cases, a better solution is to minimize the error while achieving a pre-defined simplification size. For this purpose, in this paper, we define a problem called Min-Error and develop two exact algorithms and one 2-factor approximate algorithm for the problem. Extensive experiments on real datasets verified our algorithms.


Selectivity Estimation on Streaming SpatioTextual Data Using Local Correlations
Xiaoyang Wang (University of New South Wales), Ying Zhang (University of Technology (Sydney), Wenjie Zhang (University of New South Wales), Xuemin Lin (University of New South Wales), Wei Wang (University of New South Wales)
In this paper, we investigate the selectivity estimation problem for streaming spatio-textual data, which arises in many social network and geo-location applications. Specifically, given a set of continuously and rapidly arriving spatio-textual objects, each of which is described by a geo-location and a short text, we aim to accurately estimate the cardinality of a spatial keyword query on objects seen so far, where a spatial keyword query consists of a search region and a set of query keywords. To the best of our knowledge, this is the first work to address this important problem. We first extend two existing techniques to solve this problem, and show their limitations. Inspired by two key observations on the "locality" of the correlations among query keywords, we propose a local correlation based method by utilizing an augmented adaptive space partition tree (A2SP-tree for short) to approximately learn a local Bayesian network on-the-fly for a given query and estimate its selectivity. A novel local boosting approach is presented to further enhance the learning accuracy of local Bayesian networks. Our comprehensive experiments on real-life datasets demonstrate the superior performance of the local correlation based algorithm in terms of estimation accuracy compared to other competitors.


Spatial Joins in Main Memory: Implementation Matters!
Darius Sidlauskas (Aarhus University), Christian Jensen (Aalborg University)
A recent PVLDB paper reports on experimental analyses of ten spatial join techniques in main memory. We build on this comprehensive study to raise awareness of the fact that empirical running time performance findings in main-memory settings are results of not only the algorithms and data structures employed, but also their implementation, which complicates the interpretation of the results. In particular, we re-implement the worst performing technique without changing the underlying high-level algorithm, and we then offer evidence that the resulting re-implementation is capable of outperforming all the other techniques. This study demonstrates that in main memory, where no time-consuming I/O can mask variations in implementation, implementation details are very important; and it offers a concrete illustration of how it is difficult to make conclusions from empirical running time performance findings in main-memory settings about data structures and algorithms studied.


Large Scale Real-time Ridesharing with Service Guarantee on Road Networks
Yan Huang (University of North Texas), Favyen Bastani (MIT), Ruoming Jin (Kent State University), Xiaoyang Wang (Fudan University)
Urban traffic gridlock is a familiar scene. At the same time, the mean occupancy rate of personal vehicle trips in the United States is only 1.6 persons per vehicle mile. Ridesharing has the potential to solve many environmental, congestion, pollution, and energy problems. In this paper, we introduce the problem of large scale real-time ridesharing with service guarantee on road networks. Trip requests are dynamically matched to vehicles while trip waiting and service time constraints are satisfied. We first propose two scheduling algorithms: a branch-and-bound algorithm and an integer programing algorithm. However, these algorithms do not adapt well to the dynamic nature of the ridesharing problem. Thus, we propose kinetic tree algorithms which are better suited to efficient scheduling of dynamic requests and adjust routes on-the-fly. We perform experiments on a large Shanghai taxi dataset. Results show that the kinetic tree algorithms outperform other algorithms significantly.


Compressed Spatial Hierarchical Bitmap (cSHB) Indexes for Efficiently Processing Spatial Range Query Workloads
Parth Nagarkar (Arizona State University), K. Selcuk Candan (Arizona State University), Aneesha Bhat (Arizona State University)
In most spatial data management applications, objects are represented in terms of their coordinates in a 2-dimensional space and search queries in this space are processed using spatial index structures. On the other hand, bitmap-based indexing, especially thanks to the compression opportunities bitmaps provide, has been shown to be highly effective for query processing workloads including selection and aggregation operations. In this paper, we show that bitmap based indexing can also be highly effective for managing spatial data sets. More specifically, we propose a novel compressed spatial hierarchical bitmap (cSHB) index structure to support spatial range queries. We consider query workloads involving multiple range queries over spatial data and introduce and consider the problem of bitmap selection for identifying the appropriate subset of the bitmap files for processing the given spatial range query workload. We develop cost models for compressed domain range query processing and present query planning algorithms that not only select index nodes for query processing, but also associate appropriate bitwise logical operations to identify the data objects satisfying the range queries in the given workload. Experiment results confirm the efficiency and effectiveness of the proposed compressed spatial hierarchical bitmap (cSHB) index structure and the range query planning algorithms in supporting spatial range query workloads.
Research 22: Search
Location: Kings 2
Chair: Jennie Duggan


Finding Patterns in a Knowledge Base using Keywords to Compose Table Answers
Mohan Yang (UCLA), Bolin Ding (Microsoft Research), Surajit Chaudhuri (Microsoft Research), Kaushik Chakrabarti (Microsoft Research)
We aim to provide table answers to keyword queries against knowledge bases. For queries referring to multiple entities, like ”Washington cities population” and ”Mel Gibson movies”, it is better to represent each relevant answer as a table which aggregates a set of entities or entity-joins within the same table scheme or pattern. In this paper, we study how to find highly relevant patterns in a knowledge base for user-given keyword queries to compose table answers. A knowledge base can be modeled as a directed graph called knowledge graph, where nodes represent entities in the knowledge base and edges represent the relationships among them. Each node/edge is labeled with type and text. A pattern is an aggregation of subtrees which contain all keywords in the texts and have the same structure and types on node/edges. We propose efficient algorithms to find patterns that are relevant to the query for a class of scoring functions. We show the hardness of the problem in theory, and propose path-based indexes that are affordable in memory. Two query-processing algorithms are proposed: one is fast in practice for small queries (with small patterns as answers) by utilizing the indexes; and the other one is better in theory, with running time linear in the sizes of indexes and answers, which can handle large queries better. We also conduct extensive experimental study to compare our approaches with a naive adaption of known techniques.


Searchlight: Enabling Integrated Search and Exploration over Large Multidimensional Data
Alexander Kalinin (Brown University), Ugur Cetintemel (Brown University), Stan Zdonik (Brown University)
We present a new system, called {\em Searchlight}, that uniquely integrates constraint solving and data management techniques. It allows Constraint Programming (CP) machinery to run efficiently inside a DBMS without the need to extract, transform and move the data. This marriage concurrently offers the rich expressiveness and efficiency of constraint-based search and optimization provided by modern CP solvers, and the ability of DBMSs to store and query data at scale, resulting in an enriched functionality that can effectively support both data-\textit{ and} search-intensive applications. As such, Searchlight is the first system to support {\em generic} search, exploration and mining over large multi-dimensional data collections, going beyond point algorithms designed for point search and mining tasks. Searchlight makes the following scientific contributions: \begin{itemize} \item {\bf Constraint solvers as first-class citizens} Instead of treating solver logic as a black-box, Searchlight provides native support, incorporating the necessary APIs for its specification and transparent execution as part of query plans, as well as novel algorithms for its optimized execution and parallelization. \item {\bf Speculative solving} Existing solvers assume that the entire data set is main-memory resident. Searchlight uses an innovative two stage {\em Solve-Validate} approach that allows it to operate speculatively yet safely on main-memory synopses, quickly producing candidate search results that can later be efficiently validated on real data. \item {\bf Computation and I/O load balancing} As CP solver logic can be computationally expensive, executing it on large search and data spaces requires novel CPU-I/O balancing approaches when performing search distribution. \end{itemize} We built a prototype implementation of Searchlight on Google's Or-Tools, an open-source suite of operations research tools, and the array DBMS SciDB. Extensive experimental results show that Searchlight often performs orders of magnitude faster than the next best approach (SciDB-only or CP-solver-only) in terms of end response time and time to first result.


Processing Moving kNN Queries Using Influential Neighbor Sets
Chuanwen Li (Northeastern University), Yu Gu (Northeastern University), Jianzhong Qi (University of Melbourne), Ge Yu (Northeastern University), Rui Zhang (University of Melbourne), Wang Yi (Northeastern University)
The moving k nearest neighbor query, which computes one’s k nearest neighbor set and maintains it while at move, is gaining importance due to the prevalent use of smart mobile devices such as smart phones. Safe region is a popular technique in processing the moving k nearest neighbor query. It is a region where the movement of the query object does not cause the current k nearest neighbor set to change. Processing a moving k nearest neighbor query is a continuing process of checking the validity of the safe region and recomputing it if invalidated. The size of the safe region largely decides the frequency of safe region recomputation and hence query processing efficiency. Existing moving k nearest neighbor algorithms lack efficiency due to either computing small safe regions and have to recompute frequently or computing large safe regions (i.e., an order-k Voronoi cell) with a high cost. In this paper, we take a third approach. Instead of safe regions, we use a small set of safe guarding objects. We prove that, as long as the the current k nearest neighbors are closer to the query object than the safe guarding objects, the current k nearest neighbors stay valid and no recomputation is required. This way, we avoid the high cost of safe region recomputation. We also prove that, the region defined by the safe guarding objects is the largest possible safe region. This means that the recomputation frequency of our method is also minimized. We conduct extensive experiments comparing our method with the state-of-the-art method on both real and synthetic data sets. The results confirm the superiority of our method. In this paper, we take a third approach. Instead of safe regions, we use a small set of safe guarding objects. We prove that, as long as the safe guarding objects are nearer to the query object than the current k nearest neighbors are, the current k nearest neighbors are still valid and no recomputation is required. This way, we avoid frequently computing the expensive safe regions. We also prove that, the region conceptually defined by the safe guarding objects is always the largest possible safe region. This means that the recomputation of our approach is minimized. We conduct extensive experiments comparing our method with the state-of-the-art method on both real and randomly generated data sets. The results confirm the superiority of our method.


Reverse k Nearest Neighbors Query Processing: Experiments and Analysis
Shiyu Yang (University of New South Wales), Muhammad Cheema (Monash University), Xuemin Lin (University of New South Wales), Wei Wang (University of New South Wales)
Given a set of users, a set of facilities and a query facility q, a reverse k nearest neighbors (RkNN) query returns every user u for which the query is one of its k closest facilities. RkNN queries have been extensively studied under a variety of settings and many sophisticated algorithms have been proposed to answer these queries. However, the existing experimental studies suffer from a few limitations. for example, some studies estimate the I/O cost by charging a fixed penalty per I/O and we show that this may be misleading. Also, the existing studies either use an extremely small buffer or no buffer at all which puts some algorithms at serious disadvantage. We show that the performance of these algorithms is significantly improved even when a small buffer (containing 100 pages) is used. Finally, in each of the existing studies, the proposed algorithm is mainly compared only with its predecessor assuming that it was the best algorithm at the time which is not necessarily true as shown in our experimental study. Motivated by these limitations, we present a comprehensive experimental study that addresses these limitations and compares some of the most notable algorithms under a wide variety of settings. Furthermore, we also present a carefully developed filtering strategy that significantly improves TPL which is one of the most popular RkNN algorithms. Specifically, the optimized version is up to 20 times faster than the original version and reduces its I/O cost up to two times.


Permutation Search Methods are Efficient, Yet Faster Search is Possible
Bilegsaikhan Naidan (NTNU), Leonid Boytsov (Carnegie Mellon University), Eric Nyberg (Carnegie Mellon University)
We survey permutation-based methods for approximate k-nearest neighbor search. In these methods, every data point is represented by a ranked list of pivots sorted by the distance to this point. Such ranked lists are called permutations. The underpinning assumption is that, for both metric and non-metric spaces, the distance between permutations is a good proxy for the distance between original points. Thus, it should be possible to efficiently retrieve most true nearest neighbors by examining only a tiny subset of data points whose permutations are similar to the permutation of a query. We further test this assumption by carrying out an extensive experimental evaluation where permutation methods are pitted against state-of-the art benchmarks (the multi-probe LSH, the VP-tree, and proximity-graph based retrieval) on a variety of realistically large data set from the image and textual domain. The focus is on the high-accuracy retrieval methods for generic spaces. Additionally, we assume that both data and indices are stored in main memory. We find permutation methods to be reasonably efficient and describe a setup where these methods are most useful. To ease reproducibility, we make our software and data sets publicly available.
Industrial 6: Logging, Parallel Processing, and Graph Processing
Location: Kings 3
Chair: Bettina Kemme


Building a Replicated Logging System with Apache Kafka
Guozhang Wang (LinkedIn), Joel Koshy,LinkedIn), Sriram Subramanian (LinkedIn), Kartik Paramasivam (LinkedIn), Mammad Zadeh (LinkedIn), Neha Narkhede (Confluent Inc.), Jun Rao (Confluent Inc.), Jay Kreps (Confluent Inc.), Joe Stein (Big Data Open Source Security LLC)
Apache Kafka is a scalable publish-subscribe messaging system with its core architecture as a distributed commit log. It was originally built at LinkedIn as its centralized event pipelining platform for online data integration tasks. Over the past years developing and operating Kafka, we extend its log-structured architecture as a replicated logging backbone for much wider application scopes in the distributed environment. In this abstract, we will talk about our design and engineering experience to replicate Kafka logs for various distributed data-driven systems at LinkedIn, including source-of-truth data storage and stream processing.


Optimization of Common Table Expressions in MPP Database Systems
Amr El-Helw (Pivotal Inc.), Venkatesh Raghavan (Pivotal Inc.), Mohamed Soliman (Pivotal Inc.), George Caragea (Pivotal Inc.), Zhongxian Gu (Datometry Inc.), Michalis Petropoulos (Amazon Web Services)
Big Data analytics often include complex queries with similar or identical expressions, usually referred to as Common Table Expressions (CTEs). CTEs may be explicitly defined by users to simplify query formulations, or implicitly included in queries generated by business intelligence tools, financial applications and decision support systems. In Massively Parallel Processing (MPP) database systems, CTEs pose new challenges due to the distributed nature of query processing, the overwhelming volume of underlying data and the scalability criteria that systems are required to meet. In these settings, the effective optimization and efficient execution of CTEs are crucial for the timely processing of analytical queries over Big Data. In this paper, we present a comprehensive framework for the representation, optimization and execution of CTEs in the context of ORCA - Pivotal's query optimizer for Big Data. We demonstrate experimentally the benefits of our techniques using industry standard decision support benchmark.


One Trillion Edges: Graph Processing at Facebook-Scale
Avery Ching (Facebook), Dionysios Logothetis (Facebook), Sergey Edunov (Facebook), Maja Kabiljo (Facebook), Sambavi Muthukrishnan (Facebook)
Analyzing large graphs provides valuable insights for social networking and web companies in content ranking and recommendations. While numerous graph processing systems have been developed and evaluated on available benchmark graphs of up to 6.6B edges, they often face significant difficulties in scaling to much larger graphs. Industry graphs can be two orders of magnitude larger - hundreds of billions or up to one trillion edges. In addition to scalability challenges, real world applications often require much more complex graph processing workflows than previously evaluated. In this paper, we describe the usability, performance, and scalability improvements we made to Apache Giraph, an open-source graph processing system, in order to use it on Facebook scale graphs of up to one trillion edges. We also describe several key extensions to the original Pregel model that make it possible to develop a broader range of production graph applications and workflows as well as improve code reuse. Finally, we report on real-world operations as well as performance characteristics of several large-scale production applications.
Research 23: Logic and Semantics
Location: Queens 4
Chair: Tore Risch


DREAM: Distributed RDF Engine with Adaptive Query Planner and Minimal Communication
Mohammad Hammoud (Carnegie Mellon University), Dania Abed Rabbou (Carnegie Mellon University), Reza Nouri (University of New South Wales), Amin Beheshti (University of New South Wales), Sherif Sakr (University of New South Wales)
The Resource Description Framework (RDF) and SPARQL query language are gaining wide popularity and acceptance. In this paper, we present DREAM, a distributed and adaptive RDF system. As opposed to existing RDF systems, DREAM avoids partitioning RDF datasets and partitions only SPARQL queries. By not partitioning datasets, DREAM offers a general paradigm for different types of pattern matching queries, and entirely averts intermediate data shuffling (only auxiliary data are shuffled). Besides, by partitioning queries, DREAM presents an adaptive scheme, which automatically runs queries on various numbers of machines depending on their complexities. Hence, in essence DREAM combines the advantages of the state-of-the-art centralized and distributed RDF systems, whereby data communication is avoided and cluster resources are aggregated. Likewise, it precludes their disadvantages, wherein system resources are limited and communication overhead is typically hindering. DREAM achieves all its goals via employing a novel graph-based, rule-oriented query planner and a new cost model. We implemented DREAM and conducted comprehensive experiments on a private cluster and on the Amazon EC2 platform. Results show that DREAM can significantly outperform three related popular RDF systems.


Efficient Identification of Implicit Facts in Incomplete OWL2-EL Knowledge Bases
John Liagouris (National Technical University of Athens), Manolis Terrovitis (IMIS `Athena')
Integrating incomplete and possibly inconsistent data from various sources is a challenge that arises in several application areas, especially in the management of scientific data. A rising trend for data integration is to model the data as axioms in the Web Ontology Language (OWL) and use inference rules to identify new facts. Although there are several approaches that employ OWL for data integration, there is little work on scalable algorithms able to handle large datasets that do not fit in main memory. The main contribution of this paper is an algorithm that allows the effective use of OWL for integrating data in an environment with limited memory. The core idea is to exhaustively apply a set of complex inference rules on large disk-resident datasets. To the best of our knowledge, this is the first work that proposes an I/O-aware algorithm for tackling with such an expressive subset of OWL like the one we address here. Previous approaches considered either simpler models (e.g. RDFS) or main-memory algorithms. In the paper we detail the proposed algorithm, prove its correctness, and experimentally evaluate it on real and synthetic data.


Taming Subgraph Isomorphism for RDF Query Processing
Jinha Kim (Oracle Labs), Hyungyu Shin (POSTECH), Wook-Shin Han (POSTECH), Sungpack Hong (Oracle Labs), Hassan Chafi (Oracle Labs)
RDF data are used to model knowledge in various areas such as life sciences, Semantic Web, bioinformatics, and social graphs. The size of real RDF data reaches billions of triples. This calls for a framework for efficiently processing RDF data. The core function of processing RDF data is subgraph pattern matching. There have been two completely different directions for supporting efficient subgraph pattern matching. One direction is to develop specialized RDF query processing engines exploiting the properties of RDF data for the last decade, while the other direction is to develop efficient subgraph isomorphism algorithms for general, labeled graphs for over 30 years. Although both directions have a similar goal (i.e., finding subgraphs in data graphs for a given query graph), they have been independently researched without clear reason. We argue that a subgraph isomorphism algorithm can be easily modified to handle the graph homomorphism, which is the RDF pattern matching semantics, by just removing the injectivity constraint. In this paper, based on the state-of-the-art subgraph isomorphism algorithm, we propose an in-memory solution, TurboHOM++, which is tamed for the RDF processing, and we compare it with the representative RDF processing engines for several RDF benchmarks in a server machine where billions of triples can be loaded in memory. In order to speed up TurboHOM++, we also provide a simple yet effective transformation and a series of optimization techniques. Extensive experiments using several RDF benchmarks show that TurboHOM++ consistently and significantly outperforms the representative RDF engines. Specifically, TurboHOM++ outperforms its competitors by up to five orders of magnitude.


SEMA-JOIN : Joining Semantically-Related Tables Using Big Table Corpora
Yeye He (Microsoft Research), Kris Ganjam (Microsoft Research), Xu Chu (University of Waterloo)
Join is a powerful operator that combines records from two or more tables, which is of fundamental importance in the field of relational database. However, traditional join processing mostly relies on string equality comparisons. Given the growing demand for ad-hoc data analysis, we have seen an increasing number of scenarios where the desired join relationship is not equi-join. For example, in a spreadsheet environment, a user may want to join one table with a subject column \texttt{country-name}, with another table with a subject column \texttt{country-code}. Traditional equi-join cannot handle such joins automatically, and the user typically has to manually find an intermediate mapping table in order to perform the desired join. We develop a SEMA-JOIN approach that is a first step toward allowing users to perform semantic join automatically, with a click of the button. Our main idea is to utilize a data-driven method that leverages a big table corpus with over 100 million tables to determine statistical correlation between cell values at both row-level and column-level. We use the intuition that the correct join mapping is the one that maximizes aggregate pairwise correlation, to formulate the join prediction problem as an optimization problem. We develop a linear program relaxation and a rounding argument to obtain a 2-approximation algorithm in polynomial time. Our evaluation using both public tables from the Web and proprietary Enterprise tables from a large company shows that the proposed approach can perform automatic semantic joins with high precision for a variety of common join scenarios.


QuickFOIL: Scalable Inductive Logic Programming
Qiang Zeng (University of Wisconsin-Madison), Jignesh Patel (University of Wisconsin-Madison), David Page (University of Wisconsin-Madison)
Inductive Logic Programming (ILP) is a classic machine learning technique that learns first-order rules from relational- structured data. However, to-date most ILP systems can only be applied to small datasets (tens of thousands of ex- amples). A long-standing challenge in the field is to scale ILP methods to larger data sets. This paper presents a method called QuickFOIL that addresses this limitation. QuickFOIL employs a new scoring function and a novel pruning strategy that enables the algorithm to find high- quality rules. QuickFOIL can also be implemented as an in-RDBMS algorithm. Such an implementation presents a host of query processing and optimization challenges that we address in this paper. Our empirical evaluation shows that QuickFOIL can scale to large datasets consisting of hundreds of millions tuples, and is often more than order of magnitude more efficient than other existing approaches.
Research 24: Innovative Systems
Location: Queens 5
Chair: Rainer Gemulla


ScalaGiST: Scalable Generalized Search Trees for MapReduce Systems
Peng Lu (National University of Singapore), Gang Chen (Zhejiang University), Beng Chin Ooi (National University of Singapore), Hoang Tam Vo (National University of Singapore), Sai Wu (Zhejiang University)
MapReduce has become the state-of-the-art for data parallel processing. Nevertheless, Hadoop, an open-source equivalent of MapReduce, has been noted to have sub-optimal performance in the database context since it is initially designed to operate on raw data without utilizing any type of indexes. To alleviate the problem, we present ScalaGiST -- scalable generalized search tree that can be seamlessly integrated with Hadoop, together with a cost-based data access optimizer for efficient query processing at run-time. ScalaGiST provides extensibility in terms of data and query types, hence is able to support unconventional queries (e.g., multi-dimensional range and KNN queries) in MapReduce systems, and can be dynamically deployed in large cluster environments for handling big users and data. We have built ScalaGiST and demonstrated that it can be easily instantiated to common B+-tree and R-tree indexes yet for dynamic distributed environments. Our extensive performance study confirms that ScalaGiST can provide efficient write and read performance, elastic scaling property, as well as effective support for MapReduce execution of ad-hoc analytic queries. We conduct extensive experiment to evaluate the performance of ScalaGiST, and compare it with recent proposals of specialized distributed index structures, such as SpatialHadoop, Data Mapping, and RT-CAN. The result confirms its efficiency.


DIADEM: Thousands of Websites to a Single Database
Tim Furche (Oxford University), Georg Gottlob (University of Oxford), Giovanni Grasso (Oxford University), Xiaonan Guo (Oxford University), Giorgio Orsi (University of Oxford), Christian Schallhart (Oxford University), Cheng Wang (Oxford University)
The web is overflowing with implicitly structured data, spread over hundreds of thousands of sites, hidden deep behind search forms, or siloed in marketplaces, only accessible as HTML. Automatic extraction of structured data at the scale of thousands of websites has long proven elusive, despite its central role in the “web of data”. Through an extensive evaluation spanning over 10000 web sites from multiple application domains, we show that automatic, yet accurate full-site extraction is no longer a distant dream. DIADEM is the first automatic full-site extraction system that is able to extract structured data from different domains at very high accuracy. It combines automated exploration of websites, identification of relevant data, and induction of exhaustive wrappers. Automating these components is the first challenge. DIADEM overcomes this challenge by combining phenomenological and ontological knowledge. Integrating these components is the second challenge. DIADEM overcomes this challenge through a self-adaptive network of relational transducers that produces effective wrappers for a wide variety of websites. Our extensive and publicly available evaluation shows that, for more than 90% of sites from three domains, DIADEM obtains an effective wrapper that extracts all relevant data with 97% average precision. DIADEM also tolerates noisy entity recognisers, and its components individually outperform comparable approaches.


AsterixDB: A Scalable, Open Source BDMS
Sattam Alsubaiee (UC Irvine), Yasser Altowim (UC Irvine), Hotham Altwaijry (UC Irvine), Alex Behm (Cloudera), Vinayak Borkar (UC Irvine), Yingyi Bu (UC Irvine), Michael Carey (UC Irvine), Inci Cetindil (UC Irvine), Madhusudan Cheelangi (Google), Khurram Faraaz (IBM), Eugenia Gabrielova (UC Irvine), Raman Grover (UC Irvine), Zachary Heilbron (UC Irvine), Young-Seok Kim (UC Irvine), Chen Li (University of California (Irvine), Guangqiang Li (MarkLogic), Ji Mahn Ok (UC Irvine), Nicola Onose (Pivotal Inc.), Pouria Pirzadeh (UC Irvine), Vassilis Tsotras (UC Riverside), Rares Vernica (HP Labs), Jian Wen (Oracle Lab), Till Westmann (Oracle Labs)
AsterixDB is a new, full-function BDMS (Big Data Management System) with a feature set that distinguishes it from other platforms in today's open source Big Data ecosystem. Its features make it well-suited to applications like web data warehousing, social data storage and analysis, and other use cases related to Big Data. AsterixDB has a flexible NoSQL style data model; a query language that supports a wide range of queries; a scalable runtime; partitioned, LSM-based data storage and indexing (including B+-tree, R-tree, and text indexes); support for external as well as natively stored data; a rich set of built-in types; support for fuzzy, spatial, and temporal types and queries; a built-in notion of data feeds for ingestion of data; and transaction support akin to that of a NoSQL store. Development of AsterixDB began in 2009 and led to a mid-2013 initial open source release. This paper is the first complete description of the resulting open source AsterixDB system. Covered herein are the system's data model, its query language, and its software architecture. Also included are a summary of the current status of the project and a first glimpse into how AsterixDB performs when compared to alternative technologies, including a parallel relational DBMS, a popular NoSQL store, and a popular Hadoop-based SQL data analytics platform, for things that both technologies can do. Also included is a brief description of some initial trials that the system has undergone and the lessons learned (and plans laid) based on those early "customer" engagements.


Mega-KV: A Case for GPUs to Maximize the Throughput of In-Memory Key-Value Stores
Kai Zhang (University of Science and Technology of China), Kaibo Wang (The Ohio State University), Yuan Yuan (The Ohio State University), Lei Guo (The Ohio State University), Rubao Lee (The Ohio State University), Xiaodong Zhang (The Ohio State University)
In-memory key-value stores play a critical role in data processing to provide high throughput and low latency data accesses. In-memory key-value stores have several unique properties that include (1) data intensive operations demanding high memory bandwidth for fast data accesses, (2) high data parallelism and simple computing operations demanding many slim parallel computing units, and (3) a large working set. As data volume continues to increase, our experiments show that conventional and general-purpose multicore systems are increasingly mismatched to the special properties of key-value stores because they do not provide massive data parallelism based on high memory bandwidth; the powerful but the limited number of computing cores do not satisfy the demand of the unique data processing task; and the cache hierarchy may not well benefit to the large working set. In this paper, we make a strong case for GPUs to serve as special-purpose devices to greatly accelerate the operations of in-memory key-value stores. Specifically, we present the design and implementation of Mega-KV, a GPU-based in-memory key-value store system that achieves high performance and high throughput. Effectively utilizing the high memory bandwidth and latency hiding capability of GPUs, Mega-KV provides fast data accesses and significantly boosts overall performance. Running on a commodity PC installed with two CPUs and two GPUs, Mega-KV can process up to 160+ million key-value operations per second, which is 1.4-2.8 times as fast as the state-of-the-art key-value store system on a conventional CPU-based platform.


UDA-GIST: An In-database Framework to Unify Data-Parallel and State-Parallel Analytics
Kun Li (University of Florida), Daisy Zhe Wang (University of Florida), Alin Dobra (University of Florida), Chris Dudley (University of Florida)
Enterprise applications need sophisticated in-database analytics in addition to traditional online analytical processing from a database. To meet customers' pressing demands, database vendors have been pushing advanced analytical techniques into databases. Most major DBMSes offer User-Defined Aggregate (UDA), a data-driven operator, to implement many of the analytical techniques in parallel. However, UDAs can not be used to implement statistical algorithms such as Markov chain Monte Carlo (MCMC), where most of the work is performed by iterative transitions over a large state that can not be naively partitioned due to data dependency. Typically, this type of statistical algorithm requires pre-processing to setup the large state in the first place and demands post-processing after the statistical inference. This paper presents General Iterative State Transition (GIST), a new database operator for parallel iterative state transitions over large states. GIST receives a state constructed by a UDA, and then performs rounds of transitions on the state until it converges. A final UDA performs post-processing and result extraction. We argue that the combination of UDA and GIST (UDA-GIST) unifies data-parallel and state-parallel processing in a single system, thus significantly extending the analytical capabilities of DBMSes. We exemplify the framework through two high-profile applications: cross-document coreference and image denoising. We show that the in-database framework allows us to tackle a 27 times larger problem than solved by the state-of-the-art for the first application and achieves 43 times speedup over the state-of-the-art for the second application.
Tutorial 6: Truth Discovery and Crowdsourcing Aggregation: A Unified Perspective
Location: Queens 6


Truth Discovery and Crowdsourcing Aggregation: A Unified Perspective
Jing Gao, Qi Li, Bo Zhao, Wei Fan, Jiawei Han
In the era of Big Data, data entries, even describing the same objects or events, can come from a variety of sources, where a data source can be a web page, a database or a person. Consequently, conflicts among sources become inevitable. To resolve the conflicts and achieve high quality data, truth discovery and crowdsourcing aggregation have been studied intensively. However, although these two topics have a lot in common, they are studied separately and are applied to different domains. To answer the need of a systematic introduction and comparison of the two topics, we present an organized picture on truth discovery and crowdsourcing aggregation in this tutorial. They are compared on both theory and application levels, and their related areas as well as open questions are discussed.
Demo 2: Information Retrieval, Data Quality, and Provenance
Location: Kona 4
Chair: Tianli Mo


A Topic-based Reviewer Assignment System
Ngai Meng Kou (University of Macau), Leong Hou U (University of Macau), Nikos Mamoulis (University of Hong Kong), Yuhong Li (University of Macau), Ye Li (University of Macau), Zhiguo Gong (University of Macau)
Peer reviewing is a widely accepted mechanism for assessing the quality of submitted articles to scientific conferences or journals. Conference management systems (CMS) are used by conference organizers to invite appropriate reviewers and assign them to submitted papers. Typical CMS rely on paper bids entered by the reviewers and apply simple matching algorithms to compute the paper assignment. In this paper, we demonstrate our Reviewer Assignment System (RAS), which has advanced features compared to broadly used CMSs. First, RAS automatically extracts the profiles of reviewers and submissions in the form of topic vectors. These profiles can be used to automatically assign reviewers to papers without relying on a bidding process, which can be tedious and error-prone. Second, besides supporting classic assignment models (e.g., stable marriage and optimal assignment), RAS includes a recently published assignment model by our research group, which maximizes, for each paper, the coverage of its topics by the profiles of its reviewers. The features of the demonstration include (1) automatic extraction of paper and reviewer profiles, (2) assignment computation by different models, and (3) visualization of the results by different models, in order to assess their effectiveness.


Data Profiling with Metanome
Thorsten Papenbrock (Hasso-Plattner-Institute), Tanja Bergmann (Hasso-Plattner-Institute), Moritz Finke (Hasso-Plattner-Institute), Jakob Zwiener (Hasso-Plattner-Institute), Felix Naumann (Hasso-Plattner-Institute)
Data profiling is the discipline of discovering metadata about given datasets. The metadata itself serve a variety of use cases, such as data integration, data cleansing, or query optimization. Due to the importance of data profiling in practice, many tools have emerged that support data scientists and IT professionals in this task. These tools provide good support for profiling statistics that are easy to compute, but they are usually lacking automatic and efficient discovery of complex statistics, such as inclusion dependencies, unique column combinations, or functional dependencies. We present Metanome, an extensible profiling platform that in- corporates many state-of-the-art profiling algorithms. While Meta- nome is able to calculate simple profiling statistics in relational data, its focus lies on the automatic discovery of complex metadata. Metanome’s goal is to provide novel profiling algorithms from research, perform comparative evaluations, and to support developers in building and testing new algorithms. In addition, Metanome is able to rank profiling results according to various metrics and to visualize the, at times, large metadata sets.


Provenance for SQL through Abstract Interpretation: Value-less, but Worthwhile
Tobias Müller (U Tübingen), Torsten Grust (U Tübingen)
We demonstrate the derivation of fine-grained where- and why-provenance for a rich dialect of SQL that includes recursion, (correlated) subqueries, windows, grouping/aggregation, and the RDBMS’s library of built-in functions. The approach relies on ideas that originate in the programming language community—program slicing and abstract interpretation, in particular. A two-stage process first records a query’s control flow decisions and locations of data access before it derives provenance without consultation of the actual data values (rendering the method largely “value-less”). We will bring an interactive demonstrator that uses this provenance information to make input/output dependencies in real-world SQL queries tangible.


SAASFEE: Scalable Scientific Workflow Execution Engine
Marc Bux (Humboldt-Universität zu Berlin), Jörgen Brandt (Humboldt-Universität zu Berlin), Carsten Lipka (Humboldt-Universität zu Berlin), Kamal Hakimzadeh (KTH Royal Institute of Technology), Jim Dowling (KTH Royal Institute of Technology), Ulf Leser (Humboldt Universität zu Berlin)
Across many fields of science, primary data sets like sensor read-outs, time series, and genomic sequences are analyzed by complex chains of specialized tools and scripts exchanging intermediate results in domain-specific file formats. Scientific workflow management systems (SWfMSs) support the development and execution of these tool chains by providing workflow specification languages, graphical editors, fault-tolerant execution engines, etc. However, many SWfMSs are not prepared to handle large data sets because of inadequate support for distributed computing. On the other hand, most SWfMSs that do support distributed computing only allow static task execution orders. We present SAASFEE, a SWfMS which runs arbitrarily complex work- flows on Hadoop YARN. Workflows are specified in Cuneiform, a functional workflow language focusing on parallelization and easy integration of existing software. Cuneiform workflows are executed on Hi-WAY, a higher-level scheduler for running workflows on YARN. Distinct features of SAASFEE are the ability to execute iterative workflows, an adaptive task scheduler, re-executable provenance traces, and compatibility to selected other workflow systems. In the demonstration, we present all components of SAASFEE using real-life workflows from the field of genomics.


QOCO: A Query Oriented Data Cleaning System with Oracles
Moria Bergman (Tel Aviv University), Tova Milo (Tel Aviv University), Slava Novgorodov (Tel Aviv University), Wang-Chiew Tan (University of California Santa Cruz)
As key decisions are often made based on information contained in a database, it is important for the database to be as complete and correct as possible. For this reason, many data cleaning tools have been developed to automatically resolve inconsistencies in databases. However, data cleaning tools provide only best-effort results and usually cannot eradicate all errors that may exist in a database. Even more importantly, existing data cleaning tools do not typically address the problem of determining what information is missing from a database. To tackle these problems, we present QOCO, a novel query oriented cleaning system that leverages materialized views that are defined by user queries as a trigger for identifying the remaining incorrect/missing information. Given a user query, QOCO inter- acts with domain experts (which we model as oracle crowds) to identify potentially wrong or missing answers in the result of the user query, as well as determine and correct the wrong data that is the cause for the error(s). We will demonstrate QOCO over a World Cup Games database, and illustrate the interaction between QOCO and the oracles. Our demo audience will play the role of oracles, and we show how QOCO’s underlying operations and optimization mechanisms can effectively prune the search space and minimize the number of questions that need to be posed to accelerate the cleaning process.


Collaborative Data Analytics with DataHub
Anant Bhardwaj (MIT), Amol Deshpande (University of Maryland), Aaron Elmore (University of Chicago), David Karger (MIT),Sam Madden (MIT), Aditya Parameswaran (University of Illinois at Urbana Champaign), Harihar Subramanyam (MIT), Eugene Wu (Columbia), Rebecca Zhang (MIT)
While there have been many solutions proposed for storing and analyzing large volumes of data, all of these solutions have limited support for collaborative data analytics, especially given the many individuals and teams are simultaneously analyzing, modifying and exchanging datasets, employing a number of heterogeneous tools or languages for data analysis, and writing scripts to clean, preprocess, or query data. We demonstrate DataHub, a unified platform with the ability to load, store, query, collaboratively analyze, interactively visualize, interface with external applications, and share datasets. We will demonstrate the following aspects of the DataHub platform: (a) flexible data storage, sharing, and native version- ing capabilities: multiple conference attendees can concurrently update the database and browse the different versions and inspect conflicts; (b) an app ecosystem that hosts apps for various data- processing activities: conference attendees will be able to effortlessly ingest, query, and visualize data using our existing apps; (c) thrift-based data serialization permits data analysis in any combination of 20+ languages, with DataHub as the common data store: conference attendees will be able to analyze datasets in R, Python, and Matlab, while the inputs and the results are still stored in DataHub. In particular, conference attendees will be able to use the DataHub notebook — an IPython-based notebook for analyzing data and storing the results of data analysis.


Mindtagger: A Demonstration of Data Labeling in Knowledge Base Construction
Jaeho Shin (Stanford University), Christopher Re (Stanford University), Mike Cafarella (University of Michigan)
End-to-end knowledge base construction systems using statistical inference are enabling more people to automatically extract high-quality domain-specific information from un- structured data. As a result of deploying DeepDive framework across several domains, we found new challenges in debugging and improving such end-to-end systems to construct high-quality knowledge bases. DeepDive has an iterative development cycle in which users improve the data. To help our users, we needed to develop principles for analyzing the system’s error as well as provide tooling for inspecting and labeling various data products of the system. We created guidelines for error analysis modeled after our colleagues’ best practices, in which data labeling plays a critical role in every step of the analysis. To enable more productive and systematic data labeling, we created Mindtagger, a versatile tool that can be configured to support a wide range of tasks. In this demonstration, we show in detail what data labeling tasks are modeled in our error analysis guidelines and how each of them is performed using Mindtagger.


Annotating Database Schemas to Help Enterprise Search
Eli Cortez (Microsoft), Philip Bernstein (Microsoft), Yeye He (Microsoft Research), Lev Novik (Microsoft)
In large enterprises, data discovery is a common problem faced by users who need to find relevant information in relational databases. In this scenario, schema annotation is a useful tool to enrich a database schema with descriptive keywords. In this paper, we demonstrate Barcelos, a system that automatically annotates corporate databases. Unlike existing annotation approaches that use Web oriented knowledge bases, Barcelos mines enterprise spreadsheets to find candidate annotations. Our experimental evaluation shows that Barcelos produces high quality annotations; the top-5 have an average precision of 87%.


KATARA: Reliable Data Cleaning with Knowledge Bases and Crowdsourcing
Xu Chu (University of Waterloo), John Morcos (University of Waterloo), Ihab Ilyas (University of Waterloo), Mourad Ouzzani (QCRI), Paolo Papotti (QCRI), Nan Tang (QCRI), Yin Ye (Google)
Data cleaning with guaranteed reliability is hard to achieve without accessing external sources, since the truth is not necessarily discoverable from the data at hand. Furthermore, even in the presence of external sources, mainly knowledge bases and humans, effectively leveraging them still faces many challenges, such as aligning heterogeneous data sources and decomposing a complex task into simpler units that can be consumed by humans. We present Katara, a novel end-to-end data cleaning system powered by knowledge bases and crowdsourcing. Given a table, a kb, and a crowd, Katara (i) interprets the table semantics w.r.t. the given kb; (ii) identifies correct and wrong data; and (iii) generates top-k possible repairs for the wrong data. Users will have the opportunity to experience the following features of Katara: (1) Easy specification: Users can define a Katara job with a browser-based specification; (2) Pattern validation: Users can help the system to resolve the ambiguity of different table patterns (i.e., table semantics) discovered by Katara; (3) Data annotation: Users can play the role of internal crowd workers, helping Katara annotate data. Moreover, Katara will visualize the annotated data as correct data validated by the kb, correct data jointly validated by the kb and the crowd, or erroneous tuples along with their possible repairs.


Gain Control over your Integration Evaluations
Patricia Arocena (University of Toronto), Radu Ciucanu (University of Lille (INRIA), Boris Glavic (IIT), Renee Miller (University Toronto)
Integration systems are typically evaluated using a few real-world scenarios (e.g., bibliographical or biological datasets) or using synthetic scenarios (e.g., based on star-schemas or other patterns for schemas and constraints). Reusing such evaluations is a cumbersome task because their focus is usually limited to showcasing a specific feature of an approach. This makes it difficult to compare integration solutions, understand their generality, and understand their performance for different application scenarios. Based on this observation, we demonstrate some of the requirements for develop- ing integration benchmarks. We argue that the major abstractions used for integration problems have converged in the last decade which enables the application of robust empirical methods to integration problems (from schema evolution, to data exchange, to answering queries using views and many more). Specifically, we demonstrate that schema mappings are the main abstraction that now drives most integration solutions and show how a metadata generator can be used to create more credible evaluations of the performance and scalability of data integration systems. We will use the demonstration to evangelize for more robust, shared empirical evaluations of data integration systems.


Janiform Intra-Document Analytics for Reproducible Research
Jens Dittrich (Saarland University), Patrick Bender (Saarland University)
Peer-reviewed publication of research papers is a cornerstone of science. However, one of the many issues of our publication culture is that our publications only publish a summary of the final result of a long project. This means that we put well-polished graphs de- scribing (some) of our experimental results into our publications. However, the algorithms, input datasets, benchmarks, raw result datasets, as well as scripts that were used to produce the graphs in the first place are rarely published and typically not available to other researchers. Often they are only available when personally asking the authors. In many cases, however, they are not available at all. This means from a long workflow that led to producing a graph for a research paper, we only publish the final result rather than the entire workflow. This is unfortunate and has been criticized in various scientific communities. In this demo we argue that one part of the problem is our dated view on what a “document” and hence “a publication” is, should, and can be. As a remedy, we introduce portable database files (PDbF). These files are janiform, i.e. they are at the same time a standard static pdf as well as a highly dynamic (offline) HTML-document. PDbFs allow you to access the raw data behind a graph, perform OLAP-style analysis, and reproduce your own graphs from the raw data — all of this within a portable document. We demo a tool allowing you to create PDbFs smoothly from within LATEX. This tool allows you to preserve the workflow of raw measurement data to its final graph- ical output through all processing steps. Notice that this pdf al- ready showcases our technology: rename this file to “.html” and see what happens (currently we support the desktop versions of Firefox, Chrome, and Safari). But please: do not try to rename this file to “.ova” and mount it in VirtualBox.


EFQ: Why-Not Answer Polynomials in Action
Katerina Tzompanaki (Université Paris Sud), Nicole Bidoit (Université Paris Sud - INRIA), Melanie Herschel (University of Stuttgart)
One important issue in modern database applications is supporting the user with efficient tools to debug and fix queries because such tasks are both time and skill demanding. One particular problem is known as Why-Not question and focusses on the reasons for missing tuples from query results. The EFQ platform demonstrated here has been designed in this context to efficiently leverage Why- Not Answers polynomials, a novel approach that provides the user with complete explanations to Why-Not questions and allows for automatic, relevant query refinements.


Error Diagnosis and Data Profiling with Data X-Ray
Xiaolan Wang (University of Massachusetts Amherst), Mary Feng (University of Massachusetts Amherst and University of Iowa), Yue Wang (University of Massachusetts Amherst), Xin Luna Dong (Google Inc), Alexandra Meliou (University of Massachusetts Amherst)
The problem of identifying and repairing data errors has been an area of persistent focus in data management research. However, while traditional data cleaning techniques can be effective at identifying several data discrepancies, they disregard the fact that many errors are systematic, inherent to the process that produces the data, and thus will keep occurring unless the root cause is identified and corrected. In this demonstration, we will present a large-scale diagnostic framework called DATAXRAY. Like a medical X-ray that aids the diagnosis of medical conditions by revealing problems underneath the surface, DATAXRAY reveals hidden connections and common properties among data errors. Thus, in contrast to traditional clean- ing methods, which treat the symptoms, our system investigates the underlying conditions that cause the errors. The core of DATAXRAY combines an intuitive and principled cost model derived by Bayesian analysis, and an efficient, highly- parallelizable diagnostic algorithm that discovers common proper- ties among erroneous data elements in a top-down fashion. Our system has a simple interface that allows users to load different datasets, to interactively adjust key diagnostic parameters, to explore the derived diagnoses, and to compare with solutions produced by alternative algorithms. Through this demonstration, participants will understand (1) the characteristics of good diagnoses, (2) how and why errors occur in real-world datasets, and (3) the distinctions with other related problems and approaches.


A Demonstration of TripleProv: Tracking and Querying Provenance over Web Data
Marcin Wylot (University of Fribourg), Philippe Cudré-Mauroux (University of Fribourg), Paul Groth (Elsevir Labs)
The proliferation of heterogeneous Linked Data on the Web poses new challenges to database systems. In particular, the capacity to store, track, and query provenance data is becoming a pivotal feature of modern triple stores. In this demonstration, we present TripleProv: a new system extending a native RDF store to efficiently handle the storage, tracking and querying of provenance in RDF data. In the following, we give an overview of our approach providing a reliable and understandable specification of the way results were derived from the data and how particular pieces of data were combined to answer the query. Subsequently, we present techniques enabling to tailor queries with provenance data. Finally, we describe our demonstration and how the attendees will be able to interact with our system during the conference.


WADaR: Joint Wrapper and Data Repair
Stefano Ortona (University of Oxford), Giorgio Orsi (University of Oxford), Marcello Buoncristiano (Universita della Basilicata), Tim Furche (University of Oxford)
Web scraping (or wrapping) is a popular means for acquiring data from the web. Recent advancements have made scalable wrapper-generation possible and enabled data acquisition processes involving thousands of sources. This makes wrapper analysis and maintenance both needed and challenging as no scalable tools exists that support these tasks. We demonstrate WADaR, a scalable and highly auto- mated tool for joint wrapper and data repair. WADaR uses off-the-shelf entity recognisers to locate target entities in wrapper-generated data. Markov chains are used to deter- mine structural repairs, that are then encoded into suitable repairs for both the data and corresponding wrappers. We show that WADaR is able to increase the quality of wrapper-generated relations between 15% and 60%, and to fully repair the corresponding wrapper without any knowledge of the original website in more than 50% of the cases.


Wisteria: Nurturing Scalable Data Cleaning Infrastructure
Daniel Haas (UC Berkeley), Sanjay Krishnan (UC Berkeley), Jiannan Wang (UC Berkeley), Michael Franklin (UC Berkeley), Eugene Wu (Columbia University)
Analysts report spending upwards of 80% of their time on problems in data cleaning. The data cleaning process is inherently iterative, with evolving cleaning workflows that start with basic exploratory data analysis on small samples of dirty data, then refine analysis with more sophisticated/expensive cleaning operators (e.g., crowd- sourcing), and finally apply the insights to a full dataset. While an analyst often knows at a logical level what operations need to be done, they often have to manage a large search space of physical operators and parameters. We present Wisteria, a system designed to support the iterative development and optimization of data clean- ing workflows, especially ones that utilize the crowd. Wisteria separates logical operations from physical implementations, and driven by analyst feedback, suggests optimizations and/or replace- ments to the analyst’s choice of physical implementation. We high- light research challenges in sampling, in-flight operator replace- ment, and crowdsourcing. We overview the system architecture and these techniques, then provide a demonstration designed to show- case how Wisteria can improve iterative data analysis and cleaning. The code is available at: http://www.sampleclean.org.
Thursday Sep 3rd 12:00-13:30
Lunch
Location: Lagoon Lanai
Thursday Sep 3rd 13:30-15:00
Research 25: Probabilistic Data Processing and Approximation
Location: Kings 1
Chair: Daisy Zhe Wang


Auto-Approximation of Graph Computing
Zechao Shang (Chinese University of Hong Kong), Jeffrey Xu Yu (Chinese University of Hong Kong)
In the big data era, graph computing is one of the challenging issues because there are numerous large graph datasets emerging from real applications. A question is: do we need to know the final exact answer for a large graph? When it is impossible to know the exact answer in a limited time, is it possible to approximate the final answer in an automatic and systematic way without having to designing new approximate algorithms? The main idea behind the question is: it is more important to find out something meaningful quick from a large graph, and we should focus on finding a way of making use of large graphs instead of spending time on designing approximate algorithms. In this paper, we give an innovative approach which automatically and systematically synthesizes a program to approximate the original program. We show that we can give users some answers with reasonable accuracy and high efficiency for a wide spectrum of graph algorithms, without having to know the details of graph algorithms. We have conducted extensive experimental studies using many graph algorithms that are supported in the existing graph systems and large real graphs. Our extensive experimental results reveal that our automatically approximating approach is highly feasible.


Approximate lifted inference with probabilistic databases
Wolfgang Gatterbauer (Carnegie Mellon University), Dan Suciu (University of Washington)
This paper proposes a new approach for approximate evaluation of #P-hard queries with probabilistic databases. In our approach, every query is evaluated entirely in the database engine by evaluating a fixed number of query plans, each providing an upper bound on the true probability, then taking their minimum. We provide an algorithm that takes into account important schema information to enumerate only the minimal necessary plans among all possible plans. Importantly, this algorithm is a strict generalization of all known results of PTIME self-join-free conjunctive queries: A query is safe if and only if our algorithm returns one single plan. We also apply three relational query optimization techniques to evaluate all minimal safe plans very fast. We give a detailed experimental evaluation of our approach and, in the process, provide a new way of thinking about the value of probabilistic methods over non-probabilistic methods for ranking query answers.


Incremental Knowledge Base Construction Using DeepDive
Jaeho Shin (Stanford University), Sen Wu (Stanford University), Feiran Wang (Stanford University), Christopher De Sa (Stanford University), Ce Zhang (University of Wisconsin-Madison), Christopher Re (Stanford University)
Populating a database with unstructured information is a long-standing problem in industry and research that encompasses problems of extraction, cleaning, and integration. Recent names used for this problem include dealing with dark data and knowledge base construction (KBC). In this work, we describe DeepDive, a system that combines database and machine learning ideas to help develop KBC systems, and we present techniques to make the KBC process more efficient. We observe that the KBC process is iterative, and we develop techniques to incrementally produce inference results for KBC systems. We propose two methods for incremental inference, based respectively on sampling and variational techniques. We also study the tradeoff space of these methods and develop a simple rule-based optimizer. DeepDive includes all of these contributions, and we evaluate DeepDive on five KBC systems, showing that it can speed up KBC inference tasks by up to two orders of magnitude with negligible impact on quality.


Lenses: An On-Demand Approach to ETL
Ying Yang (SUNY Buffalo), Niccolò Meneghetti (SUNY Buffalo), Ronny Fehling (Oracle), Zhen Hua Liu (Oracle), Oliver Kennedy (SUNY Buffalo)
Three mentalities have emerged in analytics. One view holds that reliable analytics is impossible without high-quality data, and relies on heavy-duty ETL processes and upfront data curation to provide it. The other view takes a more ad-hoc approach, collecting data into a data lake, and placing responsibility for data quality on the analyst querying it. A third, on-demand approach has emerged over the past decade in the form of numerous systems such as Paygo or HLog that allow for incremental curation of the data and help analysts to make principled trade-offs between data quality and effort. Though quite useful in isolation, these systems target only specific quality problems (e.g., Paygo targets only schema matching and entity resolution). In this paper, we explore the design of a general, extensible infrastructure for on-demand curation that is based on probabilistic query processing. We illustrate its generality through examples and show how such an infrastructure can be used to gracefully make existing ETL workflows ``on-demand''. Finally, we present a user interface for On-Demand ETL and address ensuing challenges, including a greedy strategy for efficiently ranking potential data curation tasks. Our experimental results that show that On-Demand ETL is feasible and that our greedy ranking strategy, called CPI, is effective.


Knowledge-Based Trust: A Method to Estimate the Trustworthiness of Web Sources
Xin Luna Dong* (Google Inc), Evgeniy Gabrilovich (Google Inc.), Kevin Murphy (Google Inc.), Van Dang (Google Inc.), Wilko Horn (Google Inc.), Camillo Lugaresi (Google Inc.), Shaohua Sun (Google Inc.), Wei Zhang (Google Inc.)
The quality of web sources has been traditionally evaluated using exogenous signals such as the hyperlink structure of the graph. We propose a new approach that relies on endogenous signals, namely, the correctness of factual information provided by the source. A source that has few false facts is considered to be trustworthy. The facts are automatically extracted from each source by information extraction methods commonly used to construct knowledge bases. We propose a way to distinguish errors made in the extraction process from errors in the web source itself, by using joint inference in a novel multi-layer probabilistic model. On synthetic data, we show that our method can reliably recover the true trustworthiness levels of the sources. We then applied it to a database of 2.8B facts extracted from the web, and thereby estimated the trustworthiness of 188M webpages. Manual evaluation of some of the results confirms the effectiveness of the method.
Research 26: Query Processing 2
Location: Kings 2
Chair: Ken Salem


DAQ: A New Paradigm for Approximate Query Processing
Navneet Potti (University of Wisconsin-Madison), Jignesh Patel (University of Wisconsin-Madison)
Many modern applications deal with exponentially increasing data volumes and aid business-critical decisions in near real-time. Particularly in exploratory data analysis, the focus is on interactive querying and some degree of error in estimated results is tolerable. A common response to this challenge is approximate query processing, where the user is presented with a quick confidence interval estimate based on a sample of the data. In this work, we highlight some of the problems that are associated with this probabilistic approach when extended to more complex queries, both in semantic interpretation and the lack of a formal algebra. As an alternative, we propose deterministic approximate querying (DAQ) schemes, formalize a closed deterministic approximation algebra, and outline some design principles for DAQ schemes. We also illustrate the utility of this approach with an example deterministic online approximation scheme which uses a bitsliced index representation and computes the most significant bits of the result first. Our prototype scheme delivers speedups over exact aggregation and predicate evaluation, and outperforms sampling-based schemes for extreme value aggregations.


On the Surprising Difficulty of Simple Things: the Case of Radix Partitioning
Felix Schuhknecht (Saarland University), Pankaj Khanchandani (Saarland University), Jens Dittrich (Saarland University)
Partitioning a dataset into ranges is a task that is common in various applications such as sorting and hashing which are in turn building blocks for almost any type of query processing. Especially radix-based partitioning is very popular due to its simplicity and high performance over comparison-based versions. In its most primitive form, coined original version from here on, it partitions a dataset into 2^R (where R ≤ 32) partitions: in the first pass over the data, we count for each partition the number of entries that will be sent to it. From this generated histogram, we calculate the start index of each partition. The second pass over the data finally copies the entries to their designated partitions. Despite of its simple nature, several interesting techniques can be applied to enhance this algorithm such as software-managed buffers, non-temporal streaming operations, prefetching, and memory layout with many variables having an influence on the performance like buffer sizes, number of partitions, and page sizes. Although being heavily used in the database literature, it is unclear how these techniques individually contribute to the performance of partitioning. Therefore, in this work we will incrementally extend the original version by the mentioned optimizations to carefully analyze the individual impact on the partitioning process. As a result this paper provides a strong guideline on when to use which optimization for partitioning.


Efficient Processing of Window Functions in Analytical SQL Queries
Viktor Leis (TU Munich), Kan Kundhikanjana (TU Munich), Alfons Kemper (TU Munich), Thomas Neumann (TU Munich)
Window functions, also known as analytic OLAP functions, have been part of the SQL standard for more than a decade and are now a widely used feature. Window functions allow to elegantly express many useful query types including time series analysis, ranking, percentiles, moving averages, and cumulative sums. Formulating such queries in plain SQL-92 is usually both cumbersome and inefficient. Despite being supported by all major database systems, there have been few publications that describe how to implement an efficient relational window operator. This work aims at filling this gap by presenting an efficient and general algorithm for the window operator. Our algorithm is optimized for high-performance main-memory database systems and has excellent performance on modern multi-core CPUs. We show how to fully parallelize all phases of the operator in order to effectively scale for arbitrary input distributions.


Scaling Similarity Joins over Tree-Structured Data
Yu Tang (University of Hong Kong and EPFL), Yilun Cai (University of Hong Kong), Nikos Mamoulis (University of Hong Kong)
Given a large collection of tree-structured objects (e.g., XML documents), the similarity join finds the pairs of objects that are similar to each other, based on a similarity threshold and a tree edit distance measure. The state-of-the-art similarity join methods compare simpler approximations of the objects (e.g., strings), in order to prune pairs that cannot be part of the similarity join result based on distance bounds derived by the approximations. In this paper, we propose a novel similarity join approach, which is based on the dynamic decomposition of the tree objects into subgraphs, according to the similarity threshold. Our technique avoids computing the exact distance between two tree objects, if the objects do not share at least one common subgraph. In order to scale up the join, the computed subgraphs are managed in a two-layer index. Our experimental results on real and synthetic data collections show that our approach outperforms the state-of-the-art methods by up to an order of magnitude.


Processing of Probabilistic Skyline Queries Using MapReduce
Yoonjae Park (Seoul National University), Jun-Ki Min (Korea University of Technology and Education), Kyuseok Shim (Seoul National University)
There has been an increased growth in a number of applications that naturally generate large volumes of uncertain data. By the advent of such applications, the support of advanced analysis queries such as the skyline and its variant operators for big uncertain data has become important. In this paper, we propose the effective parallel algorithms using MapReduce to process the probabilistic skyline queries for uncertain data modeled by both discrete and continuous models. We present three filtering methods to identify probabilistic non-skyline objects in advance. We next develop a single MapReduce phase algorithm PS-QP-MR by utilizing space partitioning based on a variant of quadtrees to distribute the instances of objects effectively and the enhanced algorithm PS-QPF-MR by applying the three filtering methods additionally. We also propose the workload balancing technique to balance the workload of reduce functions based on the number of machines available. Finally, we present the brute-force algorithms PS-BR-MR and PS-BRF-MR with partitioning randomly and applying the filtering methods. In our experiments, we demonstrate the efficiency and scalability of PS-QPF-MR compared to the other algorithms.
Industrial 7: Privacy and Visualization
Location: Kings 3
Chair: Khan Arijit


Differential Privacy in Telco Big Data Platform
Xueyang Hu (Shanghai Jiao Tong University and Huawei Noah's Ark Lab), Mingxuan Yuan (Huawei Noah's Ark Lab), Jianguo Yao (Shanghai Jiao Tong University), Yu Deng,Shanghai Jiao Tong University), Lei Chen (Hong Kong University of Science and Technology), Haibing Guan (Shanghai Jiao Tong Univerisity), Jia Zeng (Soochow University and Huawei Noah's Ark Lab)
Differential privacy (DP) has been widely explored in academia recently but less so in industry possibly due to its strong privacy guarantee. This paper makes the first attempt to implement three basic DP architectures in the deployed telecommunication (telco) big data platform for data mining applications such as the churn prediction. We find that all DP architectures have less than $5\%$ loss of prediction accuracy when the weak privacy guarantee is adopted (e.g., privacy budget parameter $\epsilon \geq 3$). However, when the strong privacy guarantee is assumed (e.g., privacy budget parameter $\epsilon \le 0.1$), all DP architectures lead to $15\% \sim 30\%$ accuracy loss, which implies that real-word industrial data mining systems cannot work well under such a strong privacy guarantee recommended by previous research work. Among the three basic DP architectures, the Hybridized DM (Data Mining) and DB (Database) architecture performs the best because of its complicated privacy protection design for the specific data mining algorithm. Through extensive experiments on big data, we also observe that the accuracy loss increases by increasing the variety of features, but decreases by increasing the volume of training data. Therefore, to make DP used practically in large-scale industrial systems, our observations suggest that we may explore three possible research directions in future:(1) Relax/adjust privacy guarantee (e.g., increasing privacy budget $\epsilon$) and study its effectiveness on specific industrial applications; (2) Design specific privacy scheme for a certain data mining algorithm; and (3) Use large volume of data but with low variety for classifier training.


Efficient Evaluation of Object-Centric Exploration Queries for Visualization
You Wu (Duke University), Boulos Harb (Google Inc.), Jun Yang (Duke University), Cong Yu (Google Research)
The most effective way to explore data is through visualizing the results of exploration queries. For example, an exploration query could be an aggregate of some measures over time intervals, and a pattern or abnormality can be discovered through a time series plot of the query results. In this paper, we examine a special kind of exploration query, namely object-centric exploration query. Common examples include claims made about athletes in sports databases, such as “it is newsworthy that LeBron James has scored 35 or more points in nine consecutive games.” We focus on one common type of visualization, i.e., 2d scatter plot with heatmap. Namely, we consider exploration queries whose results can be plotted on a two-dimensional space, possibly with colors indicating object densities in regions. While we model results as pairs of numbers, the types of the queries are limited only by the users’ imagination. In the LeBron James example above, the two dimensions are minimum points scored per game and number of consecutive games, respectively. It is easy to find other equally interesting dimensions, such as minimum rebounds per game or number of playoff games. We formalize this problem and propose an efficient, interactive speed algorithm that takes a user-provided exploration query (which can be a blackbox function) and produces an approximate visualization that preserves the two most important visual properties: the outliers and the overall distribution of all result points.
Research 27: Data Warehousing, Search, and Ranking
Location: Queens 4
Chair: H.V. Jagadish


Interpretable and Informative Explanations of Outcomes
Kareem El Gebaly (University of Waterloo), Parag Agrawal (Twitter), Lukasz Golab (University of Waterloo), Flip Korn (Google), Divesh Srivastava (AT&T Labs-Research)
In this paper, we solve the following data summarization problem: given a multi-dimensional data set augmented with a binary attribute, how can we construct an interpretable and informative summary of the factors affecting the binary attribute in terms of the combinations of values of the dimension attributes? We refer to such summaries as explanation tables. We show the hardness of constructing optimally-informative explanation tables from data, and we propose effective and efficient heuristics. The proposed heuristics are based on sampling and include optimizations related to computing the information content of a summary from a sample of the data. Using real data sets, we demonstrate the advantages of explanation tables compared to related approaches that can be adapted to solve our problem, and we show significant performance benefits of our optimizations.


Stale View Cleaning: Getting Fresh Answers from Stale Materialized Views
Sanjay Krishnan (UC Berkeley), Jiannan Wang (UC Berkeley), Michael Franklin (UC Berkeley), Ken Goldberg (UC Berkeley), Tim Kraska (Brown University)
Materialized views (MVs), stored pre-computed results, are widely used to facilitate fast queries on large datasets. When new records arrive at a high rate, it is infeasible to continuously update (maintain) MVs and a common solution is to defer maintenance by batching updates together. Between batches the MV becomes increasingly stale with incorrect, missing, and superfluous rows leading to increasingly inaccurate query results. We propose Stale View Cleaning (SVC) which addresses this problem from a data cleaning perspective. We take inspiration from recent results in data cleaning which combine sampling and cleaning for accurate query processing. In SVC, we efficiently clean a sample of rows from a stale MV, and use the clean sample to compute a query result correction to compensate for the dirtiness. While approximate, the corrected query results reflect the most recent data. SVC supports a wide variety of materialized views and aggregate queries on those views with optimality for SUM, COUNT, AVG. As sampling can be sensitive to long-tailed distributions, we further explore an outlier indexing technique to give increased accuracy when the data distributions are skewed. SVC complements existing deferred maintenance approaches by giving accurate and bounded query answers between maintenance. We evaluate our method on a real dataset of workloads from the TPC-D benchmark and a real video distribution application. Our experiments confirm our theoretical results: (1) cleaning an MV sample is more efficient than full view maintenance, (2) the corrected results are more accurate than using the stale MV, and (3) SVC can be efficiently integrated with deferred maintenance.


Scalable Topical Phrase Mining from Text Corpora
Ahmed El-Kishky (University of Illinois at Urbana Champaign), Yanglei Song (University of Illinois at Urbana Champaign), Chi Wang (Microsoft Research), Clare Voss (Army Research Laboratory), Jiawei Han (University of Illinois at Urbana Champaign)
While most topic modeling algorithms model text corpora with unigrams, human interpretation often relies on inherent grouping of terms into phrases. As such, we consider the problem of discovering topical phrases of mixed lengths. Existing work either performs post processing to the results of unigram-based topic models, or utilizes complex n-gram-discovery topic models. These methods generally produce low-quality topical phrases or suffer from poor scalability on even moderately-sized datasets. We propose a different approach that is both computationally efficient and effective. Our solution combines a novel phrase mining framework to segment a document into single and multi-word phrases, and a new topic model that operates on the induced document partition. Our approach discovers high quality topical phrases with negligible extra cost to the bag-of-words topic model in a variety of datasets including research publication titles, abstracts, reviews, and news articles.


Maximum Rank Query
Kyriakos Mouratidis (Singapore Management University), Jilian Zhang (Singapore Management University), HweeHwa Pang (Singapore Management University)
The top-k query is a common means to shortlist a number of options from a set of alternatives, based on the user's preferences. Typically, these preferences are expressed as a vector of query weights, defined over the options' attributes. The query vector implicitly associates each alternative with a numeric score, and thus imposes a ranking among them. The top-k result includes the k options with the highest scores. In this context, we define the maximum rank query (MaxRank). Given a focal option in a set of alternatives, the MaxRank problem is to compute the highest rank this option may achieve under any possible user preference, and furthermore, to report all the regions in the query vector's domain where that rank is achieved. MaxRank finds application in market impact analysis, customer profiling, targeted advertising, etc. We propose a methodology for MaxRank processing and evaluate it with experiments on real and benchmark synthetic datasets.


A Confidence-Aware Approach for Truth Discovery on Long-Tail Data
Qi Li (SUNY Buffalo), Yaliang Li (SUNY Buffalo), Jing Gao (SUNY Buffalo), Lu Su (SUNY Buffalo), Bo Zhao (Microsoft Research), Murat Demirbas (SUNY Buffalo), Wei Fan (Huawei Noah's Ark Lab), Jiawei Han (UIUC)
In many real world applications, the same item may be described by multiple sources. As a consequence, conflicts among these sources are inevitable, which leads to an important task: how to identify which piece of information is trustworthy, i.e., the truth discovery task. Intuitively, if the piece of information is from a reliable source, then it is more trustworthy, and the source that provides trustworthy information is more reliable. Based on this principle, truth discovery approaches have been proposed to infer source reliability degrees and the most trustworthy information (i.e., the truth) simultaneously. However, existing approaches overlook the ubiquitous long-tail phenomenon in the tasks, i.e., most sources only provide a few claims and only a few sources make plenty of claims, which causes the source reliability estimation for small sources to be unreasonable. To tackle this challenge, we propose a confidence-aware truth discovery (CATD) method to automatically detect truths from conflicting data with long-tail phenomenon. The proposed method not only estimates source reliability, but also considers the confidence interval of the estimation, so that it can effectively reflect real source reliability for sources with various levels of participation. Experiments on four real world tasks as well as simulated multi-source long-tail datasets demonstrate that the proposed method outperforms existing state-of-the-art truth discovery approaches by successful discounting the effect of small sources.
Research 28: Novel DB Architectures, Novel Hardware, and Resource Management
Location: Queens 5
Chair: Asterios Katsifodimos


An Architecture for Compiling UDF-centric Workflows
Andrew Crotty (Brown University), Alex Galakatos (Brown University), Kayhan Dursun (Brown University), Tim Kraska (Brown University), Carsten Binnig (Brown University), Ugur Cetintemel (Brown University), Stan Zdonik (Brown University)
Data analytics has recently grown to include increasingly sophisticated techniques, such as machine learning and advanced statistics. Users frequently express these complex analytics tasks as workflows of user-defined functions (UDFs) that specify each algorithmic step. However, given typical hardware configurations and dataset sizes, the core challenge of complex analytics is no longer sheer data volume but rather the computation itself, and the next generation of analytics frameworks must focus on optimizing for this computation bottleneck. While query compilation has gained widespread popularity as a way to tackle the computation bottleneck for traditional SQL workloads, relatively little work addresses UDF-centric workflows in the domain of complex analytics. In this paper, we describe a novel architecture for automatically compiling workflows of UDFs. We also propose several optimizations that consider properties of the data, UDFs, and hardware together in order to generate different code on a case-by-case basis. To evaluate our approach, we implemented these techniques in Tupleware, a new high-performance distributed analytics system, and our benchmarks show performance improvements of up to three orders of magnitude compared to alternative systems.


Take me to your leader! Online Optimization of Distributed Storage Configurations
Artyom Sharov (Technion Israel Institute of Technology), Alexander Shraer (Google), Arif Merchant (Google), Murray Stokely (Google)
The configuration of a distributed storage system typically includes, among other parameters, the set of servers and their roles in the replication protocol. Although mechanisms for changing the configuration at runtime exist, it is usually left to system administrators to manually determine the "best" configuration and periodically reconfigure the system, often by trial and error. This paper describes a new workload-driven optimization framework that dynamically determines the optimal configuration at runtime. We focus on optimizing leader and quorum based replication schemes and divide the framework into three optimization tiers, dynamically optimizing different configuration aspects: 1) leader placement, 2) roles of different servers in the replication protocol, and 3) replica locations. We showcase our optimization framework by applying it to a large-scale distributed storage system used internally in Google and demonstrate that most client applications significantly benefit from using our framework, reducing average operation latency by up to 94%.


SIMD- and Cache-Friendly Algorithm for Sorting an Array of Structures
Hiroshi Inoue (IBM Research-Tokyo), Kenjiro Taura (University of Tokyo)
This paper describes our new algorithm for sorting an array of structures by efficiently exploiting the SIMD instructions and cache memory of today's processors. Recently, multiway mergesort implemented with SIMD instructions has been used as a high-performance in-memory sorting algorithm for sorting integer values. For sorting an array of structures with SIMD instructions, a frequently used approach is to first pack the key and index for each record into an integer value, sort the key-index pairs using SIMD instructions, then rearrange the records based on the sorted key-index pairs. This approach can efficiently exploit SIMD instructions because it sorts the key-index pairs while packed into integer values; hence, it can use existing high-performance sorting implementations of the SIMD-based multiway mergesort for integers. However, this approach has frequent cache misses in the final rearranging phase due to its random and scattered memory accesses so that this phase limits both single-thread performance and scalability with multiple cores. Our approach is also based on multiway mergesort, but it can avoid costly random accesses for rearranging the records while still efficiently exploiting the SIMD instructions. Our results showed that our approach exhibited up to 2.1x better single-thread performance than the key-index approach implemented with SIMD instructions when sorting 512M 16-byte records on one core. Our approach also yielded better performance when we used multiple cores. Compared to an optimized radix sort, our vectorized multiway mergesort achieved better performance when the each record is large. Our vectorized multiway mergesort also yielded higher scalability with multiple cores than the radix sort.


To Lock, Swap, or Elide: On the Interplay of Hardware Transactional Memory and Lock-Free Indexing
Darko Makreshanski (ETH Zurich), Justin Levandoski (Microsoft Research), Ryan Stutsman (Microsoft Research)
The release of hardware transactional memory (HTM) in commodity CPUs has major implications on the design and implementation of main-memory databases, especially on the architecture of high performance lock-free indexing methods at the core of several of these systems. This paper studies the interplay of HTM and lock-free indexing methods. First, we evaluate whether HTM will obviate the need for crafty lock-free index designs by integrating it in a traditional B-tree architecture. HTM performs well for simple data sets with small fixed-length keys and payloads, but its benefits disappear for more complex scenarios (e.g., larger variable-length keys and payloads), making it unattractive as a general solution for achieving high performance. Second, we explore fundamental differences between HTM-based and lock-free B-tree designs. While lock-freedom entails design complexity and extra mechanism, it has performance advantages in several scenarios, especially high-contention cases where readers proceed uncontested (whereas HTM aborts readers). Finally, we explore the use of HTM as a method to simplify lock-free design. We find that using HTM to implement a multi-word compare-and-swap greatly reduces lock-free programming complexity at the cost of only a 10-15% performance degradation. Our study uses two state-of-the-art index implementations: a memory-optimized B-tree extended with HTM to provide multi-threaded concurrency and the Bw-tree lock-free B-tree used in several Microsoft production environments.


SQLite Optimization with Phase Change Memory for Mobile Applications
Gihwan Oh (Sungkyunkwan Univ), Sangchul Kim (Seoul National University), Sang-Won Lee (Sungkyunkwan University), Bongki Moon (Seoul National University)
Given its pervasive use in smart mobile platforms, it is compelling to improve the sluggish performance of SQLite databases. Popular mobile applications such as messenger, email and social network services rely on SQLite for their data management need. Those mobile applications tend to execute relatively short transactions in the autocommit mode for transactional consistency of databases. This often has adverse effect on the flash memory storage in mobile devices because the small random updates cause high write amplification and high write latency. In order to address this problem, we propose a new optimization strategy, called per-page logging, for mobile data management, and have implemented the key functions in SQLite/PPL. The hardware component of SQLite/PPL includes phase change memory (PCM) with a byte-addressable, persistent memory abstraction. By capturing an update in a physiological log record and adding it to the PCM log sector, SQLite/PPL can replace a multitude of successive page writes against the same logical page with much smaller log writes done to PCM much more efficiently. We have observed that SQLite/PPL would potentially improve the performance of mobile applications by an order of magnitude while supporting transactional atomicity and durability.
Tutorial 7: Real Time Analytics: Algorithms and Systems (1/2)
Location: Queens 6


Real Time Analytics: Algorithms and Systems (1/2)
Arun Kejariwal, Sanjeev Kulkarni, Karthik Ramasamy
Velocity is one of the 4 Vs commonly used to characterize Big Data. In this regard, Forrester remarked the following in Q3 2014: “The high velocity, white-water flow of data from innumerable real-time data sources such as market data, Internet of Things, mobile, sensors, click-stream, and even transactions remain largely unnavigated by most firms. The opportunity to leverage streaming analytics has never been greater.” Example use cases of streaming analytics include, but not limited to: (a) visualization of business metrics in real-time (b) facilitating highly personalized experiences (c) expediting response during emergencies. Streaming analytics is extensively used in a wide variety of domains such as healthcare, e-commerce, financial services, telecommunications, energy and utilities, manufacturing, government and transportation. In this tutorial, we shall present an in-depth overview of streaming analytics – applications, algorithms and platforms – landscape. We shall walk through how the field has evolved over the last decade and then discuss the current challenges – the impact of the other three Vs, viz., Volume, Variety and Veracity, on Big Data streaming analytics. The tutorial is intended for both researchers and practitioners in the industry. We shall also present state-of-the-affairs of streaming analytics at Twitter.
Panel 2: Designing for Interaction: Broadening our View of Working with Data
Location: Kona 1-2-3
Chair: Joe Hellerstein

Designing for Interaction: Broadening our View of Working with Data
Azza Abouzied (NYU-AD), Adam Marcus (Unlimited Labs), Arnab Nandi (Ohio State University), Eugene Wu (Columbia University), Joseph M. Hellerstein (UC Berkeley)
Traditionally, databases and data visualization tools were narrowly focused on individual query-response interactions. This perspective on the way people work with data seems increasingly myopic as time passes. Today, much of the time people spend working with data involves iterative and interactive exploration, transformation and analysis—often via inefficient use of outmoded tools and interaction models. Thoughtful designs for the future need to consider user behaviors and desires in a far broader scope than the narrow query-response paradigm of the past. In this panel, a number of leading young technologists working in this area will take a long view of the future of interacting with data, and discuss exploratory paths to get us from here to there.
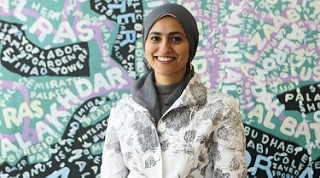
Bio: Azza Abouzied’s research work focuses on designing intuitive data querying tools. Today’s technologies are helping people collect and produce data at phenomenal rates. Despite the abundance of data, it remains largely inaccessible due to the skill required to explore, query and analyze it in a non-trivial fashion. While many users know exactly what they are looking for, they have trouble expressing sophisticated queries in interfaces that require knowledge of a programming language or a query language. Azza designs novel interfaces, such as example-driven query tools, that simplify data querying and analysis. Her research work combines techniques from various research fields such as UI-design, machine learning and databases. Azza Abouzied received her doctoral degree from Yale in 2013. She spent a year as a visiting scholar at UC Berkeley. She is also one of the co-founders of Hadapt – a Big Data analytics platform.
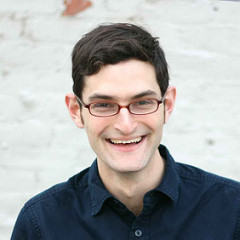
Bio: Adam just cofounded Unlimited Labs, a company dedicated to the future of work. Prior to that, Adam led the data team at Locu, a startup that was acquired by GoDaddy. He completed his Ph.D. in computer science at MIT in 2012, where his dissertation was on database systems and human computation. Adam is a recipient of the NSF and NDSEG fellowships, and has previously worked at ITA, Google, IBM, and FactSet. In his free time, Adam builds course content to get people excited about data and programming.
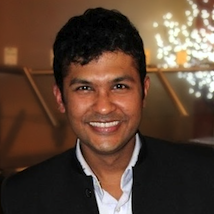
Bio: Arnab’s research is in the area of database systems, focusing on challenges in big data analytics and interactive query interfaces. The goal of his group is to empower humans to effectively interact with data. This involves solving problems that span the areas of databases, visualization, human-computer interaction, and information retrieval.
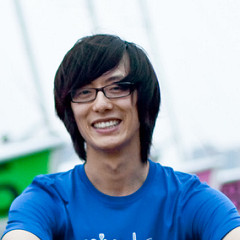
Bio: Eugene is broadly interested in technologies that help users play with their data. His goal is for users at all technical levels to effectively and quickly make sense of their information. He is interested in solutions that ultimately improve the interface between users and data, and techniques borrows from fields such as data management, systems, crowd sourcing, visualization, and HCI. Eugene is starting at Columbia University in Fall of 2015.
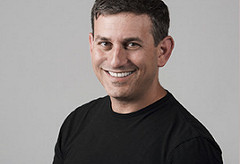
Bio: Joe is a Professor of Computer Science at the University of California, Berkeley, whose work focuses on data-centric systems and the way they drive computing. He is an ACM Fellow, an Alfred P. Sloan Research Fellow and the recipient of three SIGMOD “Test of Time” awards for his research. In 2010, Fortune Magazine included him in their list of 50 smartest people in technology , and MIT’s Technology Review magazine included his work on their TR10 list of the 10 technologies “most likely to change our world”. Joe is also the co-founder and Chief Strategy Officer of Trifacta, a software vendor providing intelligent interactive solutions to the messy problems of wrangling data. He serves on the technical advisory boards of a number of computing and Internet companies including EMC, SurveyMonkey, Captricity, and Dato, and previously served as the Director of Intel Research, Berkeley.
Thursday Sep 3rd 15:00-15:30
Refreshments: Coffee Break
Location: Grand Promenade
Thursday Sep 3rd 15:30-17:00
Research 29: Privacy and Security
Location: Kings 1
Chair: Johann Christoph Freytag


Practical Authenticated Pattern Matching with Optimal Proof Size
Dimitrios Papadopoulos (Boston University), Charalampos Papamanthou (University of Maryland), Roberto Tamassia (Brown University), Nikos Triandopoulos (RSA Laboratories and Boston University)
We address the problem of authenticating pattern matching queries over textual data that is outsourced to an untrusted cloud server. By employing cryptographic accumulators in a novel optimal integrity-checking tool built directly over a suffix tree, we design the first authenticated data structure for verifiable answers to pattern matching queries featuring fast generation of constant-size proofs. We present two main applications of our new construction to authenticate: (i) pattern matching queries over text documents, and (ii) exact path queries over XML documents. Answers to queries are verified by proofs of size at most 500 bytes for text pattern matching, and at most 243 bytes for exact path XML search, independently of the document or answer size. By design, our authentication schemes can also be parallelized to offer extra efficiency during data outsourcing. We provide a detailed experimental evaluation of our schemes showing that for both applications the times required to compute and verify a proof are very small—e.g., it takes less than 10μs to generate a proof for a pattern (mis)match of 10^2 characters in a text of 10^6 characters, once the query has been evaluated.


Fast Range Query Processing with Strong Privacy Protection for Cloud Computing
Rui Li (Hunan university), Alex Liu (Michigan State University), Ann Wang (Michigan State University), Bezawada Bruhadeshwar (Nanjing University)
Privacy has been the key road block to cloud computing as clouds may not be fully trusted. This paper concerns the problem of privacy preserving range query processing on clouds. Prior schemes are weak in privacy protection as they cannot achieve index indistinguishability, and therefore allow the cloud to statistically estimate the values of data and queries using domain knowledge and history query results. In this paper, we propose the first range query processing scheme that achieves index indistinguishability under the \emph{indistinguishability against chosen keyword attack} (IND-CKA). Our key idea is to organize indexing elements in a complete binary tree called PBtree, which satisfies \emph{structure indistinguishability} (\ie, two sets of data items have the same PBtree structure if and only if the two sets have the same number of data items) and \emph{node indistinguishability} (\ie, the values of PBtree nodes are completely random and have no statistical meaning). We prove that our scheme is secure under the widely adopted IND-CKA security model. We propose two algorithms, namely PBtree traversal width minimization and PBtree traversal depth minimization, to improve query processing efficiency. We prove that the worse case complexity of our query processing algorithm using PBtree is $O(|R|\log n)$, where $n$ is the total number of data items and $R$ is the set of data items in the query result. We implemented and evaluated our scheme on a real world data set with 5 million items. For example, for a query whose results contain ten data items, it takes only $0.17$ milliseconds.


DPT: Differentially Private Trajectory Synthesis Using Hierarchical Reference Systems
Xi He (Duke University), Graham Cormode (University of Warwick), Ashwin Machanavajjhala (Duke University), Cecilia Procopiuc (AT&T Labs-Research), Divesh Srivastava (AT&T Labs-Research)
GPS-enabled devices are now ubiquitous, from airplanes and cars to smartphones and wearable technology. This has resulted in a wealth of data about the movements of individuals and populations, which can be analyzed for useful information to aid in city and traffic planning, disaster preparedness and so on. However, the places that people go can disclose extremely sensitive information about them, and thus their use needs to be filtered through privacy preserving mechanisms. This turns out to be a highly challenging task: raw trajectories are highly detailed, and typically no pair is alike. Previous attempts fail either to provide adequate privacy protection, or to remain sufficiently faithful to the original behavior. This paper presents DPT, a system to synthesize mobility data based on raw GPS trajectories of individuals while ensuring strong privacy protection in the form of epsilon-differential privacy. DPT makes a number of novel modeling and algorithmic contributions including (i) discretization of raw trajectories using hierarchical reference systems (at multiple resolutions) to capture individual movements at differing speeds, (ii) adaptive mechanisms to select a small set of reference systems and construct prefix tree counts privately, and (iii) use of direction-weighted sampling for improved utility. While there have been prior attempts to solve the subproblems required to generate synthetic trajectories, to the best of our knowledge, ours is the first system that provides an end-to-end solution. We show the efficacy of our synthetic trajectory generation system using an extensive empirical evaluation.


Privacy Implications of Database Ranking
Md Farhadur Rahman (University of Texas at Arlington), Weimo Liu (George Washington University), Saravanan Thirumuruganathan (University of Texas at Arlingt), Nan Zhang (George Washington University), Gautam Das (University of Texas at Arlington)
In recent years, there has been much research in the adoption of Ranked Retrieval model (in addition to the Boolean retrieval model) in structured databases, especially those in a client-server environment (e.g., web databases). With this model, a search query returns top-k tuples according to not just exact matches of selection conditions, but a suitable ranking function. While much research has gone into the design of ranking functions and the efficient processing of top-k queries, this paper studies a novel problem on the privacy implications of database ranking. The motivation is a novel yet serious privacy leakage we found on real-world web databases which is caused by the ranking function design. Many such databases feature private attributes - e.g., a social network allows users to specify certain attributes as only visible to him/herself, but not to others. While these websites generally respect the privacy settings by not directly displaying private attribute values in search query answers, many of them nevertheless take into account such private attributes in the ranking function design. The conventional belief might be that tuple ranks alone are not enough to reveal the private attribute values. Our investigation, however, shows that this is not the case in reality. To address the problem, we introduce a taxonomy of the problem space with two dimensions, (1) the type of query interface and (2) the capability of adversaries. For each subspace, we develop a novel technique which either guarantees the successful inference of private attributes, or does so for a significant portion of real-world tuples. We demonstrate the effectiveness and efficiency of our techniques through theoretical analysis, extensive experiments over real-world datasets, as well as successful online attacks over websites with tens to hundreds of millions of users - e.g., Amazon Goodreads and Renren.com.
Research 30: Logic Programming, Web Data Management and Query Processing
Location: Kings 2
Chair: Yannis Papakonstantinou


Selective Provenance for Datalog Programs Using Top-k Queries
Daniel Deutch (Tel Aviv University), Amir Gilad (Tel Aviv University), Yuval Moskovitch (Tel Aviv University)
Highly expressive declarative languages, such as Datalog, are now commonly used to model the operational logic of data-intensive applications. The typical complexity of such Datalog programs, and the large volume of data that they process, call for result explanation. Results may be explained through the tracking and presentation of data provenance, and here we focus on a detailed form of provenance (how-provenance), defining it as the set of derivation trees of a given fact. While informative, the size of such full provenance information is typically too large and complex (even when compactly represented) to allow displaying it to the user. To this end, we propose a novel top-k query language for querying Datalog provenance, supporting selection criteria based on tree patterns and ranking based on the rules and database facts used in derivation. We propose an efficient algorithm based on (1) instrumenting the Datalog program so that, upon evaluation, it generates only relevant provenance, and (2) efficient top-k (relevant) provenance generation, combined with bottom-up Datalog evaluation. The algorithm computes in polynomial data complexity a compact representation of the top-k trees which may then be explicitly constructed in linear time with respect to their size. We further experimentally study its performance, showing its scalability even for complex Datalog programs where full provenance tracking is infeasible.


Asynchronous and Fault-Tolerant Recursive Datalog Evaluation in Shared-Nothing Engines
Jingjing Wang (University of Washington), Magdalena Balazinska (Univsersity of Washington), Daniel Halperin (University of Washington)
We develop a new approach for data analytics with iterations. Users express their analysis in Datalog with bag-monotonic aggregate operators, which enables the expression of computations from a broad variety of application domains. Queries are translated into query plans that can execute in shared-nothing engines, are incremental, and support a variety of iterative models (synchronous, asynchronous, different processing priorities) and failure-handling techniques. The plans require only small extensions to an existing shared-nothing engine, making the approach easily implementable. We implement the approach in the Myria big-data management system and use our implementation to empirically study the performance characteristics of different combinations of iterative models, failure handling methods, and applications. Our evaluation uses workloads from a variety of application domains. We find that no single method outperforms others but rather that application properties must drive the selection of the iterative query execution model.


Aggregate Estimations over Location Based Services
Weimo Liu (George Washington University), Md Farhadur Rahman (University of Texas at Arlington), Saravanan Thirumuruganathan (University of Texas at Arlingt), Nan Zhang (George Washington University), Gautam Das (University of Texas at Arlington)
Location based services (LBS) have become very popular in recent years. They range from map services (e.g., Google Maps) that store geographic locations of points of interests, to online social networks (e.g., WeChat, Sina Weibo, FourSquare) that leverage user geographic locations to enable various recommendation functions. The public query interfaces of these services may be abstractly modeled as a kNN interface over a database of two dimensional points on a plane: given an arbitrary query point, the system returns the k points in the database that are nearest to the query point. In this paper we conisder the problem of obtaining approximate estimates of SUM and COUNT aggregates by only querying such databases via their restrictive public interfaces. We distinguish between interfaces that return location information of the returned tuples (e.g., Google Maps), and interfaces that do not return location information (e.g., Sina Weibo). For both types of interfaces, we develop aggregate estimation algorithms that are based on novel techniques for precisely computing or approximately estimating the Voronoi cell of tuples. We discuss a comprehensive set of real-world experiments for testing our algorithms, including experiments on Google Maps, WeChat, and Sina Weibo.


PARADIS: An Efficient Parallel Algorithm for In-place Radix Sort
Minsik Cho (IBM T.J. Watson Research Center), Daniel Brand (IBM T.J. Watson Research Center), Rajesh Bordawekar (IBM T.J. Watson Research Center), Ulrich Finkler (IBM T.J. Watson Research Center), Vincent Kulandaisamy (IBM T.J. Watson Research Center), Ruchir Puri (IBM T.J. Watson Research Center)
In-place radix sort is a popular distribution-based sorting algorithm for short numeric or string keys. It has a linear run-time and constant memory complexity. However, efficient parallelization of in-place radix sort is very challenging for two reasons. First, the initial phase of permuting elements into buckets suffers read-write dependency inherent in its in-place nature. Secondly, load-balancing of the recursive application of the algorithm to the resulting buckets is difficult when the buckets are of very different sizes, which happens for skewed distributions of the input data. In this paper, we present PARADIS, which addresses both problems: a) “speculative permutation” solves the first problem by assigning multiple non-continuous array stripes to each processor. The resulting shared-nothing scheme achieves full parallelization. Since our speculative permutation is not complete, it is followed by a repair phase, which can again be done in parallel without any data sharing among the processors. b) “distribution-adaptive load-balancing” solves the second problem. We dynamically allocate processors in the context of radix sort, so as to minimize the overall completion time. Our experimental results show that PARADIS offers excellent performance/scalability on a wide range of input data sets.


Performance and Scalability of Indexed Subgraph Query Processing Methods
Foteini Katsarou (University of Glasgow), Nikos Ntarmos (University of Glasgow), Peter Triantafillou (University of Glasgow)
Graph data management systems have become very popular as graphs are the natural data model for many applications. One of the main problems addressed by these systems is subgraph query processing; i.e., given a query graph, return all graphs that contain the query. The naive method for processing such queries is to perform a subgraph isomorphism test against each graph in the dataset. This obviously does not scale, as subgraph isomorphism is NP-Complete. Thus, many indexing methods have been proposed to reduce the number of candidate graphs that have to underpass the subgraph isomorphism test. In this paper, we identify a set of key factors-parameters, that influence the performance of related methods: namely, the number of nodes per graph, the graph density, the number of distinct labels, the number of graphs in the dataset, and the query graph size. We then conduct comprehensive and systematic experiments that analyze the sensitivity of the various methods on the values of the key parameters. Our aims are twofold: first to derive conclusions about the algorithms' relative performance, and, second, to stress-test all algorithms, deriving insights as to their scalability, and highlight how both performance and scalability depend on the above factors. We choose six well-established indexing methods, namely Grapes, CT-Index, GraphGrepSX, gIndex, Tree+Delta, and gCode, as representative approaches of the overall design space, including the most recent and best performing methods. We report on their index construction time and index size, and on query processing performance in terms of time and false positive ratio. We employ both real and synthetic datasets. Specifically, four real datasets of different characteristics are used: AIDS, PDBS, PCM, and PPI. In addition, we generate a large number of synthetic graph datasets, empowering us to systematically study the algorithms' performance and scalability as they depend on the aforementioned key parameters.
Research 31: Structure and Dependency Discovery, and Spatial Databases
Location: Kings 3
Chair: Ziawasch Abedjan


Spatial Partitioning Techniques in Spatial Hadoop
Ahmed Eldawy (University of Minnesota), Louai Alarabi (University of Minnesota), Mohamed Mokbel (University of Minnesota)
SpatialHadoop is an extended MapReduce framework that provides orders of magnitude better performance, when dealing with spatial data, compared to plain-vanilla Hadoop. This performance boost is resulted mainly by the {\em global index} which spatially partitions the data across machines. In this paper, we describe seven alternative partitioning techniques and experimentally study their effect on the quality of the generated index and the performance of range and spatial join queries. We found that using a 1\% sample is enough to produce partitions of high quality. Also, we found that the total area of partitions is a reasonable measure for the quality of an index especially when running an expensive operation such as spatial join in MapReduce. We believe that this study will assist researchers and developers to choose the most appropriate spatial partitioning technique based on the properties of the dataset and the desired queries.


Divide & Conquer-based Inclusion Dependency Discovery
Thorsten Papenbrock (Hasso-Plattner-Institute), Sebastian Kruse (Hasso-Plattner-Institute), Jorge-Arnulfo Quiane-Ruiz (Qatar Research Institute), Felix Naumann (Hasso Plattner Institut Potsdam)
The discovery of all inclusion dependencies (INDs) in a dataset is an important part of any data profiling effort. Apart from the detection of foreign key relationships, INDs can help to perform data integration, query optimization, integrity checking, or schema (re-)design. However, the detection of INDs gets harder as datasets become larger in terms of contained tuples as well as attributes. To this end, we propose BINDER, an IND detection system that is capable of detecting both unary and n-ary INDs. It is based on a divide & conquer approach, which allows to handle very large datasets – an important property on the face of the ever increasing size of today’s data. In particular, we do not rely on existing database functionality nor assume that inspected datasets fit into main memory, in contrast to much related work. This renders BINDER an efficient and scalable competitor. An exhaustive experimental evaluation shows the high superiority of BINDER over state-of-the-art in both unary and n-ary IND discovery. Binder is up to 26x faster than SPIDER and more than 2496x faster than MIND.


Functional Dependency Discovery: An Experimental Evaluation of Seven Algorithms
Thorsten Papenbrock (Hasso-Plattner-Institute), Jens Ehrlich (Hasso-Plattner-Institute), jannik Marten (Hasso-Plattner-Institute), Tommy Neubert (Hasso-Plattner-Institute), Jan-Peer Rudolph (Hasso-Plattner-Institute), Martin Schönberg (Hasso-Plattner-Institute), Jakob Zwiener (Hasso-Plattner-Institute), Felix Naumann (Hasso-Plattner-Institute)
Functional dependencies are important metadata used for schema normalization, data cleansing and many other tasks. The efficient discovery of functional dependencies in tables is a well-known challenge in database research and has seen several approaches. Because no comprehensive comparison between these algorithms exist at the time, it is hard to choose the best algorithm for a given dataset. In this experimental paper, we describe, evaluate, and compare the seven most cited and most important algorithms, all solving this same problem. First, we classify the algorithms into three different categories, explaining their commonalities. We then describe all algorithms with their main ideas. The descriptions provide additional details where the original papers were ambiguous or incomplete. Our evaluation of careful re-implementations of all algorithms spans a broad test space including synthetic and real-world data. We show that all functional dependency algorithms optimize for certain data characteristics and provide hints on when to choose which algorithm. In summary, however, all current approaches scale surprisingly poorly, showing potential for future research.


Extracting Logical Hierarchical Structure of HTML Documents Based on Headings
Tomohiro Manabe (Kyoto University), Keishi Tajima (Kyoto University)
We propose a method for extracting logical hierarchical structure of marked-up electric documents, such as those in HTML format and those in various office document formats. Documents in these formats include nested mark-up structure, but it does not necessarily coincide with logical hierarchical structure of the documents. Because of their discrepancy, it is not easy to automatically convert these documents into well-structured data for machine processing. Human readers, however, easily understand logical structure of these documents. The key information used by human readers for understanding it is hierarchical headings in the documents. Our method extracts logical hierarchical structure of documents by simulating this behavior of human readers, i.e., by identifying hierarchical headings in them. Identification of headings is also done by simulating the process of heading recognition by human readers. Because a heading concisely describes the topic of its associated logical block, a user reading a block first reads its heading and determine whether the block is relevant to her needs. If it is not relevant, the user may skip the block, and jump to the next heading of the same level. If the block is relevant, and it includes lower-level headings, the user recursively repeats the same process. In order to help this process, headings are given prominent visual styles, headings of the same level share the same visual style for helping skips, and headings of higher levels are given more prominent visual styles. Our method extracts hierarchical headings based on these properties of headings. Our experiment shows that our method achieves a far better recall ratio while maintaining satisfactory precision compared with the existing document segmentation methods. In addition, unlike the existing methods, our method identifies not only hierarchical blocks but also their associated headings.


Bonding Vertex Sets Over Distributed Graph: A Betweenness Aware Approach
Xiaofei Zhang (HKSUT), Hong Cheng (The Chinese University of Hong Kong), Lei Chen (Hong Kong University of Science and Technology)
Given two sets of vertices in a graph, it is often a great interest to find out how these vertices are connected, especially to identify the vertices of high prominence defined on the topological structure. In this work, we formally define a Vertex Set Bonding query (shorted as VSB), which returns a minimum set of vertices with the maximum importance w.r.t. total betweenness and shortest path reachability in connecting two sets of input vertices. We find that such kind of query is representative and could be widely applied in many real world scenarios, e.g., logistic planning, social community bonding and etc. As many of such applications require rapid updates on the graph data structure, the grand challenge of VSB query evaluation, which is NP-hard, lies in smoothly coping with the graph evolvement and returning the near optimal result in almost real time. We propose a generic solution framework for the VSB query evaluation. With the development of two novel techniques, guided graph exploration and betweenness ranking on exploration, we are able to efficiently evaluate queries for error bounded results with bounded space cost. We demonstrate the effectiveness of our solution with extensive experiments over both real and synthetic large graphs on the Google's Cloud platform. Comparing to the exploration only baseline method, our method achieves several times of speedup.
Research 32: Time Series and Streams
Location: Queens 4
Chair: Themis Palpanis


CANDS: Continuous Optimal Navigation via Distributed Stream Processing
Dingyu Yang (Shanghai Jiao Tong University), Dongxiang Zhang (National University of Singapore), Kian-Lee Tan (National University of Singapore), Jian Cao (Shanghai Jiao Tong University), Frédéric Le Mouël (University of Lyon)
Shortest path query over a dynamic road network is a prominent problem for the optimization of real-time traffic systems. Existing solutions rely either on a centralized index system with tremendous pre-computation overhead, or on a distributed graph processing system such as Pregel that requires much synchronization effort. However, the performance of these systems degenerates with frequent route path updates caused by continuous traffic condition change. In this paper, we build CANDS, a distributed stream processing platform for continuous optimal shortest path queries. It provides an asynchronous solution to answering a large quantity of shortest path queries. It is able to efficiently detect affected paths and adjust their paths in the face of traffic updates. Moreover, the affected paths can be quickly updated to the optimal solutions throughout the whole navigation process. Experimental results demonstrate that the performance for answering shortest path queries by CANDS is two orders of magnitude better than that of GPS, an open-source implementation of Pregel. In addition, CANDS provides fast response to traffic updates to guarantee the optimality of answering shortest path queries.


General Incremental Sliding-Window Aggregation
Kanat Tangwongsan (Mahidol University International College), Martin Hirzel (IBM T. J. Watson Research Center), Scott Schneider (IBM T. J. Watson Research Center), Kun-Lung Wu (IBM T.J. Watson Research Center)
Stream processing is gaining importance as more data becomes available in the form of continuous streams and companies compete to promptly extract insights from them. In such applications, sliding-window aggregation is a central operator, and incremental aggregation helps avoid the performance penalty of re-aggregating from scratch for each window change. This paper presents Reactive Aggregator (RA), a new framework for incremental sliding-window aggregation. RA is general in that it does not require aggregation functions to be invertible or commutative, and it does not require windows to be FIFO. We implemented RA as a drop-in replacement for the Aggregate operator of a commercial streaming engine. Given m updates on a window of size n, RA has an algorithmic complexity of O(m+m\log(n/m)), rivaling the best prior algorithms for any m. Furthermore, RA's implementation minimizes overheads from allocation and pointer traversals by using a single flat array.


YADING: Fast Clustering of Large-Scale Time Series Data
Rui Ding (Microsoft Research), Qiang Wang (Microsoft Research), Yingnong Dang (Microsoft Research), Qiang Fu (Microsoft Research), Haidong Zhang (Microsoft Research), Dongmei Zhang (Microsoft Research)
Fast and scalable techniques are becoming increasingly important in the era of big data, because they are the enabling techniques to create real-time and interactive experiences in data analysis. Time series are widely available in diverse application areas. Due to the large number of time series instances (e.g., millions) and the high dimensionality of each time series instance (e.g., thousands), it is challenging to conduct clustering on large-scale time series, and it is even more challenging to do so in real-time to support interactive exploration. In this paper, we propose a novel end-to-end time series clustering algorithm, YADING, which automatically clusters large-scale time series with fast performance and quality results. Specifically, YADING consists of three steps: sampling the input dataset, conducting clustering on the sampled dataset, and assigning the rest of the input data to the clusters generated on the sampled dataset. In particular, we provide theoretical proof on the lower and upper bounds to determine the sample size, which not only guarantees YADING’s high performance, but also ensures the distribution consistency between the input dataset and the sampled dataset. We also select L_1 norm as similarity measure and the multi-density approach as the clustering method. With theoretical bound, this selection ensures YADING’s robustness to time series variations due to phase perturbation and random noise. Evaluation results have demonstrated that on typical-scale (100,000 time series each with 1,000 dimensions) datasets, YADING is about 40 times faster than the state-of-the-art, sampling-based clustering algorithm DENCLUE 2.0, and about 1,000 times faster than DBSCAN and CLARANS. YADING has also been used by product teams at Microsoft to analyze service performance. Two of such use cases are shared in this paper.


Monitoring Distributed Streams using Convex Decompositions
Arnon Lazerson (Technion), Daniel Keren (Haifa University), Izchak Sharfman (Technion), Minos Garofalakis (Technical University of Crete), Vasilis Samoladas (Technical University of Crete), Assaf Schuster (Technion)
Emerging large-scale monitoring applications rely on continuous tracking of complex data-analysis queries over collections of massive, physically-distributed data streams. Thus, in addition to the space- and time-efficiency requirements of conventional stream processing (at each remote monitor site), effective solutions also need to guarantee communication efficiency (over the underlying communication network). The complexity of the monitored query adds to the difficulty of the problem --- this is especially true for non-linear queries (e.g., joins), where no obvious solutions exist for distributing the monitored condition across sites. The recently proposed geometric method, based on the notion of covering spheres, offers a generic methodology for splitting an arbitrary (non-linear) global condition into a collection of local site constraints, and has been applied to massive distributed stream-monitoring tasks, achieving state-of-the-art performance. In this paper, we present a far more general geometric approach, based on the convex decomposition of an appropriate subset of the domain of the monitoring query, and formally prove that it is always guaranteed to perform at least as good as the covering spheres method. We analyze our approach and demonstrate its effectiveness for the important case of sketch-based approximate tracking for norm, range-aggregate, and join-aggregate queries, which have numerous applications in streaming data analysis. Experimental results on real-life data streams verify the superiority of our approach in practical settings, showing that it substantially outperforms the covering spheres method.
Research 33: Transaction Processing
Location: Queens 5
Chair: Heiko Schuldt


Staring into the Abyss: An Evaluation of Concurrency Control with One Thousand Cores
Xiangyao Yu (MIT), George Bezerra (MIT), Andy Pavlo (Carnegie Mellon University), Srinivas Devadas (MIT), Michael Stonebraker (MIT)
Computer architectures are moving towards an era dominated by many-core machines with dozens or even hundreds of cores on a single chip. This unprecedented level of on-chip parallelism introduces a new dimension to scalability that current database management systems (DBMSs) were not designed for. In particular, as the number of cores increases the problem of concurrency control becomes extremely challenging. With hundreds of threads running in parallel, the complexity of coordinating competing accesses to data will likely diminish the gains from increased core counts. To better understand just how unprepared current DBMSs are for future CPU architectures, we performed an evaluation of concurrency control for on-line transaction processing (OLTP) workloads on many-core chips. We implemented seven concurrency control algorithms on a main-memory DBMS and using computer simulations scale our system to 1024 cores. Our analysis shows that all algorithms fail to scale to this magnitude but for different reasons. In each case, we identify fundamental bottlenecks that are independent of the particular database implementation and argue that even state-of-the-art DBMSs suffer from these limitations. We conclude that rather than pursuing incremental solutions, many-core chips may require a completely redesigned DBMS architecture that is built from ground up and is tightly coupled with the hardware.


E-Store: Fine-Grained Elastic Partitioning for Distributed Transaction Processing Systems
Rebecca Taft (MIT), Essam Mansour (QCRI), Marco Serafini (QCRI), Jennie Duggan (MIT), Aaron Elmore (MIT), Ashraf Aboulnaga (QCRI), Andy Pavlo (Carnegie Mellon University), Michael Stonebraker (MIT)
On-line transaction processing (OLTP) database management systems (DBMSs) often serve time-varying workloads due to daily, weekly or seasonal fluctuations in demand, or because of rapid growth in demand due to a company's business success. In addition, many OLTP workloads are heavily skewed to "hot" tuples or ranges of tuples. For example, the majority of NYSE volume involves only 40 stocks. To deal with such fluctuations, an OLTP DBMS needs to be elastic; that is, it must be able to expand and contract resources in response to load fluctuations and dynamically balance load as hot tuples vary over time. This paper presents E-Store, an elastic partitioning framework for distributed OLTP DBMSs. It automatically scales resources in response to demand spikes, periodic events, and gradual changes in an application's workload. E-Store addresses localized bottlenecks through a two-tier data placement strategy: cold data is distributed in large chunks, while smaller ranges of hot tuples are assigned explicitly to individual nodes. This is in contrast to traditional single-tier hash and range partitioning strategies. Our experimental evaluation of E-Store shows the viability of our approach and its efficacy under variations in load across a cluster of machines. Compared to single-tier approaches, E-Store improves throughput by up to 130% while reducing latency by 80%.


ADDICT: Advanced Instruction Chasing for Transactions
Pinar Tozun (EPFL), Islam Atta (University of Toronto), Anastasia Ailamaki (EPFL), Andrea Moshovos (University of Toronto)
Recent studies highlight that traditional transaction processing systems utilize the micro-architectural features of modern processors very poorly. L1 instruction cache and long-latency data misses dominate execution time. As a result, more than half of the execution cycles are wasted on memory stalls. Previous works on reducing stall time aim at improving locality through either hardware or software techniques. However, exploiting hardware resources based on the hints given by the software-side has not been widely studied for data management systems. In this paper, we observe that, independently of their high-level functionality, transactions running in parallel on a multicore system execute actions chosen from a limited subset of predefined database operations. Therefore, we initially perform a memory characterization study of modern transaction processing systems using standardized benchmarks. The analysis demonstrates that same-type transactions exhibit at most 6% overlap in their data footprints whereas there is up to 98% overlap in instructions. Based on the findings, we design ADDICT, a transaction scheduling mechanism that aims at maximizing the instruction cache locality. ADDICT determines the most frequent actions of database operations, whose instruction footprint can fit in an L1 instruction cache, and assigns a core to execute each of these actions. Then, it schedules each action on its corresponding core. Our prototype implementation of ADDICT reduces L1 instruction misses by 85% and the long latency data misses by 20%. As a result, ADDICT leads up to a 50% reduction in the total execution time for the evaluated workloads.


Rethinking serializable multiversion concurrency control
Jose Faleiro (Yale University), Daniel Abadi (Yale University)
Multi-versioned database systems have the potential to significantly increase the amount of concurrency in transaction processing because they can avoid read-write conflicts. Unfortunately, the increase in concurrency usually comes at the cost of transaction serializability. If a database user requests full serializability, modern multi-versioned systems significantly constrain read-write concurrency among conflicting transactions and employ expensive synchronization patterns in their design. In main-memory multi-core settings, these additional constraints are so burdensome that multi-versioned systems are often significantly outperformed by single-version systems. We propose Bohm, a new concurrency control protocol for main-memory multi-versioned database systems. Bohm guarantees serializable execution while ensuring that reads never block writes. In addition, Bohm does not require reads to perform any book-keeping whatsoever, thereby avoiding the overhead of tracking reads via contended writes to shared memory. This leads to excellent scalability and performance in multi-core settings. Bohm has all the above characteristics without performing validation based concurrency control. Instead, it is pessimistic, and is therefore not prone to excessive aborts in the presence of contention. An experimental evaluation shows that Bohm performs well in both high contention and low contention settings, and is able to dramatically outperform state-of-the-art multi-versioned systems despite maintaining the full set of serializability guarantees.
Tutorial 8: Real Time Analytics: Algorithms and Systems (2/2)
Location: Queens 6


Real Time Analytics: Algorithms and Systems (2/2)
Arun Kejariwal, Sanjeev Kulkarni, Karthik Ramasamy
Velocity is one of the 4 Vs commonly used to characterize Big Data. In this regard, Forrester remarked the following in Q3 2014: “The high velocity, white-water flow of data from innumerable real-time data sources such as market data, Internet of Things, mobile, sensors, click-stream, and even transactions remain largely unnavigated by most firms. The opportunity to leverage streaming analytics has never been greater.” Example use cases of streaming analytics include, but not limited to: (a) visualization of business metrics in real-time (b) facilitating highly personalized experiences (c) expediting response during emergencies. Streaming analytics is extensively used in a wide variety of domains such as healthcare, e-commerce, financial services, telecommunications, energy and utilities, manufacturing, government and transportation. In this tutorial, we shall present an in-depth overview of streaming analytics – applications, algorithms and platforms – landscape. We shall walk through how the field has evolved over the last decade and then discuss the current challenges – the impact of the other three Vs, viz., Volume, Variety and Veracity, on Big Data streaming analytics. The tutorial is intended for both researchers and practitioners in the industry. We shall also present state-of-the-affairs of streaming analytics at Twitter.
Demo 3: Systems, User Interfaces, and Visualization
Location: Kona 4
Chair: Tianli Mo


FP-Hadoop: Efficient Execution of Parallel Jobs Over Skewed Data
Miguel Liroz-Gistau (INRIA), Reza Akbarinia (INRIA), Patrick Valduriez (INRIA)
Big data parallel frameworks, such as MapReduce or Spark have been praised for their high scalability and performance, but show poor performance in the case of data skew. There are important cases where a high percentage of processing in the reduce side ends up being done by only one node. In this demonstration, we illustrate the use of FP-Hadoop, a system that efficiently deals with data skew in MapReduce jobs. In FP-Hadoop, there is a new phase, called inter- mediate reduce (IR), in which blocks of intermediate values, constructed dynamically, are processed by intermediate reduce workers in parallel, by using a scheduling strategy. Within the IR phase, even if all intermediate values belong to only one key, the main part of the reducing work can be done in parallel using the computing resources of all available workers. We implemented a prototype of FP-Hadoop, and conducted extensive experiments over synthetic and real datasets. We achieve excellent performance gains compared to native Hadoop, e.g. more than 10 times in reduce time and 5 times in total execution time. During our demonstration, we give the users the possibility to execute and compare job executions in FP-Hadoop and Hadoop. They can retrieve general information about the job and the tasks and a summary of the phases. They can also visually compare different configurations to explore the difference between the approaches.


SDB: A Secure Query Processing System with Data Interoperability
Zhian He (Hong Kong Polytechnic University), WaiKit Wong (Hang Seng Management College), Ben Kao (University of Hong Kong), David W. Cheung (University of Hong Kong), Rongbin Li (University of Hong Kong), Siu Ming Yiu (University of Hong Kong), Eric Lo (Polytecnic University of Hong Kong)
We address security issues in a cloud database system which em- ploys the DBaaS model — a data owner (DO) exports data to a cloud database service provider (SP). To provide data security, sensitive data is encrypted by the DO before it is uploaded to the SP. Compared to existing secure query processing systems like CryptDB [7] and MONOMI [8], in which data operations (e.g., comparison or addition) are supported by specialized encryption schemes, our demo system, SDB, is implemented based on a set of data- interoperable secure operators, i.e., the output of an operator can be used as input of another operator. As a result, SDB can sup- port a wide range of complex queries (e.g., all TPC-H queries) efficiently. In this demonstration, we show how our SDB prototype supports secure query processing on complex workload like TPC-H. We also demonstrate how our system protects sensitive in- formation from malicious attackers.


A Demonstration of HadoopViz: An Extensible MapReduce-based System for Visualizing Big Spatial Data
Ahmed Eldawy (University of Minnesota), Mohamed Mokbel (University of Minnesota), Christopher Jonathan (University of Minnesota)
This demonstration presents HadoopViz; an extensible MapReduce-based system for visualizing Big Spatial Data. HadoopViz has two main unique features that distinguish it from other techniques. (1) It provides an extensible interface that allows users to visualize various types of data by defining five abstract functions, without delving into the details of the MapReduce algorithms. We show how it is used to create four types of visualizations, namely, scatter plot, road network, frequency heat map, and temperature heat map. (2) HadoopViz is capable of generating big images with giga-pixel resolution by employing a three-phase approach of partitioning, rasterize, and merging. HadoopViz generates single and multi-level images, where the latter allows users to zoom in/out to get more/less details. Both types of images are generated with a very high resolution using the extensible and scalable framework of HadoopViz.


A Demonstration of the BigDAWG Polystore System
Aaron Elmore (MIT), Jennie Duggan (Northwestern), Michael Stonebraker (MIT), Manasi Vartak (MIT), Sam Madden (MIT), Vijay Gadepally (MIT), Jeremy Kepner (MIT), Timothy Mattson (Intel), Jeff Parhurst (Intel), Stavros Papadopoulos (MIT), Nesime Tatbul (Intel Labs and MIT), Magdalena Balazinska (Univsersity of Washington), Bill Howe (University of Washington), Jeffrey Heer (University of Washington), David Maier (Portland State University), Tim Kraska (Brown), Ugur Cetintemel (Brown University), Stan Zdonik (Brown University)
This paper presents BigDAWG, a reference implementation of a new architecture for “Big Data” applications. Such applications not only call for large-scale analytics, but also for real-time streaming support, smaller analytics at interactive speeds, data visualization, and cross-storage-system queries. Guided by the principle that “one size does not fit all”, we build on top of a variety of storage engines, each designed for a specialized use case. To illustrate the promise of this approach, we demonstrate its effective- ness on a hospital application using data from an intensive care unit (ICU). This complex application serves the needs of doctors and re- searchers and provides real-time support for streams of patient data. It showcases novel approaches for querying across multiple storage engines, data visualization, and scalable real-time analytics.


RINSE: Interactive Data Series Exploration with ADS+
Kostas Zoumpatianos (University of Trento), Stratos Idreos (Harvard), Themis Palpanas (Paris Descartes University)
Numerous applications continuously produce big amounts of data series, and in several time critical scenarios analysts need to be able to query these data as soon as they be- come available. An adaptive index data structure, ADS+, which is specifically tailored to solve the problem of index- ing and querying very large data series collections has been recently proposed as a solution to this problem. The main idea is that instead of building the complete index over the complete data set up-front and querying only later, we inter- actively and adaptively build parts of the index, only for the parts of the data on which the users pose queries. The net effect is that instead of waiting for extended periods of time for the index creation, users can immediately start exploring the data series. In this work, we present a demonstration of ADS+; we introduce RINSE, a system that allows users to experience the benefits of the ADS+ adaptive index through an intuitive web interface. Users can explore large datasets and find patterns of interest, using nearest neighbor search. They can draw queries (data series) using a mouse, or touch screen, or they can select from a predefined list of data se- ries. RINSE can scale to large data sizes, while drastically reducing the data to query delay: by the time state-of-the- art indexing techniques finish indexing 1 billion data series (and before answering even a single query), adaptive data series indexing can already answer 3 ∗ 105 queries.


Smart Drill-Down: A New Data Exploration Operator
Manas Joglekar (Stanford University), Hector Garcia-Molina (Stanford University), Aditya Parameswaran (University of Illinois at Urbana Champaign)
We present a data exploration system equipped with smart drill- down, a novel operator for interactively exploring a relational table to discover and summarize “interesting” groups of tuples. Each such group of tuples is represented by a rule. For instance, the rule (a, b, ⋆, 1000) tells us that there are a thousand tuples with value a in the first column and b in the second column (and any value in the third column). Smart drill-down presents an analyst with a list of rules that together describe interesting aspects of the table. The analyst can tailor the definition of interesting, and can interactively apply smart drill-down on an existing rule to explore that part of the table. In the demonstration, conference attendees will be able to use the data exploration system equipped with smart drill-down, and will be able to contrast smart drill-down to traditional drill-down, for various interestingness measures, and resource constraints.


VIIQ: auto-suggestion enabled visual interface for interactive graph query formulation
Nandish Jayaram (University of Texas at Arlingt), Sidharth Goyal (University of Texas at Arlington), Chengkai Li (University of Texas at Arlington)
We present VIIQ (pronounced as wick), an interactive and iterative visual query formulation interface that helps users construct query graphs specifying their exact query intent. Heterogeneous graphs are increasingly used to represent complex relationships in schema- less data, which are usually queried using query graphs. Existing graph query systems offer little help to users in easily choosing the exact labels of the edges and vertices in the query graph. VIIQ helps users easily specify their exact query intent by providing a visual interface that lets them graphically add various query graph com- ponents, backed by an edge suggestion mechanism that suggests edges relevant to the user’s query intent. In this demo we present: 1) a detailed description of the various features and user-friendly graphical interface of VIIQ, 2) a brief description of the edge sug- gestion algorithm, and 3) a demonstration scenario that we intend to show the audience.


VINERy: A Visual IDE for Information Extraction
Yunyao Li (IBM Research-Almaden), Elmer Kim (Treasuer Data (Inc.), Marc Touchette (IBM Silicon Valley Lab), Ramiya Venkatachalam (IBM Silicon Valley Lab), Hao Wang (IBM Silicon Valley Lab)
Information Extraction (IE) is the key technology enabling analytics over unstructured and semi-structured data. Not surprisingly, it is becoming a critical building block for a wide range of emerging applications. To satisfy the rising demands for information extraction in real-world applications, it is crucial to lower the barrier to entry for IE development and enable users with general computer science background to develop higher quality extractors. In this demonstration1, we present VINERY, an intuitive yet expressive visual IDE for information extraction. We show how it supports the full cycle of IE development without requiring a single line of code and enables a wide range of users to develop high quality IE extractors with minimal efforts. The extractors visually built in VINERY are automatically translated into semantically equivalent extractors in a state-of-the-art declarative language for IE. We also demonstrate how the auto-generated extractors can then be imported into a conventional Eclipse-based IDE for further enhancement. The results of our user studies indicate that VINERY is a significant step forward in facilitating extractor development for both expert and novice IE developers.


GIS navigation boosted by column stores
Foteini Alvanaki (CWI), Romulo Goncalves (Netherlands eScience Center), Milena Ivanova (NuoDB), Martin Kersten (CWI), Kostis Kyzirakos (CWI)
Earth observation sciences, astronomy, and seismology have large data sets which have inherently rich spatial and geospatial information. In combination with large collections of semantically rich objects which have a large number of thematic properties, they form a new source of knowledge for urban planning, smart cities and natural resource management. Modeling and storing these properties indicating the relationships between them is best handled in a relational database. Furthermore, the scalability requirements posed by the latest 26-attribute light detection and ranging (LI- DAR) data sets are a challenge for file-based solutions. In this demo we show how to query a 640 billion point data set using a column store enriched with GIS functionality. Through a lightweight and cache conscious secondary index called Imprints, spatial queries performance on a flat table storage is comparable to traditional file-based solutions. All the results are visualised in real time using QGIS.


AIDE: An Automatic User Navigation System for Interactive Data Exploration
Yanlei Diao (University of Massachusetts Amherst), Kyriaki Dimitriadou (Brandeis university), Zhan Li (Brandeis University), Wenzhao Liu (University of Massachusetts Amherst), Olga Papaemmanouil (Brandeis University), Kemi Peng (Brandeis University), Liping Peng (University of Massachusetts Amherst)
Data analysts often engage in data exploration tasks to discover interesting data patterns, without knowing exactly what they are looking for. Such exploration tasks can be very labor-intensive because they often require the user to review many results of ad-hoc queries and adjust the predicates of subsequent queries to balance the trade-off between collecting all interesting information and reducing the size of returned data. In this demonstration we introduce AIDE , a system that automates these exploration tasks. AIDE steers the user towards interesting data areas based on her relevance feedback on database samples, aiming to achieve the goal of identifying all database objects that match the user interest with high efficiency. In our demonstration, conference attendees will see AIDE in action for a variety of exploration tasks on real-world datasets.


A Demonstration of AQWA: Adaptive Query-Workload-Aware Partitioning of Big Spatial Data
Ahmed Aly (Purdue University), Ahmed Abdelhamid (Purdue University), Ahmed Mahmood,Purdue University), Walid Aref (Purdue University), Mohamed Hassan (Purdue University), Hazem Elmeleegy (Turn Inc), Mourad Ouzzani (Qatar Computing Research Institute)
The ubiquity of location-aware devices, e.g., smartphones and GPS devices, has led to a plethora of location-based services in which huge amounts of geotagged information need to be efficiently pro- cessed by large-scale computing clusters. This demo presents AQWA, an adaptive and query-workload-aware data partitioning mechanism for processing large-scale spatial data. Unlike existing cluster-based systems, e.g., SpatialHadoop, that apply static parti- tioning of spatial data, AQWA has the ability to react to changes in the query-workload and data distribution. A key feature of AQWA is that it does not assume prior knowledge of the query-workload or data distribution. Instead, AQWA reacts to changes in both the data and the query-workload by incrementally updating the partitioning of the data. We demonstrate two prototypes of AQWA deployed over Hadoop and Spark. In both prototypes, we process spatial range and k-nearest-neighbor (kNN, for short) queries over large- scale spatial datasets, and we exploit the performance of AQWA under different query-workloads.


DATASPREAD: Unifying Databases and Spreadsheets
Mangesh Bendre (University of Illinois at Urbana-Champaign), Bofan Sun (University of Illinois at Urbana-Champaign), Ding Zhang (University of Illinois at Urbana-Champaign), Xinyan Zhou (University of Illinois at Urbana-Champaign), Kevin Chang (University of Illinois at Urbana-Champaign), Aditya Parameswaran (University of Illinois at Urbana-Champaign)
Spreadsheet software is often the tool of choice for ad-hoc tabular data management, processing, and visualization, especially on tiny data sets. On the other hand, relational database systems offer significant power, expressivity, and efficiency over spreadsheet software for data management, while lacking in the ease of use and ad-hoc analysis capabilities. We demonstrate DATASPREAD, a data exploration tool that holistically unifies databases and spread- sheets. It continues to offer a Microsoft Excel-based spreadsheet front-end, while in parallel managing all the data in a back-end database, specifically, PostgreSQL. DATASPREAD retains all the advantages of spreadsheets, including ease of use, ad-hoc analysis and visualization capabilities, and a schema-free nature, while also adding the advantages of traditional relational databases, such as scalability and the ability to use arbitrary SQL to import, filter, or join external or internal tables and have the results appear in the spreadsheet. DATASPREAD needs to reason about and reconcile differences in the notions of schema, addressing of cells and tuples, and the current “pane” (which exists in spreadsheets but not in traditional databases), and support data modifications at both the front-end and the back-end. Our demonstration will center on our first and early prototype of the DATASPREAD, and will give the attendees a sense for the enormous data exploration capabilities offered by unifying spreadsheets and databases.

CODD: A Dataless Approach to Big Data Testing
Ashoke S (Indian Institute of Science), Jayant Haritsa (IISc)


Vizdom: Interactive Analytics through Pen and Touch
Andrew Crotty (Brown University), Alex Galakatos (Brown University), Emanuel Zgraggen (Brown University), Carsten Binnig (Brown University), Tim Kraska (Brown University)
Machine learning (ML) and advanced statistics are impor- tant tools for drawing insights from large datasets. How- ever, these techniques often require human intervention to steer computation towards meaningful results. In this demo, we present Vizdom, a new system for interactive analytics through pen and touch. Vizdom’s frontend allows users to visually compose complex workflows of ML and statis- tics operators on an interactive whiteboard, and the back- end leverages recent advances in workflow compilation tech- niques to run these computations at interactive speeds. Ad- ditionally, we are exploring approximation techniques for quickly visualizing partial results that incrementally refine over time. This demo will show Vizdom’s capabilities by allowing users to interactively build complex analytics work- flows using real-world datasets.


DBSeer: Pain-free Database Administration through Workload Intelligence
Dong Young Yoon (University of Michigan Ann Arbor), Barzan Mozafari (University of Michigan Ann Arbor),Douglas Brown (Teradata Inc.)
The pressing need for achieving and maintaining high performance in database systems has made database administration one of the most stressful jobs in information technology. On the other hand, the increasing complexity of database systems has made qualified database administrators (DBAs) a scarce resource. DBAs are now responsible for an array of demanding tasks; they need to (i) provi- sion and tune their database according to their application require- ments, (ii) constantly monitor their database for any performance failures or slowdowns, (iii) diagnose the root cause of the perfor- mance problem in an accurate and timely fashion, and (iv) take prompt actions that can restore acceptable database performance. However, much of the research in the past years has focused on improving the raw performance of the database systems, rather than improving their manageability. Besides sophisticated consoles for monitoring performance and a few auto-tuning wizards, DBAs are not provided with any help other than their own many years of experience. Typically, their only resort is trial-and-error, which is a tedious, ad-hoc and often sub-optimal solution. In this demonstration, we present DBSeer, a workload intelligence framework that exploits advanced machine learning and causal- ity techniques to aid DBAs in their various responsibilities. DBSeer analyzes large volumes of statistics and telemetry data collected from various log files to provide the DBA with a suite of rich functionalities including performance prediction, performance diagno- sis, bottleneck explanation, workload insight, optimal admission control, and what-if analysis. In this demo, we showcase various features of DBSeer by predicting and analyzing the performance of a live database system. Will also reproduce a number of realistic performance problems in the system, and allow the audience to use DBSeer to quickly diagnose and resolve their root cause.

Sharing and Reproducing Database Applications
Quan Pham (University of Chicago), Severin Thaler (University of Chicago), Tanu Malik (University of Chicago), Ian Foster (University of Chicago), Boris Glavic (IIT)
Thursday Sep 3rd 17:15-19:00
Reception and Poster Session 2
Location: Kohala Ballroom
Chair: Tianli Mo
TOP: A Framework for Enabling Algorithmic Optimizations for Distance-Related Problems
Yufei Ding - Xipeng Shen - Madanlal Musuvathi - Todd Mytkowicz
SCAN++: Efficient Algorithm for Finding Clusters, Hubs and Outliers on Large-scale Graphs
Hiroaki Shiokawa - Yasuhiro Fujiwara - Makoto Onizuka
GraphTwist: Fast Iterative Graph Computation with Two-tier Optimizations
Yang Zhou - Ling Liu - Kisung Lee - Qi Zhang
A Scalable Distributed Graph Partitioner
Daniel Margo - Margo Seltzer
Keys for Graphs
Wenfei Fan - Zhe Fan - Chao Tian - Xin Luna Dong
Scaling Up Concurrent Main-Memory Column-Store Scans: Towards Adaptive NUMA-aware Data and Task Placement
Iraklis Psaroudakis - Tobias Scheuer - Norman May - Abdelkader Sellami - Anastassia Ailamaki
In-Memory Performance for Big Data
Goetz Graefe - Haris Volos - Hideaki Kimura - Harumi Kuno - Joseph Tucek - Mark Lillibridge - Alistair Veitch
Profiling R on a Contemporary Processor
Shriram Sridharan - Jignesh Patel
Deployment of Query Plans on Multicores
Jana Giceva - Gustavo Alonso - Timothy Roscoe - Tim Harris
Faster Set Intersection with SIMD instructions by Reducing Branch Mispredictions
Hiroshi Inoue - Moriyoshi Ohara - Kenjiro Taura
Resource Bricolage for Parallel Database Systems
Jiexing Li - Jeffrey Naughton - Rimma Nehme
Multi-Objective Parametric Query Optimization
Immanuel Trummer - Christoph Koch
Querying with Access Patterns and Integrity Constraints
Michael Benedikt - Julien Leblay - Efi Tsamoura
Uncertainty Aware Query Execution Time Prediction
Wentao Wu - Xi Wu - Hakan Hacigumus - Jeffrey Naughton
Join Size Estimation Subject to Filter Conditions
David Vengerov - Andre Menck - Sunil Chakkappen - Mohamed Zait
Scaling Up Crowd-Sourcing to Very Large Datasets: A Case for Active Learning
Barzan Mozafari - Purna Sarkar - Michael Franklin - Michael Jordan - Sam Madden
Hear the Whole Story: Towards the Diversity of Opinion in Crowdsourcing Markets
Ting Wu - Lei Chen - Pan Hui - CHEN ZHANG - Weikai Li
Where To: Crowd-Aided Path Selection
Chen Zhang - Yongxin Tong - Lei Chen
Reliable Diversity-Based Spatial Crowdsourcing by Moving Workers
Peng Cheng - Xiang Lian - Zhao Chen - Rui Fu - Lei Chen - Jinsong Han - Jizhong Zhao
Learning User Preferences By Adaptive Pairwise Comparison
Li Qian - Jinyang Gao - H V Jagadish
Pregelix: Big(ger) Graph Analytics on A Dataflow Engine
Yingyi Bu - Vinayak Borkar - Jianfeng Jia - Michael Carey - Tyson Condie
Large-Scale Distributed Graph Computing Systems: An Experimental Evaluation
Yi Lu - James Cheng - Da Yan - Huanhuan Wu
Fast Failure Recovery in Distributed Graph Processing Systems
Yanyan Shen - Gang Chen - H V Jagadish - Wei Lu - Beng Chin Ooi - Bogdan Tudor
Giraph Unchained: Barrierless Asynchronous Parallel Execution in Pregel-like Graph Processing Systems
Minyang Han - Khuzaima Daudjee
GraphMat: High performance graph analytics made productive
Narayanan Sundaram - Nadathur Satish - Mostofa Ali Patwary - Subramanya Dulloor - Michael Anderson - Satya Gautam Vadlamudi - Dipankar Das - Pradeep Dubey
In-Cache Query Co-Processing on Coupled CPU-GPU Architectures
Jiong He - Shuhao Zhang - Bingsheng He
NVRAM-aware Logging in Transaction Systems
Jian Huang - Karsten Schwan - Moinuddin Qureshi
Improving Main Memory Hash Joins on Intel Xeon Phi Processors: An Experimental Approach
Saurabh Jha - Bingsheng He - Mian Lu - Xuntao Cheng - Phung Huynh Huynh
REWIND: Recovery Write-Ahead System for In-Memory Non-Volatile Data-Structures
Andreas Chatzistergiou - Marcelo Cintra - Stratis Viglas
Persistent B+-Trees in Non-Volatile Main Memory
Shimin Chen - Qin Jin
Robust Local Community Detection: On Free Rider Effect and Its Elimination
Yubao Wu - Ruoming Jin - Jing Li - Xiang Zhang
Viral Marketing Meets Social Advertising: Ad Allocation with Minimum Regret
Cigdem Aslay - Wei Lu - Francesco Bonchi - Amit Goyal - Laks Lakshmanan
Community Detection in Social Networks: An In-depth Benchmarking Study with a Procedure-Oriented Framework
Meng Wang - Chaokun Wang - Jeffrey Xu Yu - Jun Zhang
Leveraging History for Faster Sampling of Online Social Networks
Zhuojie Zhou - Nan Zhang - Gautam Das
Real-time Targeted Influence Maximization for Online Advertisements
Yuchen Li - Dongxiang Zhang - Kian-Lee Tan
Top-k Nearest Neighbor Search In Uncertain Data Series
Michele Dallachiesa - Themis Palpanas - Ihab Ilyas
Scaling Manifold Ranking Based Image Retrieval
Yasuhiro Fujiwara - Go Irie - Shari Kuroyama - Makoto Onizuka
Optimal Enumeration: Efficient Top-k Tree Matching
Lijun Chang - Xuemin Lin - Wenjie Zhang - Jeffrey Xu Yu - Ying Zhang - Lu Qin
Generating Top-k Packages via Preference Elicitation
Min Xie - Laks V. S. Lakshmanan - Peter Wood
Rank aggregation with ties: Experiments and Analysis
Bryan Brancotte - Bo Yang - Guillaume Blin - Sarah Cohen-Boulakia - Alain Denise - Sylvie Hamel
Trajectory Simplification: On Minimizing the Direction-based Error
Cheng Long - Raymond Chi-Wing Wong - H V Jagadish
Selectivity Estimation on Streaming SpatioTextual Data Using Local Correlations
Xiaoyang Wang - Ying Zhang - Wenjie Zhang - Xuemin Lin - Wei Wang
Spatial Joins in Main Memory: Implementation Matters!
Darius Sidlauskas - Christian Jensen
Large Scale Real-time Ridesharing with Service Guarantee on Road Networks
Yan Huang - Favyen Bastani - Ruoming Jin - Xiaoyang Wang
Compressed Spatial Hierarchical Bitmap (cSHB) Indexes for Efficiently Processing Spatial Range Query Workloads
Parth Nagarkar - K. Selcuk Candan - Aneesha Bhat
Finding Patterns in a Knowledge Base using Keywords to Compose Table Answers
Mohan Yang - Bolin Ding - Surajit Chaudhuri - Kaushik Chakrabarti
Searchlight: Enabling Integrated Search and Exploration over Large Multidimensional Data
Alexander Kalinin - Ugur Cetintemel - Stan Zdonik
Processing Moving kNN Queries Using Influential Neighbor Sets
Chuanwen Li - Yu Gu - Jianzhong Qi - Ge Yu - Rui Zhang - Wang Yi
Reverse k Nearest Neighbors Query Processing: Experiments and Analysis
Shiyu Yang - Muhammad Cheema - Xuemin Lin - Wei Wang
Permutation Search Methods are Efficient, Yet Faster Search is Possible
Bilegsaikhan Naidan - Leonid Boytsov - Eric Nyberg
DREAM: Distributed RDF Engine with Adaptive Query Planner and Minimal Communication
Mohammad Hammoud - Dania Abed Rabbou - Reza Nouri - Seyed-Mehdi-Reza Beheshti - Sherif Sakr
Efficient Identification of Implicit Facts in Incomplete OWL2-EL Knowledge Bases
John Liagouris - Manolis Terrovitis
Taming Subgraph Isomorphism for RDF Query Processing
Jinha Kim - Hyungyu Shin - Wook-Shin Han - Sungpack Hong - Hassan Chafi
SEMA-JOIN : Joining Semantically-Related Tables Using Big Table Corpora
Yeye He - Kris Ganjam - Xu Chu
QuickFOIL: Scalable Inductive Logic Programming
Qiang Zeng - Jignesh Patel - David Page
ScalaGiST: Scalable Generalized Search Trees for MapReduce Systems
Peng Lu - Gang Chen - Beng Chin Ooi - Hoang Tam Vo - Sai Wu
DIADEM: Thousands of Websites to a Single Database
Tim Furche - Georg Gottlob - Giovanni Grasso - Xiaonan Guo - Giorgio Orsi - Christian Schallhart - Cheng Wang
AsterixDB: A Scalable, Open Source BDMS
Sattam Alsubaiee - Yasser Altowim - Hotham Altwaijry - Alex Behm - Vinayak Borkar - Yingyi Bu - Michael Carey - Inci Cetindil - Madhusudan Cheelangi - Khurram Faraaz - Eugenia Gabrielova - Raman Grover - Zachary Heilbron - Young-Seok Kim - Chen Li - Guangqiang Li - Ji Mahn Ok - Nicola Onose - Pouria Pirzadeh - Vassilis Tsotras - Rares Vernica - Jian Wen - Till Westmann
Mega-KV: A Case for GPUs to Maximize the Throughput of In-Memory Key-Value Stores
Kai Zhang - Kaibo Wang - Yuan Yuan - Lei Guo - Rubao Lee - Xiaodong Zhang
UDA-GIST: An In-database Framework to Unify Data-Parallel and State-Parallel Analytics
Kun Li - Daisy Zhe Wang - Alin Dobra - Chris Dudley
Auto-Approximation of Graph Computing
Zechao Shang - Jeffrey Xu Yu
Approximate lifted inference with probabilistic databases
Wolfgang Gatterbauer - Dan Suciu
Incremental Knowledge Base Construction Using DeepDive
Jaeho Shin - Sen Wu - Feiran Wang - Christopher De Sa - Ce Zhang - Christopher Re
Lenses: An On-Demand Approach to ETL
Ying Yang - Niccolo Meneghetti - Ronny Fehling - Zhen Hua Liu - Oliver Kennedy
Knowledge-Based Trust: A Method to Estimate the Trustworthiness of Web Sources
Xin Luna Dong - Evgeniy Gabrilovich - Kevin Murphy - Van Dang - Wilko Horn - Camillo Lugaresi - Shaohua Sun - Wei Zhang
DAQ: A New Paradigm for Approximate Query Processing
Navneet Potti - Jignesh Patel
On the Surprising Difficulty of Simple Things: the Case of Radix Partitioning
Felix Schuhknecht - Pankaj Khanchandani - Jens Dittrich
Efficient Processing of Window Functions in Analytical SQL Queries
Viktor Leis - Kan Kundhikanjana - Alfons Kemper - Thomas Neumann
Scaling Similarity Joins over Tree-Structured Data
Yu Tang - Yilun Cai - Nikos Mamoulis
Processing of Probabilistic Skyline Queries Using MapReduce
Yoonjae Park - Jun-Ki Min - Kyuseok Shim
Interpretable and Informative Explanations of Outcomes
Kareem El Gebaly - Parag Agrawal - Lukasz Golab - Flip Korn - Divesh Srivastava
Stale View Cleaning: Getting Fresh Answers from Stale Materialized Views
Sanjay Krishnan - Jiannan Wang - Michael Franklin - Ken Goldberg - Tim Kraska
Scalable Topical Phrase Mining from Text Corpora
Ahmed El-Kishky - Yanglei Song - Chi Wang - Clare Voss - Jiawei Han
Maximum Rank Query
Kyriakos Mouratidis - Jilian Zhang - HweeHwa Pang
A Confidence-Aware Approach for Truth Discovery on Long-Tail Data
Qi Li - Yaliang Li - Jing Gao - Lu Su - Bo Zhao - Murat Demirbas - Wei Fan - Jiawei Han
An Architecture for Compiling UDF-centric Workflows
Andrew Crotty - Alex Galakatos - Kayhan Dursun - Tim Kraska - Carsten Binnig - Ugur Cetintemel - Stan Zdonik
Take me to your leader! Online Optimization of Distributed Storage Configurations
Artyom Sharov - Alexander Shraer - Arif Merchant - Murray Stokely
SIMD- and Cache-Friendly Algorithm for Sorting an Array of Structures
Hiroshi Inoue - Kenjiro Taura
To Lock, Swap, or Elide: On the Interplay of Hardware Transactional Memory and Lock-Free Indexing
Darko Makreshanski - Justin Levandoski - Ryan Stutsman
SQLite Optimization with Phase Change Memory for Mobile Applications
Gihwan Oh - Sangchul Kim - Sang-Won Lee - Bongki Moon
Practical Authenticated Pattern Matching with Optimal Proof Size
Dimitrios Papadopoulos - Charalampos Papamanthou - Roberto Tamassia - Nikos Triandopoulos
Fast Range Query Processing with Strong Privacy Protection for Cloud Computing
Rui Li - Alex Liu - Ann Wang - Bezawada Bruhadeshwar
DPT: Differentially Private Trajectory Synthesis Using Hierarchical Reference Systems
Xi He - Graham Cormode - Ashwin Machanavajjhala - Cecilia Procopiuc - Divesh Srivastava
Privacy Implications of Database Ranking
Md Farhadur Rahman - Weimo Liu - Saravanan Thirumuruganathan - Nan Zhang - Gautam Das
Selective Provenance for Datalog Programs Using Top-k Queries
Daniel Deutch - Amir Gilad - Yuval Moskovitch
Asynchronous and Fault-Tolerant Recursive Datalog Evaluation in Shared-Nothing Engines
Jingjing Wang - Magdalena Balazinska - Daniel Halperin
Aggregate Estimations over Location Based Services
Weimo Liu - Md Farhadur Rahman - Saravanan Thirumuruganathan - Nan Zhang - Gautam Das
PARADIS: An Efficient Parallel Algorithm for In-place Radix Sort
Minsik Cho - Daniel Brand - Rajesh Bordawekar - Ulrich Finkler - Vincent Kulandaisamy - Ruchir Puri
Performance and Scalability of Indexed Subgraph Query Processing Methods
Foteini Katsarou - Nikos Ntarmos - Peter Triantafillou
Spatial Partitioning Techniques in SpatialHadoop
Ahmed Eldawy - Louai Alarabi - Mohamed Mokbel
Divide & Conquer-based Inclusion Dependency Discovery
Thorsten Papenbrock - Sebastian Kruse - Jorge-Arnulfo Quiane-Ruiz - Felix Naumann
Functional Dependency Discovery: An Experimental Evaluation of Seven Algorithms
Thorsten Papenbrock - Jens Ehrlich - jannik Marten - Tommy Neubert - Jan-Peer Rudolph - Martin Schˆnberg - Jakob Zwiener - Felix Naumann
Extraction of Logical Structure of Documents Based on Hierarchical Headings
Tomohiro Manabe - Keishi Tajima
Bonding Vertex Sets Over Distributed Graph: A Betweenness Aware Approach
Xiaofei Zhang - Hong Cheng - Lei Chen
CANDS: Continuous Optimal Navigation via Distributed Stream Processing
Dingyu Yang - Dongxiang Zhang - Kian-Lee Tan - Jian Cao - Frederic Le Mouel
General Incremental Sliding-Window Aggregation
Kanat Tangwongsan - Martin Hirzel - Scott Schneider - Kun-Lung Wu
YADING: Fast Clustering of Large-Scale Time Series Data
Rui Ding - Qiang Wang - Yingnong Dang - Qiang Fu - Haidong Zhang - Dongmei Zhang
Monitoring Distributed Streams using Convex Decompositions
Arnon Lazerson - Daniel Keren - Izchak Sharfman - Minos Garofalakis - Vasilis Samoladas - Assaf Schuste
Staring into the Abyss: An Evaluation of Concurrency Control with One Thousand Cores
Xiangyao Yu - George Bezerra - Andy Pavlo - Srinivas Devadas - Michael Stonebraker
E-Store: Fine-Grained Elastic Partitioning for Distributed Transaction Processing Systems
Rebecca Taft - Essam Mansour - Marco Serafini - Jennie Duggan - Aaron Elmore - Ashraf Aboulnaga - Andy Pavlo - Michael Stonebraker
ADDICT: Advanced Instruction Chasing for Transactions
Pinar Tozun - Islam Atta - Anastasia Ailamaki - Andrea Moshovos
Rethinking serializable multiversion concurrency control
Jose Faleiro - Daniel Abadi
Friday Sep 4th 08:00-09:00
Light Breakfast
Location: Grand Promenade
Friday Sep 4th 09:00-10:30
DMAH Session 1
Location: Kings 1
Chair: Fusheng Wang; Gang Luo; Chunhua Weng

First International Workshop on Data Management and Analytics for Medicine and Healthcare (DMAH 2015)
Fusheng Wang (Stony Brook University), Gang Luo (University of Utah), Chunhua Weng (Columbia University)
BPOE Session 1
Location: Kings 2
Chair: Jianfeng Zhan; Roberto V. Zicari; Rui Han

Sixth workshop on Big Data Benchmarks, Performance Optimization, and Emerging Hardware (BPOE-6)
Jianfeng Zhan (Chinese Academy of Sciences), Roberto V. Zicari (Goethe University), Rui Han (Chinese Academy of Sciences)
BOSS Session 1
Location: Queens 4-5-6
Chair: Tilmann Rabl

First workshop on Big Data Open Source System (BOSS 2015)
Tilmann Rabl, TU Berlin
Friday Sep 4th 10:30-11:00
Refreshments: Coffee Break
Location: Grand Promenade
Friday Sep 4th 11:00-12:30
DMAH Session 2
Location: Kings 1

First International Workshop on Data Management and Analytics for Medicine and Healthcare (DMAH 2015)
Fusheng Wang (Stony Brook University), Gang Luo (University of Utah), Chunhua Weng (Columbia University)
BPOE Session 2
Location: Kings 2

Sixth workshop on Big Data Benchmarks, Performance Optimization, and Emerging Hardware (BPOE-6)
Jianfeng Zhan (Chinese Academy of Sciences), Roberto V. Zicari (Goethe University), Rui Han (Chinese Academy of Sciences)
BOSS Session 2
Location: Queens 4-5-6

First workshop on Big Data Open Source System (BOSS 2015)
Tilmann Rabl, TU Berlin
Friday Sep 4th 12:30-14:00
Lunch
Location: Lagoon Lanai
Friday Sep 4th 14:00-15:00
DMAH Session 3
Location: Kings 1

First International Workshop on Data Management and Analytics for Medicine and Healthcare (DMAH 2015)
Fusheng Wang (Stony Brook University), Gang Luo (University of Utah), Chunhua Weng (Columbia University)
BPOE Session 3
Location: Kings 2

Sixth workshop on Big Data Benchmarks, Performance Optimization, and Emerging Hardware (BPOE-6)
Jianfeng Zhan (Chinese Academy of Sciences), Roberto V. Zicari (Goethe University), Rui Han (Chinese Academy of Sciences)
BOSS Session 3
Location: Queens 4-5-6

First workshop on Big Data Open Source System (BOSS 2015)
Tilmann Rabl, TU Berlin
Friday Sep 4th 15:00-15:30
Refreshments: Coffee Break
Location: Grand Promenade
Friday Sep 4th 15:30-18:00
DMAH Session 4
Location: Kings 1

First International Workshop on Data Management and Analytics for Medicine and Healthcare (DMAH 2015)
Fusheng Wang (Stony Brook University), Gang Luo (University of Utah), Chunhua Weng (Columbia University)
BPOE Session 4
Location: Kings 2

Sixth workshop on Big Data Benchmarks, Performance Optimization, and Emerging Hardware (BPOE-6)
Jianfeng Zhan (Chinese Academy of Sciences), Roberto V. Zicari (Goethe University), Rui Han (Chinese Academy of Sciences)
BOSS Session 4
Location: Queens 4-5-6

First workshop on Big Data Open Source System (BOSS 2015)
Tilmann Rabl, TU Berlin